01.01.2025
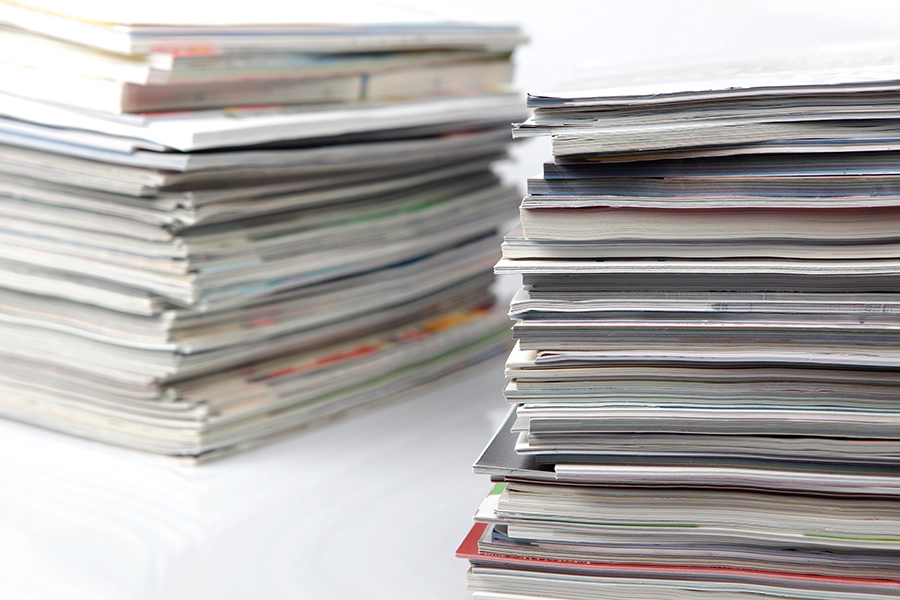
©Joachim Wendler - stock-adobe.com
53 Papers in Highly-Ranked Journals
We are happy to announce that MCML researchers are represented in 2025 with 53 papers in highly-ranked Journals. Congrats to our researchers!
Integrating Automated Electrochemistry and High-Throughput Characterization with Machine Learning to Explore Si─Ge─Sn Thin-Film Lithium Battery Anodes.
Advanced Energy Materials Early Access.2404961 (Jan. 2025). DOI
Abstract
High-performance batteries need accelerated discovery and optimization of new anode materials. Herein, we explore the Si─Ge─Sn ternary alloy system as a candidate fast-charging anode materials system by utilizing a scanning droplet cell (SDC) as an autonomous electrochemical characterization tool with the goal of subsequent upscaling. As the SDC is performing experiments sequentially, an exploration of the entire ternary space is unfeasible due to time constraints. Thus, closed-loop optimization, guided by real-time data analysis and sequential learning algorithms, is utilized to direct experiments. The lead material identified is scaled up to a coin cell to validate the findings from the autonomous millimeter-scale thin-film electrochemical experimentation. Explainable machine learning (ML) models incorporating data from high-throughput Raman spectroscopy and X-ray diffraction (XRD) are used to elucidate the effect of short and long-range ordering on material performance.
MCML Authors
Data-driven simulations to assess the impact of study imperfections in time-to-event analyses.
American Journal of Epidemiology 194.1 (Jan. 2025). DOI
Abstract
Quantitative bias analysis (QBA) permits assessment of the expected impact of various imperfections of the available data on the results and conclusions of a particular real-world study. This article extends QBA methodology to multivariable time-to-event analyses with right-censored endpoints, possibly including time-varying exposures or covariates. The proposed approach employs data-driven simulations, which preserve important features of the data at hand while offering flexibility in controlling the parameters and assumptions that may affect the results. First, the steps required to perform data-driven simulations are described, and then two examples of real-world time-to-event analyses illustrate their implementation and the insights they may offer. The first example focuses on the omission of an important time-invariant predictor of the outcome in a prognostic study of cancer mortality, and permits separating the expected impact of confounding bias from noncollapsibility. The second example assesses how imprecise timing of an interval-censored event—ascertained only at sparse times of clinic visits—affects its estimated association with a time-varying drug exposure. The simulation results also provide a basis for comparing the performance of two alternative strategies for imputing the unknown event times in this setting. The R scripts that permit the reproduction of our examples are provided.
MCML Authors
Biometry in Molecular Medicine
How robust is randomized blind deconvolution via nuclear norm minimization against adversarial noise?
Applied and Computational Harmonic Analysis 76.101746 (Apr. 2025). DOI
Abstract
In this paper, we study the problem of recovering two unknown signals from their convolution, which is commonly referred to as blind deconvolution. Reformulation of blind deconvolution as a low-rank recovery problem has led to multiple theoretical recovery guarantees in the past decade due to the success of the nuclear norm minimization heuristic. In particular, in the absence of noise, exact recovery has been established for sufficiently incoherent signals contained in lower-dimensional subspaces. However, if the convolution is corrupted by additive bounded noise, the stability of the recovery problem remains much less understood. In particular, existing reconstruction bounds involve large dimension factors and therefore fail to explain the empirical evidence for dimension-independent robustness of nuclear norm minimization. Recently, theoretical evidence has emerged for ill-posed behavior of low-rank matrix recovery for sufficiently small noise levels. In this work, we develop improved recovery guarantees for blind deconvolution with adversarial noise which exhibit square-root scaling in the noise level. Hence, our results are consistent with existing counterexamples which speak against linear scaling in the noise level as demonstrated for related low-rank matrix recovery problems.
MCML Authors
Mathematical Algorithm Design for Deep Learning under Societal and Judicial Constraints: The Algorithmic Transparency Requirement.
Applied and Computational Harmonic Analysis 77.101763 (Jun. 2025). DOI
Abstract
Deep learning still has drawbacks in terms of trustworthiness, which describes a comprehensible, fair, safe, and reliable method. To mitigate the potential risk of AI, clear obligations associated to trustworthiness have been proposed via regulatory guidelines, e.g., in the European AI Act. Therefore, a central question is to what extent trustworthy deep learning can be realized. Establishing the described properties constituting trustworthiness requires that the factors influencing an algorithmic computation can be retraced, i.e., the algorithmic implementation is transparent. Motivated by the observation that the current evolution of deep learning models necessitates a change in computing technology, we derive a mathematical framework which enables us to analyze whether a transparent implementation in a computing model is feasible. We exemplarily apply our trustworthiness framework to analyze deep learning approaches for inverse problems in digital and analog computing models represented by Turing and Blum-Shub-Smale Machines, respectively. Based on previous results, we find that Blum-Shub-Smale Machines have the potential to establish trustworthy solvers for inverse problems under fairly general conditions, whereas Turing machines cannot guarantee trustworthiness to the same degree.
MCML Authors
Mathematical Foundations of Artificial Intelligence
Technical note: Towards atmospheric compound identification in chemical ionization mass spectrometry with pesticide standards and machine learning.
Atmospheric Chemistry and Physics 25.1 (Jan. 2025). DOI
Abstract
Chemical ionization mass spectrometry (CIMS) is widely used in atmospheric chemistry studies. However, due to the complex interactions between reagent ions and target compounds, chemical understanding remains limited and compound identification difficult. In this study, we apply machine learning to a reference dataset of pesticides in two standard solutions to build a model that can provide insights from CIMS analyses in atmospheric science. The CIMS measurements were performed with an Orbitrap mass spectrometer coupled to a thermal desorption multi-scheme chemical ionization inlet unit (TD-MION-MS) with both negative and positive ionization modes utilizing Br−, , H3O+ and (CH3)2COH+ (AceH+) as reagent ions. We then trained two machine learning methods on these data: (1) random forest (RF) for classifying if a pesticide can be detected with CIMS and (2) kernel ridge regression (KRR) for predicting the expected CIMS signals. We compared their performance on five different representations of the molecular structure: the topological fingerprint (TopFP), the molecular access system keys (MACCS), a custom descriptor based on standard molecular properties (RDKitPROP), the Coulomb matrix (CM) and the many-body tensor representation (MBTR). The results indicate that MACCS outperforms the other descriptors. Our best classification model reaches a prediction accuracy of 0.85 ± 0.02 and a receiver operating characteristic curve area of 0.91 ± 0.01. Our best regression model reaches an accuracy of 0.44 ± 0.03 logarithmic units of the signal intensity. Subsequent feature importance analysis of the classifiers reveals that the most important sub-structures are NH and OH for the negative ionization schemes and nitrogen-containing groups for the positive ionization schemes.
MCML Authors
High-Dimensional Variable Selection With Competing Events Using Cooperative Penalized Regression.
