19
Jun
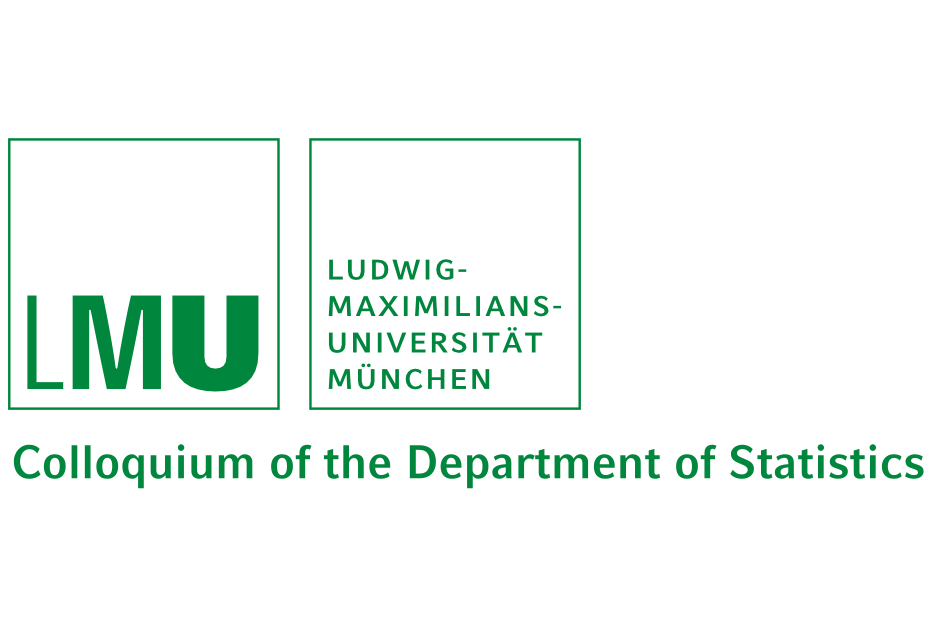
Colloquium
Resampling-Based Inference for the Average Treatment Effect in Observational Studies With Competing Risks
Sarah Friedrich, Universität Augsburg
19.06.2024
4:15 pm - 5:45 pm
LMU Department of Statistics and via zoom
In observational studies with time-to-event outcomes subject to competing risks, the g-formula can be used to estimate a treatment effect in the presence of confounding factors. The construction of valid pointwise confidence intervals and time-simultaneous confidence bands for the causal risk difference, however, is complicated. A convenient solution is to approximate the asymptotic distribution of the corresponding stochastic process by means of resampling approaches.
In this talk, we consider three different resampling methods, namely the classical nonparametric bootstrap, the influence function equipped with a resampling approach as well as a martingale-based bootstrap version, the so-called wild bootstrap. We compare these approaches with regard to asymptotic properties and based on simulation studies and demonstrate their usage in a data example.