23.04.2025
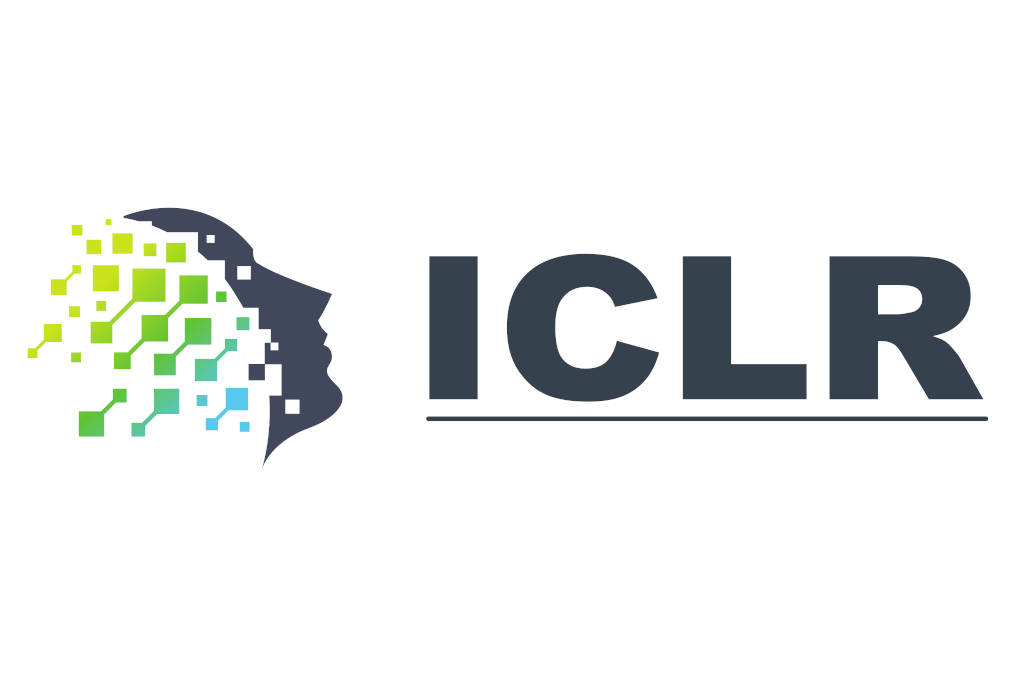
MCML Researchers With 51 Papers at ICLR 2025
13th International Conference on Learning Representations (ICLR 2025). Singapore, 24.04.2025–28.04.2024
We are happy to announce that MCML researchers are represented with 51 papers at ICLR 2025. Congrats to our researchers!
Main Track (34 papers)
ToddlerDiffusion: Interactive Structured Image Generation with Cascaded Schrödinger Bridge.
ICLR 2025 - 13th International Conference on Learning Representations. Singapore, Apr 24-28, 2025. To be published. Preprint available. arXiv GitHub
Abstract
Diffusion models break down the challenging task of generating data from high-dimensional distributions into a series of easier denoising steps. Inspired by this paradigm, we propose a novel approach that extends the diffusion framework into modality space, decomposing the complex task of RGB image generation into simpler, interpretable stages. Our method, termed ToddlerDiffusion, cascades modality-specific models, each responsible for generating an intermediate representation, such as contours, palettes, and detailed textures, ultimately culminating in a high-quality RGB image. Instead of relying on the naive LDM concatenation conditioning mechanism to connect the different stages together, we employ Schrödinger Bridge to determine the optimal transport between different modalities. Although employing a cascaded pipeline introduces more stages, which could lead to a more complex architecture, each stage is meticulously formulated for efficiency and accuracy, surpassing Stable-Diffusion (LDM) performance. Modality composition not only enhances overall performance but enables emerging proprieties such as consistent editing, interaction capabilities, high-level interpretability, and faster convergence and sampling rate. Extensive experiments on diverse datasets, including LSUN-Churches, ImageNet, CelebHQ, and LAION-Art, demonstrate the efficacy of our approach, consistently outperforming state-of-the-art methods. For instance, ToddlerDiffusion achieves notable efficiency, matching LDM performance on LSUN-Churches while operating 2× faster with a 3× smaller architecture.
MCML Authors
Efficient and Accurate Explanation Estimation with Distribution Compression.
ICLR 2025 - 13th International Conference on Learning Representations. Singapore, Apr 24-28, 2025. Spotlight Presentation. To be published. Preprint available. arXiv
Abstract
We discover a theoretical connection between explanation estimation and distribution compression that significantly improves the approximation of feature attributions, importance, and effects. While the exact computation of various machine learning explanations requires numerous model inferences and becomes impractical, the computational cost of approximation increases with an ever-increasing size of data and model parameters. We show that the standard i.i.d. sampling used in a broad spectrum of algorithms for post-hoc explanation leads to an approximation error worthy of improvement. To this end, we introduce Compress Then Explain (CTE), a new paradigm of sample-efficient explainability. It relies on distribution compression through kernel thinning to obtain a data sample that best approximates its marginal distribution. CTE significantly improves the accuracy and stability of explanation estimation with negligible computational overhead. It often achieves an on-par explanation approximation error 2-3x faster by using fewer samples, i.e. requiring 2-3x fewer model evaluations. CTE is a simple, yet powerful, plug-in for any explanation method that now relies on i.i.d. sampling.
MCML Authors
Statistical Learning and Data Science
Statistical Learning and Data Science
PRDP: Progressively Refined Differentiable Physics.
ICLR 2025 - 13th International Conference on Learning Representations. Singapore, Apr 24-28, 2025. To be published. Preprint available. arXiv
Abstract
The physics solvers employed for neural network training are primarily iterative, and hence, differentiating through them introduces a severe computational burden as iterations grow large. Inspired by works in bilevel optimization, we show that full accuracy of the network is achievable through physics significantly coarser than fully converged solvers. We propose Progressively Refined Differentiable Physics (PRDP), an approach that identifies the level of physics refinement sufficient for full training accuracy. By beginning with coarse physics, adaptively refining it during training, and stopping refinement at the level adequate for training, it enables significant compute savings without sacrificing network accuracy. Our focus is on differentiating iterative linear solvers for sparsely discretized differential operators, which are fundamental to scientific computing. PRDP is applicable to both unrolled and implicit differentiation. We validate its performance on a variety of learning scenarios involving differentiable physics solvers such as inverse problems, autoregressive neural emulators, and correction-based neural-hybrid solvers. In the challenging example of emulating the Navier-Stokes equations, we reduce training time by 62%.
MCML Authors
Decoupling Angles and Strength in Low-rank Adaptation.
ICLR 2025 - 13th International Conference on Learning Representations. Singapore, Apr 24-28, 2025. To be published. Preprint available. URL
Abstract
Parameter Efficient FineTuning (PEFT) methods have recently gained extreme popularity thanks to the vast availability of large-scale models, allowing to quickly adapt pretrained models to downstream tasks with minimal computational costs. However, current additive finetuning methods such as LoRA show low robustness to prolonged training and hyperparameter choices, not allowing for optimal out-of-the-box usage. On the other hand, multiplicative and bounded approaches such as ETHER, even if providing higher robustness, only allow for extremely low-rank adaptations and are limited to a fixed-strength transformation, hindering the expressive power of the adaptation. In this work, we propose the DeLoRA finetuning method that first normalizes and then scales the learnable low-rank matrices, thus effectively bounding the transformation strength, which leads to increased hyperparameter robustness at no cost in performance. We show that this proposed approach effectively and consistently improves over popular PEFT methods by evaluating our method on two finetuning tasks, subject-driven image generation and LLM instruction tuning.
MCML Authors
Interpretable and Reliable Machine Learning
Tailoring Mixup to Data for Calibration.
ICLR 2025 - 13th International Conference on Learning Representations. Singapore, Apr 24-28, 2025. To be published. Preprint available. URL
Abstract
Among all data augmentation techniques proposed so far, linear interpolation of training samples, also called Mixup, has found to be effective for a large panel of applications. Along with improved predictive performance, Mixup is also a good technique for improving calibration. However, mixing data carelessly can lead to manifold mismatch, i.e., synthetic data lying outside original class manifolds, which can deteriorate calibration. In this work, we show that the likelihood of assigning a wrong label with mixup increases with the distance between data to mix. To this end, we propose to dynamically change the underlying distributions of interpolation coefficients depending on the similarity between samples to mix, and define a flexible framework to do so without losing in diversity. We provide extensive experiments for classification and regression tasks, showing that our proposed method improves predictive performance and calibration of models, while being much more efficient.
MCML Authors
Interpretable and Reliable Machine Learning
SIM: Surface-based fMRI Analysis for Inter-Subject Multimodal Decoding from Movie-Watching Experiments.