Biometrical Journal 67.1 (Feb. 2025). DOI
Abstract
Variable selection is an important step in the analysis of high-dimensional data, yet there are limited options for survival outcomes in the presence of competing risks. Commonly employed penalized Cox regression considers each event type separately through cause-specific models, neglecting possibly shared information between them. We adapt the feature-weighted elastic net (fwelnet), an elastic net generalization, to survival outcomes and competing risks. For two causes, our proposed algorithm fits two alternating cause-specific models, where each model receives the coefficient vector of the complementary model as prior information. We dub this ‘‘cooperative penalized regression’’, as it enables the modeling of competing risk data with cause-specific models while accounting for shared effects between causes. Coefficients that are shrunken toward zero in the model for the first cause will receive larger penalization weights in the model for the second cause and vice versa. Through multiple iterations, this process ensures stronger penalization of uninformative predictors in both models. We demonstrate our method’s variable selection capabilities on simulated genomics data and apply it to bladder cancer microarray data. We evaluate selection performance using the positive predictive value for the correct selection of informative features and the false positive rate for the selection of uninformative variables. The benchmark compares results with cause-specific penalized Cox regression, random survival forests, and likelihood-boosted Cox regression. Results indicate that our approach is more effective at selecting informative features and removing uninformative features. In settings without shared effects, variable selection performance is similar to cause-specific penalized Cox regression.
MCML Authors
Statistical Learning and Data Science
Machine Learning Consulting Unit (MLCU)
To tweak or not to tweak. How exploiting flexibilities in gene set analysis leads to over-optimism.
Biometrical Journal 67.1 (Feb. 2025). DOI
Abstract
Gene set analysis, a popular approach for analyzing high-throughput gene expression data, aims to identify sets of genes that show enriched expression patterns between two conditions. In addition to the multitude of methods available for this task, users are typically left with many options when creating the required input and specifying the internal parameters of the chosen method. This flexibility can lead to uncertainty about the “right” choice, further reinforced by a lack of evidence-based guidance. Especially when their statistical experience is scarce, this uncertainty might entice users to produce preferable results using a ’trial-and-error’ approach. While it may seem unproblematic at first glance, this practice can be viewed as a form of ‘cherry-picking’ and cause an optimistic bias, rendering the results nonreplicable on independent data. After this problem has attracted a lot of attention in the context of classical hypothesis testing, we now aim to raise awareness of such overoptimism in the different and more complex context of gene set analyses. We mimic a hypothetical researcher who systematically selects the analysis variants yielding their preferred results, thereby considering three distinct goals they might pursue. Using a selection of popular gene set analysis methods, we tweak the results in this way for two frequently used benchmark gene expression data sets. Our study indicates that the potential for overoptimism is particularly high for a group of methods frequently used despite being commonly criticized. We conclude by providing practical recommendations to counter overoptimism in research findings in gene set analysis and beyond.
MCML Authors
Biometry in Molecular Medicine
Biometry in Molecular Medicine
Biometry in Molecular Medicine
Biometry in Molecular Medicine
Identification of the potential for roof greening using remote sensing and deep learning.
Cities 159.105782 (Apr. 2025). DOI
Abstract
Under the mounting pressure from global warming, green roofs emerge as a valuable source for climate adaptation, particularly in compact metropolises where green space is limited. Consequently, there is a need to quantitatively evaluate the potential for roof greening where it is most needed and suitable. Despite the increasing importance of this issue, there have been limited studies on the effectiveness of remote sensing and deep learning in identifying the potential for roof greening in many cities. To address this, we have created a GreenRoof dataset, comprising approximately 6400 pairs of remote sensing images and corresponding masks of roofs with high greening potential in four European cities. Afterward, we exploit the capabilities of deep learning methods to identify roofs that are suitable for greening from remote sensing images. Using 15 German cities as a case study for future urban rooftop planning, we estimate the spatial potential for retrofitting green roofs. Structural parameters for prioritizing green roof implementation include vegetation coverage, thermal environment, and building density. Results indicate that the total area suitable for green roof retrofitting exceeds 20% of the roof area in the 15 German cities examined. The spatial analysis effectively reflects variation in demand and suitability for green roof retrofitting across different cities. In conclusion, this study provides a versatile screening approach utilizing remote sensing, deep learning, and spatial analysis, which can be readily adapted to inform municipal policies in other cities aiming to promote green roofs and enhance sustainable urban development.
MCML Authors
A PDE Framework of Consensus-Based Optimization for Objectives with Multiple Global Minimizers.
Communications in Partial Differential Equations 50.4 (Feb. 2025). DOI
Abstract
Introduced in 2017, Consensus-Based Optimization (CBO) has rapidly emerged as a significant breakthrough in global optimization. This straightforward yet powerful multi-particle, zero-order optimization method draws inspiration from Simulated Annealing and Particle Swarm Optimization. Using a quantitative mean-field approximation, CBO dynamics can be described by a nonlinear Fokker-Planck equation with degenerate diffusion, which does not follow a gradient flow structure. In this paper, we demonstrate that solutions to the CBO equation remain positive and maintain full support. Building on this foundation, we establish the { unconditional} global convergence of CBO methods to global minimizers. Our results are derived through an analysis of solution regularity and the proof of existence for smooth, classical solutions to a broader class of drift-diffusion equations, despite the challenges posed by degenerate diffusion.
MCML Authors
ChatEarthNet: a global-scale image–text dataset empowering vision–language geo-foundation models.
Earth System Science Data 17.3 (Mar. 2025). DOI
Abstract
The rapid development of remote sensing technology has led to an exponential growth in satellite images, yet their inherent complexity often makes them difficult for non-expert users to understand. Natural language, as a carrier of human knowledge, can bridge the gap between common users and complicated satellite imagery. Additionally, when paired with visual data, natural language can be utilized to train large vision–language foundation models, significantly improving performance in various tasks. Despite these advancements, the remote sensing community still faces a challenge due to the lack of large-scale, high-quality vision–language datasets for satellite images. To address this challenge, we introduce a new image–text dataset, providing high-quality natural language descriptions for global-scale satellite data. Specifically, we utilize Sentinel-2 data for its global coverage as the foundational image source, employing semantic segmentation labels from the European Space Agency’s WorldCover project to enrich the descriptions of land cover types. By conducting in-depth semantic analysis, we formulate detailed prompts to elicit rich descriptions from ChatGPT. We then include a manual verification process to enhance the dataset’s quality further. This step involves manual inspection and correction to refine the dataset. Finally, we offer the community ChatEarthNet, a large-scale image–text dataset characterized by global coverage, high quality, wide-ranging diversity, and detailed descriptions. ChatEarthNet consists of 163 488 image–text pairs with captions generated by ChatGPT-3.5 and an additional 10 000 image–text pairs with captions generated by ChatGPT-4V(ision). This dataset has significant potential for both training and evaluating vision–language geo-foundation models for remote sensing. The code is publicly available at https://doi.org/10.5281/zenodo.11004358 (Yuan et al., 2024b), and the ChatEarthNet dataset is available at https://doi.org/10.5281/zenodo.11003436 (Yuan et al., 2024c).
MCML Authors
Data-driven dynamic police patrolling: An efficient Monte Carlo tree search.
European Journal of Operational Research 321.1 (Feb. 2025). DOI
Abstract
Crime is responsible for major financial losses and serious harm to the well-being of individuals, and, hence, a crucial task of police operations is effective patrolling. Yet, in existing decision models aimed at police operations, microscopic routing decisions from patrolling are not considered, and, furthermore, the objective is limited to surrogate metrics (e. g., response time) instead of crime prevention. In this paper, we thus formalize the decision problem of dynamic police patrolling as a Markov decision process that models microscopic routing decisions, so that the expected number of prevented crimes are maximized. We experimentally show that standard solution approaches for our decision problem are not scalable to real-world settings. As a remedy, we present a tailored and highly efficient Monte Carlo tree search algorithm. We then demonstrate our algorithm numerically using real-world crime data from Chicago and show that the decision-making by our algorithm offers significant improvements for crime prevention over patrolling tactics from current practice. Informed by our results, we finally discuss implications for improving the patrolling tactics in police operations.
MCML Authors
Artificial Intelligence in Management
A fused large language model for predicting startup success.
European Journal of Operational Research 322.1 (Apr. 2025). DOI
Abstract
Investors are continuously seeking profitable investment opportunities in startups and, hence, for effective decision-making, need to predict a startup’s probability of success. Nowadays, investors can use not only various fundamental information about a startup (e.g., the age of the startup, the number of founders, and the business sector) but also textual description of a startup’s innovation and business model, which is widely available through online venture capital (VC) platforms such as Crunchbase. To support the decision-making of investors, we develop a machine learning approach with the aim of locating successful startups on VC platforms. Specifically, we develop, train, and evaluate a tailored, fused large language model to predict startup success. Thereby, we assess to what extent self-descriptions on VC platforms are predictive of startup success. Using 20,172 online profiles from Crunchbase, we find that our fused large language model can predict startup success, with textual self-descriptions being responsible for a significant part of the predictive power. Our work provides a decision support tool for investors to find profitable investment opportunities.
MCML Authors
Artificial Intelligence in Management
Artificial Intelligence in Management
Replication study of PD-L1 status prediction in NSCLC using PET/CT radiomics.
European Journal of Radiology 183.111825 (Feb. 2025). DOI
Abstract
This study investigates the predictive capability of radiomics in determining programmed cell death ligand 1 (PD-L1) expression (>=1%) status in non-small cell lung cancer (NSCLC) patients using a newly collected [18F]FDG PET/CT dataset. We aimed to replicate and validate the radiomics-based machine learning (ML) model proposed by Zhao et al. [2] predicting PD-L1 status from PET/CT-imaging.
