26.09.2024
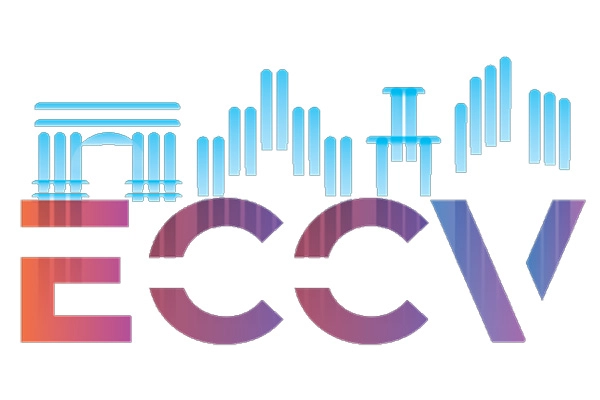
MCML Researchers With 29 Papers at ECCV 2024
18th European Conference on Computer Vision (ECCV 2024). Milano, Italy, 29.09.2024–04.10.2024
We are happy to announce that MCML researchers are represented with 29 papers at ECCV 2024. Congrats to our researchers!
Main Track (23 papers)
Embracing Events and Frames with Hierarchical Feature Refinement Network for Object Detection.
ECCV 2024 - 18th European Conference on Computer Vision. Milano, Italy, Sep 29-Oct 04, 2024. DOI GitHub
Abstract
In frame-based vision, object detection faces substantial performance degradation under challenging conditions due to the limited sensing capability of conventional cameras. Event cameras output sparse and asynchronous events, providing a potential solution to solve these problems. However, effectively fusing two heterogeneous modalities remains an open issue. In this work, we propose a novel hierarchical feature refinement network for event-frame fusion. The core concept is the design of the coarse-to-fine fusion module, denoted as the cross-modality adaptive feature refinement (CAFR) module. In the initial phase, the bidirectional cross-modality interaction (BCI) part facilitates information bridging from two distinct sources. Subsequently, the features are further refined by aligning the channel-level mean and variance in the two-fold adaptive feature refinement (TAFR) part. We conducted extensive experiments on two benchmarks: the low-resolution PKU-DDD17-Car dataset and the high-resolution DSEC dataset. Experimental results show that our method surpasses the state-of-the-art by an impressive margin of 8% on the DSEC dataset. Besides, our method exhibits significantly better robustness (69.5% versus 38.7%) when introducing 15 different corruption types to the frame images.
MCML Authors
Geometry Fidelity for Spherical Images.
ECCV 2024 - 18th European Conference on Computer Vision. Milano, Italy, Sep 29-Oct 04, 2024. DOI
Abstract
Spherical or omni-directional images offer an immersive visual format appealing to a wide range of computer vision applications. However, geometric properties of spherical images pose a major challenge for models and metrics designed for ordinary 2D images. Here, we show that direct application of Fréchet Inception Distance (FID) is insufficient for quantifying geometric fidelity in spherical images. We introduce two quantitative metrics accounting for geometric constraints, namely Omnidirectional FID (OmniFID) and Discontinuity Score (DS). OmniFID is an extension of FID tailored to additionally capture field-of-view requirements of the spherical format by leveraging cubemap projections. DS is a kernel-based seam alignment score of continuity across borders of 2D representations of spherical images. In experiments, OmniFID and DS quantify geometry fidelity issues that are undetected by FID.
MCML Authors
FMBoost: Boosting Latent Diffusion with Flow Matching.
ECCV 2024 - 18th European Conference on Computer Vision. Milano, Italy, Sep 29-Oct 04, 2024. Oral Presentation. DOI
Abstract
Visual synthesis has recently seen significant leaps in performance, largely due to breakthroughs in generative models. Diffusion models have been a key enabler, as they excel in image diversity. However, this comes at the cost of slow training and synthesis, which is only partially alleviated by latent diffusion. To this end, flow matching is an appealing approach due to its complementary characteristics of faster training and inference but less diverse synthesis. We demonstrate our FMBoost approach, which introduces flow matching between a frozen diffusion model and a convolutional decoder that enables high-resolution image synthesis at reduced computational cost and model size. A small diffusion model can then effectively provide the necessary visual diversity, while flow matching efficiently enhances resolution and detail by mapping the small to a high-dimensional latent space, producing high-resolution images. Combining the diversity of diffusion models, the efficiency of flow matching, and the effectiveness of convolutional decoders, state-of-the-art high-resolution image synthesis is achieved at 10242 pixels with minimal computational cost. Cascading FMBoost optionally boosts this further to 20482 pixels. Importantly, this approach is orthogonal to recent approximation and speed-up strategies for the underlying model, making it easily integrable into the various diffusion model frameworks.
