21
Jun
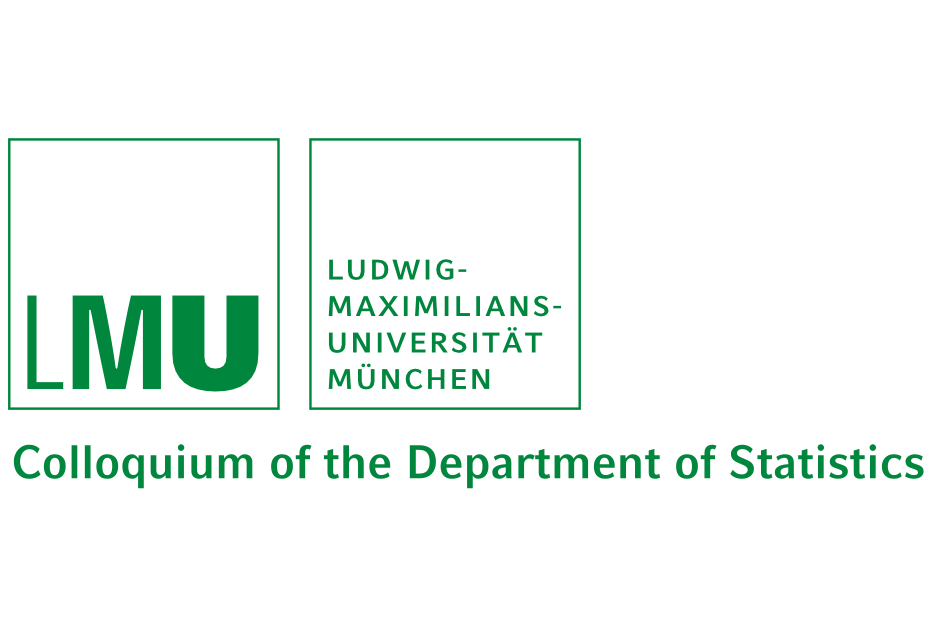
Rank-based support vector machines for highly imbalanced data using nominated samples
Mohammad Jafari Jozani, University of Manitoba, Winnipeg, Canada
21.06.2023
4:15 pm - 5:45 pm
LMU Department of Statistics and via zoom
The talk proposes a novel approach, MaxNS, that tackles highly imbalanced binary classification using expert opinions and rank information. Biasing training samples towards the minority class, it employs rank-based Hinge and Logistic loss functions.
Related
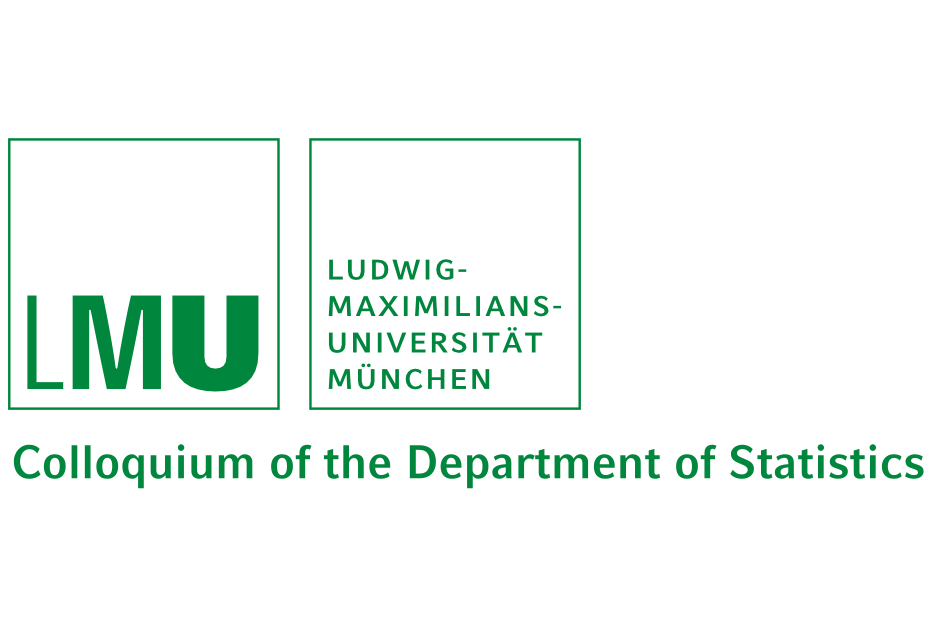
Colloquium • 16.07.2024 • LMU Department of Statistics and via zoom
Privacy, Data Privacy, and Differential Privacy
Colloquium at the LMU Department of Statistics with James Bailie from Harvard University.