An independent cohort of 254 NSCLC patients underwent [18F]FDG PET/CT imaging, with primary tumor segmentation conducted using lung tissue window (LTW) and more conservative soft tissue window (STW) methods. Radiomics models (“Rad-score” and “complex model”) and a clinical-stage model from Zhao et al. were evaluated via 10-fold cross-validation and AUC analysis, alongside a benchmark-study comparing different ML-model pipelines. Clinicopathological data were collected from medical records.
On our data, the Rad-score model yielded mean AUCs of 0.593 (STW) and 0.573 (LTW), below Zhao et al.’s 0.761. The complex model achieved mean AUCs of 0.505 (STW) and 0.519 (LTW), lower than Zhao et al.’s 0.769. The clinical model showed a mean AUC of 0.555, below Zhao et al.’s 0.64. All models performed significantly lower than Zhao et al.’s findings. Our benchmark study on four ML pipelines revealed consistently low performance across all configurations.
Our study failed to replicate original findings, suggesting poor model performance and questioning predictive value of radiomics features in classifying PD-L1 expression from PET/CT imaging. These results highlight challenges in replicating radiomics-based ML models and stress the need for rigorous validation
MCML Authors
Optimizing lower extremity CT angiography: A prospective study of individualized vs. fixed post-trigger delays in bolus tracking.
European Journal of Radiology 185.112009 (Apr. 2025). DOI
Abstract
Purpose: To compare the contrast media opacification and diagnostic quality in lower-extremity runoff CT angiography (CTA) between bolus-tracking using conventional fixed trigger delay and patient-specific individualized post-trigger delay.
Methods: In this prospective study, lower-extremity runoff CTA was performed in two cohorts, using either fixed or individualized trigger delay. Both cohorts had identical CT protocols, contrast media applications, and image reconstructions. Objective image quality (mean contrast opacification in HU), and subjective image quality (5-point Likert-scale), were assessed in six vessels: abdominal aorta (AA), common iliac artery (CIA), superficial femoral artery (SFA), popliteal artery (PA), posterior tibial artery (PTA), and dorsalis pedis artery (DPA) by one rater for objective and two raters for subjective image quality. Objective image quality was analyzed using Student t-tests, while subjective ratings were compared with Fisher’s exact test.
Results: Overall, 65 patients were included (mean age: 71 ± 14; 39 men), 35 in the individualized cohort and 30 in the fixed cohort. No differences were found between the groups regarding demographics or radiation exposure. Individualized trigger delay ranged from 2 to 23 s (mean: 8.7 ± 4.0 s) and was 10 s in the fixed cohort. The individualized cohort showed higher opacification in the peripheral arteries (PTA: 479 ± 140 HU vs. 379 ± 106 HU; p = 0.009; DPA: 477 ± 191 HU vs. 346 ± 137 HU; p = 0.009). Overall subjective “image quality” was rated higher in the individualized group (“excellent” or “good” in Rater 1: 97% vs. 57%; p < 0.001; and Rater 2: 89% vs. 53%; p = 0.002).
Conclusion: Individualized post-trigger delay enhances diagnostic quality, by improving vessel opacification in peripheral arteries and increasing subjective image quality in lower extremity runoff CTA.
MCML Authors
Effect of artificial intelligence-aided differentiation of adenomatous and non-adenomatous colorectal polyps at CT colonography on radiologists’ therapy management.
European Radiology Early Access (Jan. 2025). DOI
Abstract
Objectives: Adenomatous colorectal polyps require endoscopic resection, as opposed to non-adenomatous hyperplastic colorectal polyps. This study aims to evaluate the effect of artificial intelligence (AI)-assisted differentiation of adenomatous and non-adenomatous colorectal polyps at CT colonography on radiologists’ therapy management.
Materials and methods: Five board-certified radiologists evaluated CT colonography images with colorectal polyps of all sizes and morphologies retrospectively and decided whether the depicted polyps required endoscopic resection. After a primary unassisted reading based on current guidelines, a second reading with access to the classification of a radiomics-based random-forest AI-model labelling each polyp as ’non-adenomatous’ or ‘adenomatous’ was performed. Performance was evaluated using polyp histopathology as the reference standard.
Results: 77 polyps in 59 patients comprising 118 polyp image series (47% supine position, 53% prone position) were evaluated unassisted and AI-assisted by five independent board-certified radiologists, resulting in a total of 1180 readings (subsequent polypectomy: yes or no). AI-assisted readings had higher accuracy (76% +/− 1% vs. 84% +/− 1%), sensitivity (78% +/− 6% vs. 85% +/− 1%), and specificity (73% +/− 8% vs. 82% +/− 2%) in selecting polyps eligible for polypectomy (p < 0.001). Inter-reader agreement was improved in the AI-assisted readings (Fleiss’ kappa 0.69 vs. 0.92).
Conclusion: AI-based characterisation of colorectal polyps at CT colonography as a second reader might enable a more precise selection of polyps eligible for subsequent endoscopic resection. However, further studies are needed to confirm this finding and histopathologic polyp evaluation is still mandatory.
MCML Authors
Automating airborne pollen classification: Identifying and interpreting hard samples for classifiers.
Heliyon 11.2 (Jan. 2025). DOI GitHub
Abstract
Deep-learning-based classification of pollen grains has been a major driver towards automatic monitoring of airborne pollen. Yet, despite an abundance of available datasets, little effort has been spent to investigate which aspects pose the biggest challenges to the (often black-box- resembling) pollen classification approaches. To shed some light on this issue, we conducted a sample-level difficulty analysis based on the likelihood for one of the largest automatically-generated datasets of pollen grains on microscopy images and investigated the reason for which certain airborne samples and specific pollen taxa pose particular problems to deep learning algorithms. It is here concluded that the main challenges lie in A) the (partly) co-occurring of multiple pollen grains in a single image, B) the occlusion of specific markers through the 2D capturing of microscopy images, and C) for some taxa, a general lack of salient, unique features.
MCML Authors
An On-Board Executable Multi-Feature Transfer-Enhanced Fusion Model for Three-Lead EEG Sensor-Assisted Depression Diagnosis.
IEEE Journal of Biomedical and Health Informatics 29.1 (Jan. 2025). DOI
Abstract
The development of affective computing and medical electronic technologies has led to the emergence of Artificial Intelligence (AI)-based methods for the early detection of depression. However, previous studies have often overlooked the necessity for the AI-assisted diagnosis system to be wearable and accessible in practical scenarios for depression recognition. In this work, we present an on-board executable multi-feature transfer-enhanced fusion model for our custom-designed wearable three-lead Electroencephalogram (EEG) sensor, based on EEG data collected from 73 depressed patients and 108 healthy controls. Experimental results show that the proposed model exhibits low-computational complexity (65.0 K parameters), promising Floating-Point Operations (FLOPs) performance (25.6 M), real-time processing (1.5 s/execution), and low power consumption (320.8 mW). Furthermore, it requires only 202.0 KB of Random Access Memory (RAM) and 279.6 KB of Read-Only Memory (ROM) when deployed on the EEG sensor. Despite its low computational and spatial complexity, the model achieves a notable classification accuracy of 95.2%, specificity of 94.0%, and sensitivity of 96.9% under independent test conditions. These results underscore the potential of deploying the model on the wearable three-lead EEG sensor for assisting in the diagnosis of depression.
MCML Authors
A Review of AIoT-Based Human Activity Recognition: From Application to Technique.
IEEE Journal of Biomedical and Health Informatics 29.4 (Apr. 2025). DOI
Abstract
This scoping review paper redefines the Artificial Intelligence-based Internet of Things (AIoT) driven Human Activity Recognition (HAR) field by systematically extrapolating from various application domains to deduce potential techniques and algorithms. We distill a general model with adaptive learning and optimization mechanisms by conducting a detailed analysis of human activity types and utilizing contact or non-contact devices. It presents various system integration mathematical paradigms driven by multimodal data fusion, covering predictions of complex behaviors and redefining valuable methods, devices, and systems for HAR. Additionally, this paper establishes benchmarks for behavior recognition across different application requirements, from simple localized actions to group activities. It summarizes open research directions, including data diversity and volume, computational limitations, interoperability, real-time recognition, data security, and privacy concerns. Finally, we aim to serve as a comprehensive and foundational resource for researchers delving into the complex and burgeoning realm of AIoT-enhanced HAR, providing insights and guidance for future innovations and developments.
MCML Authors
Toward Integrating ChatGPT Into Satellite Image Annotation Workflows: A Comparison of Label Quality and Costs of Human and Automated Annotators.