ICLR 2025 - 13th International Conference on Learning Representations. Singapore, Apr 24-28, 2025. To be published. Preprint available. arXiv GitHub
Abstract
Current AI frameworks for brain decoding and encoding, typically train and test models within the same datasets. This limits their utility for brain computer interfaces (BCI) or neurofeedback, for which it would be useful to pool experiences across individuals to better simulate stimuli not sampled during training. A key obstacle to model generalisation is the degree of variability of inter-subject cortical organisation, which makes it difficult to align or compare cortical signals across participants. In this paper we address this through the use of surface vision transformers, which build a generalisable model of cortical functional dynamics, through encoding the topography of cortical networks and their interactions as a moving image across a surface. This is then combined with tri-modal self-supervised contrastive (CLIP) alignment of audio, video, and fMRI modalities to enable the retrieval of visual and auditory stimuli from patterns of cortical activity (and vice-versa). We validate our approach on 7T task-fMRI data from 174 healthy participants engaged in the movie-watching experiment from the Human Connectome Project (HCP). Results show that it is possible to detect which movie clips an individual is watching purely from their brain activity, even for individuals and movies not seen during training. Further analysis of attention maps reveals that our model captures individual patterns of brain activity that reflect semantic and visual systems. This opens the door to future personalised simulations of brain function.
MCML Authors
What is Wrong with Perplexity for Long-context Language Modeling?
ICLR 2025 - 13th International Conference on Learning Representations. Singapore, Apr 24-28, 2025. To be published. Preprint available. URL GitHub
Abstract
Handling long-context inputs is crucial for large language models (LLMs) in tasks such as extended conversations, document summarization, and many-shot in-context learning. While recent approaches have extended the context windows of LLMs and employed perplexity (PPL) as a standard evaluation metric, PPL has proven unreliable for assessing long-context capabilities. The underlying cause of this limitation has remained unclear. In this work, we provide a comprehensive explanation for this issue. We find that PPL overlooks key tokens, which are essential for long-context understanding, by averaging across all tokens and thereby obscuring the true performance of models in long-context scenarios. To address this, we propose textbf{LongPPL}, a novel metric that focuses on key tokens by employing a long-short context contrastive method to identify them. Our experiments demonstrate that LongPPL strongly correlates with performance on various long-context benchmarks (e.g., Pearson correlation of -0.96), significantly outperforming traditional PPL in predictive accuracy. Additionally, we introduce textbf{LongCE} (Long-context Cross-Entropy) loss, a re-weighting strategy for fine-tuning that prioritizes key tokens, leading to consistent improvements across diverse benchmarks. In summary, these contributions offer deeper insights into the limitations of PPL and present effective solutions for accurately evaluating and enhancing the long-context capabilities of LLMs.
MCML Authors
Inverse Constitutional AI: Compressing Preferences into Principles.
ICLR 2025 - 13th International Conference on Learning Representations. Singapore, Apr 24-28, 2025. To be published. Preprint available. URL GitHub
Abstract
Feedback data is widely used for fine-tuning and evaluating state-of-the-art AI models. Pairwise text preferences, where human or AI annotators select the “better” of two options, are particularly common. Such preferences are used to train (reward) models or to rank models with aggregate statistics. For many applications it is desirable to understand annotator preferences in addition to modelling them – not least because extensive prior work has shown various unintended biases in preference datasets. Yet, preference datasets remain challenging to interpret. Neither black-box reward models nor statistics can answer why one text is preferred over another. Manual interpretation of the numerous (long) response pairs is usually equally infeasible. In this paper, we introduce the Inverse Constitutional AI (ICAI) problem, formulating the interpretation of pairwise text preference data as a compression task. In constitutional AI, a set of principles (a constitution) is used to provide feedback and fine-tune AI models. ICAI inverts this process: given a feedback dataset, we aim to extract a constitution that best enables a large language model (LLM) to reconstruct the original annotations. We propose a corresponding ICAI algorithm and validate its generated constitutions quantitatively based on annotation reconstruction accuracy on several datasets: (a) synthetic feedback data with known principles; (b) AlpacaEval cross-annotated human feedback data; (c) crowdsourced Chatbot Arena data; and (d) PRISM data from diverse demographic groups. As an example application, we further demonstrate the detection of biases in human feedback data. As a short and interpretable representation of the original dataset, generated constitutions have many potential use cases: they may help identify undesirable annotator biases, better understand model performance, scale feedback to unseen data, or assist with adapting AI models to individual user or group preferences.
MCML Authors
Artificial Intelligence and Machine Learning
Model-agnostic meta-learners for estimating heterogeneous treatment effects over time.
ICLR 2025 - 13th International Conference on Learning Representations. Singapore, Apr 24-28, 2025. To be published. Preprint available. arXiv
Abstract
Estimating heterogeneous treatment effects (HTEs) over time is crucial in many disciplines such as personalized medicine. For example, electronic health records are commonly collected over several time periods and then used to personalize treatment decisions. Existing works for this task have mostly focused on model-based learners (i.e., learners that adapt specific machine-learning models). In contrast, model-agnostic learners – so-called meta-learners – are largely unexplored. In our paper, we propose several meta-learners that are model-agnostic and thus can be used in combination with arbitrary machine learning models (e.g., transformers) to estimate HTEs over time. Here, our focus is on learners that can be obtained via weighted pseudo-outcome regressions, which allows for efficient estimation by targeting the treatment effect directly. We then provide a comprehensive theoretical analysis that characterizes the different learners and that allows us to offer insights into when specific learners are preferable. Finally, we confirm our theoretical insights through numerical experiments. In sum, while meta-learners are already state-of-the-art for the static setting, we are the first to propose a comprehensive set of meta-learners for estimating HTEs in the time-varying setting.
MCML Authors
Artificial Intelligence in Management
Artificial Intelligence in Management
Artificial Intelligence in Management
Revealing and Reducing Gender Biases in Vision and Language Assistants (VLAs).
ICLR 2025 - 13th International Conference on Learning Representations. Singapore, Apr 24-28, 2025. To be published. Preprint available. arXiv
Abstract
Pre-trained large language models (LLMs) have been reliably integrated with visual input for multimodal tasks. The widespread adoption of instruction-tuned image-to-text vision-language assistants (VLAs) like LLaVA and InternVL necessitates evaluating gender biases. We study gender bias in 22 popular open-source VLAs with respect to personality traits, skills, and occupations. Our results show that VLAs replicate human biases likely present in the data, such as real-world occupational imbalances. Similarly, they tend to attribute more skills and positive personality traits to women than to men, and we see a consistent tendency to associate negative personality traits with men. To eliminate the gender bias in these models, we find that finetuning-based debiasing methods achieve the best tradeoff between debiasing and retaining performance on downstream tasks. We argue for pre-deploying gender bias assessment in VLAs and motivate further development of debiasing strategies to ensure equitable societal outcomes.
MCML Authors
Interpretable and Reliable Machine Learning
Interpretable and Reliable Machine Learning
Higher-Order Graphon Neural Networks: Approximation and Cut Distance.
ICLR 2025 - 13th International Conference on Learning Representations. Singapore, Apr 24-28, 2025. Spotlight Presentation. To be published. Preprint available. URL
Abstract
Graph limit models, like graphons for limits of dense graphs, have recently been used to study size transferability of graph neural networks (GNNs). While most literature focuses on message passing GNNs (MPNNs), in this work we attend to the more powerful higher-order GNNs. First, we extend the -WL test for graphons (Böker, 2023) to the graphon-signal space and introduce signal-weighted homomorphism densities as a key tool. As an exemplary focus, we generalize Invariant Graph Networks (IGNs) to graphons, proposing Invariant Graphon Networks (IWNs) defined via a subset of the IGN basis corresponding to bounded linear operators. Even with this restricted basis, we show that IWNs of order are at least as powerful as the -WL test, and we establish universal approximation results for graphon-signals in distances. This significantly extends the prior work of Cai & Wang (2022), showing that IWNs—a subset of their IGN-small—retain effectively the same expressivity as the full IGN basis in the limit. In contrast to their approach, our blueprint of IWNs also aligns better with the geometry of graphon space, for example facilitating comparability to MPNNs. We highlight that, while typical higher-order GNNs are discontinuous w.r.t. cut distance—which causes their lack of convergence and is inherently tied to the definition of -WL—their transferability remains comparable to MPNNs.
MCML Authors
Foundations of Deep Neural Networks
Stabilized Neural Prediction of Potential Outcomes in Continuous Time.
ICLR 2025 - 13th International Conference on Learning Representations. Singapore, Apr 24-28, 2025. To be published. Preprint available. arXiv
Abstract
Patient trajectories from electronic health records are widely used to predict potential outcomes of treatments over time, which then allows to personalize care. Yet, existing neural methods for this purpose have a key limitation: while some adjust for time-varying confounding, these methods assume that the time series are recorded in discrete time. In other words, they are constrained to settings where measurements and treatments are conducted at fixed time steps, even though this is unrealistic in medical practice. In this work, we aim to predict potential outcomes in continuous time. The latter is of direct practical relevance because it allows for modeling patient trajectories where measurements and treatments take place at arbitrary, irregular timestamps. We thus propose a new method called stabilized continuous time inverse propensity network (SCIP-Net). For this, we further derive stabilized inverse propensity weights for robust prediction of the potential outcomes. To the best of our knowledge, our SCIP-Net is the first neural method that performs proper adjustments for time-varying confounding in continuous time.
