02.05.2025
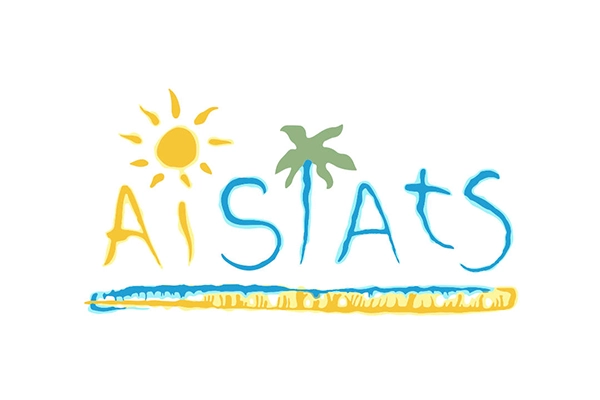
MCML Researchers With Four Papers at AISTATS 2025
28th International Conference on Artificial Intelligence and Statistics (AISTATS 2025). Mai Khao, Thailand, 29.04.2025–05.05.2024
We are happy to announce that MCML researchers are represented with four papers at AISTATS 2025. Congrats to our researchers!
Main Track (4 papers)
Get rid of your constraints and reparametrize: A study in NNLS and implicit bias.
AISTATS 2025 - 28th International Conference on Artificial Intelligence and Statistics. Mai Khao, Thailand, May 03-05, 2025. To be published. URL
Abstract
Over the past years, there has been significant interest in understanding the implicit bias of gradient descent optimization and its connection to the generalization properties of overparametrized neural networks. Several works observed that when training linear diagonal networks on the square loss for regression tasks (which corresponds to overparametrized linear regression) gradient descent converges to special solutions, e.g., non-negative ones. We connect this observation to Riemannian optimization and view overparametrized GD with identical initialization as a Riemannian GD. We use this fact for solving non-negative least squares (NNLS), an important problem behind many techniques, e.g., non-negative matrix factorization. We show that gradient flow on the reparametrized objective converges globally to NNLS solutions, providing convergence rates also for its discretized counterpart. Unlike previous methods, we do not rely on the calculation of exponential maps or geodesics. We further show accelerated convergence using a second-order ODE, lending itself to accelerated descent methods. Finally, we establish the stability against negative perturbations and discuss generalization to other constrained optimization problems.
MCML Authors
Mathematical Data Science and Artificial Intelligence
Paths and Ambient Spaces in Neural Loss Landscapes.
AISTATS 2025 - 28th International Conference on Artificial Intelligence and Statistics. Mai Khao, Thailand, May 03-05, 2025. To be published. URL
Abstract
Understanding the structure of neural network loss surfaces, particularly the emergence of low-loss tunnels, is critical for advancing neural network theory and practice. In this paper, we propose a novel approach to directly embed loss tunnels into the loss landscape of neural networks. Exploring the properties of these loss tunnels offers new insights into their length and structure and sheds light on some common misconceptions. We then apply our approach to Bayesian neural networks, where we improve subspace inference by identifying pitfalls and proposing a more natural prior that better guides the sampling procedure.
MCML Authors
Statistics, Data Science and Machine Learning
Computational Statistics & Data Science
Statistics, Data Science and Machine Learning
Statistics, Data Science and Machine Learning
Incremental Uncertainty-aware Performance Monitoring with Active Labeling Intervention.
AISTATS 2025 - 28th International Conference on Artificial Intelligence and Statistics. Mai Khao, Thailand, May 03-05, 2025. To be published. URL
Abstract
We study the problem of monitoring machine learning models under gradual distribution shifts, where circumstances change slowly over time, often leading to unnoticed yet significant declines in accuracy. To address this, we propose Incremental Uncertainty-aware Performance Monitoring (IUPM), a novel label-free method that estimates performance changes by modeling gradual shifts using optimal transport. In addition, IUPM quantifies the uncertainty in the performance prediction and introduces an active labeling procedure to restore a reliable estimate under a limited labeling budget. Our experiments show that IUPM outperforms existing performance estimation baselines in various gradual shift scenarios and that its uncertainty awareness guides label acquisition more effectively compared to other strategies.
MCML Authors
Additive Model Boosting: New Insights and Path(ologie)s.
AISTATS 2025 - 28th International Conference on Artificial Intelligence and Statistics. Mai Khao, Thailand, May 03-05, 2025. Oral Presentation. To be published. Preprint available. URL
Abstract
Additive models (AMs) have sparked a lot of interest in machine learning recently, allowing the incorporation of interpretable structures into a wide range of model classes. Many commonly used approaches to fit a wide variety of potentially complex additive models build on the idea of boosting additive models. While boosted additive models (BAMs) work well in practice, certain theoretical aspects are still poorly understood, including general convergence behavior and what optimization problem is being solved when accounting for the implicit regularizing nature of boosting. In this work, we study the solution paths of BAMs and establish connections with other approaches for certain classes of problems. Along these lines, we derive novel convergence results for BAMs, which yield crucial insights into the inner workings of the method. While our results generally provide reassuring theoretical evidence for the practical use of BAMs, they also uncover some ‘pathologies’ of boosting for certain additive model classes concerning their convergence behavior that require caution in practice. We empirically validate our theoretical findings through several numerical experiments.
