06
Jun
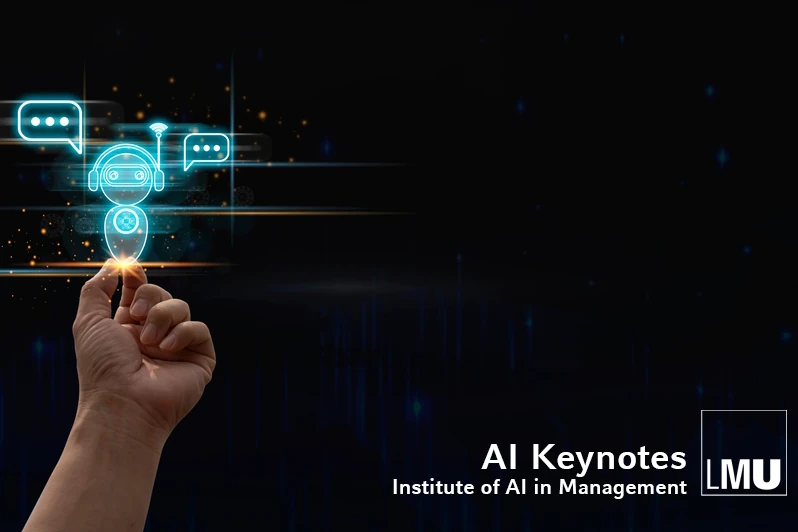
©jittawit.21 - stock.adobe.com
AI Keynote Series
Interpretable Prediction With Missing Values
Fredrik Johansson, Chalmers University of Technology
06.06.2024
12:00 pm - 1:30 pm
Online via Zoom
Missing values plague many application domains of machine learning, both in training data and in deployment. Healthcare is just one example—patient records are notorious for omissions of important variables and collecting them during clinical practice can be costly and time consuming. Healthcare also tends to demand interpretability so that predictions can be quickly calculated and justified, often using rule-based risk scores. Surprisingly, prediction with missing values and interpretability are largely incompatible using classical methods. Imputation obfuscates predictions and algorithms designed for interpretability typically have no native handling of prediction with missing values. In this talk, I will introduce two solutions to this problem, suitable under different conditions, and propose directions for future work.
Organized by:
LMU Munich, Institute of AI in Management