08
Aug
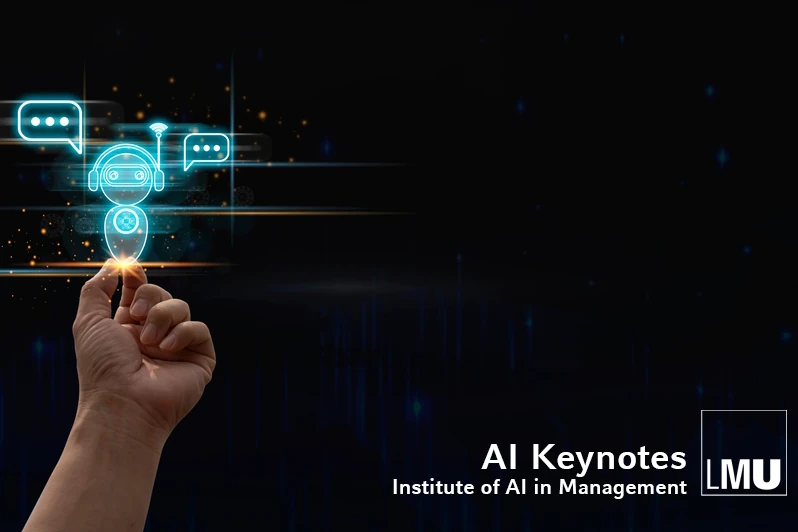
©jittawit.21 - stock.adobe.com
tba
Michael Oberst, Johns Hopkins University
08.08.2024
4:00 pm - 5:30 pm
Online via Zoom
Organized by:
Institute of AI in Management LMU Munich
Related
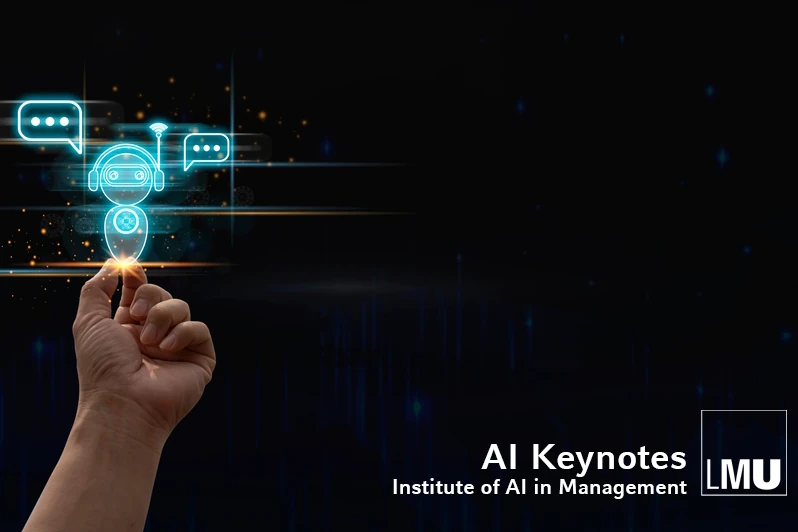
©jittawit.21 - stock.adobe.com
AI Keynote Series • 18.07.2024 • Online via Zoom
Representation Learning: A Causal Perspective
Lecture with Yixin Wang from University of Michigan, applying causal insights to create clear, efficient representations using counterfactuals.
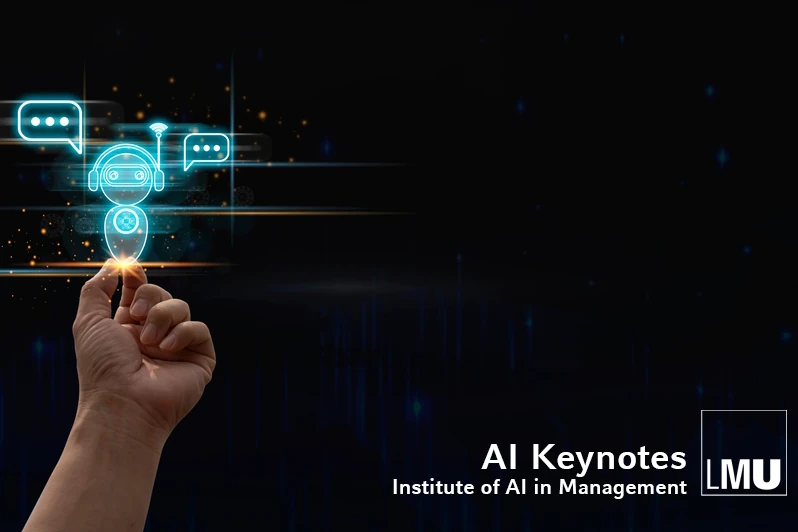
©jittawit.21 - stock.adobe.com
AI Keynote Series • 06.06.2024 • Online via Zoom
Interpretable prediction with missing values
Missing values in healthcare data hinder interpretability and predictions. Fredrik Johansson's talk presents two solutions and suggests future research directions.
