05.10.2023
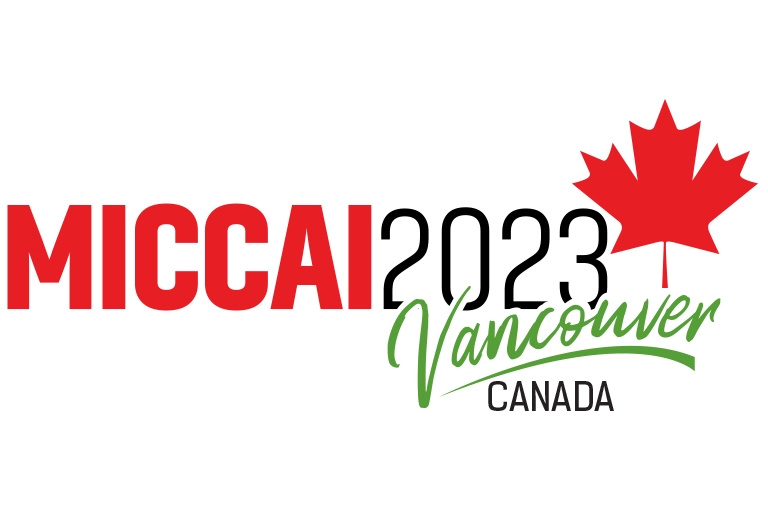
MCML Researchers With Seven Papers at MICCAI 2023
26th International Conference on Medical Image Computing and Computer Assisted Intervention (MICCAI 2023). Vancouver, Canada, 08.10.2023–12.10.2023
We are happy to announce that MCML researchers are represented with seven papers at MICCAI 2023. Congrats to our researchers!
Main Track (2 papers)
Clustering Disease Trajectories in Contrastive Feature Space for Biomarker Proposal in Age-Related Macular Degeneration.
MICCAI 2023 - 26th International Conference on Medical Image Computing and Computer Assisted Intervention. Vancouver, Canada, Oct 08-12, 2023. DOI
Abstract
Age-related macular degeneration (AMD) is the leading cause of blindness in the elderly. Current grading systems based on imaging biomarkers only coarsely group disease stages into broad categories that lack prognostic value for future disease progression. It is widely believed that this is due to their focus on a single point in time, disregarding the dynamic nature of the disease. In this work, we present the first method to automatically propose biomarkers that capture temporal dynamics of disease progression. Our method represents patient time series as trajectories in a latent feature space built with contrastive learning. Then, individual trajectories are partitioned into atomic sub-sequences that encode transitions between disease states. These are clustered using a newly introduced distance metric. In quantitative experiments we found our method yields temporal biomarkers that are predictive of conversion to late AMD. Furthermore, these clusters were highly interpretable to ophthalmologists who confirmed that many of the clusters represent dynamics that have previously been linked to the progression of AMD, even though they are currently not included in any clinical grading system.
MCML Authors
NISF: Neural implicit segmentation functions.
MICCAI 2023 - 26th International Conference on Medical Image Computing and Computer Assisted Intervention. Vancouver, Canada, Oct 08-12, 2023. DOI
Abstract
Segmentation of anatomical shapes from medical images has taken an important role in the automation of clinical measurements. While typical deep-learning segmentation approaches are performed on discrete voxels, the underlying objects being analysed exist in a real-valued continuous space. Approaches that rely on convolutional neural networks (CNNs) are limited to grid-like inputs and not easily applicable to sparse or partial measurements. We propose a novel family of image segmentation models that tackle many of CNNs’ shortcomings: Neural Implicit Segmentation Functions (NISF). Our framework takes inspiration from the field of neural implicit functions where a network learns a mapping from a real-valued coordinate-space to a shape representation. NISFs have the ability to segment anatomical shapes in high-dimensional continuous spaces. Training is not limited to voxelized grids, and covers applications with sparse and partial data. Interpolation between observations is learnt naturally in the training procedure and requires no post-processing. Furthermore, NISFs allow the leveraging of learnt shape priors to make predictions for regions outside of the original image plane. We go on to show the framework achieves dice scores of on a (3D+t) short-axis cardiac segmentation task using the UK Biobank dataset. We also provide a qualitative analysis on our frameworks ability to perform segmentation and image interpolation on unseen regions of an image volume at arbitrary resolutions.