IEEE Journal of Selected Topics in Applied Earth Observations and Remote Sensing 18 (Jan. 2025). DOI
Abstract
High-quality annotations are a critical success factor for machine learning (ML) applications. To achieve this, we have
traditionally relied on human annotators, navigating the challenges of limited budgets and the varying task-specific expertise, costs, and availability. Since the emergence of Large Language Models (LLMs), their popularity for generating automated annotations has grown, extending possibilities and complexity of designing an efficient annotation strategy. Increasingly, computer vision capabilities have been integrated into general-purpose LLMs like ChatGPT. This raises the question of how effectively LLMs can be used in satellite image annotation tasks and how they compare to traditional annotator types. This study presents a comprehensive investigation and comparison of various human and automated annotators for image classification. We evaluate the feasibility and economic competitiveness of using the ChatGPT4-V model for a complex land usage annotation task and compare it with alternative human annotators. A set of satellite images is annotated by a domain expert and 15 additional human and automated annotators, differing in expertise and costs. Our analyses examine the annotation quality loss between the expert and other annotators. This comparison is conducted through (1) descriptive analyses, (2) fitting linear probability models, and (3) comparing F1-scores. Ultimately, we simulate annotation strategies where samples are split according to an automatically assigned certainty score. Routing low-certainty images to human annotators can cut total annotation costs by over 50% with minimal impact on label quality. We discuss implications regarding the economic competitiveness of annotation strategies, prompt engineering and the task-specificity of expertise.
MCML Authors
Gaze-Guided Robotic Vascular Ultrasound Leveraging Human Intention Estimation.
IEEE Robotics and Automation Letters 10.4 (Apr. 2025). DOI
Abstract
Medical ultrasound has been widely used to examine vascular structure in modern clinical practice. However, traditional ultrasound examination often faces challenges related to inter- and intra-operator variation. The robotic ultrasound system (RUSS) appears as a potential solution for such challenges because of its superiority in stability and reproducibility. Given the complex anatomy of human vasculature, multiple vessels often appear in ultrasound images, or a single vessel bifurcates into branches, complicating the examination process. To tackle this challenge, this work presents a gaze-guided RUSS for vascular applications. A gaze tracker captures the eye movements of the operator. The extracted gaze signal guides the RUSS to follow the correct vessel when it bifurcates. Additionally, a gaze-guided segmentation network is proposed to enhance segmentation robustness by exploiting gaze information. However, gaze signals are often noisy, requiring interpretation to accurately discern the operator’s true intentions. To this end, this study proposes a stabilization module to process raw gaze data. The inferred attention heatmap is utilized as a region proposal to aid segmentation and serve as a trigger signal when the operator needs to adjust the scanning target, such as when a bifurcation appears. To ensure appropriate contact between the probe and surface during scanning, an automatic ultrasound confidence-based orientation correction method is developed. In experiments, we demonstrated the efficiency of the proposed gaze-guided segmentation pipeline by comparing it with other methods. Besides, the performance of the proposed gaze-guided RUSS was also validated as a whole on a realistic arm phantom with an uneven surface.
MCML Authors
Computer Aided Medical Procedures & Augmented Reality
Improving Audio Explanations using Audio Language Models.
IEEE Signal Processing Letters Early Access (Jan. 2025). DOI
Abstract
Foundation models are widely utilised for their strong representational capabilities, driven by training on extensive datasets with self-supervised learning. The increasing complexity of these models highlights the importance of interpretability to enhance transparency and improve human understanding of their decision-making processes. Most existing interpretability methods explain model behaviour by attributing importance to individual data elements across different layers, based on their influence on the final prediction. These approaches often emphasise only the most relevant features, overlooking the broader representational space, removing less important features. In this study, we propose a novel framework for explanation generation that serves as an alternative to feature removal, offering a more comprehensive understanding of model behaviour. Our framework leverages the generative abilities of audio language models to replace removed features with contextually appropriate alternatives, providing a more complete view of the model’s decision-making process. Through extensive evaluations on standard benchmarks, including keyword spotting and speech emotion recognition, our approach demonstrates its effectiveness in generating high-quality audio explanations.
MCML Authors
Improving Bird Vocalization Recognition in Open-Set Cross-Corpus Scenarios with Semantic Feature Reconstruction and Dual Strategy Scoring.
IEEE Signal Processing Letters Early Access (Mar. 2025). DOI
Abstract
Automated recognition of bird vocalizations (BVs) is essential for biodiversity monitoring through passive acoustic monitoring (PAM), yet deep learning (DL) models encounter substantial challenges in open environments. These include difficulties in detecting unknown classes, extracting species-specific features, and achieving robust cross-corpus recognition. To address these challenges, this letter presents a DL-based open-set cross-corpus recognition method for BVs that combines feature construction with open-set recognition (OSR) techniques. We introduce a three-channel spectrogram that integrates both amplitude and phase information to enhance feature representation. To improve the recognition accuracy of known classes across corpora, we employ a class-specific semantic reconstruction model to extract deep features. For unknown class discrimination, we propose a Dual Strategy Coupling Scoring (DSCS) mechanism, which synthesizes the log-likelihood ratio score (LLRS) and reconstruction error score (RES). Our method achieves the highest weighted accuracy among existing approaches on a public dataset, demonstrating its effectiveness for open-set cross-corpus bird vocalization recognition.
MCML Authors
Enhancing Emotion Regulation in Mental Disorder Treatment: An AIGC-based Closed-Loop Music Intervention System.
IEEE Transactions on Affective Computing Early Access (Apr. 2025). DOI
Abstract
Mental disorders have increased rapidly and have emerged as a serious social health issue in the recent decade. Undoubtedly, the timely treatment of mental disorders is crucial. Emotion regulation has been proven to be an effective method for treating mental disorders. Music therapy as one of the methods that can achieve emotional regulation has gained increasing attention in the field of mental disorder treatment. However, traditional music therapy methods still face some unresolved issues, such as the lack of real-time capability and the inability to form closed-loop systems. With the advancement of artificial intelligence (AI), especially AI-generated content (AIGC), AI-based music therapy holds promise in addressing these issues. In this paper, an AIGC-based closed-loop music intervention system demonstration is proposed to regulate emotions for mental disorder treatment. This system demonstration consists of an emotion recognition model and a music generation model. The emotion recognition model can assess mental states, while the music generation model generates the corresponding emotional music for regulation. The system continuously performs recognition and regulation, thus forming a closed-loop process. In the experiment, we first conduct experiments on both the emotion recognition model and the music generation model to validate the accuracy of the recognition model and the music quality generated by the music generation models. In conclusion, we conducted comprehensive tests on the entire system to verify its feasibility and effectiveness.
MCML Authors
Weakly-Supervised Depression Detection in Speech Through Self-Learning Based Label Correction.
IEEE Transactions on Audio, Speech and Language Processing Early Access (Jan. 2025). DOI
Abstract
Automated Depression Detection (ADD) in speech aims to automatically estimate one’s depressive attributes through artificial intelligence tools towards spoken signals. Nevertheless, existing speech-based ADD works fail to sufficiently consider weakly-supervised cases with inaccurate labels, which may typically appear in intelligent mental health. In this regard, we propose the Self-Learning-based Label Correction (SLLC) approach for weakly-supervised depression detection in speech. The proposed approach employs a self-learning manner connecting a label correction module and a depression detection module. Within the approach, the label correction module fuses likelihood-ratio-based and prototype-based label correction strategies in order to effectively correct the inaccurate labels, while the depression detection module aims at detecting depressed samples through a 1D convolutional recurrent neural network with multiple types of losses. The experimental results on two depression detection corpora show that our proposed SLLC approach performs better compared with existing state-of-the-art speech-based depression detection approaches, in the case of weak supervision with inaccurate labels for depression detection in speech.
MCML Authors
Semi-Supervised Building Footprint Extraction Using Debiased Pseudo-Labels.
IEEE Transactions on Geoscience and Remote Sensing 63 (Jan. 2025). DOI GitHub
Abstract
Accurate extraction of building footprints from satellite imagery is of high value. Currently, deep learning methods are predominant in this field due to their powerful representation capabilities. However, they generally require extensive pixel-wise annotations, which constrains their practical application. Semi-supervised learning (SSL) significantly mitigates this requirement by leveraging large volumes of unlabeled data for model self-training (ST), thus enhancing the viability of building footprint extraction. Despite its advantages, SSL faces a critical challenge: the imbalanced distribution between the majority background class and the minority building class, which often results in model bias toward the background during training. To address this issue, this article introduces a novel method called DeBiased matching (DBMatch) for semi-supervised building footprint extraction. DBMatch comprises three main components: 1) a basic supervised learning module (SUP) that uses labeled data for initial model training; 2) a classical weak-to-strong ST module that generates pseudo-labels from unlabeled data for further model ST; and 3) a novel logit debiasing (LDB) module that calculates a global logit bias between building and background, allowing for dynamic pseudo-label calibration. To verify the effectiveness of the proposed DBMatch, extensive experiments are performed on three public building footprint extraction datasets covering six global cities in SSL setting. The experimental results demonstrate that our method significantly outperforms some advanced SSL methods in semi-supervised building footprint extraction.
MCML Authors
CromSS: Cross-Modal Pretraining With Noisy Labels for Remote Sensing Image Segmentation.