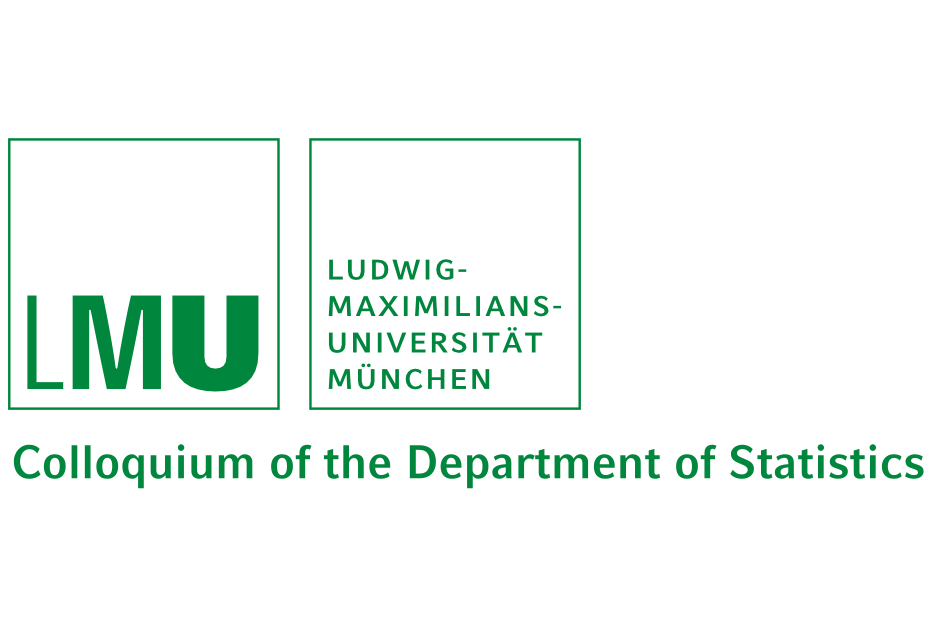
Colloquium • 10.07.2024 • LMU Department of Statistics and via zoom
Variational Learning for Large Deep Networks
Colloquium at the LMU Department of Statistics with Thomas Möllenhoff from RIKEN, Tokyo.
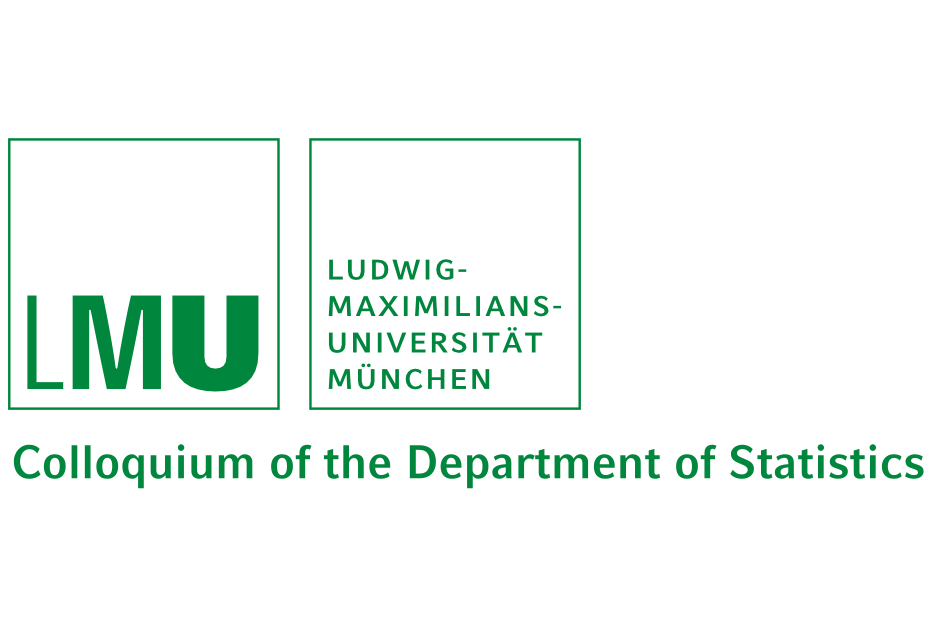
Colloquium • 03.07.2024 • LMU Department of Statistics and via zoom
Can today’s intention to treat have a causal effect on tomorrow’s hazard function?