MCML Authors
RGNet: A Unified Clip Retrieval and Grounding Network for Long Videos.
ECCV 2024 - 18th European Conference on Computer Vision. Milano, Italy, Sep 29-Oct 04, 2024. DOI GitHub
Abstract
Locating specific moments within long videos (20–120 min) presents a significant challenge, akin to finding a needle in a haystack. Adapting existing short video (5–30 s) grounding methods to this problem yields poor performance. Since most real-life videos, such as those on YouTube and AR/VR, are lengthy, addressing this issue is crucial. Existing methods typically operate in two stages: clip retrieval and grounding. However, this disjoint process limits the retrieval module’s fine-grained event understanding, crucial for specific moment detection. We propose RGNet which deeply integrates clip retrieval and grounding into a single network capable of processing long videos into multiple granular levels, e.g., clips and frames. Its core component is a novel transformer encoder, RG-Encoder, that unifies the two stages through shared features and mutual optimization. The encoder incorporates a sparse attention mechanism and an attention loss to model both granularity jointly. Moreover, we introduce a contrastive clip sampling technique to mimic the long video paradigm closely during training. RGNet surpasses prior methods, showcasing state-of-the-art performance on long video temporal grounding (LVTG) datasets MAD and Ego4D.
MCML Authors
DiffCD: A Symmetric Differentiable Chamfer Distance for Neural Implicit Surface Fitting.
ECCV 2024 - 18th European Conference on Computer Vision. Milano, Italy, Sep 29-Oct 04, 2024. DOI GitHub
Abstract
Neural implicit surfaces can be used to recover accurate 3D geometry from imperfect point clouds. In this work, we show that state-of-the-art techniques work by minimizing an approximation of a one-sided Chamfer distance. This shape metric is not symmetric, as it only ensures that the point cloud is near the surface but not vice versa. As a consequence, existing methods can produce inaccurate reconstructions with spurious surfaces. Although one approach against spurious surfaces has been widely used in the literature, we theoretically and experimentally show that it is equivalent to regularizing the surface area, resulting in over-smoothing. As a more appealing alternative, we propose DiffCD, a novel loss function corresponding to the symmetric Chamfer distance. In contrast to previous work, DiffCD also assures that the surface is near the point cloud, which eliminates spurious surfaces without the need for additional regularization. We experimentally show that DiffCD reliably recovers a high degree of shape detail, substantially outperforming existing work across varying surface complexity and noise levels.
MCML Authors
Computer Vision & Artificial Intelligence
Imaging with Confidence: Uncertainty Quantification for High-dimensional Undersampled MR Images.
ECCV 2024 - 18th European Conference on Computer Vision. Milano, Italy, Sep 29-Oct 04, 2024. DOI GitHub
Abstract
Establishing certified uncertainty quantification (UQ) in imaging processing applications continues to pose a significant challenge. In particular, such a goal is crucial for accurate and reliable medical imaging if one aims for precise diagnostics and appropriate intervention. In the case of magnetic resonance imaging, one of the essential tools of modern medicine, enormous advancements in fast image acquisition were possible after the introduction of compressive sensing and, more recently, deep learning methods. Still, as of now, there is no UQ method that is both fully rigorous and scalable. This work takes a step towards closing this gap by proposing a total variation minimization-based method for pixel-wise sharp confidence intervals for undersampled MRI. We demonstrate that our method empirically achieves the predicted confidence levels. We expect that our approach will also have implications for other imaging modalities as well as deep learning applications in computer vision.
MCML Authors
Mathematical Data Science and Artificial Intelligence
Representation Enhancement-Stabilization: Reducing Bias-Variance of Domain Generalization.