MCML Authors
Artificial Intelligence in Management
Artificial Intelligence in Management
Laplace Sample Information: Data Informativeness Through a Bayesian Lens.
ICLR 2025 - 13th International Conference on Learning Representations. Singapore, Apr 24-28, 2025. To be published. Preprint available. URL
Abstract
Accurately estimating the informativeness of individual samples in a dataset is an important objective in deep learning, as it can guide sample selection, which can improve model efficiency and accuracy by removing redundant or potentially harmful samples. We propose Laplace Sample Information (LSI) measure of sample informativeness grounded in information theory widely applicable across model architectures and learning settings. LSI leverages a Bayesian approximation to the weight posterior and the KL divergence to measure the change in the parameter distribution induced by a sample of interest from the dataset. We experimentally show that LSI is effective in ordering the data with respect to typicality, detecting mislabeled samples, measuring class-wise informativeness, and assessing dataset difficulty. We demonstrate these capabilities of LSI on image and text data in supervised and unsupervised settings. Moreover, we show that LSI can be computed efficiently through probes and transfers well to the training of large models.
MCML Authors
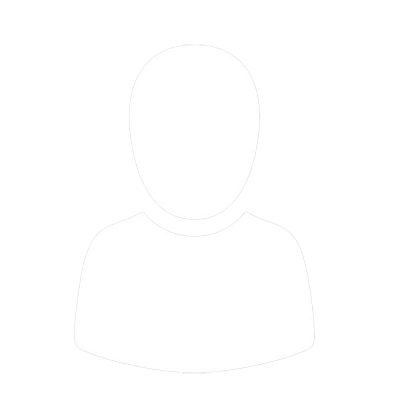
Georgios Kaissis
Dr.
* Former Member
Multi-Accurate CATE is Robust to Unknown Covariate Shifts.
ICLR 2025 - 13th International Conference on Learning Representations. Singapore, Apr 24-28, 2025. To be published. Preprint available. URL
Abstract
Estimating heterogeneous treatment effects is important to tailor treatments to those individuals who would most likely benefit. However, conditional average treatment effect predictors may often be trained on one population but possibly deployed on different, possibly unknown populations. We use methodology for learning multi-accurate predictors to post-process CATE T-learners (differenced regressions) to become robust to unknown covariate shifts at the time of deployment. The method works in general for pseudo-outcome regression, such as the DR-learner. We show how this approach can combine (large) confounded observational and (smaller) randomized datasets by learning a confounded predictor from the observational dataset, and auditing for multi-accuracy on the randomized controlled trial. We show improvements in bias and mean squared error in simulations with increasingly larger covariate shift, and on a semi-synthetic case study of a parallel large observational study and smaller randomized controlled experiment. Overall, we establish a connection between methods developed for multi-distribution learning and achieve appealing desiderata (e.g. external validity) in causal inference and machine learning.
MCML Authors
Deep Weight Factorization: Sparse Learning Through the Lens of Artificial Symmetries.
ICLR 2025 - 13th International Conference on Learning Representations. Singapore, Apr 24-28, 2025. To be published. Preprint available. arXiv
Abstract
Sparse regularization techniques are well-established in machine learning, yet their application in neural networks remains challenging due to the non-differentiability of penalties like the L1 norm, which is incompatible with stochastic gradient descent. A promising alternative is shallow weight factorization, where weights are decomposed into two factors, allowing for smooth optimization of L1-penalized neural networks by adding differentiable L2 regularization to the factors. In this work, we introduce deep weight factorization, extending previous shallow approaches to more than two factors. We theoretically establish equivalence of our deep factorization with non-convex sparse regularization and analyze its impact on training dynamics and optimization. Due to the limitations posed by standard training practices, we propose a tailored initialization scheme and identify important learning rate requirements necessary for training factorized networks. We demonstrate the effectiveness of our deep weight factorization through experiments on various architectures and datasets, consistently outperforming its shallow counterpart and widely used pruning methods.
MCML Authors
Statistical Learning and Data Science
Statistical Learning and Data Science
Statistics, Data Science and Machine Learning
Flow Matching with Gaussian Process Priors for Probabilistic Time Series Forecasting.
ICLR 2025 - 13th International Conference on Learning Representations. Singapore, Apr 24-28, 2025. To be published. Preprint available. URL
Abstract
Recent advancements in generative modeling, particularly diffusion models, have opened new directions for time series modeling, achieving state-of-the-art performance in forecasting and synthesis. However, the reliance of diffusion-based models on a simple, fixed prior complicates the generative process since the data and prior distributions differ significantly. We introduce TSFlow, a conditional flow matching (CFM) model for time series combining Gaussian processes, optimal transport paths, and data-dependent prior distributions. By incorporating (conditional) Gaussian processes, TSFlow aligns the prior distribution more closely with the temporal structure of the data, enhancing both unconditional and conditional generation. Furthermore, we propose conditional prior sampling to enable probabilistic forecasting with an unconditionally trained model. In our experimental evaluation on eight real-world datasets, we demonstrate the generative capabilities of TSFlow, producing high-quality unconditional samples. Finally, we show that both conditionally and unconditionally trained models achieve competitive results across multiple forecasting benchmarks.
MCML Authors
Data Analytics & Machine Learning
Self-supervised contrastive learning performs non-linear system identification.
ICLR 2025 - 13th International Conference on Learning Representations. Singapore, Apr 24-28, 2025. To be published. Preprint available. URL
Abstract
Self-supervised learning (SSL) approaches have brought tremendous success across many tasks and domains. It has been argued that these successes can be attributed to a link between SSL and identifiable representation learning: Temporal structure and auxiliary variables ensure that latent representations are related to the true underlying generative factors of the data. Here, we deepen this connection and show that SSL can perform system identification in latent space. We propose DynCL, a framework to uncover linear, switching linear and non-linear dynamics under a non-linear observation model, give theoretical guarantees and validate them empirically.
MCML Authors
Sparse autoencoders reveal selective remapping of visual concepts during adaptation.
ICLR 2025 - 13th International Conference on Learning Representations. Singapore, Apr 24-28, 2025. To be published. Preprint available. URL
Abstract
Adapting foundation models for specific purposes has become a standard approach to build machine learning systems for downstream applications. Yet, it is an open question which mechanisms take place during adaptation. Here we develop a new Sparse Autoencoder (SAE) for the CLIP vision transformer, named PatchSAE, to extract interpretable concepts at granular levels (e.g., shape, color, or semantics of an object) and their patch-wise spatial attributions. We explore how these concepts influence the model output in downstream image classification tasks and investigate how recent state-of-the-art prompt-based adaptation techniques change the association of model inputs to these concepts. While activations of concepts slightly change between adapted and non-adapted models, we find that the majority of gains on common adaptation tasks can be explained with the existing concepts already present in the non-adapted foundation model. This work provides a concrete framework to train and use SAEs for Vision Transformers and provides insights into explaining adaptation mechanisms.
MCML Authors
Calibrating LLMs with Information-Theoretic Evidential Deep Learning.
ICLR 2025 - 13th International Conference on Learning Representations. Singapore, Apr 24-28, 2025. To be published. Preprint available. URL
Abstract
Fine-tuned large language models (LLMs) often exhibit overconfidence, particularly when trained on small datasets, resulting in poor calibration and inaccurate uncertainty estimates. Evidential Deep Learning (EDL), an uncertainty-aware approach, enables uncertainty estimation in a single forward pass, making it a promising method for calibrating fine-tuned LLMs. However, despite its computational efficiency, EDL is prone to overfitting, as its training objective can result in overly concentrated probability distributions. To mitigate this, we propose regularizing EDL by incorporating an information bottleneck (IB). Our approach IB-EDL suppresses spurious information in the evidence generated by the model and encourages truly predictive information to influence both the predictions and uncertainty estimates. Extensive experiments across various fine-tuned LLMs and tasks demonstrate that IB-EDL outperforms both existing EDL and non-EDL approaches. By improving the trustworthiness of LLMs, IB-EDL facilitates their broader adoption in domains requiring high levels of confidence calibration.
MCML Authors
Statistical Learning and Data Science
Statistics, Data Science and Machine Learning
Statistical Learning and Data Science
Statistical Learning and Data Science
Topograph: An efficient Graph-Based Framework for Strictly Topology Preserving Image Segmentation.
ICLR 2025 - 13th International Conference on Learning Representations. Singapore, Apr 24-28, 2025. To be published. Preprint available. arXiv
Abstract
Topological correctness plays a critical role in many image segmentation tasks, yet most networks are trained using pixel-wise loss functions, such as Dice, neglecting topological accuracy. Existing topology-aware methods often lack robust topological guarantees, are limited to specific use cases, or impose high computational costs. In this work, we propose a novel, graph-based framework for topologically accurate image segmentation that is both computationally efficient and generally applicable. Our method constructs a component graph that fully encodes the topological information of both the prediction and ground truth, allowing us to efficiently identify topologically critical regions and aggregate a loss based on local neighborhood information. Furthermore, we introduce a strict topological metric capturing the homotopy equivalence between the union and intersection of prediction-label pairs. We formally prove the topological guarantees of our approach and empirically validate its effectiveness on binary and multi-class datasets. Our loss demonstrates state-of-the-art performance with up to fivefold faster loss computation compared to persistent homology methods.