IEEE Transactions on Geoscience and Remote Sensing 63 (Mar. 2025). DOI GitHub
Abstract
We explore the potential of large-scale noisily labeled data to enhance feature learning by pretraining semantic segmentation models within a multimodal framework for geospatial applications. We propose a novel cross-modal sample selection (CromSS) method, a weakly supervised pretraining strategy designed to improve feature representations through cross-modal consistency and noise mitigation techniques. Unlike conventional pretraining approaches, CromSS exploits massive amounts of noisy and easy-to-come-by labels for improved feature learning beneficial to semantic segmentation tasks. We investigate middle and late fusion strategies to optimize the multimodal pretraining architecture design. We also introduce a cross-modal sample selection module to mitigate the adverse effects of label noise, which employs a cross-modal entangling strategy to refine the estimated confidence masks within each modality to guide the sampling process. Additionally, we introduce a spatial–temporal label smoothing technique to counteract overconfidence for enhanced robustness against noisy labels. To validate our approach, we assembled the multimodal dataset, NoLDO-S12, which consists of a large-scale noisy label subset from Google’s Dynamic World (DW) dataset for pretraining and two downstream subsets with high-quality labels from Google DW and OpenStreetMap (OSM) for transfer learning. Experimental results on two downstream tasks and the publicly available DFC2020 dataset demonstrate that when effectively utilized, the low-cost noisy labels can significantly enhance feature learning for segmentation tasks.
MCML Authors
Hybrid Quantum Deep Learning With Superpixel Encoding for Earth Observation Data Classification.
IEEE Transactions on Neural Networks and Learning Systems Early Access (Jan. 2025). DOI URL
Abstract
Earth observation (EO) has inevitably entered the Big Data era. The computational challenge associated with analyzing large EO data using sophisticated deep learning models has become a significant bottleneck. To address this challenge, there has been a growing interest in exploring quantum computing as a potential solution. However, the process of encoding EO data into quantum states for analysis potentially undermines the efficiency advantages gained from quantum computing. This article introduces a hybrid quantum deep learning model that effectively encodes and analyzes EO data for classification tasks. The proposed model uses an efficient encoding approach called superpixel encoding, which reduces the quantum resources required for large image representation by incorporating the concept of superpixels. To validate the effectiveness of our model, we conducted evaluations on multiple EO benchmarks, including Overhead-MNIST, So2Sat LCZ42, and SAT-6 datasets. In addition, we studied the impacts of different interaction gates and measurements on classification performance to guide model optimization. The experimental results suggest the validity of our model for accurate classification of EO data.
MCML Authors
Explainable Depression Classification Based on EEG Feature Selection from Audio Stimuli.
IEEE Transactions on Neural Systems and Rehabilitation Engineering Early Access (Apr. 2025). DOI
Abstract
With the development of affective computing and Artificial Intelligence (AI) technologies, Electroencephalogram (EEG)-based depression detection methods have been widely proposed. However, existing studies have mostly focused on the accuracy of depression recognition, ignoring the association between features and models. Additionally, there is a lack of research on the contribution of different features to depression recognition. To this end, this study introduces an innovative approach to depression detection using EEG data, integrating Ant-Lion Optimization (ALO) and Multi-Agent Reinforcement Learning (MARL) for feature fusion analysis. The inclusion of Explainable Artificial Intelligence (XAI) methods enhances the explainability of the model’s features. The Time-Delay Embedded Hidden Markov Model (TDE-HMM) is employed to infer internal brain states during depression, triggered by audio stimulation. The ALO-MARL algorithm, combined with hyper-parameter optimization of the XGBoost classifier, achieves high accuracy (93.69%), sensitivity (88.60%), specificity (97.08%), and F1-score (91.82%) on a auditory stimulus-evoked three-channel EEG dataset. The results suggest that this approach outperforms state-of-the-art feature selection methods for depression recognition on this dataset, and XAI elucidates the critical impact of the minimum value of Power Spectral Density (PSD), Sample Entropy (SampEn), and Réenyi Entropy (Ren) on depression recognition. The study also explores dynamic brain state transitions revealed by audio stimuli, providing insights for the clinical application of AI algorithms in depression recognition.
MCML Authors
BANet: A bilateral attention network for extracting changed buildings between remote sensing imagery and cadastral maps.
International Journal of Applied Earth Observation and Geoinformation 139.104486 (May. 2025). DOI
Abstract
Up-to-date cadastral maps are vital to local governments in administrating real estate in cities. With its growing availability, remote sensing imagery is the cost-effective data for updating semantic contents on cadastral maps. In this study, we address the problem of updating buildings on cadastral maps, as city renewal is mainly characterized by new construction and demolition. While previous works focus on extracting all buildings from remote sensing images, we argue that these methods not only disregard preliminary information on cadastral maps but also fail to preserve building priors in unchanged areas on cadastral maps. Therefore, we focus on the task of extracting changed buildings (i.e., newly built and demolished buildings) from remote sensing images and cadastral maps. To address this task, we create an image-map building change detection (IMBCD) dataset, formed by around 27K pairs of remote sensing images and maps and their corresponding changed buildings in six distinct geographical areas across the globe. Accordingly, we propose a Bilateral Attention Network (BANet), introducing a novel attention mechanism: changed-first (CF) attention and non-changed-first (NCF) attention. This bilateral attention mechanism helps to refine the uncertain areas between changed and non-changed regions. Extensive experiments on our IMBCD dataset showcase the superior performance of BANet. Specifically, our BANet outperforms state-of-the-art models with F1 scores of 90.00% and 63.00% for the IMBCD-WHU and IMBCD-Inria datasets. This confirms that the leverage of bilateral attention blocks (BAB) can boost performance.
MCML Authors
Assessing the Clinical and Functional Status of COPD Patients Using Speech Analysis During and After Exacerbation.
International Journal of Chronic Obstructive Pulmonary Disease 20 (Jan. 2025). DOI
Abstract
Background: Chronic obstructive pulmonary disease (COPD) affects breathing, speech production, and coughing. We evaluated a machine learning analysis of speech for classifying the disease severity of COPD.
Methods: In this single centre study, non-consecutive COPD patients were prospectively recruited for comparing their speech characteristics during and after an acute COPD exacerbation. We extracted a set of spectral, prosodic, and temporal variability features, which were used as input to a support vector machine (SVM). Our baseline for predicting patient state was an SVM model using self-reported BORG and COPD Assessment Test (CAT) scores.
Results: In 50 COPD patients (52% males, 22% GOLD II, 44% GOLD III, 32% GOLD IV, all patients group E), speech analysis was superior in distinguishing during and after exacerbation status compared to BORG and CAT scores alone by achieving 84% accuracy in prediction. CAT scores correlated with reading rhythm, and BORG scales with stability in articulation. Pulmonary function testing (PFT) correlated with speech pause rate and speech rhythm variability.
Conclusion: Speech analysis may be a viable technology for classifying COPD status, opening up new opportunities for remote disease monitoring.
MCML Authors
A probability model for estimating age in young individuals relative to key legal thresholds: 15, 18 or 21-year.
International Journal of Legal Medicine 139.1 (Jan. 2025). DOI
Abstract
Age estimations are relevant for pre-trial detention, sentencing in criminal cases and as part of the evaluation in asylum processes to protect the rights and privileges of minors. No current method can determine an exact chronological age due to individual variations in biological development. This study seeks to develop a validated statistical model for estimating an age relative to key legal thresholds (15, 18, and 21 years) based on a skeletal (CT-clavicle, radiography-hand/wrist or MR-knee) and tooth (radiography-third molar) developmental stages. The whole model is based on 34 scientific studies, divided into examinations of the hand/wrist (15 studies), clavicle (5 studies), distal femur (4 studies), and third molars (10 studies). In total, data from approximately 27,000 individuals have been incorporated and the model has subsequently been validated with data from 5,000 individuals. The core framework of the model is built upon transition analysis and is further developed by a combination of a type of parametric bootstrapping and Bayesian theory. Validation of the model includes testing the models on independent datasets of individuals with known ages and shows a high precision with separate populations aligning closely with the model’s predictions. The practical use of the complex statistical model requires a user-friendly tool to provide probabilities together with the margin of error. The assessment based on the model forms the medical component for the overall evaluation of an individual’s age.
MCML Authors
Global high categorical resolution land cover mapping via weak supervision.
ISPRS Journal of Photogrammetry and Remote Sensing 220 (Feb. 2025). DOI GitHub
Abstract
Land cover information is indispensable for advancing the United Nations’ sustainable development goals, and land cover mapping under a more detailed category system would significantly contribute to economic livelihood tracking and environmental degradation measurement. However, the substantial difficulty in acquiring fine-grained training data makes the implementation of this task particularly challenging. Here, we propose to combine fully labeled source domain and weakly labeled target domain for weakly supervised domain adaptation (WSDA). This is beneficial as the utilization of sparse and coarse weak labels can considerably alleviate the labor required for precise and detailed land cover annotation. Specifically, we introduce the Prototype-based pseudo-label Rectification and Expansion (PRE) approach, which leverages the prototypes (i.e., the class-wise feature centroids) as the bridge to connect sparse labels and global feature distributions. According to the feature distances to the prototypes, the confidence of pseudo-labels predicted in the unlabeled regions of the target domain is assessed. This confidence is then utilized to guide the dynamic expansion and rectification of pseudo-labels. Based on PRE, we carry out high categorical resolution land cover mapping for 10 cities in different regions around the world, severally using PlanetScope, Gaofen-1, and Sentinel-2 satellite images. In the study areas, we achieve cross-sensor, cross-category, and cross-continent WSDA, with the overall accuracy exceeding 80%. The promising results indicate that PRE is capable of reducing the dependency of land cover classification on high-quality annotations, thereby improving label efficiency. We expect our work to enable global fine-grained land cover mapping, which in turn promote Earth observation to provide more precise and thorough information for environmental monitoring.