ECCV 2024 - 18th European Conference on Computer Vision. Milano, Italy, Sep 29-Oct 04, 2024. DOI GitHub
Abstract
Domain Generalization (DG) focuses on enhancing the generalization of deep learning models trained on multiple source domains to adapt to unseen target domains. This paper explores DG through the lens of bias-variance decomposition, uncovering that test errors in DG predominantly arise from cross-domain bias and variance. Inspired by this insight, we introduce a Representation Enhancement-Stabilization (RES) framework, comprising a Representation Enhancement (RE) module and a Representation Stabilization (RS) module. In RE, a novel set of feature frequency augmentation techniques is used to progressively reduce cross-domain bias during feature extraction. Furthermore, in RS, a novel Mutual Exponential Moving Average (MEMA) strategy is designed to stabilize model optimization for diminishing cross-domain variance during training. Collectively, the whole RES method can significantly enhance model generalization. We evaluate RES on five benchmark datasets and the results show that it outperforms multiple advanced DG methods.
MCML Authors
ZigMa: A DiT-style Zigzag Mamba Diffusion Model.
ECCV 2024 - 18th European Conference on Computer Vision. Milano, Italy, Sep 29-Oct 04, 2024. DOI
Abstract
The diffusion model has long been plagued by scalability and quadratic complexity issues, especially within transformer-based structures. In this study, we aim to leverage the long sequence modeling capability of a State-Space Model called Mamba to extend its applicability to visual data generation. Firstly, we identify a critical oversight in most current Mamba-based vision methods, namely the lack of consideration for spatial continuity in the scan scheme of Mamba. Secondly, building upon this insight, we introduce Zigzag Mamba, a simple, plug-and-play, minimal-parameter burden, DiT style solution, which outperforms Mamba-based baselines and demonstrates improved speed and memory utilization compared to transformer-based baselines, also this heterogeneous layerwise scan enables zero memory and speed burden when we consider more scan paths. Lastly, we integrate Zigzag Mamba with the Stochastic Interpolant framework to investigate the scalability of the model on large-resolution visual datasets, such as FacesHQ and UCF101, MultiModal-CelebA-HQ, and MS COCO .
MCML Authors
EgoCVR: An Egocentric Benchmark for Fine-Grained Composed Video Retrieval.
ECCV 2024 - 18th European Conference on Computer Vision. Milano, Italy, Sep 29-Oct 04, 2024. DOI GitHub
Abstract
In Composed Video Retrieval, a video and a textual description which modifies the video content are provided as inputs to the model. The aim is to retrieve the relevant video with the modified content from a database of videos. In this challenging task, the first step is to acquire large-scale training datasets and collect high-quality benchmarks for evaluation. In this work, we introduce EgoCVR, a new evaluation benchmark for fine-grained Composed Video Retrieval using large-scale egocentric video datasets. EgoCVR consists of 2,295 queries that specifically focus on high-quality temporal video understanding. We find that existing Composed Video Retrieval frameworks do not achieve the necessary high-quality temporal video understanding for this task. To address this shortcoming, we adapt a simple training-free method, propose a generic re-ranking framework for Composed Video Retrieval, and demonstrate that this achieves strong results on EgoCVR.
MCML Authors
Interpretable and Reliable Machine Learning
Interpretable and Reliable Machine Learning
DataDream: Few-shot Guided Dataset Generation.
ECCV 2024 - 18th European Conference on Computer Vision. Milano, Italy, Sep 29-Oct 04, 2024. DOI GitHub
Abstract
While text-to-image diffusion models have been shown to achieve state-of-the-art results in image synthesis, they have yet to prove their effectiveness in downstream applications. Previous work has proposed to generate data for image classifier training given limited real data access. However, these methods struggle to generate in-distribution images or depict fine-grained features, thereby hindering the generalization of classification models trained on synthetic datasets. We propose DataDream, a framework for synthesizing classification datasets that more faithfully represents the real data distribution when guided by few-shot examples of the target classes. DataDream fine-tunes LoRA weights for the image generation model on the few real images before generating the training data using the adapted model. We then fine-tune LoRA weights for CLIP using the synthetic data to improve downstream image classification over previous approaches on a large variety of datasets. We demonstrate the efficacy of DataDream through extensive experiments, surpassing state-of-the-art classification accuracy with few-shot data across 7 out of 10 datasets, while being competitive on the other 3. Additionally, we provide insights into the impact of various factors, such as the number of real-shot and generated images as well as the fine-tuning compute on model performance.