MCML Authors
Signature Kernel Conditional Independence Tests in Causal Discovery for Stochastic Processes.
ICLR 2025 - 13th International Conference on Learning Representations. Singapore, Apr 24-28, 2025. To be published. Preprint available. arXiv
Abstract
Inferring the causal structure underlying stochastic dynamical systems from observational data holds great promise in domains ranging from science and health to finance. Such processes can often be accurately modeled via stochastic differential equations (SDEs), which naturally imply causal relationships via ‘which variables enter the differential of which other variables’. In this paper, we develop conditional independence (CI) constraints on coordinate processes over selected intervals that are Markov with respect to the acyclic dependence graph (allowing self-loops) induced by a general SDE model. We then provide a sound and complete causal discovery algorithm, capable of handling both fully and partially observed data, and uniquely recovering the underlying or induced ancestral graph by exploiting time directionality assuming a CI oracle. Finally, to make our algorithm practically usable, we also propose a flexible, consistent signature kernel-based CI test to infer these constraints from data. We extensively benchmark the CI test in isolation and as part of our causal discovery algorithms, outperforming existing approaches in SDE models and beyond.
MCML Authors
Ethics in Systems Design and Machine Learning
Ethics in Systems Design and Machine Learning
Exact Computation of Any-Order Shapley Interactions for Graph Neural Networks.
ICLR 2025 - 13th International Conference on Learning Representations. Singapore, Apr 24-28, 2025. To be published. Preprint available. arXiv
Abstract
Albeit the ubiquitous use of Graph Neural Networks (GNNs) in machine learning (ML) prediction tasks involving graph-structured data, their interpretability remains challenging. In explainable artificial intelligence (XAI), the Shapley Value (SV) is the predominant method to quantify contributions of individual features to a ML model’s output. Addressing the limitations of SVs in complex prediction models, Shapley Interactions (SIs) extend the SV to groups of features. In this work, we explain single graph predictions of GNNs with SIs that quantify node contributions and interactions among multiple nodes. By exploiting the GNN architecture, we show that the structure of interactions in node embeddings are preserved for graph prediction. As a result, the exponential complexity of SIs depends only on the receptive fields, i.e. the message-passing ranges determined by the connectivity of the graph and the number of convolutional layers. Based on our theoretical results, we introduce GraphSHAP-IQ, an efficient approach to compute any-order SIs exactly. GraphSHAP-IQ is applicable to popular message passing techniques in conjunction with a linear global pooling and output layer. We showcase that GraphSHAP-IQ substantially reduces the exponential complexity of computing exact SIs on multiple benchmark datasets. Beyond exact computation, we evaluate GraphSHAP-IQ’s approximation of SIs on popular GNN architectures and compare with existing baselines. Lastly, we visualize SIs of real-world water distribution networks and molecule structures using a SI-Graph.
MCML Authors
Artificial Intelligence and Machine Learning
Generalization, Expressivity, and Universality of Graph Neural Networks on Attributed Graphs.
ICLR 2025 - 13th International Conference on Learning Representations. Singapore, Apr 24-28, 2025. To be published. Preprint available. URL
Abstract
We analyze the universality and generalization of graph neural networks (GNNs) on attributed graphs, i.e., with node attributes. To this end, we propose pseudometrics over the space of all attributed graphs that describe the fine-grained expressivity of GNNs. Namely, GNNs are both Lipschitz continuous with respect to our pseudometrics and can separate attributed graphs that are distant in the metric. Moreover, we prove that the space of all attributed graphs is relatively compact with respect to our metrics. Based on these properties, we prove a universal approximation theorem for GNNs and generalization bounds for GNNs on any data distribution of attributed graphs. The proposed metrics compute the similarity between the structures of attributed graphs via a hierarchical optimal transport between computation trees. Our work extends and unites previous approaches which either derived theory only for graphs with no attributes, derived compact metrics under which GNNs are continuous but without separation power, or derived metrics under which GNNs are continuous and separate points but the space of graphs is not relatively compact, which prevents universal approximation and generalization analysis.
MCML Authors
Implicit Neural Surface Deformation with Explicit Velocity Fields.
ICLR 2025 - 13th International Conference on Learning Representations. Singapore, Apr 24-28, 2025. To be published. Preprint available. arXiv
Abstract
In this work, we introduce the first unsupervised method that simultaneously predicts time-varying neural implicit surfaces and deformations between pairs of point clouds. We propose to model the point movement using an explicit velocity field and directly deform a time-varying implicit field using the modified level-set equation. This equation utilizes an iso-surface evolution with Eikonal constraints in a compact formulation, ensuring the integrity of the signed distance field. By applying a smooth, volume-preserving constraint to the velocity field, our method successfully recovers physically plausible intermediate shapes. Our method is able to handle both rigid and non-rigid deformations without any intermediate shape supervision. Our experimental results demonstrate that our method significantly outperforms existing works, delivering superior results in both quality and efficiency.
MCML Authors
ParFam -- (Neural Guided) Symbolic Regression Based on Continuous Global Optimization.
ICLR 2025 - 13th International Conference on Learning Representations. Singapore, Apr 24-28, 2025. To be published. Preprint available. arXiv GitHub
Abstract
The problem of symbolic regression (SR) arises in many different applications, such as identifying physical laws or deriving mathematical equations describing the behavior of financial markets from given data. Various methods exist to address the problem of SR, often based on genetic programming. However, these methods are usually complicated and involve various hyperparameters. In this paper, we present our new approach ParFam that utilizes parametric families of suitable symbolic functions to translate the discrete symbolic regression problem into a continuous one, resulting in a more straightforward setup compared to current state-of-the-art methods. In combination with a global optimizer, this approach results in a highly effective method to tackle the problem of SR. We theoretically analyze the expressivity of ParFam and demonstrate its performance with extensive numerical experiments based on the common SR benchmark suit SRBench, showing that we achieve state-of-the-art results. Moreover, we present an extension incorporating a pre-trained transformer network DL-ParFam to guide ParFam, accelerating the optimization process by up to two magnitudes.
MCML Authors
Mathematical Foundations of Artificial Intelligence
Mathematical Foundations of Artificial Intelligence
Differentially private learners for heterogeneous treatment effects.
ICLR 2025 - 13th International Conference on Learning Representations. Singapore, Apr 24-28, 2025. To be published. Preprint available. URL
Abstract
Patient data is widely used to estimate heterogeneous treatment effects and understand the effectiveness and safety of drugs. Yet, patient data includes highly sensitive information that must be kept private. In this work, we aim to estimate the conditional average treatment effect (CATE) from observational data under differential privacy. Specifically, we present DP-CATE, a novel framework for CATE estimation that is doubly robust and ensures differential privacy of the estimates. For this, we build upon non-trivial tools from semi-parametric and robust statistics to exploit the connection between privacy and model robustness. Our framework is highly general and applies to any two-stage CATE meta-learner with a Neyman-orthogonal loss function. It can be used with all machine learning models employed for nuisance estimation. We further provide an extension of DP-CATE where we employ RKHS regression to release the complete doubly robust CATE function while ensuring differential privacy. We demonstrate the effectiveness of DP-CATE across various experiments using synthetic and real-world datasets. To the best of our knowledge, we are the first to provide a framework for CATE estimation that is doubly robust and differentially private.
MCML Authors
Artificial Intelligence in Management
Artificial Intelligence in Management
Artificial Intelligence in Management
Improved Sampling Of Diffusion Models In Fluid Dynamics With Tweedie's Formula.
ICLR 2025 - 13th International Conference on Learning Representations. Singapore, Apr 24-28, 2025. To be published. Preprint available. URL
Abstract
State-of-the-art Denoising Diffusion Probabilistic Models (DDPMs) rely on an expensive sampling process with a large Number of Function Evaluations (NFEs) to provide high-fidelity predictions. This computational bottleneck renders diffusion models less appealing as surrogates for the spatio-temporal prediction of physics-based problems with long rollout horizons. We propose Truncated Sampling Models, enabling single-step and few-step sampling with elevated fidelity by simple truncation of the diffusion process, reducing the gap between DDPMs and deterministic single-step approaches. We also introduce a novel approach, Iterative Refinement, to sample pre-trained DDPMs by reformulating the generative process as a refinement process with few sampling steps. Both proposed methods enable significant improvements in accuracy compared to DDPMs, DDIMs, and EDMs with NFEs ≤ 10 on a diverse set of experiments, including incompressible and compressible turbulent flow and airfoil flow uncertainty simulations. Our proposed methods provide stable predictions for long rollout horizons in time-dependent problems and are able to learn all modes of the data distribution in steady-state problems with high uncertainty.
