11.02.2025
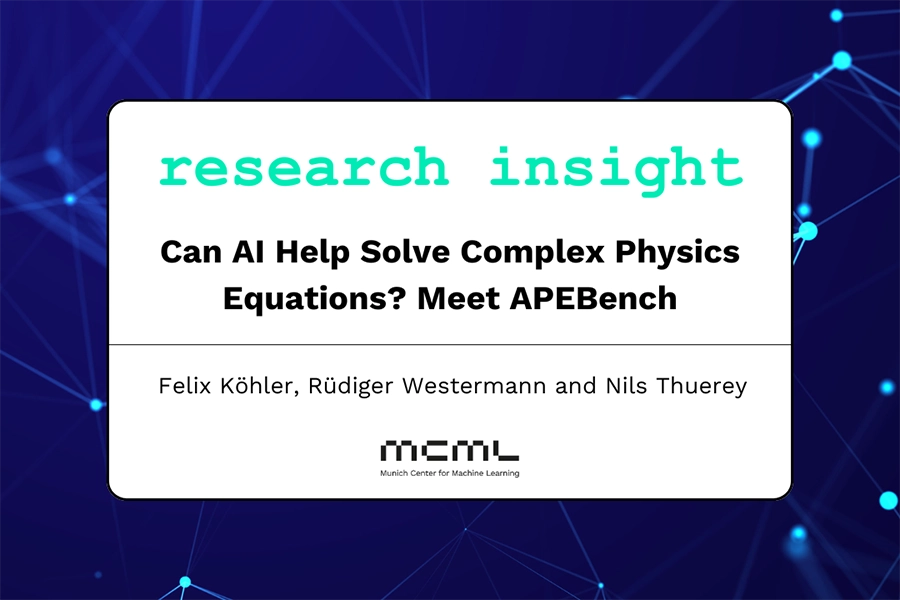
Can AI Help Solve Complex Physics Equations? Meet APEBench
MCML Research Insight - With Felix Köhler, Rüdiger Westermann and Nils Thuerey
Our Junior Member Felix Köhler, together with our PIs Rüdiger Westermann and Nils Thuerey, and collaborator Simon Niedermayr, have introduced APEBench, an innovative benchmark suite. APEBench sets a new standard for evaluating autoregressive neural emulators, which are designed to solve partial differential equations (PDEs)—the fundamental mathematical framework for modeling natural phenomena such as weather patterns, fluid dynamics, and chemical reactions.
«APEBench is a comprehensive benchmark suite to evaluate autoregressive neural emulators for solving partial differential equations (PDEs).»
Felix Köhler
MCML Junior Member
What is APEBench?
Partial Differential Equations (PDEs) are the backbone of many scientific fields, modeling everything from ocean currents to climate patterns. However, solving them accurately demands massive computational resources. Traditional numerical solvers provide precision but at a high cost, while AI-based alternatives offer speed but struggle with stability. To address this challenge, the authors introduce APEBench, a benchmark designed to evaluate and enhance AI-based PDE solvers.
APEBench is an open-source benchmark that evaluates neural emulators—AI models trained to approximate complex PDE solutions. Unlike traditional simulators, which compute every detail from first principles, emulators learn from data to make fast, step-by-step predictions. But how do we know if these AI models are reliable? That’s where APEBench’s key contributions come in.
«APEBench bridges the gap between neural emulators and classical solvers, enabling insights into long-term stability and performance.»
Felix Köhler
MCML Junior Member
Four Key Innovations of APEBench
1) A Large Selection of PDEs
APEBench includes 46 different PDE scenarios across 1D, 2D, and 3D, covering a wide range of real-world physics problems. This diversity allows researchers to compare AI models across simple and complex systems, ensuring that AI-driven solvers are tested under realistic conditions.
2) A Unique System for Identifying PDE Dynamics
Unlike previous benchmarks, APEBench provides a structured way to classify PDEs based on their mathematical properties. It introduces a unique identifier system that links each PDE to well-known stability criteria from classical numerical methods. This allows researchers to quantify the difficulty of solving a given PDE and compare how different AI models handle complex dynamics.
3) A Differentiable Simulation Suite
APEBench includes a JAX-based differentiable simulator, enabling AI models to interact directly with physics-based solvers during training. This means that instead of relying on precomputed datasets, models can be trained with real-time simulation feedback, improving their accuracy and adaptability. This feature is especially valuable for hybrid AI-solvers, which blend AI with traditional numerical techniques.