MCML Authors
Moral parenthood and gestation: replies to Cordeiro, Murphy, Robinson and Baron.
Journal of Medical Ethics 51.2 (Jan. 2025). DOI
Abstract
I am grateful to James Cordeiro, Timothy Murphy, Heloise Robinson and Teresa Baron for their perceptive and stimulating comments on my article in this journal. In what follows, I seek to respond to some of the main points raised in each commentary.
MCML Authors
Moral parenthood: not gestational.
Journal of Medical Ethics 51.2 (Jan. 2025). DOI
Abstract
Parenting our biological children is a centrally important matter, but how, if it all, can it be justified? According to a contemporary influential line of thinking, the acquisition by parents of a moral right to parent their biological children should be grounded by appeal to the value of the intimate emotional relationship that gestation facilitates between a newborn and a gestational procreator. I evaluate two arguments in defence of this proposal and argue that both are unconvincing.Data are available in a public, open access repository.
MCML Authors
Flexible modelling of time-varying exposures and recurrent events to analyse training load effects in team sports injuries.
Journal of the Royal Statistical Society. Series C (Applied Statistics) 74.2 (Mar. 2025). DOI
Abstract
We present a flexible modelling approach to analyse time-varying exposures and recurrent events in team sports injuries. The approach is based on the piece-wise exponential additive mixed model where the effects of past exposures (i.e. high-intensity training loads) may accumulate over time and present complex forms of association. In order to identify a relevant time window at which past exposures have an impact on the current risk, we propose a penalty approach. We conduct a simulation study to evaluate the performance of the proposed model, under different true weight functions and different levels of heterogeneity between recurrent events. Finally, we illustrate the approach with a case study application involving an elite male football team participating in the Spanish LaLiga competition. The cohort includes time-loss injuries and external training load variables tracked by Global Positioning System devices, during the seasons 2017–2018 and 2018–2019.
MCML Authors
Machine Learning Consulting Unit (MLCU)
Statistical modelling of on-street parking spot occupancy in smart cities.
Journal of the Royal Statistical Society. Series C (Applied Statistics).qlaf017 (Mar. 2025). DOI
Abstract
Many studies suggest that searching for parking is associated with significant direct and indirect costs. Therefore, it is appealing to reduce the time that car drivers spend on finding an available parking spot, especially in urban areas where the space for all road users is limited. The prediction of on-street parking spot occupancy can provide drivers with guidance on where clear parking spaces are likely to be found. This field of research has gained more and more attention in the last decade through the increasing availability of real-time parking spot occupancy data. In this paper, we pursue a statistical approach for the prediction of parking spot occupancy, where we make use of time-to-event models and semi-Markov process theory. The latter involves the employment of Laplace transformations as well as their inversion, which is an ambitious numerical task. We apply our methodology to data from the City of Melbourne in Australia. Our main result is that the semi-Markov model outperforms a Markov model in terms of both true negative rate and true positive rate while this is essentially achieved by respecting the current duration that a parking space already spends in its initial state.
MCML Authors
Applied Statistics in Social Sciences, Economics and Business
Probabilistic scoring lists for interpretable machine learning.
Machine Learning 114.55 (Feb. 2025). DOI
Abstract
A scoring system is a simple decision model that checks a set of features, adds a certain number of points to a total score for each feature that is satisfied, and finally makes a decision by comparing the total score to a threshold. Scoring systems have a long history of active use in safety-critical domains such as healthcare and justice, where they provide guidance for making objective and accurate decisions. Given their genuine interpretability, the idea of learning scoring systems from data is obviously appealing from the perspective of explainable AI. In this paper, we propose a practically motivated extension of scoring systems called probabilistic scoring lists (PSL), as well as a method for learning PSLs from data. Instead of making a deterministic decision, a PSL represents uncertainty in the form of probability distributions, or, more generally, probability intervals. Moreover, in the spirit of decision lists, a PSL evaluates features one by one and stops as soon as a decision can be made with enough confidence. To evaluate our approach, we conduct case studies in the medical domain and on standard benchmark data.
MCML Authors
Artificial Intelligence and Machine Learning
Unlocking the diagnostic potential of electrocardiograms through information transfer from cardiac magnetic resonance imaging.
Medical Image Analysis 101.103451 (Apr. 2025). DOI GitHub
Abstract
Cardiovascular diseases (CVD) can be diagnosed using various diagnostic modalities. The electrocardiogram (ECG) is a cost-effective and widely available diagnostic aid that provides functional information of the heart. However, its ability to classify and spatially localise CVD is limited. In contrast, cardiac magnetic resonance (CMR) imaging provides detailed structural information of the heart and thus enables evidence-based diagnosis of CVD, but long scan times and high costs limit its use in clinical routine. In this work, we present a deep learning strategy for cost-effective and comprehensive cardiac screening solely from ECG. Our approach combines multimodal contrastive learning with masked data modelling to transfer domain-specific information from CMR imaging to ECG representations. In extensive experiments using data from 40,044 UK Biobank subjects, we demonstrate the utility and generalisability of our method for subject-specific risk prediction of CVD and the prediction of cardiac phenotypes using only ECG data. Specifically, our novel multimodal pre-training paradigm improves performance by up to 12.19% for risk prediction and 27.59% for phenotype prediction. In a qualitative analysis, we demonstrate that our learned ECG representations incorporate information from CMR image regions of interest.
MCML Authors
Self-supervised 3D medical image segmentation by flow-guided mask propagation learning.
Medical Image Analysis 101.103478 (Apr. 2025). DOI GitHub
Abstract
Despite significant progress in 3D medical image segmentation using deep learning, manual annotation remains a labor-intensive bottleneck. Self-supervised mask propagation (SMP) methods have emerged to alleviate this challenge, allowing intra-volume segmentation with just a single slice annotation. However, the previous SMP methods often rely on 2D information and ignore volumetric contexts. While our previous work, called Vol2Flow, attempts to address this concern, it exhibits limitations, including not focusing enough on local (i.e., slice-pair) information, neglecting global information (i.e., volumetric contexts) in the objective function, and error accumulation during slice-to-slice reconstruction. This paper introduces Flow2Mask, a novel SMP method, developed to overcome the limitations of previous SMP approaches, particularly Vol2Flow. During training, Flow2Mask proposes the Local-to-Global (L2G) loss to learn inter-slice flow fields among all consecutive slices within a volume in an unsupervised manner. This dynamic loss is based on curriculum learning to gradually learn information within a volume from local to global contexts. Additionally, the Inter-Slice Smoothness (ISS) loss is introduced as a regularization term to encourage changes between the slices occur consistently and continuously. During inference, Flow2Mask leverages these 3D flow fields for inter-slice mask propagation in a 3D image, spreading annotation from a single annotated slice to the entire volume. Moreover, we propose an automatic strategy to select the most representative slice as initial annotation in the mask propagation process. Experimental evaluations on different abdominal datasets demonstrate that our proposed SMP method outperforms previous approaches and improves the overall mean DSC of Vol2Flow by +2.1%, +8.2%, and +4.0% for the Sliver, CHAOS, and 3D-IRCAD datasets, respectively. Furthermore, Flow2Mask even exhibits substantial improvements in weakly-supervised and self-supervised few-shot segmentation methods when applied as a mask completion tool.
MCML Authors
Computer Aided Medical Procedures & Augmented Reality
Computer Aided Medical Procedures & Augmented Reality
Reconstructing and analyzing the invariances of low-dose CT image denoising networks.
Medical Physics 52 (Jan. 2025). DOI
Abstract
Background: Deep learning-based methods led to significant advancements in many areas of medical imaging, most of which are concerned with the reduction of artifacts caused by motion, scatter, or noise. However, with most neural networks being black boxes, they remain notoriously difficult to interpret, hindering their clinical implementation. In particular, it has been shown that networks exhibit invariances w.r.t. input features, that is, they learn to ignore certain information in the input data.
Purpose: To improve the interpretability of deep learning-based low-dose CT image denoising networks.
Methods: We learn a complete data representation of low-dose input images using a conditional variational autoencoder (cVAE). In this representation, invariances of any given denoising network are then disentangled from the information it is not invariant to using a conditional invertible neural network (cINN). At test time, image-space invariances are generated by applying the inverse of the cINN and subsequent decoding using the cVAE. We propose two methods to analyze sampled invariances and to find those that correspond to alterations of anatomical structures.
Results: The proposed method is applied to four popular deep learning-based low-dose CT image denoising networks. We find that the networks are not only invariant to noise amplitude and realizations, but also to anatomical structures.
Conclusions: The proposed method is capable of reconstructing and analyzing invariances of deep learning-based low-dose CT image denoising networks. This is an important step toward interpreting deep learning-based methods for medical imaging, which is essential for their clinical implementation.
MCML Authors
Biases in machine-learning models of human single-cell data.