MCML Authors
Microcanonical Langevin Ensembles: Advancing the Sampling of Bayesian Neural Networks.
ICLR 2025 - 13th International Conference on Learning Representations. Singapore, Apr 24-28, 2025. To be published. Preprint available. URL
Abstract
Despite recent advances, sampling-based inference for Bayesian Neural Networks (BNNs) remains a significant challenge in probabilistic deep learning. While sampling-based approaches do not require a variational distribution assumption, current state-of-the-art samplers still struggle to navigate the complex and highly multimodal posteriors of BNNs. As a consequence, sampling still requires considerably longer inference times than non-Bayesian methods even for small neural networks, despite recent advances in making software implementations more efficient. Besides the difficulty of finding high-probability regions, the time until samplers provide sufficient exploration of these areas remains unpredictable. To tackle these challenges, we introduce an ensembling approach that leverages strategies from optimization and a recently proposed sampler called Microcanonical Langevin Monte Carlo (MCLMC) for efficient, robust and predictable sampling performance. Compared to approaches based on the state-of-the-art No-U-Turn Sampler, our approach delivers substantial speedups up to an order of magnitude, while maintaining or improving predictive performance and uncertainty quantification across diverse tasks and data modalities. The suggested Microcanonical Langevin Ensembles and modifications to MCLMC additionally enhance the method’s predictability in resource requirements, facilitating easier parallelization. All in all, the proposed method offers a promising direction for practical, scalable inference for BNNs.
MCML Authors
Statistics, Data Science and Machine Learning
Statistics, Data Science and Machine Learning
Generalization Bounds for Canonicalization: A Comparative Study with Group Averaging.
ICLR 2025 - 13th International Conference on Learning Representations. Singapore, Apr 24-28, 2025. To be published. Preprint available. URL
Abstract
Canonicalization, a popular method for generating invariant or equivariant function classes from arbitrary function sets, involves initial data projection onto a reduced input space subset, followed by applying any learning method to the projected dataset. Despite recent research on the expressive power and continuity of functions represented by canonicalization, its generalization capabilities remain less explored. This paper addresses this gap by theoretically examining the generalization benefits and sample complexity of canonicalization, comparing them with group averaging, another popular technique for creating invariant or equivariant function classes. Our findings reveal two distinct regimes where canonicalization may outperform or underperform compared to group averaging, with precise quantification of this phase transition in terms of sample size, group action characteristics, and a newly introduced concept of alignment. To the best of our knowledge, this study represents the first theoretical exploration of such behavior, offering insights into the relative effectiveness of canonicalization and group averaging under varying conditions.
MCML Authors
Disentangled Representation Learning with the Gromov-Monge Gap.
ICLR 2025 - 13th International Conference on Learning Representations. Singapore, Apr 24-28, 2025. To be published. Preprint available. arXiv
Abstract
Learning disentangled representations from unlabelled data is a fundamental challenge in machine learning. Solving it may unlock other problems, such as generalization, interpretability, or fairness. Although remarkably challenging to solve in theory, disentanglement is often achieved in practice through prior matching. Furthermore, recent works have shown that prior matching approaches can be enhanced by leveraging geometrical considerations, e.g., by learning representations that preserve geometric features of the data, such as distances or angles between points. However, matching the prior while preserving geometric features is challenging, as a mapping that fully preserves these features while aligning the data distribution with the prior does not exist in general. To address these challenges, we introduce a novel approach to disentangled representation learning based on quadratic optimal transport. We formulate the problem using Gromov-Monge maps that transport one distribution onto another with minimal distortion of predefined geometric features, preserving them as much as can be achieved. To compute such maps, we propose the Gromov-Monge-Gap (GMG), a regularizer quantifying whether a map moves a reference distribution with minimal geometry distortion. We demonstrate the effectiveness of our approach for disentanglement across four standard benchmarks, outperforming other methods leveraging geometric considerations.
MCML Authors
Surgical, Cheap, and Flexible: Mitigating False Refusal in Language Models via Single Vector Ablation.
ICLR 2025 - 13th International Conference on Learning Representations. Singapore, Apr 24-28, 2025. To be published. Preprint available. URL
Abstract
Training a language model to be both helpful and harmless requires careful calibration of refusal behaviours: Models should refuse to follow malicious instructions or give harmful advice (e.g.‘how do I kill someone?’), but they should not refuse safe requests, even if they superficially resemble unsafe ones (e.g. ‘how do I kill a Python process?’). Avoiding such false refusal, as prior work has shown, is challenging even for highly-capable language models. In this paper, we propose a simple and surgical method for mitigating false refusal in language models via single vector ablation. For a given model, we extract a false refusal vector and show that ablating this vector reduces false refusal rate while preserving the model’s safety and general capabilities. We also show that our approach can be used for fine-grained calibration of model safety. Our approach is training-free and model-agnostic, making it useful for mitigating the problem of false refusal in current and future language models.
MCML Authors
AI and Computational Linguistics
Constructing Confidence Intervals for Average Treatment Effects from Multiple Datasets.
ICLR 2025 - 13th International Conference on Learning Representations. Singapore, Apr 24-28, 2025. To be published. Preprint available. arXiv
Abstract
Constructing confidence intervals (CIs) for the average treatment effect (ATE) from patient records is crucial to assess the effectiveness and safety of drugs. However, patient records typically come from different hospitals, thus raising the question of how multiple observational datasets can be effectively combined for this purpose. In our paper, we propose a new method that estimates the ATE from multiple observational datasets and provides valid CIs. Our method makes little assumptions about the observational datasets and is thus widely applicable in medical practice. The key idea of our method is that we leverage prediction-powered inferences and thereby essentially `shrink’ the CIs so that we offer more precise uncertainty quantification as compared to naïve approaches. We further prove the unbiasedness of our method and the validity of our CIs. We confirm our theoretical results through various numerical experiments. Finally, we provide an extension of our method for constructing CIs from combinations of experimental and observational datasets.
MCML Authors
Artificial Intelligence in Management
Artificial Intelligence in Management
Artificial Intelligence in Management
Artificial Intelligence in Management
Artificial Intelligence in Management
Artificial Intelligence in Management
MaskBit: Embedding-free Image Generation via Bit Tokens.
ICLR 2025 - 13th International Conference on Learning Representations. Singapore, Apr 24-28, 2025. To be published. Preprint available. arXiv
Abstract
Masked transformer models for class-conditional image generation have become a compelling alternative to diffusion models. Typically comprising two stages - an initial VQGAN model for transitioning between latent space and image space, and a subsequent Transformer model for image generation within latent space - these frameworks offer promising avenues for image synthesis. In this study, we present two primary contributions: Firstly, an empirical and systematic examination of VQGANs, leading to a modernized VQGAN. Secondly, a novel embedding-free generation network operating directly on bit tokens - a binary quantized representation of tokens with rich semantics. The first contribution furnishes a transparent, reproducible, and high-performing VQGAN model, enhancing accessibility and matching the performance of current state-of-the-art methods while revealing previously undisclosed details. The second contribution demonstrates that embedding-free image generation using bit tokens achieves a new state-of-the-art FID of 1.52 on the ImageNet 256x256 benchmark, with a compact generator model of mere 305M parameters.
MCML Authors
Beyond Interpretability: The Gains of Feature Monosemanticity on Model Robustness.
ICLR 2025 - 13th International Conference on Learning Representations. Singapore, Apr 24-28, 2025. To be published. Preprint available. URL
Abstract
Deep learning models often suffer from a lack of interpretability due to polysemanticity, where individual neurons are activated by multiple unrelated semantics, resulting in unclear attributions of model behavior. Recent advances in monosemanticity, where neurons correspond to consistent and distinct semantics, have significantly improved interpretability but are commonly believed to compromise accuracy. In this work, we challenge the prevailing belief of the accuracy-interpretability tradeoff, showing that monosemantic features not only enhance interpretability but also bring concrete gains in model performance. Across multiple robust learning scenarios-including input and label noise, few-shot learning, and out-of-domain generalization-our results show that models leveraging monosemantic features significantly outperform those relying on polysemantic features. Furthermore, we provide empirical and theoretical understandings on the robustness gains of feature monosemanticity. Our preliminary analysis suggests that monosemanticity, by promoting better separation of feature representations, leads to more robust decision boundaries. This diverse evidence highlights the generality of monosemanticity in improving model robustness. As a first step in this new direction, we embark on exploring the learning benefits of monosemanticity beyond interpretability, supporting the long-standing hypothesis of linking interpretability and robustness.
MCML Authors
Workshops (17 papers)
Graph Neural Networks for Enhancing Ensemble Forecasts of Extreme Rainfall.