4) A New Approach to Evaluating Long-Term Accuracy
Traditional PDE benchmarks often focus on short-term performance, but in real-world applications, long-term stability is crucial. APEBench introduces rollout metrics, which track how well an AI model generalizes over extended simulations. By analyzing these metrics, researchers can identify which training methods lead to more stable and reliable AI solvers.
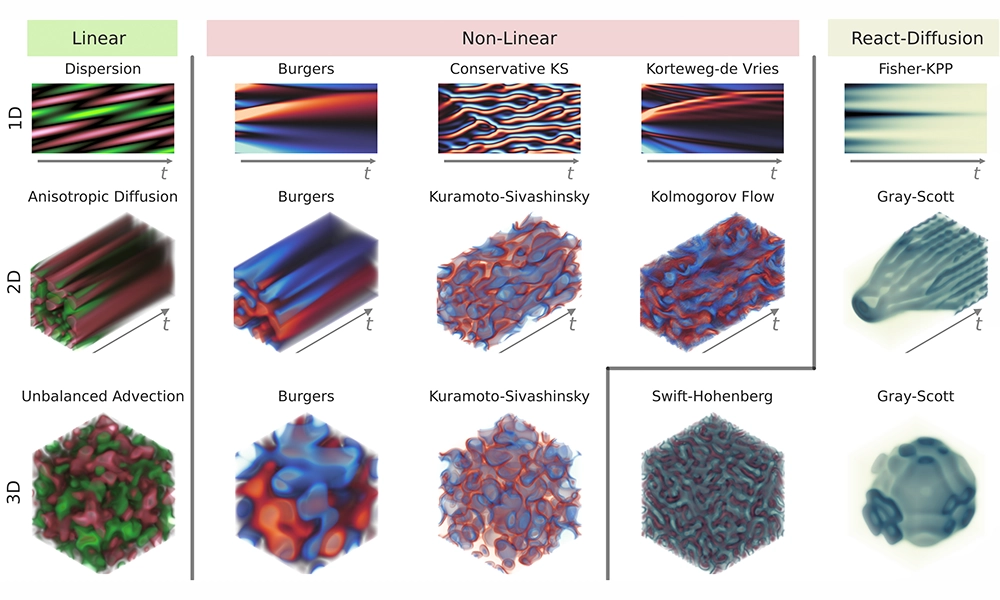
©Felix Köhler, Rüdiger Westermann and Nils Thuerey
Examples of the 46 PDE dynamics
Why Does This Matter?
Neural emulators have the potential to dramatically accelerate PDE simulations, making applications like weather prediction, climate modeling, and engineering simulations more efficient. APEBench provides a structured and rigorous way to evaluate these AI-driven solvers, ensuring that they are not only fast but also accurate and stable over time.
Discover More
Want to see APEBench in action? Check out the animated visual summary on the APEBench Project Page.
Felix Köhler also created a Quickstart Video to help you dive in—check it out on YouTube.
Explore the full paper, published at NeurIPS 2024, including its methodology, experiments, and future directions:
APEBench: A Benchmark for Autoregressive Neural Emulators of PDEs.
NeurIPS 2024 - 38th Conference on Neural Information Processing Systems. Vancouver, Canada, Dec 10-15, 2024. URL GitHub
Abstract
We introduce the Autoregressive PDE Emulator Benchmark (APEBench), a comprehensive benchmark suite to evaluate autoregressive neural emulators for solving partial differential equations. APEBench is based on JAX and provides a seamlessly integrated differentiable simulation framework employing efficient pseudo-spectral methods, enabling 46 distinct PDEs across 1D, 2D, and 3D. Facilitating systematic analysis and comparison of learned emulators, we propose a novel taxonomy for unrolled training and introduce a unique identifier for PDE dynamics that directly relates to the stability criteria of classical numerical methods. APEBench enables the evaluation of diverse neural architectures, and unlike existing benchmarks, its tight integration of the solver enables support for differentiable physics training and neural-hybrid emulators. Moreover, APEBench emphasizes rollout metrics to understand temporal generalization, providing insights into the long-term behavior of emulating PDE dynamics. In several experiments, we highlight the similarities between neural emulators and numerical simulators.