MCML Authors
Statistics, Data Science and Machine Learning
Statistics, Data Science and Machine Learning
02.05.2025
Related
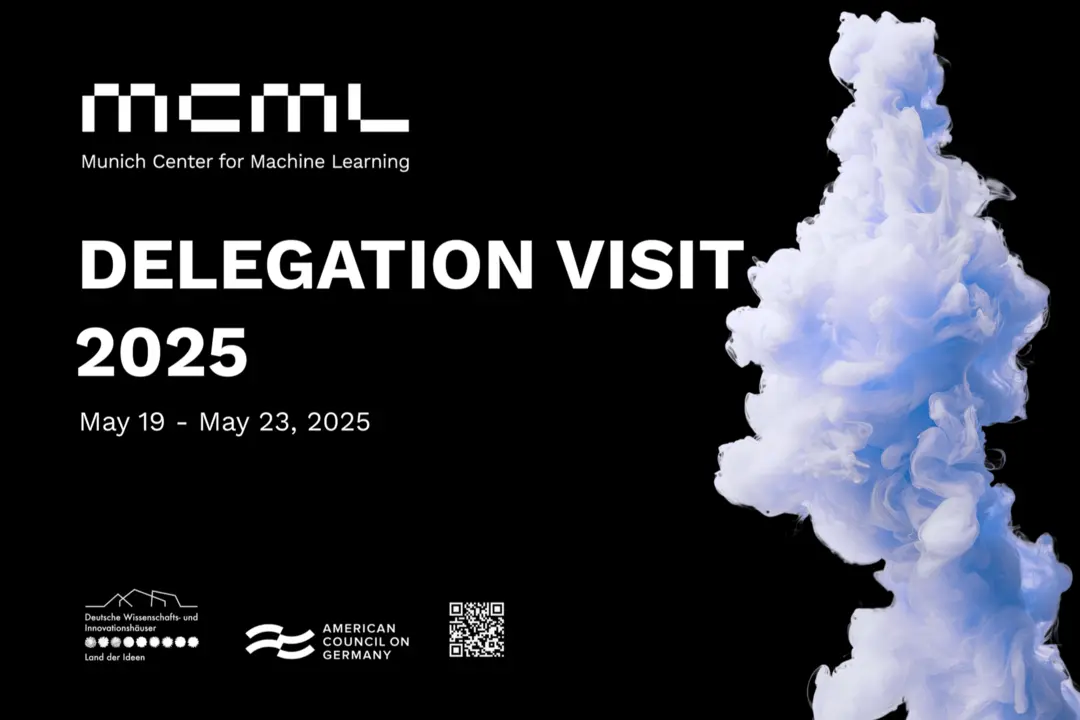
28.04.2025
MCML Delegation Visit to the USA
MCML delegation visits top US institutions to foster AI research collaborations in Generative and Medical AI, May 19–23, 2025.
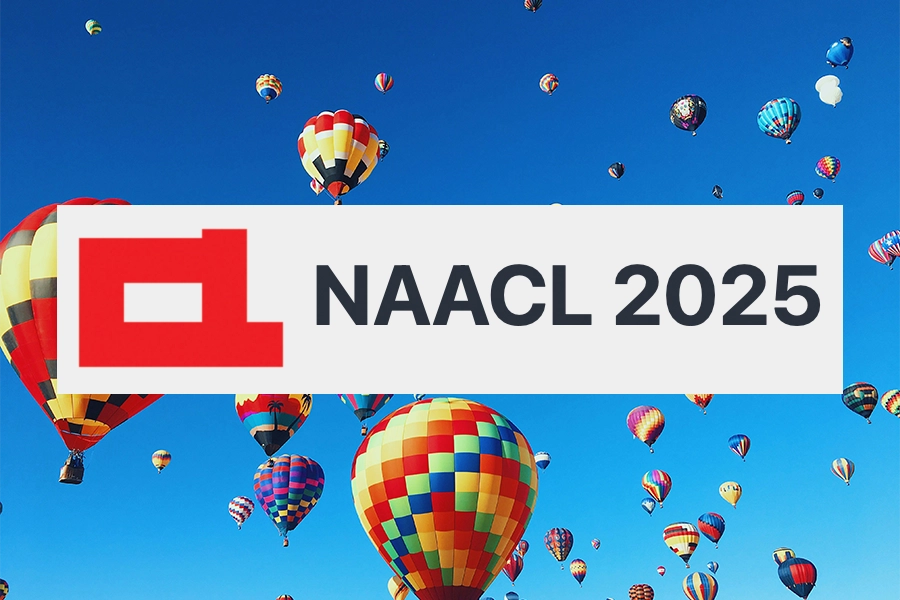
28.04.2025
MCML Researchers With Eleven Papers at NAACL 2025