Climate Change AI @ICLR 2025 - Workshop on Tackling Climate Change with Machine Learning at the 13th International Conference on Learning Representations (ICLR 2025). Singapore, Apr 24-28, 2025. To be published. Preprint available. arXiv
Abstract
Climate change is increasing the occurrence of extreme precipitation events, threatening infrastructure, agriculture, and public safety. Ensemble prediction systems provide probabilistic forecasts but exhibit biases and difficulties in capturing extreme weather. While post-processing techniques aim to enhance forecast accuracy, they rarely focus on precipitation, which exhibits complex spatial dependencies and tail behavior. Our novel framework leverages graph neural networks to post-process ensemble forecasts, specifically modeling the extremes of the underlying distribution. This allows to capture spatial dependencies and improves forecast accuracy for extreme events, thus leading to more reliable forecasts and mitigating risks of extreme precipitation and flooding.
MCML Authors
Mathematical Foundations of Artificial Intelligence
Mathematical Foundations of Artificial Intelligence
Mathematical Foundations of Artificial Intelligence
Mathematical Foundations of Artificial Intelligence
Mathematical Foundations of Artificial Intelligence
Why Uncertainty Calibration Matters for Reliable Perturbation-based Explanations.
XAI4Science @ICLR 2025 - Workshop XAI4Science: From Understanding Model Behavior to Discovering New Scientific Knowledge at the 13th International Conference on Learning Representations (ICLR 2025). Singapore, Apr 24-28, 2025. To be published. Preprint available. URL
Abstract
Perturbation-based explanations are widely utilized to enhance the transparency of modern machine-learning models. However, their reliability is often compromised by the unknown model behavior under the specific perturbations used. This paper investigates the relationship between uncertainty calibration - the alignment of model confidence with actual accuracy - and perturbation-based explanations. We show that models frequently produce unreliable probability estimates when subjected to explainability-specific perturbations and theoretically prove that this directly undermines explanation quality. To address this, we introduce ReCalX, a novel approach to recalibrate models for improved perturbation-based explanations while preserving their original predictions. Experiments on popular computer vision models demonstrate that our calibration strategy produces explanations that are more aligned with human perception and actual object locations.
MCML Authors
How to Merge Multimodal Models Over Time?
MCDC @ICLR 2025 - Workshop on Modularity for Collaborative, Decentralized, and Continual Deep Learning at the 13th International Conference on Learning Representations (ICLR 2025). Singapore, Apr 24-28, 2025. To be published. Preprint available. URL
Abstract
Model merging combines multiple expert models finetuned from a base foundation model on diverse tasks and domains into a single, more capable model. However, most existing model merging approaches assume that all experts are available simultaneously. In reality, new tasks and domains emerge progressively over time, requiring strategies to integrate the knowledge of expert models as they become available: a process we call temporal model merging. The temporal dimension introduces unique challenges not addressed in prior work, raising new questions such as: when training for a new task, should the expert model start from the merged past experts or from the original base model? Should we merge all models at each time step? Which merging techniques are best suited for temporal merging? Should different strategies be used to initialize the training and deploy the model? To answer these questions, we propose a unified framework called TIME (Temporal Integration of Model Expertise) which defines temporal model merging across three axes: (1) initialization, (2) deployment, and (3) merging technique. Using TIME, we study temporal model merging across model sizes, compute budgets, and learning horizons on the FoMo-in-Flux benchmark. Our comprehensive suite of experiments across TIME allows us to build a better understanding of current challenges and best practices for effective temporal model merging.
MCML Authors
Tracking ESG Disclosures of European Companies with Retrieval-Augmented Generation.
Climate Change AI @ICLR 2025 - Workshop on Tackling Climate Change with Machine Learning at the 13th International Conference on Learning Representations (ICLR 2025). Singapore, Apr 24-28, 2025. To be published.
Abstract
Corporations play a crucial role in mitigating climate change and accelerating progress toward environmental, social, and governance (ESG) objectives. However, structured information on the current state of corporate ESG efforts remains limited. In this paper, we propose a machine learning framework based on a retrieval-augmented generation (RAG) pipeline to track ESG indicators from N = 9, 200 corporate reports. Our analysis includes ESG indicators from 600 of the largest listed corporations in Europe between 2014 and 2023. We focus on two key dimensions: first, we identify gaps in corporate sustainability reporting in light of existing standards. Second, we provide comprehensive bottom-up estimates of key ESG indicators across European industries. Our findings enable policymakers and financial markets to effectively assess corporate ESG transparency and track progress toward global sustainability objectives.
MCML Authors
Artificial Intelligence in Management
Artificial Intelligence in Management
Approximate Posteriors in Neural Networks: A Sampling Perspective.
FPI @ICLR 2025 - Workshop on Frontiers in Probabilistic Inference: Learning meets Sampling at the 13th International Conference on Learning Representations (ICLR 2025). Singapore, Apr 24-28, 2025. Spotlight Talk. To be published. Preprint available. URL
Abstract
The landscape of neural network loss functions is known to be highly complex, and the ability of gradient-based approaches to find well-generalizing solutions to such high-dimensional problems is often considered a miracle. Similarly, Bayesian neural networks (BNNs) inherit this complexity through the model’s likelihood. In applications where BNNs are used to account for weight uncertainty, recent advantages in sampling-based inference (SAI) have shown promising results outperforming other approximate Bayesian inference (ABI) methods. In this work, we analyze the approximate posterior implicitly defined by SAI and uncover key insights into its success. Among other things, we demonstrate how SAI handles symmetries differently than ABI, and examine the role of overparameterization. Further, we investigate the characteristics of approximate posteriors with sampling budgets scaled far beyond previously studied limits and explain why the localized behavior of samplers does not inherently constitute a disadvantage.
MCML Authors
Statistics, Data Science and Machine Learning
Statistics, Data Science and Machine Learning
Statistics, Data Science and Machine Learning
On multi-scale Graph Representation Learning.
LMRL @ICLR 2025 - Workshop on Learning Meaningful Representations of Life at the 13th International Conference on Learning Representations (ICLR 2025). Singapore, Apr 24-28, 2025. To be published. Preprint available. URL
Abstract
While Graph Neural Networks (GNNs) are widely used in modern computational biology, an underexplored drawback of common GNN methods,is that they are not inherently multiscale consistent: Two graphs describing the same object or situation at different resolution scales are assigned vastly different latent representations. This prevents graph networks from generating data representations that are consistent across scales. It also complicates the integration of representations at the molecular scale with those generated at the biological scale. Here we discuss why existing GNNs struggle with multiscale consistency and show how to overcome this problem by modifying the message passing paradigm within GNNs.
MCML Authors
Computer Vision & Artificial Intelligence
Graph Networks struggle with variable Scale.
ICBINB @ICLR 2025 - Workshop I Can’t Believe It’s Not Better: Challenges in Applied Deep Learning at the 13th International Conference on Learning Representations (ICLR 2025). Singapore, Apr 24-28, 2025. To be published. Preprint available. URL
Abstract
Standard graph neural networks assign vastly different latent embeddings to graphs describing the same object at different resolution scales. This precludes consistency in applications and prevents generalization between scales as would fundamentally be needed e.g. in AI4Science. We uncover the underlying obstruction, investigate its origin and show how to overcome it by modifying the message passing paradigm.
MCML Authors
Computer Vision & Artificial Intelligence
On Incorporating Scale into Graph Networks.
MLMP @ICLR 2025 - Workshop on Machine Learning Multiscale Processes at the 13th International Conference on Learning Representations (ICLR 2025). Singapore, Apr 24-28, 2025. MLMP Best Paper Award. To be published. Preprint available. URL
Abstract
Standard graph neural networks assign vastly different latent embeddings to graphs describing the same physical system at different resolution scales. This precludes consistency in applications and prevents generalization between scales as would fundamentally be needed in many scientific applications. We uncover the underlying obstruction, investigate its origin and show how to overcome it.
MCML Authors
Computer Vision & Artificial Intelligence
Differentiable Attention Sparsity via Structured D-Gating.
SLLM @ICLR 2025 - Workshop on Sparsity in LLMs at the 13th International Conference on Learning Representations (ICLR 2025). Singapore, Apr 24-28, 2025. To be published. Preprint available. URL
Abstract
A core component of modern large language models is the attention mechanism, but its immense parameter count necessitates structured sparsity for resource-efficient optimization and inference. Traditional sparsity penalties, such as the group lasso, are non-smooth and thus incompatible with standard stochastic gradient descent methods. To address this, we propose a deep gating mechanism that reformulates the structured sparsity penalty into a fully differentiable optimization problem, allowing effective and principled norm-based group sparsification without requiring specialized non-smooth optimizers. Our theoretical analysis and empirical results demonstrate that this approach enables structured sparsity with simple stochastic gradient descent or variants while maintaining predictive performance.
MCML Authors
Statistical Learning and Data Science
Statistical Learning and Data Science
Statistics, Data Science and Machine Learning
Beyond Single-Step: Multi-Frame Action-Conditiones Video Generation for Reinforcement Learning Environments.