MCML Authors
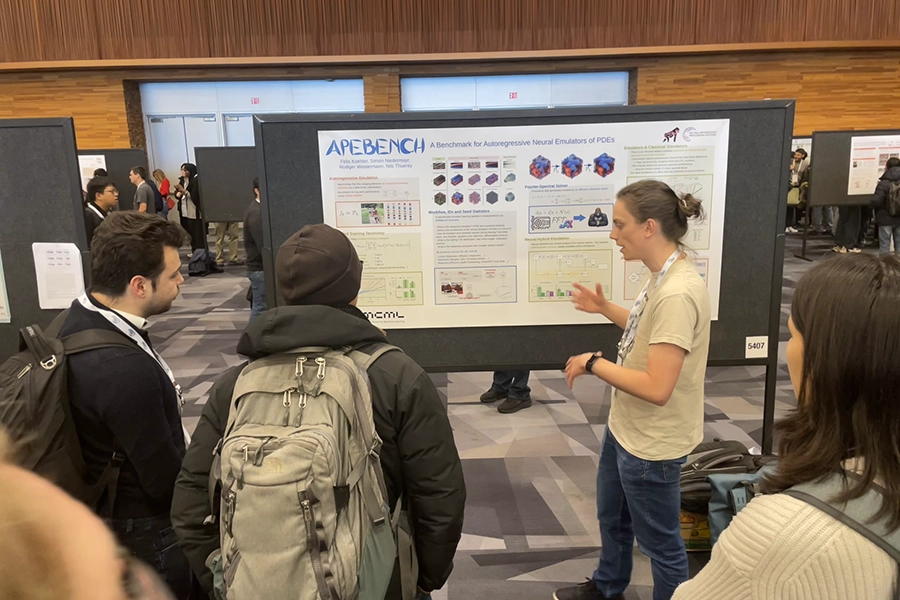
©Felix Köhler
Behind the scenes: Snapshot from the poster session at NeurIPS 2024
Share Your Research!
Get in touch with us!
Are you an MCML Junior Member and interested in showcasing your research on our blog?
We’re happy to feature your work—get in touch with us to present your paper.
11.02.2025
Related
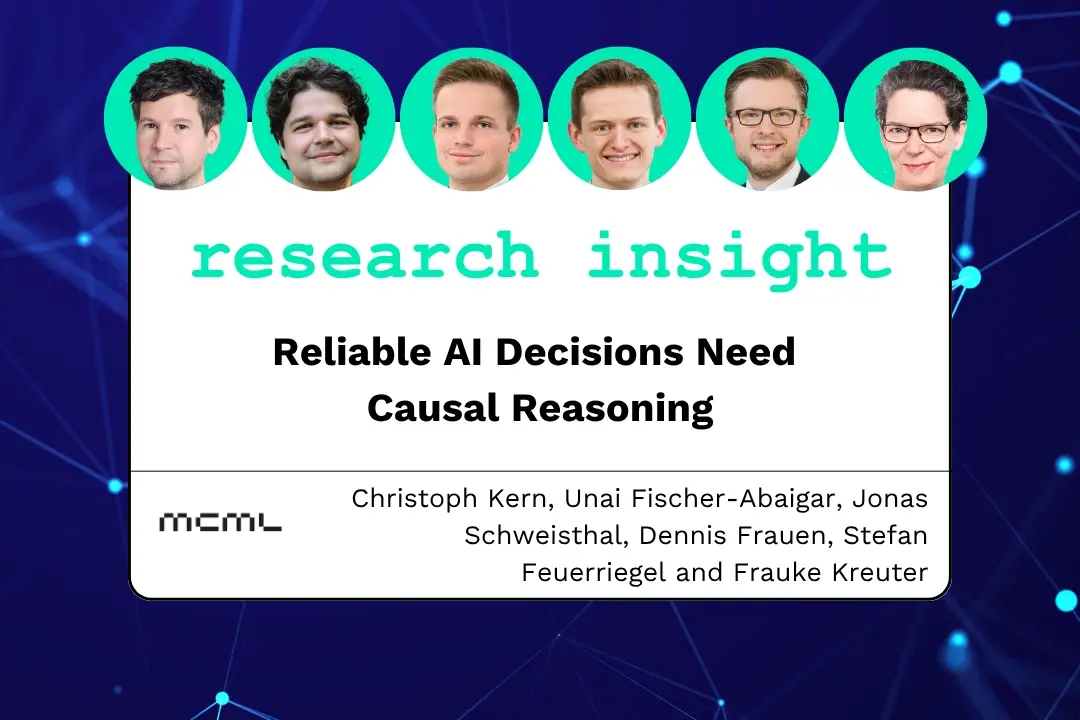
12.06.2025
Why Causal Reasoning Is Crucial for Reliable AI Decisions
Christoph Kern and colleagues show why causal reasoning is key for AI to make reliable, real-world decisions—not just predictions.