MCML Authors
Artificial Intelligence in Healthcare and Medicine
Workshops (5 papers)
Metrics to Quantify Global Consistency in Synthetic Medical Images.
DGM4 @MICCAI 2023 - 3rd International Workshop on Deep Generative Models at the 26th International Conference on Medical Image Computing and Computer Assisted Intervention (MICCAI 2023). Vancouver, Canada, Oct 08-12, 2023. DOI
Abstract
Image synthesis is increasingly being adopted in medical image processing, for example for data augmentation or inter-modality image translation. In these critical applications, the generated images must fulfill a high standard of biological correctness. A particular requirement for these images is global consistency, i.e an image being overall coherent and structured so that all parts of the image fit together in a realistic and meaningful way. Yet, established image quality metrics do not explicitly quantify this property of synthetic images. In this work, we introduce two metrics that can measure the global consistency of synthetic images on a per-image basis. To measure the global consistency, we presume that a realistic image exhibits consistent properties, e.g., a person’s body fat in a whole-body MRI, throughout the depicted object or scene. Hence, we quantify global consistency by predicting and comparing explicit attributes of images on patches using supervised trained neural networks. Next, we adapt this strategy to an unlabeled setting by measuring the similarity of implicit image features predicted by a self-supervised trained network. Our results demonstrate that predicting explicit attributes of synthetic images on patches can distinguish globally consistent from inconsistent images. Implicit representations of images are less sensitive to assess global consistency but are still serviceable when labeled data is unavailable. Compared to established metrics, such as the FID, our method can explicitly measure global consistency on a per-image basis, enabling a dedicated analysis of the biological plausibility of single synthetic images.
MCML Authors
SCOPE: Structural Continuity Preservation for Medical Image Segmentation.
GRAIL @MICCAI 2023 - 5th Workshop on GRaphs in biomedicAl Image anaLysis at the 26th International Conference on Medical Image Computing and Computer Assisted Intervention (MICCAI 2023). Vancouver, Canada, Oct 08-12, 2023. DOI
Abstract
Although the preservation of shape continuity and physiological anatomy is a natural assumption in the segmentation of medical images, it is often neglected by deep learning methods that mostly aim for the statistical modeling of input data as pixels rather than interconnected structures. In biological structures, however, organs are not separate entities; for example, in reality, a severed vessel is an indication of an underlying problem, but traditional segmentation models are not designed to strictly enforce the continuity of anatomy, potentially leading to inaccurate medical diagnoses. To address this issue, we propose a graph-based approach that enforces the continuity and connectivity of anatomical topology in medical images. Our method encodes the continuity of shapes as a graph constraint, ensuring that the network’s predictions maintain this continuity. We evaluate our method on two public benchmarks on retinal vessel segmentation, showing significant improvements in connectivity metrics compared to traditional methods while getting better or on-par performance on segmentation metrics.
MCML Authors
Computer Aided Medical Procedures & Augmented Reality
Computer Aided Medical Procedures & Augmented Reality
Anatomy-Aware Masking for Inpainting in Medical Imaging.
ShapeMI @MICCAI 2023 - 3rd Workshop on Shape in Medical Imaging at the 26th International Conference on Medical Image Computing and Computer Assisted Intervention (MICCAI 2023). Vancouver, Canada, Oct 08-12, 2023. DOI GitHub
Abstract
Inpainting has recently been employed as a successful deep-learning technique for unsupervised model discovery in medical image analysis by taking advantage of the strong priors learned by models to reconstruct the structure and texture of missing parts in images. Even though the learned features depend on the masks as well as the images, the masks used for inpainting are typically random and independent of the dataset, due to the unpredictability of the content of images, i.e., different objects and shapes can appear in different locations in images. However, this is rarely the case for medical imaging data since they are obtained from similar anatomies. Still, random square masks are the most popular technique for inpainting in medical imaging. In this work, we propose a pipeline to generate, position and sample the masks to efficiently learn the shape and structures of the anatomy and generate a myriad of diverse anatomy-aware masks, aiding the model in learning the statistical shape prior to the topology of the organs of interest. We demonstrate the impact of our approach compared to other masking mechanisms in the reconstruction of anatomy. We compare the effectiveness of our proposed masking approach over square-shaped masks, which are traditionally used in medical imaging, and irregular shape masks, which are used in SOTA inpainting literature.