World Models @ICLR 2025 - Workshop on World Models: Understanding, Modelling and Scaling at the 13th International Conference on Learning Representations (ICLR 2025). Singapore, Apr 24-28, 2025. To be published. Preprint available. URL
Abstract
World models achieved great success in learning the dynamics from both low-dimensional and high-dimensional states. Yet, there is no existing work to address multi-step generation task with high dimensional data. In this paper, we propose the first action-conditioned multi-frame video generation model, advancing world
model development by generating future states from actions. As opposed to recent single-step or autoregressive approaches, our model directly generates multiple future frames conditioned on past observations and action sequences. Our framework extends its capabilities to action-conditioned video generation by introducing an action encoder. This addition enables the spatiotemporal variational autoencoder and diffusion transformer in Open-Sora to effectively incorporate action information, ensuring precise and coherent video generation. We evaluated performance on Atari environments (Breakout, Pong, DemonAttack) using MSE, PSNR, and LPIPS. Results show that conditioning solely on future actions and embedding-based encoding improve generation accuracy and perceptual quality while capturing complex temporal dependencies like inertia. Our work paves the way for action-conditioned multi-step generative world models in dynamic environment.
MCML Authors
Structure Is Not Enough: Leveraging Behavior for Neural Network Weight Reconstruction.
Weight Space Learning @ICLR 2025 - Workshop on Weight Space Learning at the 13th International Conference on Learning Representations (ICLR 2025). Singapore, Apr 24-28, 2025. To be published. Preprint available. arXiv
Abstract
The weights of neural networks (NNs) have recently gained prominence as a new data modality in machine learning, with applications ranging from accuracy and hyperparameter prediction to representation learning or weight generation. One approach to leverage NN weights involves training autoencoders (AEs), using contrastive and reconstruction losses. This allows such models to be applied to a wide variety of downstream tasks, and they demonstrate strong predictive performance and low reconstruction error. However, despite the low reconstruction error, these AEs reconstruct NN models with deteriorated performance compared to the original ones, limiting their usability with regard to model weight generation. In this paper, we identify a limitation of weight-space AEs, specifically highlighting that a structural loss, that uses the Euclidean distance between original and reconstructed weights, fails to capture some features critical for reconstructing high-performing models. We analyze the addition of a behavioral loss for training AEs in weight space, where we compare the output of the reconstructed model with that of the original one, given some common input. We show a strong synergy between structural and behavioral signals, leading to increased performance in all downstream tasks evaluated, in particular NN weights reconstruction and generation.
MCML Authors
Applied Statistics in Social Sciences, Economics and Business
MemLLM: Finetuning LLMs to Use An Explicit Read-Write Memory.
NFAM @ICLR 2025 - Workshop on New Frontiers in Associative Memories at the 13th International Conference on Learning Representations (ICLR 2025). Singapore, Apr 24-28, 2025. To be published. Preprint available. arXiv
Abstract
While current large language models (LLMs) demonstrate some capabilities in knowledge-intensive tasks, they are limited by relying on their parameters as an implicit storage mechanism. As a result, they struggle with infrequent knowledge and temporal degradation. In addition, the uninterpretable nature of parametric memorization makes it challenging to understand and prevent hallucination. Parametric memory pools and model editing are only partial solutions. Retrieval Augmented Generation (RAG) – though non-parametric – has its own limitations: it lacks structure, complicates interpretability and makes it hard to effectively manage stored knowledge. In this paper, we introduce MemLLM, a novel method of enhancing LLMs by integrating a structured and explicit read-and-write memory module. MemLLM tackles the aforementioned challenges by enabling dynamic interaction with the memory and improving the LLM’s capabilities in using stored knowledge. Our experiments indicate that MemLLM enhances the LLM’s performance and interpretability, in language modeling in general and knowledge-intensive tasks in particular. We see MemLLM as an important step towards making LLMs more grounded and factual through memory augmentation.
MCML Authors
Computational Linguistics
Uncertainty Quantification for Prior-Fitted Networks using Martingale Posteriors.
FPI @ICLR 2025 - Workshop on Frontiers in Probabilistic Inference: Learning meets Sampling at the 13th International Conference on Learning Representations (ICLR 2025). Singapore, Apr 24-28, 2025. To be published. Preprint available. URL
Abstract
Prior-fitted networks (PFNs) have emerged as promising foundation models for prediction from tabular data sets, achieving state-of-the-art performance on small to moderate data sizes without tuning. While PFNs are motivated by Bayesian ideas, they do not provide any uncertainty quantification for predictive means, quantiles, or similar quantities. We propose a principled and efficient method to construct Bayesian posteriors for such estimates based on Martingale Posteriors. Several simulated and real-world data examples are used to showcase the resulting uncertainty quantification of our method in inference applications.
MCML Authors
Computational Statistics & Data Science
Statistics, Data Science and Machine Learning
Can Transformers Learn Full Bayesian Inference in Context?
FPI @ICLR 2025 - Workshop on Frontiers in Probabilistic Inference: Learning meets Sampling at the 13th International Conference on Learning Representations (ICLR 2025). Singapore, Apr 24-28, 2025. To be published. Preprint available. arXiv
Abstract
Transformers have emerged as the dominant architecture in the field of deep learning, with a broad range of applications and remarkable in-context learning (ICL) capabilities. While not yet fully understood, ICL has already proved to be an intriguing phenomenon, allowing transformers to learn in context – without requiring further training. In this paper, we further advance the understanding of ICL by demonstrating that transformers can perform full Bayesian inference for commonly used statistical models in context. More specifically, we introduce a general framework that builds on ideas from prior fitted networks and continuous normalizing flows which enables us to infer complex posterior distributions for methods such as generalized linear models and latent factor models. Extensive experiments on real-world datasets demonstrate that our ICL approach yields posterior samples that are similar in quality to state-of-the-art MCMC or variational inference methods not operating in context.
MCML Authors
Statistics, Data Science and Machine Learning
Can Transformers Learn Full Bayesian Inference in Context?
SynthData @ICLR 2025 - Workshop SynthData: Will Synthetic Data Finally Solve the Data Access Problem? at the 13th International Conference on Learning Representations (ICLR 2025). Singapore, Apr 24-28, 2025. To be published. Preprint available. arXiv
Abstract
Transformers have emerged as the dominant architecture in the field of deep learning, with a broad range of applications and remarkable in-context learning (ICL) capabilities. While not yet fully understood, ICL has already proved to be an intriguing phenomenon, allowing transformers to learn in context – without requiring further training. In this paper, we further advance the understanding of ICL by demonstrating that transformers can perform full Bayesian inference for commonly used statistical models in context. More specifically, we introduce a general framework that builds on ideas from prior fitted networks and continuous normalizing flows which enables us to infer complex posterior distributions for methods such as generalized linear models and latent factor models. Extensive experiments on real-world datasets demonstrate that our ICL approach yields posterior samples that are similar in quality to state-of-the-art MCMC or variational inference methods not operating in context.
MCML Authors
Statistics, Data Science and Machine Learning
Efficiently Warmstarting MCMC for BNNS.
FPI @ICLR 2025 - Workshop on Frontiers in Probabilistic Inference: Learning meets Sampling at the 13th International Conference on Learning Representations (ICLR 2025). Singapore, Apr 24-28, 2025. To be published. Preprint available. URL
Abstract
Markov Chain Monte Carlo (MCMC) algorithms are widely regarded as the gold standard for approximate inference in Bayesian neural networks (BNNs). However, they remain computationally expensive and prone to inefficiencies, such
as dying samplers, frequently leading to substantial waste of computational resources. While prior work has presented warmstarting techniques as an effective method to mitigate these inefficiencies, we provide a more comprehensive empirical analysis of how initializations of samplers affect their behavior. Based on various experiments examining the dynamics of warmstarting MCMC, we propose novel warmstarting strategies that leverage performance predictors and adaptive termination criteria to achieve better-performing, yet more cost-efficient, models. In numerical experiments, we demonstrate that this approach provides a practical pathway to more resource-efficient approximate inference in BNNs.
MCML Authors
Statistical Learning and Data Science
Statistics, Data Science and Machine Learning
Statistical Learning and Data Science
Statistics, Data Science and Machine Learning
Statistical Learning and Data Science
Trust Me, I Know the Way: Predictive Uncertainty in the Presence of Shortcut Learning.
SCSL @ICLR 2025 - Workshop on Spurious Correlation and Shortcut Learning: Foundations and Solutions at the 13th International Conference on Learning Representations (ICLR 2025). Singapore, Apr 24-28, 2025. To be published. Preprint available. arXiv
Abstract
The correct way to quantify predictive uncertainty in neural networks remains a topic of active discussion. In particular, it is unclear whether the state-of-the art entropy decomposition leads to a meaningful representation of model, or epistemic, uncertainty (EU) in the light of a debate that pits ignorance against disagreement perspectives. We aim to reconcile the conflicting viewpoints by arguing that both are valid but arise from different learning situations. Notably, we show that the presence of shortcuts is decisive for EU manifesting as disagreement.
