18
Jul
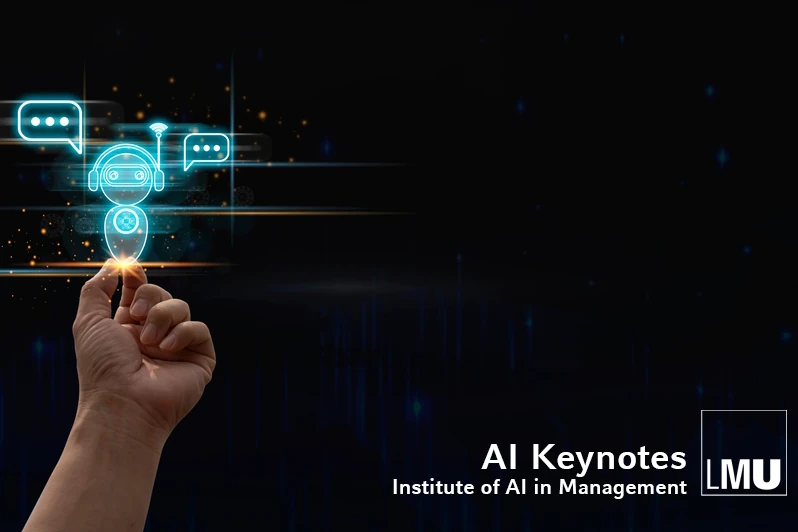
©jittawit.21 - stock.adobe.com
Representation Learning: A Causal Perspective
Yixin Wang, University of Michigan
18.07.2024
5:00 pm - 6:30 pm
Online via Zoom
Representation learning aims to create low-dimensional representations that capture essential features of high-dimensional data, such as images and texts. Ideally, these representations should efficiently capture meaningful, non-spurious features and be disentangled for interpretability. However, defining and enforcing these qualities is challenging.
In this talk, a causal perspective on representation learning is presented. The desiderata for effective representation learning are formalized using counterfactual concepts, which lead to metrics and algorithms designed to achieve efficient, non-spurious, and disentangled representations. The talk covers the theoretical foundations of the proposed algorithm and demonstrates its performance in both supervised and unsupervised settings.
Organized by:
Institute of AI in Management LMU Munich
Related
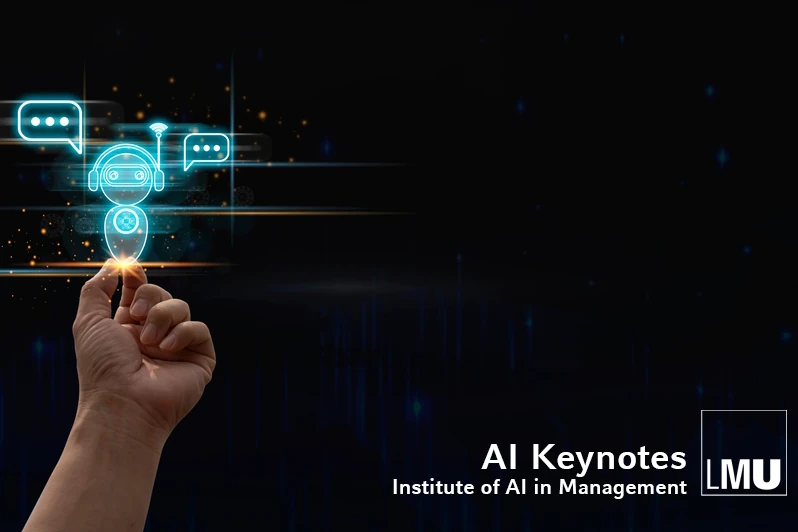
©jittawit.21 - stock.adobe.com
AI Keynote Series • 08.08.2024 • Online via Zoom
tba
Join the lecture with Michael Oberst from Johns Hopkins University.
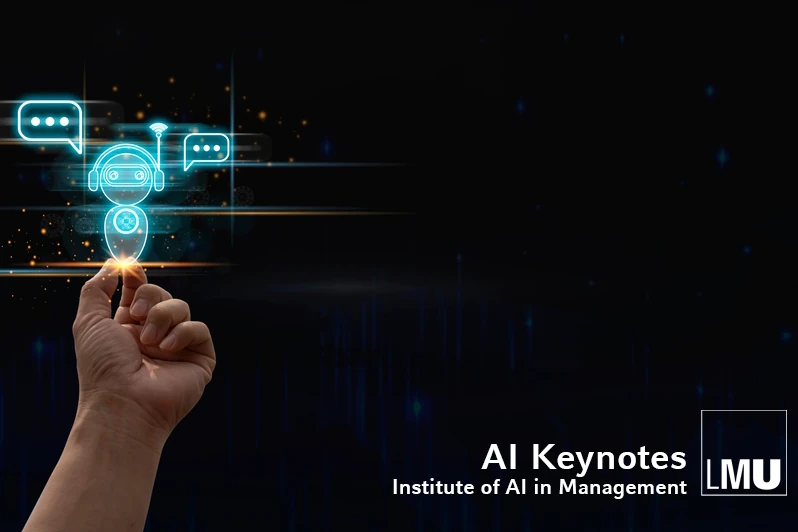
©jittawit.21 - stock.adobe.com
AI Keynote Series • 06.06.2024 • Online via Zoom
Interpretable prediction with missing values
Missing values in healthcare data hinder interpretability and predictions. Fredrik Johansson's talk presents two solutions and suggests future research directions.