Nature Cell Biology (Feb. 2025). DOI
Abstract
Recent machine-learning (ML)-based advances in single-cell data science have enabled the stratification of human tissue donors at single-cell resolution, promising to provide valuable diagnostic and prognostic insights. However, such insights are susceptible to biases. Here we discuss various biases that emerge along the pipeline of ML-based single-cell analysis, ranging from societal biases affecting whose samples are collected, to clinical and cohort biases that influence the generalizability of single-cell datasets, biases stemming from single-cell sequencing, ML biases specific to (weakly supervised or unsupervised) ML models trained on human single-cell samples and biases during the interpretation of results from ML models. We end by providing methods for single-cell data scientists to assess and mitigate biases, and call for efforts to address the root causes of biases.
MCML Authors
Algorithmic Machine Learning & Explainable AI
Evaluating normative representation learning in generative AI for robust anomaly detection in brain imaging.
Nature Communications 16.1624 (Feb. 2025). DOI GitHub
Abstract
Normative representation learning focuses on understanding the typical anatomical distributions from large datasets of medical scans from healthy individuals. Generative Artificial Intelligence (AI) leverages this attribute to synthesize images that accurately reflect these normative patterns. This capability enables the AI allowing them to effectively detect and correct anomalies in new, unseen pathological data without the need for expert labeling. Traditional anomaly detection methods often evaluate the anomaly detection performance, overlooking the crucial role of normative learning. In our analysis, we introduce novel metrics, specifically designed to evaluate this facet in AI models. We apply these metrics across various generative AI frameworks, including advanced diffusion models, and rigorously test them against complex and diverse brain pathologies. In addition, we conduct a large multi-reader study to compare these metrics to experts’ evaluations. Our analysis demonstrates that models proficient in normative learning exhibit exceptional versatility, adeptly detecting a wide range of unseen medical conditions.
MCML Authors
Pervasive glacier retreats across Svalbard from 1985 to 2023.
Nature Communications 16.705 (Jan. 2025). DOI
Abstract
A major uncertainty in predicting the behaviour of marine-terminating glaciers is ice dynamics driven by non-linear calving front retreat, which is poorly understood and modelled. Using 124919 calving front positions for 149 marine-terminating glaciers in Svalbard from 1985 to 2023, generated with deep learning, we identify pervasive calving front retreats for non-surging glaciers over the past 38 years. We observe widespread seasonal cycles in calving front position for over half of the glaciers. At the seasonal timescale, peak retreat rates exhibit a several-month phase lag, with changes on the west coast occurring before those on the east coast, coincident with regional ocean warming. This spatial variability in seasonal patterns is linked to different timings of warm ocean water inflow from the West Spitsbergen Current, demonstrating the dominant role of ice-ocean interaction in seasonal front changes. The interannual variability of calving front retreat shows a strong sensitivity to both atmospheric and oceanic warming, with immediate responses to large air and ocean temperature anomalies in 2016 and 2019, likely driven by atmospheric blocking that can influence extreme temperature variability. With more frequent blocking occurring and continued regional warming, future calving front retreats will likely intensify, leading to more significant glacier mass loss.
MCML Authors
Causal machine learning for single-cell genomics.
Nature Genetics (Mar. 2025). DOI
Abstract
Advances in single-cell ‘-omics’ allow unprecedented insights into the transcriptional profiles of individual cells and, when combined with large-scale perturbation screens, enable measuring of the effect of targeted perturbations on the whole transcriptome. These advances provide an opportunity to better understand the causative role of genes in complex biological processes. In this Perspective, we delineate the application of causal machine learning to single-cell genomics and its associated challenges. We first present the causal model that is most commonly applied to single-cell biology and then identify and discuss potential approaches to three open problems: the lack of generalization of models to novel experimental conditions, the complexity of interpreting learned models, and the difficulty of learning cell dynamics.
MCML Authors
Algorithmic Machine Learning & Explainable AI
FloodCastBench: A Large-Scale Dataset and Foundation Models for Flood Modeling and Forecasting.
Scientific Data 12.431 (Mar. 2025). DOI
Abstract
Effective flood forecasting is crucial for informed decision-making and emergency response. Existing flood datasets mainly describe flood events but lack dynamic process data suitable for machine learning (ML). This work introduces the FloodCastBench dataset, designed for ML-based flood modeling and forecasting, featuring four major flood events: Pakistan 2022, UK 2015, Australia 2022, and Mozambique 2019. FloodCastBench details the process of flood dynamics data acquisition, starting with input data preparation (e.g., topography, land use, rainfall) and flood measurement data collection (e.g., SAR-based maps, surveyed outlines) for hydrodynamic modeling. We deploy a widely recognized finite difference numerical solution to construct high-resolution spatiotemporal dynamic processes with 30-m spatial and 300-second temporal resolutions. Flood measurement data are used to calibrate the hydrodynamic model parameters and validate the flood inundation maps. FloodCastBench provides comprehensive low-fidelity and high-fidelity flood forecasting datasets specifically for ML. Furthermore, we establish a benchmark of foundational models for neural flood forecasting using FloodCastBench, validating its effectiveness in supporting ML models for spatiotemporal, cross-regional, and downscaled flood forecasting.
MCML Authors
Bias-corrected serum creatinine from UK Biobank electronic medical records generates an important data resource for kidney function trajectories.
Scientific Reports 15.3540 (Jan. 2025). DOI
Abstract
Loss of kidney function is a substantial personal and public health burden. Kidney function is typically assessed as estimated glomerular filtration rate (eGFR) based on serum creatinine. UK Biobank provides serum creatinine measurements from study center assessments (SC, n = 425,147 baseline, n = 15,314 with follow-up) and emerging electronic Medical Records (eMR, ‘GP-clinical’) present a promising resource to augment this data longitudinally. However, it is unclear whether eMR-based and SC-based creatinine values can be used jointly for research on eGFR decline. When comparing eMR-based with SC-based creatinine by calendar year (n = 70,231), we found a year-specific multiplicative bias for eMR-based creatinine that decreased over time (factor 0.84 for 2007, 0.97 for 2013). Deriving eGFR based on SC- and bias-corrected eMR-creatinine yielded 454,907 individuals with ≥ 1eGFR assessment (2,102,174 assessments). This included 206,063 individuals with ≥ 2 assessments over up to 60.2 years (median 6.00 assessments, median time = 8.7 years), where we also obtained eMR-based information on kidney disease or renal replacement therapy. We found an annual eGFR decline of 0.11 (95%-CI = 0.10–0.12) versus 1.04 mL/min/1.73m2/year (9%-CI = 1.03–1.05) without and with bias-correction, the latter being in line with literature. In summary, our bias-corrected eMR-based creatinine values enabled a 4-fold increased number of eGFR assessments in UK Biobank suitable for kidney function research.
MCML Authors
Statistical Consulting Unit (StaBLab)
Instrumental variable estimation for compositional treatments.
Scientific Reports 15.5158 (Feb. 2025). DOI
Abstract
Many scientific datasets are compositional in nature. Important biological examples include species abundances in ecology, cell-type compositions derived from single-cell sequencing data, and amplicon abundance data in microbiome research. Here, we provide a causal view on compositional data in an instrumental variable setting where the composition acts as the cause. First, we crisply articulate potential pitfalls for practitioners regarding the interpretation of compositional causes from the viewpoint of interventions and warn against attributing causal meaning to common summary statistics such as diversity indices in microbiome data analysis. We then advocate for and develop multivariate methods using statistical data transformations and regression techniques that take the special structure of the compositional sample space into account while still yielding scientifically interpretable results. In a comparative analysis on synthetic and real microbiome data we show the advantages and limitations of our proposal. We posit that our analysis provides a useful framework and guidance for valid and informative cause-effect estimation in the context of compositional data.
MCML Authors
Minimax Problems for Ensembles of Control-Affine Systems.
SIAM Journal on Control and Optimization 63.1 (Jan. 2025). DOI
Abstract
In this paper, we consider ensembles of control-affine systems in ℝd, and we study simultaneous optimal control problems related to the worst-case minimization. After proving that such problems admit solutions, denoting with (ΘN)N a sequence of compact sets that parametrize the ensembles of systems, we first show that the corresponding minimax optimal control problems are Γ-convergent whenever (ΘN)N has a limit with respect to the Hausdorff distance. Besides its independent interest, the previous result plays a crucial role for establishing the Pontryagin Maximum Principle (PMP) when the ensemble is parametrized by a set Θ consisting of infinitely many points. Namely, we first approximate Θ by finite and increasing-in-size sets (ΘN)N for which the PMP is known, and then we derive the PMP for the Γ-limiting problem. The same strategy can be pursued in applications, where we can reduce infinite ensembles to finite ones to compute the minimizers numerically. We bring as a numerical example the Schrödinger equation for a qubit with uncertain resonance frequency.
MCML Authors
Applied Numerical Analysis
Evaluating Machine Learning Models in Non-Standard Settings: An Overview and New Findings.