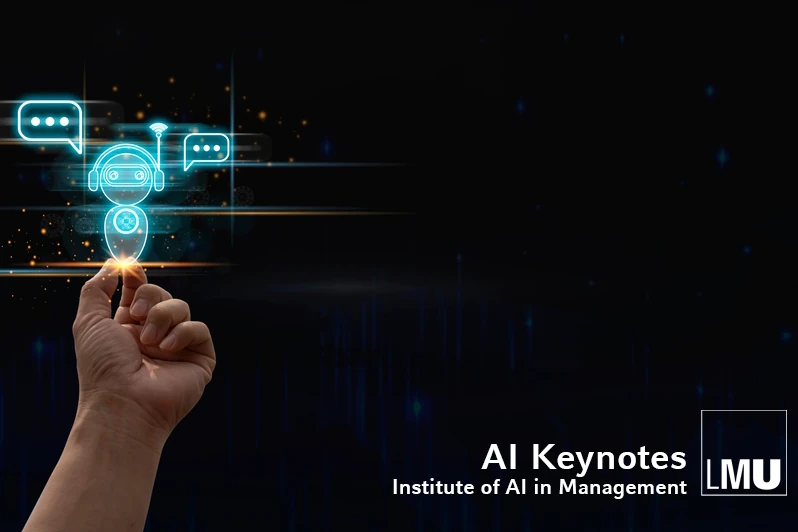
©jittawit.21 - stock.adobe.com
AI Keynote Series • 23.05.2024 • Online via Zoom
Innovating AI Products for Social Good in the Age of Foundational Models
Professor Qian Yang explores how LLMs necessitate considering societal impacts and innovating for social good in education and mental healthcare.
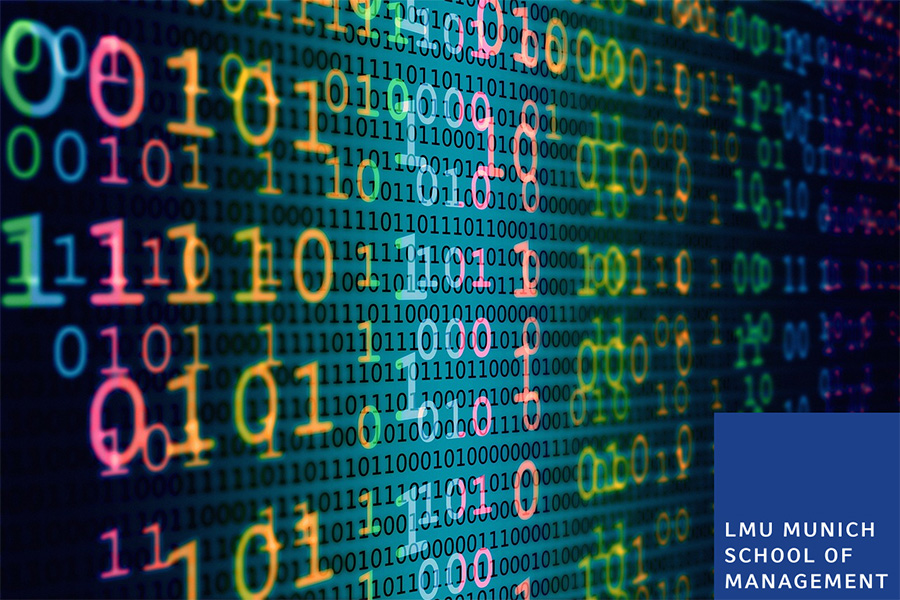
©geralt - pixabay.com
AI Keynote Series • 08.02.2024 • LMU Institute of AI in Management via zoom
Causal Scoring: A Framework for Effect Estimation, Effect Ordering, and Effect Classification
The presentation introduces causal scoring for decision-making, with interpretations.
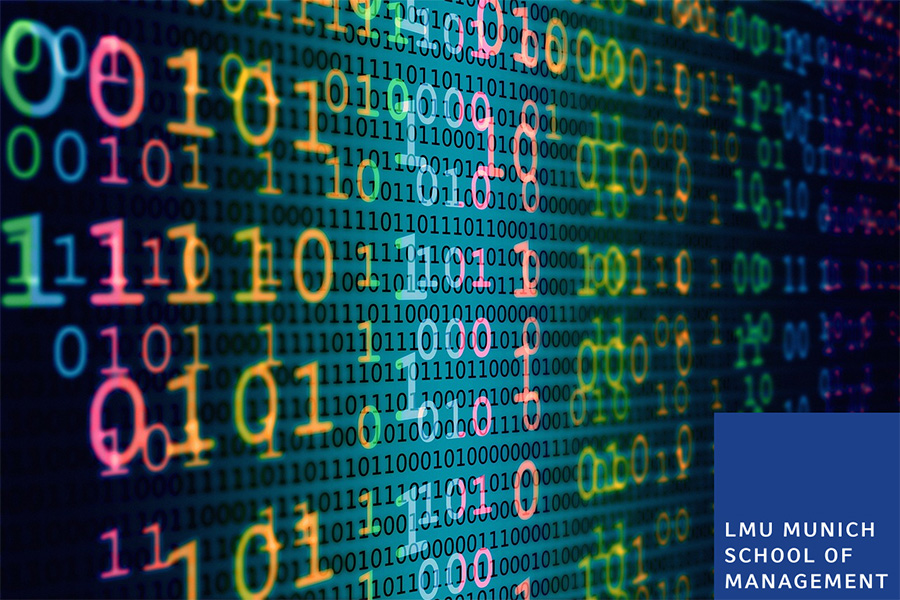
©geralt - pixabay.com
AI Keynote Series • 18.01.2024 • LMU Institute of AI in Management via zoom
Machine Learning Who to Nudge: Causal vs Predictive Targeting in a Field Experiment on Student Financial Aid Renewal
Join the presentation of Jann Spiess, Stanford Graduate School of Business, on the nuances of intervention effectiveness on targeting strategies.