MCML Authors
Statistical Learning and Data Science
Statistical Learning and Data Science
Statistical Learning and Data Science
23.04.2025
Related
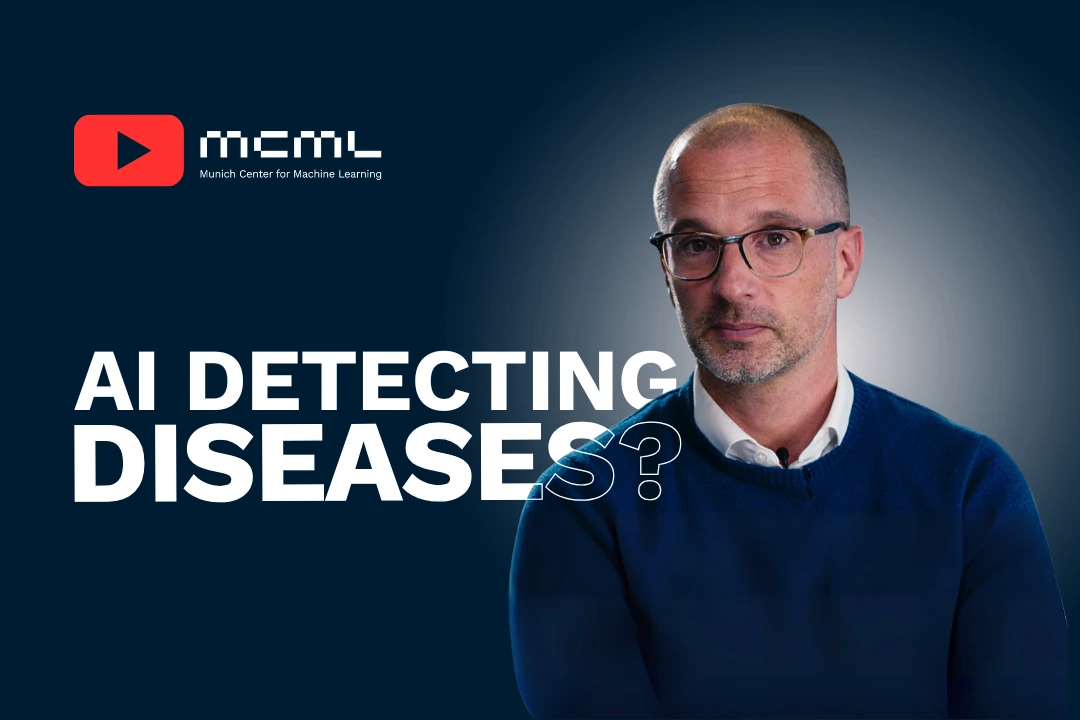
25.06.2025
When Clinical Expertise Meets AI Innovation – With Michael Ingrisch
The new research film features Michael Ingrisch, who shows how AI and clinical expertise can solve real challenges in radiology together.
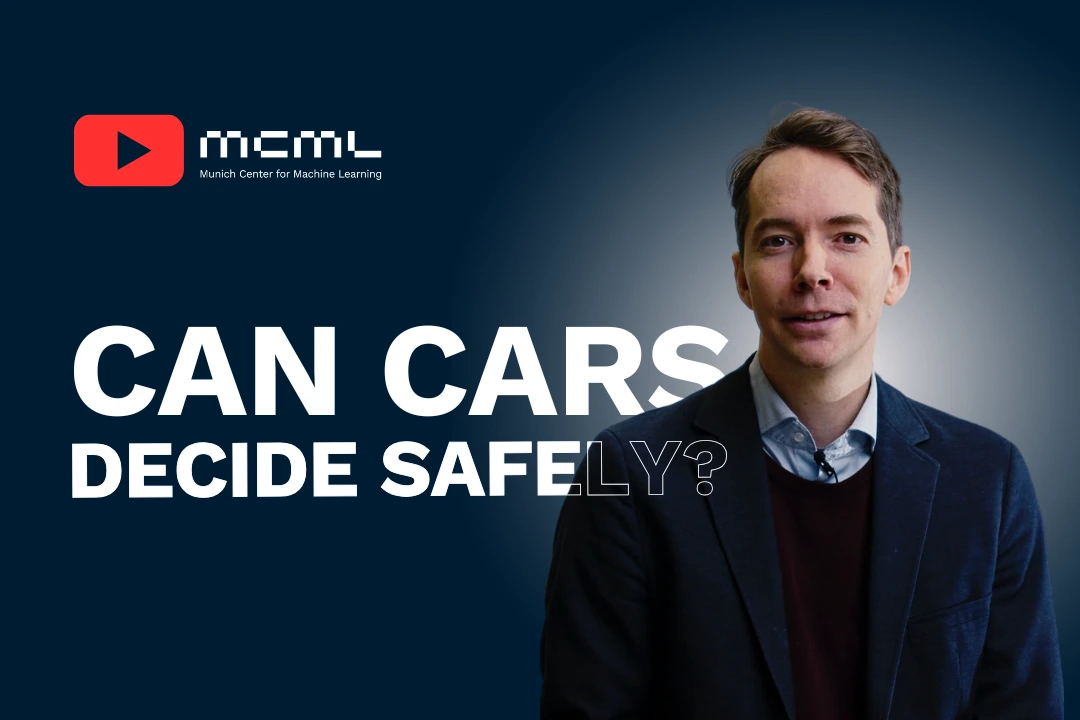
23.06.2025
Autonomous Driving: From Infinite Possibilities to Safe Decisions— With Matthias Althoff
The new research film features Matthias Althoff explaining how his team verifies autonomous vehicle safety using EDGAR and rigorous testing.
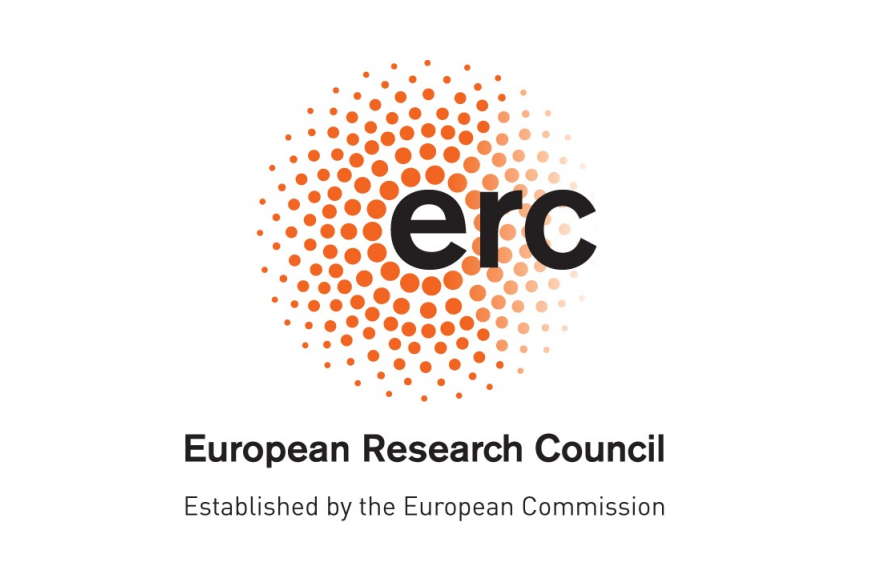
20.06.2025
ERC Advanced Grant for Massimo Fornasier
Massimo Fornasier was awarded ERC Advanced Grant to develop advanced algorithms for solving complex nonconvex optimization problems.
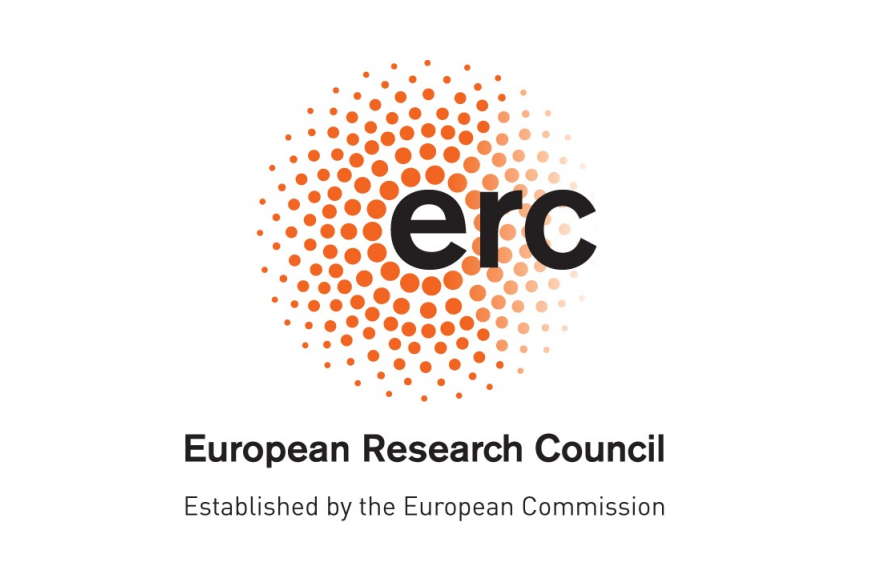
18.06.2025
ERC Advanced Grant for Albrecht Schmidt
Albrecht Schmidt receives ERC Advanced Grant for research on personalized generative AI to support memory, planning, and creativity.