Annual Conference of the North American Chapter of the Association for Computational Linguistics (NAACL 2025). Albuquerque, NM, USA, 29.04.2025 - 04.05.2024
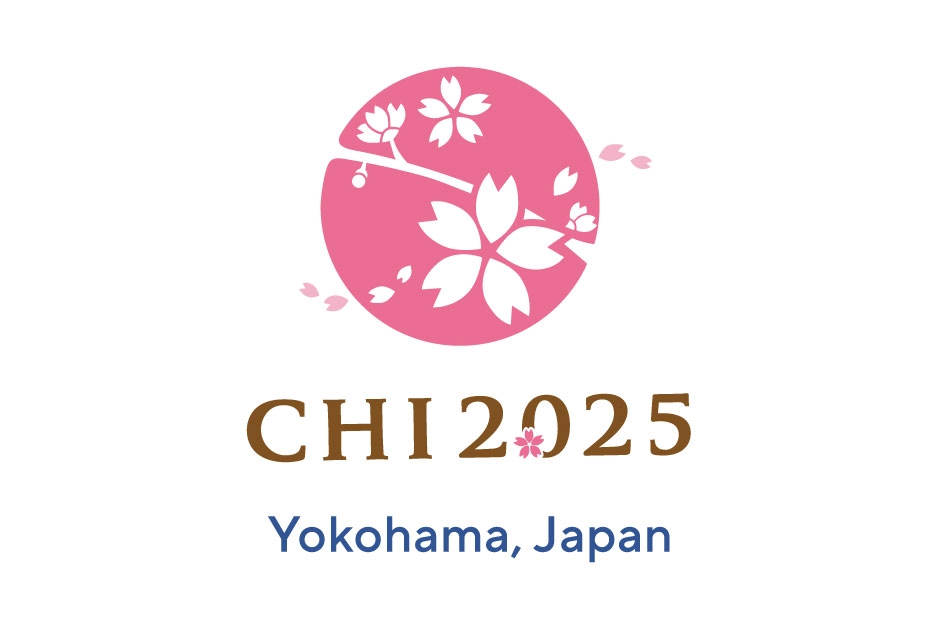
25.04.2025
MCML Researchers With Seven Papers at CHI 2025
Conference on Human Factors in Computing Systems (CHI 2025). Yokohama, Japan, 26.04.2025 - 01.05.2024
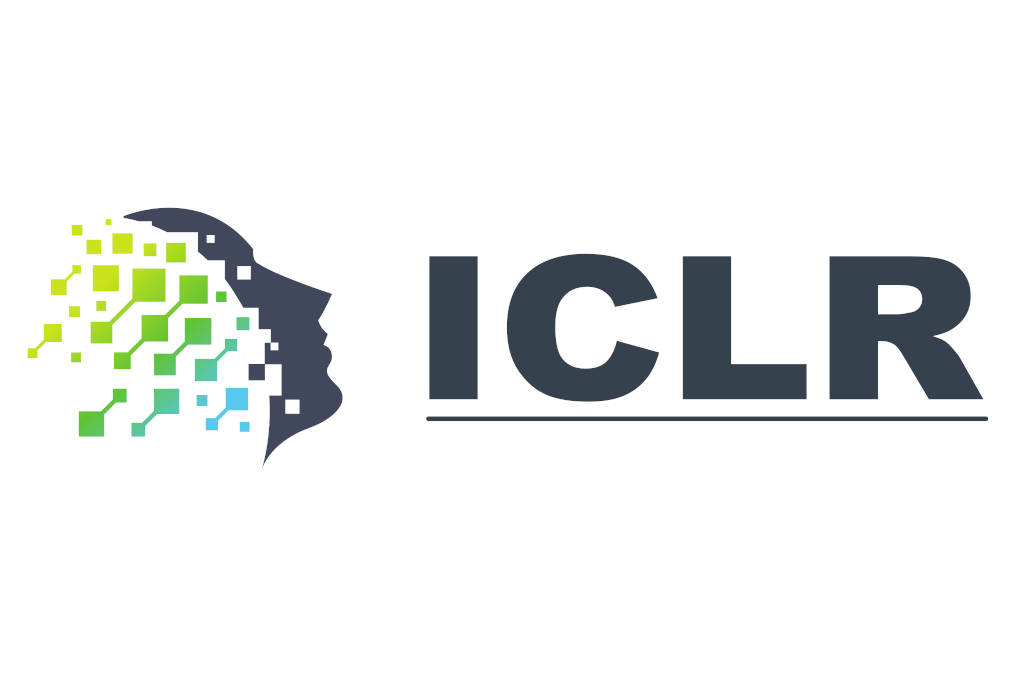
23.04.2025
MCML Researchers With 51 Papers at ICLR 2025
13th International Conference on Learning Representations (ICLR 2025). Singapore, 24.04.2025 - 28.04.2024