Statistical Science (Mar. 2025). To be published. Preprint available. arXiv
Abstract
Estimating the generalization error (GE) of machine learning models is fundamental, with resampling methods being the most common approach. However, in non-standard settings, particularly those where observations are not independently and identically distributed, resampling using simple random data divisions may lead to biased GE estimates. This paper strives to present well-grounded guidelines for GE estimation in various such non-standard settings: clustered data, spatial data, unequal sampling probabilities, concept drift, and hierarchically structured outcomes. Our overview combines well-established methodologies with other existing methods that, to our knowledge, have not been frequently considered in these particular settings. A unifying principle among these techniques is that the test data used in each iteration of the resampling procedure should reflect the new observations to which the model will be applied, while the training data should be representative of the entire data set used to obtain the final model. Beyond providing an overview, we address literature gaps by conducting simulation studies. These studies assess the necessity of using GE-estimation methods tailored to the respective setting. Our findings corroborate the concern that standard resampling methods often yield biased GE estimates in non-standard settings, underscoring the importance of tailored GE estimation.
MCML Authors
Biometry in Molecular Medicine
Statistical Learning and Data Science
Machine Learning Consulting Unit (MLCU)
Statistical Learning and Data Science
Statistical Learning and Data Science
Biometry in Molecular Medicine
Conditional independence in stationary distributions of diffusions.
Stochastic Processes and their Applications 184.104604 (Jun. 2025). DOI
Abstract
Stationary distributions of multivariate diffusion processes have recently been proposed as probabilistic models of causal systems in statistics and machine learning. Motivated by these developments, we study stationary multivariate diffusion processes with a sparsely structured drift. Our main result gives a characterization of the conditional independence relations that hold in a stationary distribution. The result draws on a graphical representation of the drift structure and pertains to conditional independence relations that hold generally as a consequence of the drift’s sparsity pattern.
MCML Authors
Physics-aware machine learning for glacier ice thickness estimation: a case study for Svalbard.
The Cryosphere 19.2 (Feb. 2025). DOI
Abstract
The ice thickness of the world’s glaciers is mostly unmeasured, and physics-based models to reconstruct ice thickness cannot always deliver accurate estimates. In this study, we use deep learning paired with physical knowledge to generate ice thickness estimates for all glaciers of Spitsbergen, Barentsøya, and Edgeøya in Svalbard. We incorporate mass conservation and other physically derived conditions into a neural network to predict plausible ice thicknesses even for glaciers without any in situ ice thickness measurements. With a glacier-wise cross-validation scheme, we evaluate the performance of the physics-informed neural network. The results of these proof-of-concept experiments let us identify several challenges and opportunities that affect the model’s performance in a real-world setting.
MCML Authors
Active learning of molecular data for task-specific objectives.
The Journal of Chemical Physics 162.014103 (Jan. 2025). DOI
Abstract
Active learning (AL) has shown promise to be a particularly data-efficient machine learning approach. Yet, its performance depends on the application, and it is not clear when AL practitioners can expect computational savings. Here, we carry out a systematic AL performance assessment for three diverse molecular datasets and two common scientific tasks: compiling compact, informative datasets and targeted molecular searches. We implemented AL with Gaussian processes (GP) and used the many-body tensor as molecular representation. For the first task, we tested different data acquisition strategies, batch sizes, and GP noise settings. AL was insensitive to the acquisition batch size, and we observed the best AL performance for the acquisition strategy that combines uncertainty reduction with clustering to promote diversity. However, for optimal GP noise settings, AL did not outperform the randomized selection of data points. Conversely, for targeted searches, AL outperformed random sampling and achieved data savings of up to 64%. Our analysis provides insight into this task-specific performance difference in terms of target distributions and data collection strategies. We established that the performance of AL depends on the relative distribution of the target molecules in comparison to the total dataset distribution, with the largest computational savings achieved when their overlap is minimal.
MCML Authors
An equivalency and efficiency study for one year digital pathology for clinical routine diagnostics in an accredited tertiary academic center.
Virchows Archiv (Feb. 2025). DOI
Abstract
Digital pathology is revolutionizing clinical diagnostics by offering enhanced efficiency, accuracy, and accessibility of pathological examinations. This study explores the implementation and validation of digital pathology in a large tertiary academic center, focusing on its gradual integration and transition into routine clinical diagnostics. In a comprehensive validation process over a 6-month period, we compared sign-out of digital and physical glass slides of a wide range of different tissue specimens and histopathological diagnoses. Key metrics such as diagnostic concordance and user satisfaction were assessed by involving the pathologists in a validation training and study phase. We measured turnaround times before and after transitioning to digital pathology to assess the impact on overall efficiency. Our results demonstrate a 99% concordance between the analog and digital reports while at the same time reducing the time to sign out a case by almost a minute, suggesting potential long-term efficiency gains. Our digital transition positively impacted our pathology workflow: Pathologists reported increased flexibility and satisfaction due to the ease of accessing and sharing digital slides. However, challenges were identified, including technical issues related to image quality and system integration. Lessons learned from this study emphasize the importance of robust training programs, adequate IT support, and ongoing evaluation to ensure successful integration. This validation study confirms that digital pathology is a viable and beneficial tool for accurate clinical routine diagnostics in large academic centers, offering insights for other institutions considering similar endeavors.
MCML Authors
01.01.2025
Related
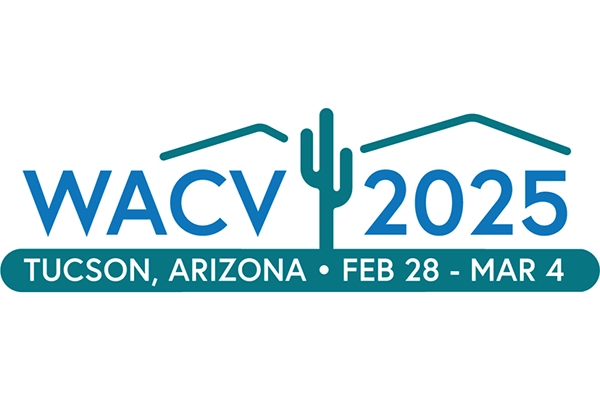
27.02.2025
MCML Researchers With Six Papers at WACV 2025
IEEE/CVF Winter Conference on Applications of Computer Vision (WACV 2025). Tucson, AZ, USA, 28.02.2025 - 04.03.2024
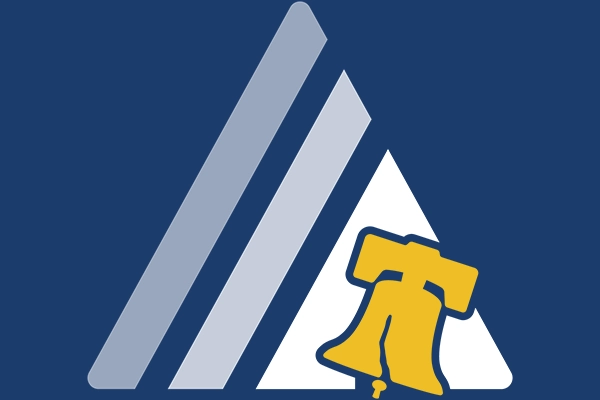
24.02.2025
MCML Researchers With Seven Papers at AAAI 2025
39th Conference on Artificial Intelligence (AAAI 2025). Philadelphia, PA, USA, 25.02.2025 - 04.03.2024
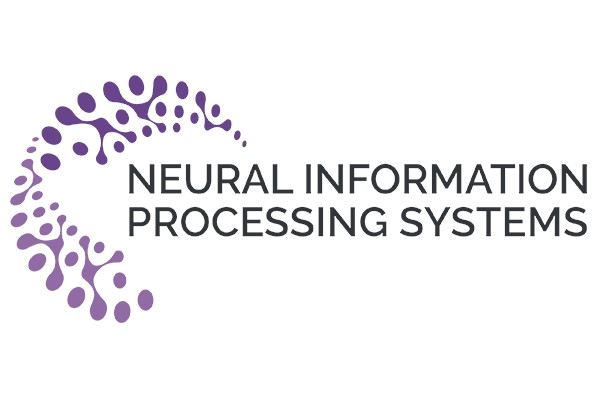
05.12.2024
MCML Researchers With 31 Papers at NeurIPS 2024
38th Conference on Neural Information Processing Systems (NeurIPS 2024). Vancouver, Canada, 10.12.2024 - 15.12.2024
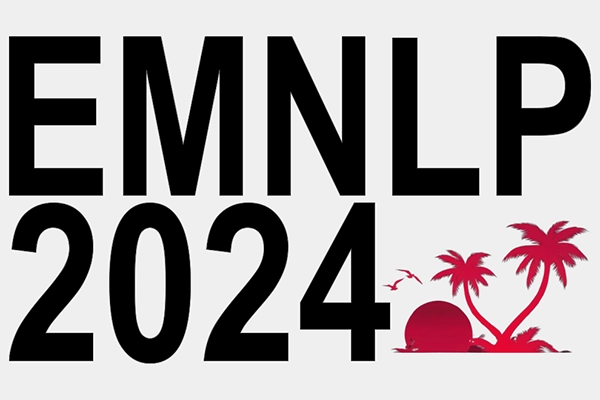
06.11.2024
MCML Researchers With 22 Papers at EMNLP 2024
Conference on Empirical Methods in Natural Language Processing (EMNLP 2024). Miami, FL, USA, 12.11.2024 - 16.11.2024