10
Jul
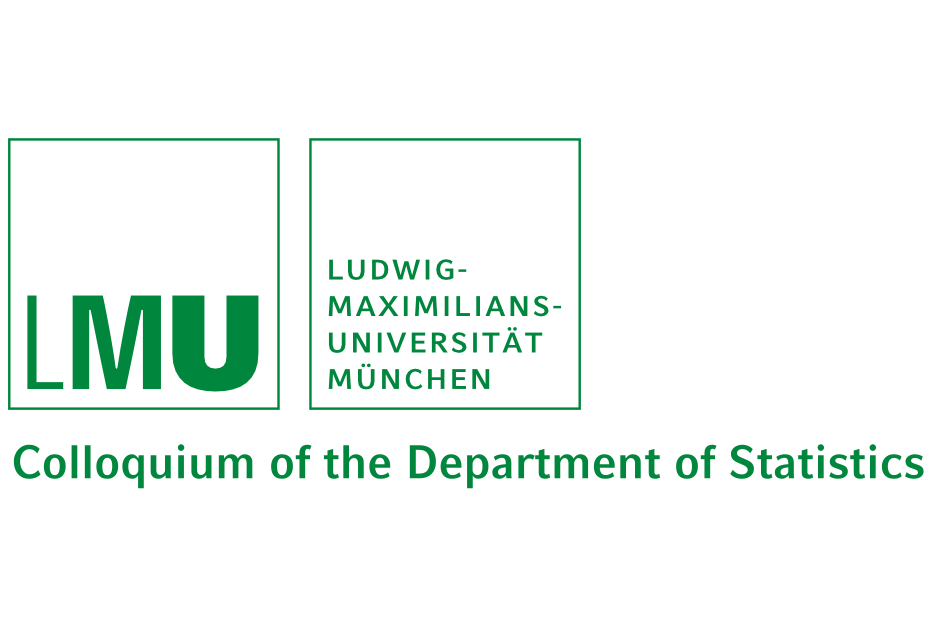
Colloquium
Variational Learning for Large Deep Networks
Thomas Möllenhoff, RIKEN, Tokyo
10.07.2024
3:15 pm - 4:45 pm
LMU Department of Statistics and via zoom
Thomas Möllenhoff presents extensive evidence against the common belief that variational Bayesian learning is ineffective for large neural networks.
First, he shows that a recent deep learning method called sharpness-aware minimization (SAM) solves an optimal convex relaxation of the variational Bayesian objective.
Then, he demonstrates that a direct optimization of the variational objective with an Improved Variational Online Newton method (IVON) can consistently match or outperforms Adam for training large networks such as GPT-2 and ResNets from scratch. IVON’s computational costs are nearly identical to Adam but its predictive uncertainty is better.
He shows several new use cases of variational learning where he improves fine-tuning and model merging in Large Language Models, accurately predict generalization error, and faithfully estimate sensitivity to data.
Organized by:
Department of Statistics LMU Munich