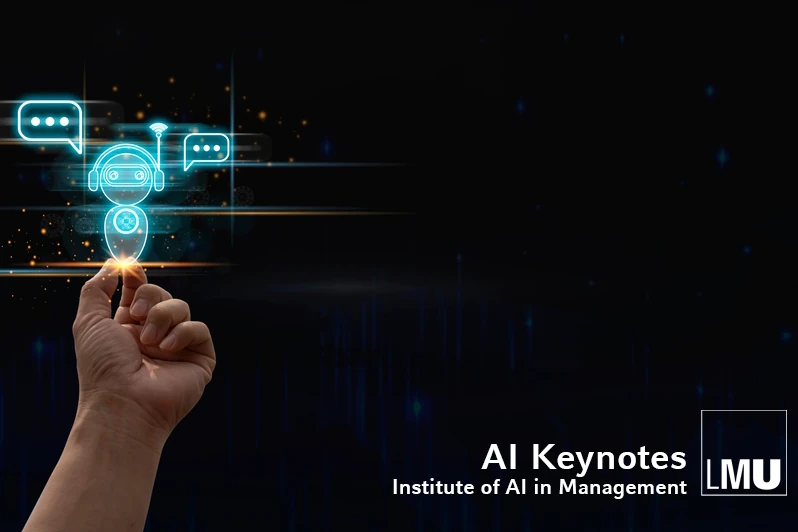
©jittawit.21 - stock.adobe.com
AI Keynote Series • 23.05.2024 • Online via Zoom
Innovating AI Products for Social Good in the Age of Foundational Models
Professor Qian Yang explores how LLMs necessitate considering societal impacts and innovating for social good in education and mental healthcare.
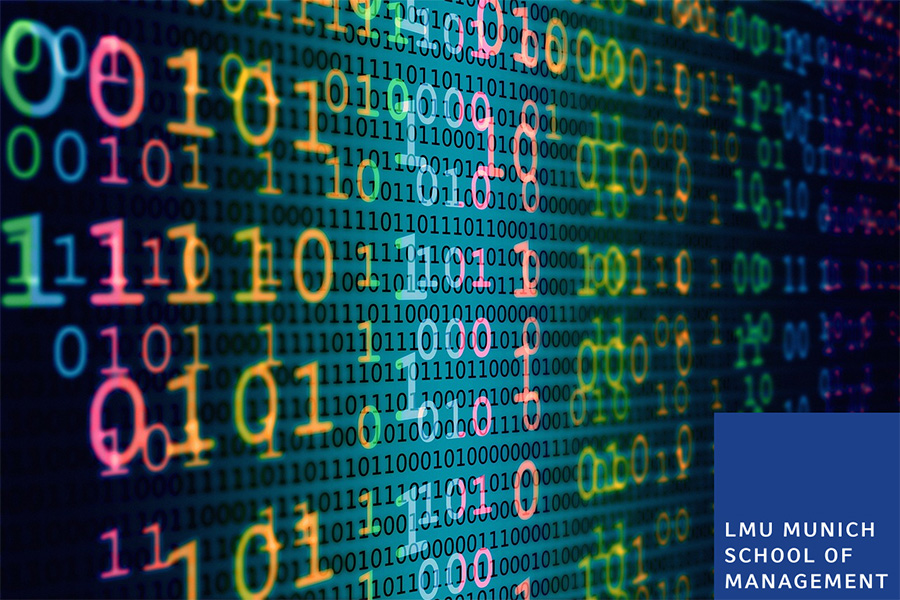
©geralt - pixabay.com
AI Keynote Series • 08.02.2024 • LMU Institute of AI in Management via zoom
Causal Scoring: A Framework for Effect Estimation, Effect Ordering, and Effect Classification
The presentation introduces causal scoring for decision-making, with interpretations.
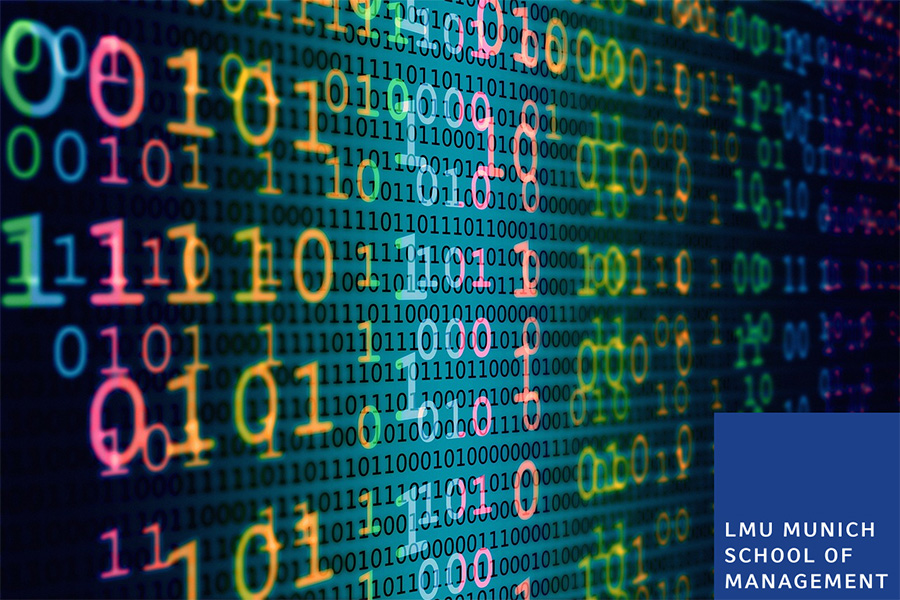
©geralt - pixabay.com
AI Keynote Series • 18.01.2024 • LMU Institute of AI in Management via zoom
Machine Learning Who to Nudge: Causal vs Predictive Targeting in a Field Experiment on Student Financial Aid Renewal
Join the presentation of Jann Spiess, Stanford Graduate School of Business, on the nuances of intervention effectiveness on targeting strategies.