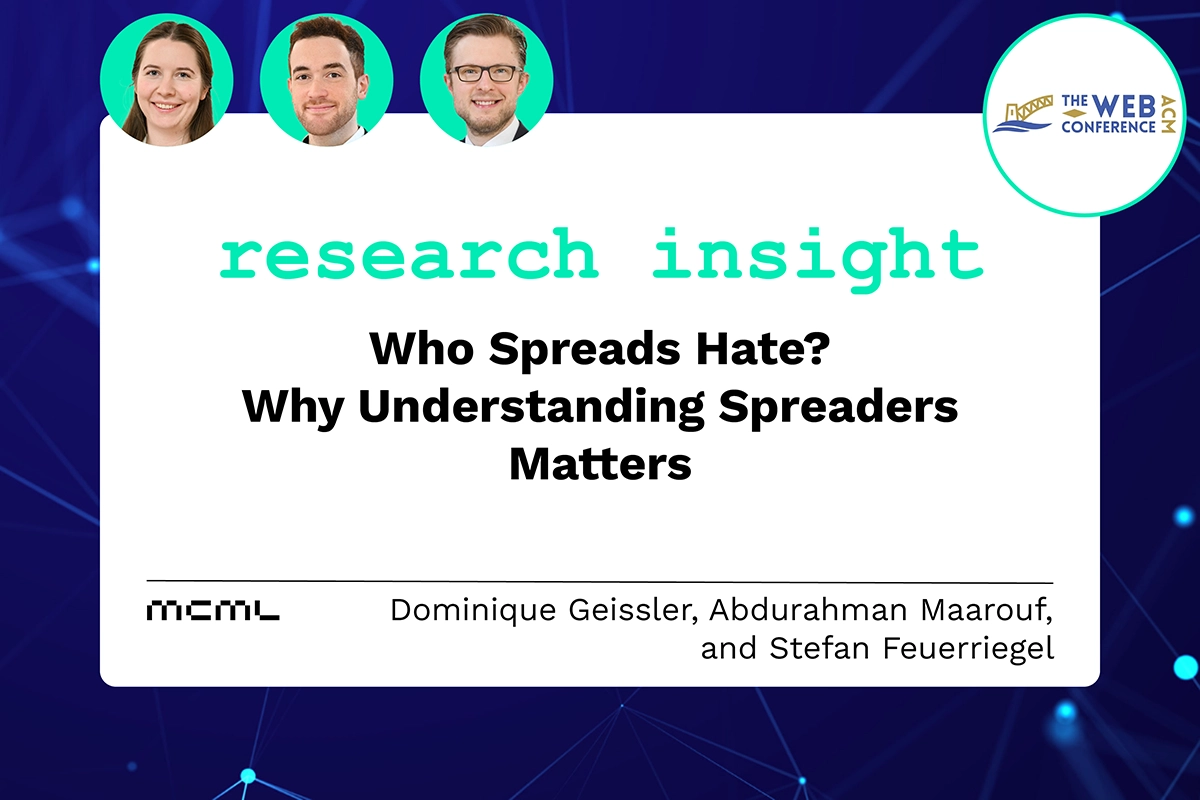
30.04.2025
Who Spreads Hate?
Dominique Geissler, Abdurahman Maarouf, and Stefan Feuerriegel reveal who spreads hate speech online by analyzing user characteristics.
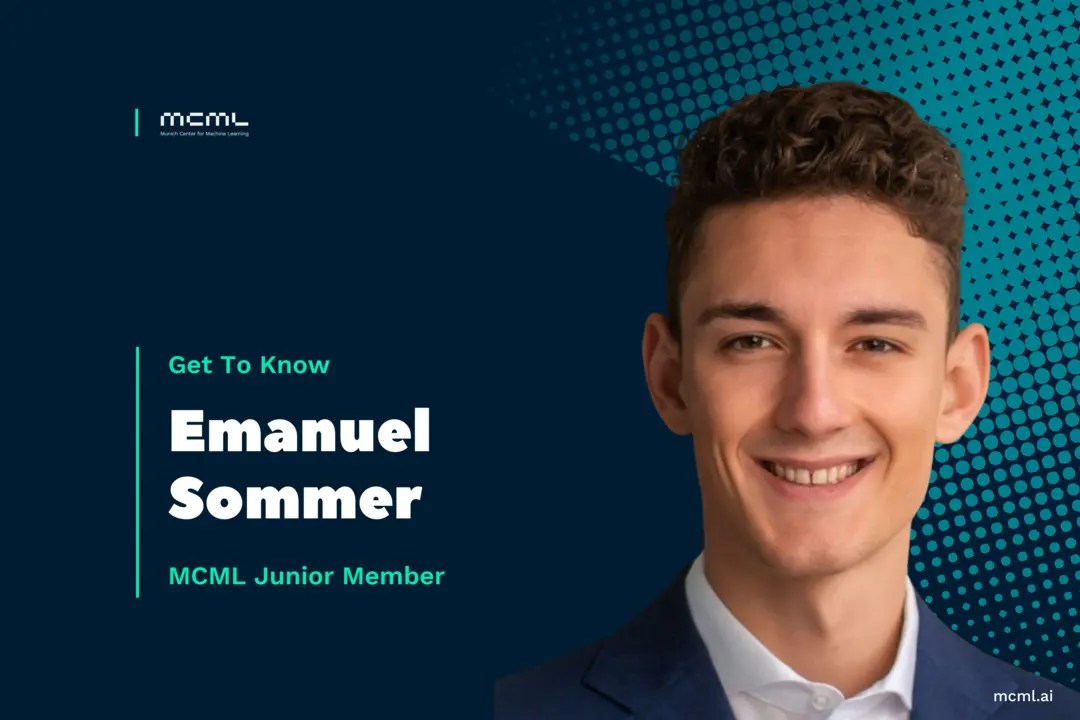
29.04.2025
How Certain Is AI? an Introduction to Bayesian Deep Learning
Researcher in Focus Emanuel Sommer improves uncertainty estimation in deep learning using scalable Bayesian methods for more trustworthy AI.