MCML Authors
Computer Aided Medical Procedures & Augmented Reality
Computer Aided Medical Procedures & Augmented Reality
SCOPE: Structural Continuity Preservation for Retinal Vessel Segmentation.
GRAIL @MICCAI 2023 - 5th Workshop on GRaphs in biomedicAl Image anaLysis at the 26th International Conference on Medical Image Computing and Computer Assisted Intervention (MICCAI 2023). Vancouver, Canada, Oct 08-12, 2023. DOI
Abstract
Although the preservation of shape continuity and physiological anatomy is a natural assumption in the segmentation of medical images, it is often neglected by deep learning methods that mostly aim for the statistical modeling of input data as pixels rather than interconnected structures. In biological structures, however, organs are not separate entities; for example, in reality, a severed vessel is an indication of an underlying problem, but traditional segmentation models are not designed to strictly enforce the continuity of anatomy, potentially leading to inaccurate medical diagnoses. To address this issue, we propose a graph-based approach that enforces the continuity and connectivity of anatomical topology in medical images. Our method encodes the continuity of shapes as a graph constraint, ensuring that the network’s predictions maintain this continuity. We evaluate our method on three public benchmarks of retinal vessel segmentation and one neuronal structure segmentation benchmark, showing significant improvements in connectivity metrics compared to previous works while getting better or on-par performance on segmentation metrics.
MCML Authors
Computer Aided Medical Procedures & Augmented Reality
Computer Aided Medical Procedures & Augmented Reality
Towards Generalised Neural Implicit Representations for Image Registration.
DGM4 @MICCAI 2023 - 3rd International Workshop on Deep Generative Models at the 26th International Conference on Medical Image Computing and Computer Assisted Intervention (MICCAI 2023). Vancouver, Canada, Oct 08-12, 2023. DOI
Abstract
Neural implicit representations (NIRs) enable to generate and parametrize the transformation for image registration in a continuous way. By design, these representations are image-pair-specific, meaning that for each signal a new multi-layer perceptron has to be trained. In this work, we investigate for the first time the potential of existent NIR generalisation methods for image registration and propose novel methods for the registration of a group of image pairs using NIRs. To exploit the generalisation potential of NIRs, we encode the fixed and moving image volumes