Colloquium at the LMU Department of Statistics with Jan Beyersmann, University of Ulm.
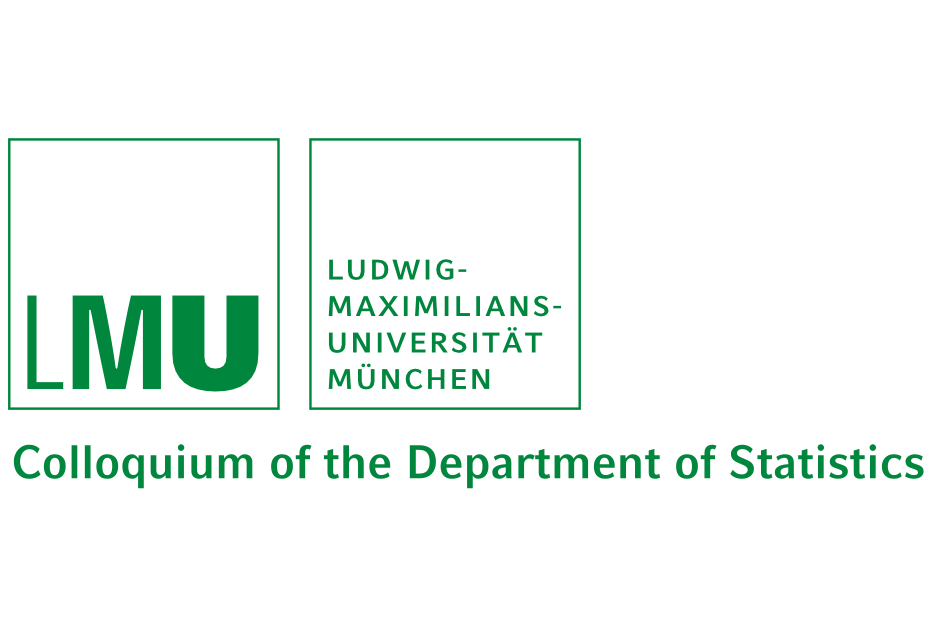
Colloquium • 26.06.2024 • LMU Department of Statistics and via zoom
The Complexities of Differential Privacy for Survey Data
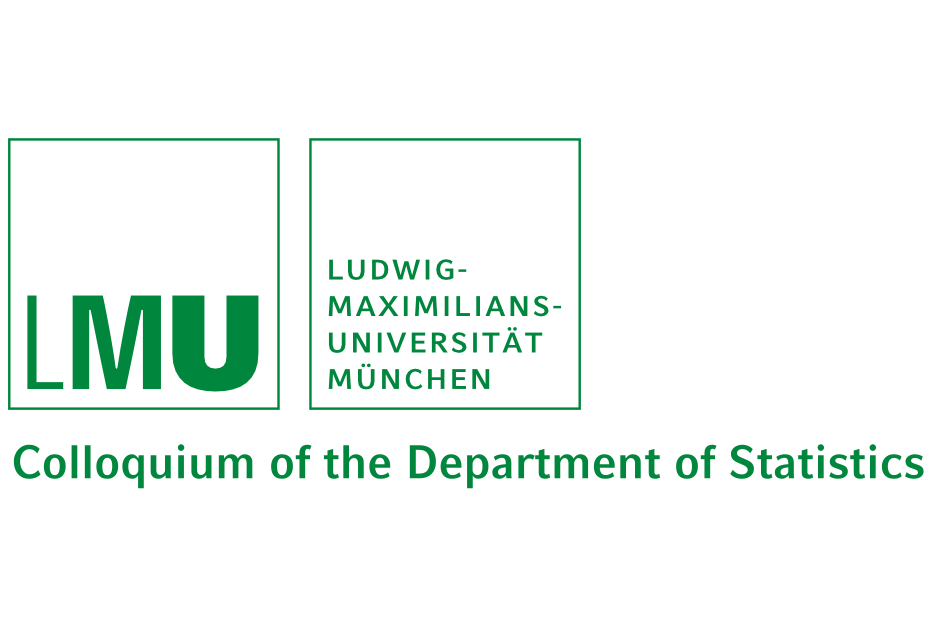
Colloquium • 19.06.2024 • LMU Department of Statistics and via zoom
Resampling-based inference for the average treatment effect in observational studies with competing risks
This talk explores three resampling methods to construct valid confidence intervals and bands for treatment effect estimation in competing risks studies.