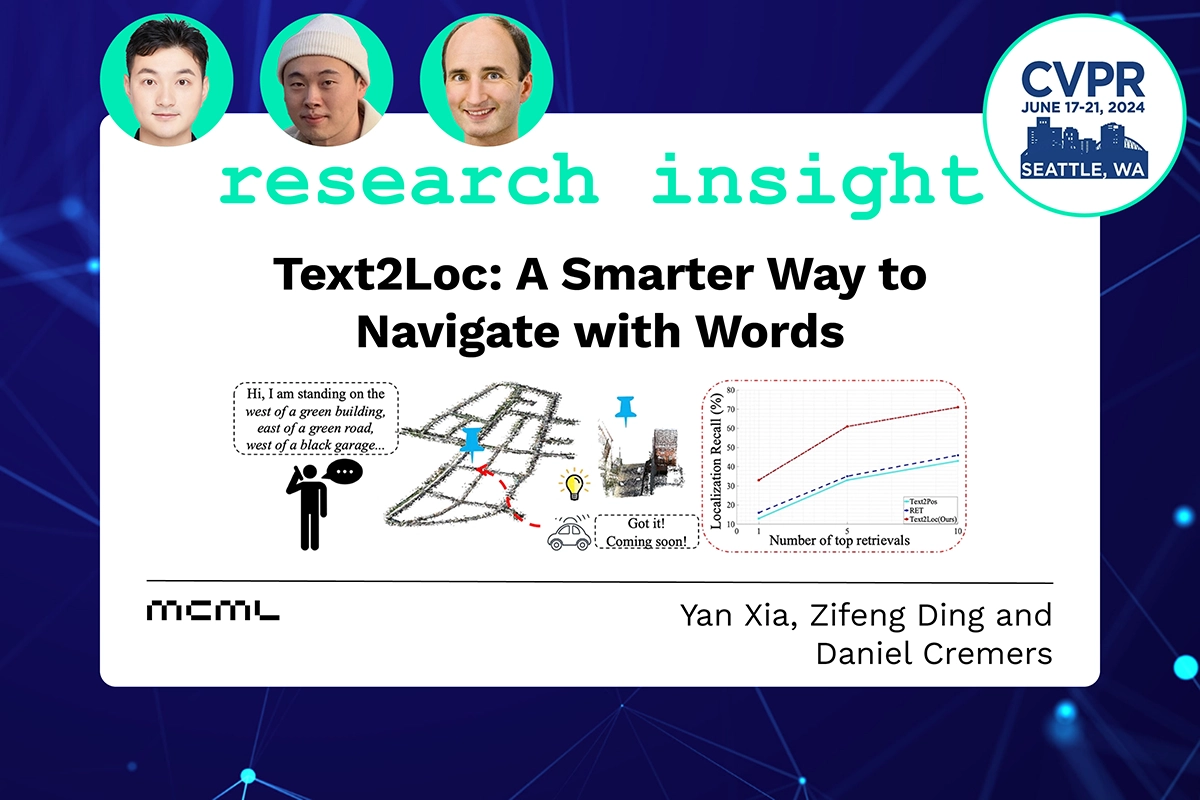
10.04.2025
Text2Loc: A Smarter Way to Navigate With Words
The team of MCML Director Daniel Cremers introduce Text2Loc, a solution designed for city-scale position localization using textual descriptions.