to latent representations, which are then used to condition or modulate the NIR. Using ablation studies on a 3D benchmark dataset, we show that our methods are able to generalise to a set of image pairs with a performance comparable to pairwise registration using NIRs when trained on and datasets. Our results demonstrate the potential of generalised NIRs for 3D deformable image registration.
MCML Authors
05.10.2023
Related
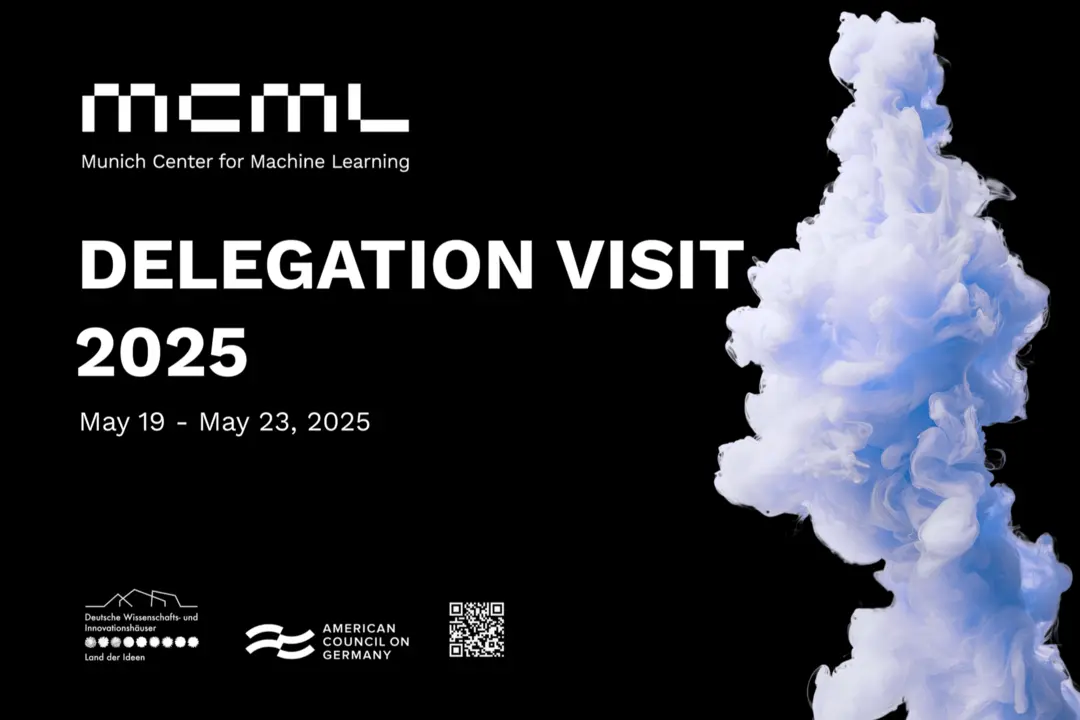
28.04.2025
MCML Delegation Visit to the USA
MCML delegation visits top US institutions to foster AI research collaborations in Generative and Medical AI, May 19–23, 2025.
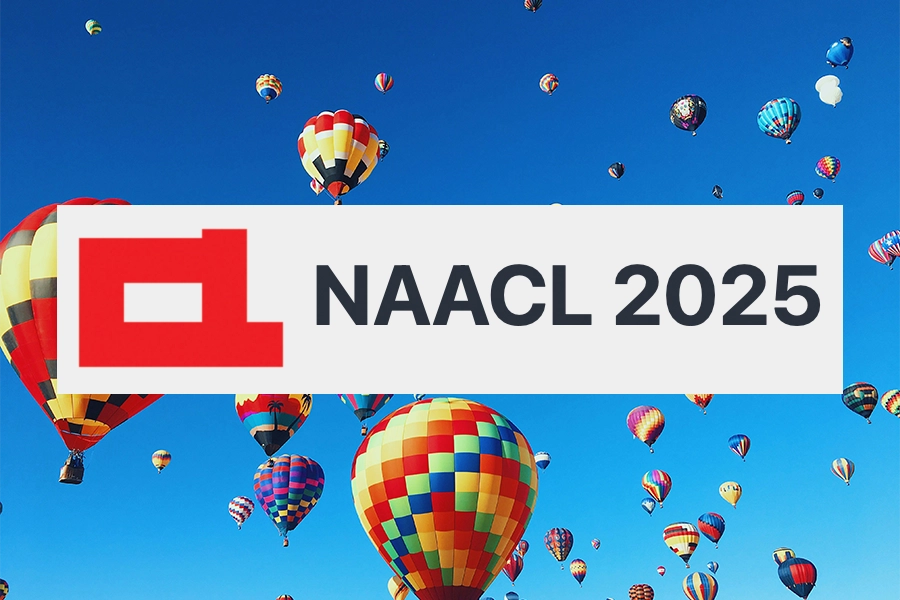
28.04.2025
MCML Researchers With Eleven Papers at NAACL 2025
Annual Conference of the North American Chapter of the Association for Computational Linguistics (NAACL 2025). Albuquerque, NM, USA, 29.04.2025 - 04.05.2024
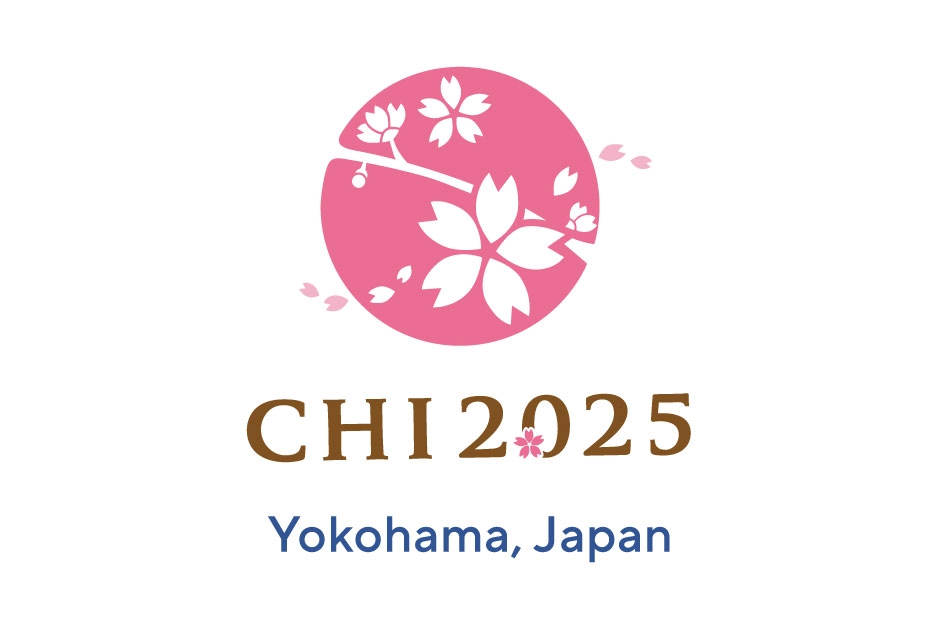
25.04.2025
MCML Researchers With Seven Papers at CHI 2025
Conference on Human Factors in Computing Systems (CHI 2025). Yokohama, Japan, 26.04.2025 - 01.05.2024
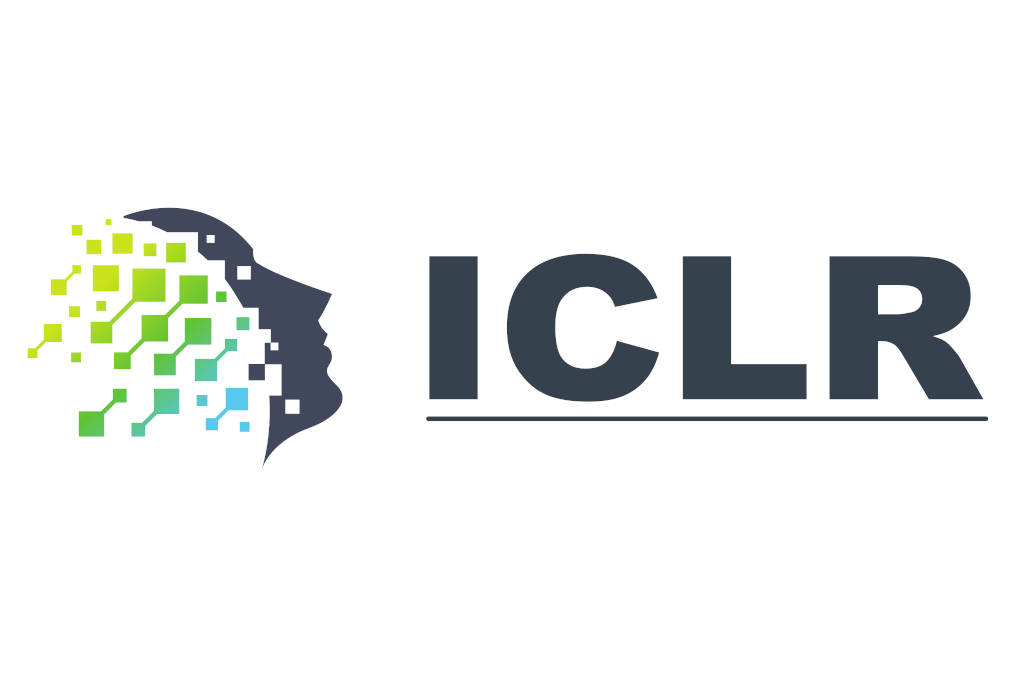
23.04.2025
MCML Researchers With 51 Papers at ICLR 2025
13th International Conference on Learning Representations (ICLR 2025). Singapore, 24.04.2025 - 28.04.2024