MCML Authors
Interpretable and Reliable Machine Learning
WaSt-3D: Wasserstein-2 Distance for Scene-to-Scene Stylization on 3D Gaussians.
ECCV 2024 - 18th European Conference on Computer Vision. Milano, Italy, Sep 29-Oct 04, 2024. DOI GitHub
Abstract
While style transfer techniques have been well-developed for 2D image stylization, the extension of these methods to 3D scenes remains relatively unexplored. Existing approaches demonstrate proficiency in transferring colors and textures but often struggle with replicating the geometry of the scenes. In our work, we leverage an explicit Gaussian Scale (GS) representation and directly match the distributions of Gaussians between style and content scenes using the Earth Mover’s Distance (EMD). By employing the entropy-regularized Wasserstein-2 distance, we ensure that the transformation maintains spatial smoothness. Additionally, we decompose the scene stylization problem into smaller chunks to enhance efficiency. This paradigm shift reframes stylization from a pure generative process driven by latent space losses to an explicit matching of distributions between two Gaussian representations. Our method achieves high-resolution 3D stylization by faithfully transferring details from 3D style scenes onto the content scene. Furthermore, WaSt-3D consistently delivers results across diverse content and style scenes without necessitating any training, as it relies solely on optimization-based techniques.
MCML Authors
GlobalPointer: Large-Scale Plane Adjustment with Bi-Convex Relaxation.
ECCV 2024 - 18th European Conference on Computer Vision. Milano, Italy, Sep 29-Oct 04, 2024. DOI GitHub
Abstract
Plane adjustment (PA) is crucial for many 3D applications, involving simultaneous pose estimation and plane recovery. Despite recent advancements, it remains a challenging problem in the realm of multi-view point cloud registration. Current state-of-the-art methods can achieve globally optimal convergence only with good initialization. Furthermore, their high time complexity renders them impractical for large-scale problems. To address these challenges, we first exploit a novel optimization strategy termed Bi-Convex Relaxation, which decouples the original problem into two simpler sub-problems, reformulates each sub-problem using a convex relaxation technique, and alternately solves each one until the original problem converges. Building on this strategy, we propose two algorithmic variants for solving the plane adjustment problem, namely GlobalPointer and GlobalPointer++, based on point-to-plane and plane-to-plane errors, respectively. Extensive experiments on both synthetic and real datasets demonstrate that our method can perform large-scale plane adjustment with linear time complexity, larger convergence region, and robustness to poor initialization, while achieving similar accuracy as prior methods.
MCML Authors
MeshFeat: Multi-Resolution Features for Neural Fields on Meshes.
ECCV 2024 - 18th European Conference on Computer Vision. Milano, Italy, Sep 29-Oct 04, 2024. DOI
Abstract
Parametric feature grid encodings have gained significant attention as an encoding approach for neural fields since they allow for much smaller MLPs, which significantly decreases the inference time of the models. In this work, we propose MeshFeat, a parametric feature encoding tailored to meshes, for which we adapt the idea of multi-resolution feature grids from Euclidean space. We start from the structure provided by the given vertex topology and use a mesh simplification algorithm to construct a multi-resolution feature representation directly on the mesh. The approach allows the usage of small MLPs for neural fields on meshes, and we show a significant speed-up compared to previous representations while maintaining comparable reconstruction quality for texture reconstruction and BRDF representation. Given its intrinsic coupling to the vertices, the method is particularly well-suited for representations on deforming meshes, making it a good fit for object animation.
MCML Authors
Computer Vision & Artificial Intelligence
TTT-MIM: Test-Time Training with Masked Image Modeling for Denoising Distribution Shifts.
ECCV 2024 - 18th European Conference on Computer Vision. Milano, Italy, Sep 29-Oct 04, 2024. DOI GitHub
Abstract
Neural networks trained end-to-end give state-of-the-art performance for image denoising. However, when applied to an image outside of the training distribution, the performance often degrades significantly. In this work, we propose a test-time training (TTT) method based on masked image modeling (MIM) to improve denoising performance for out-of-distribution images. The method, termed TTT-MIM, consists of a training stage and a test time adaptation stage. At training, we minimize a standard supervised loss and a self-supervised loss aimed at reconstructing masked image patches. At test-time, we minimize a self-supervised loss to fine-tune the network to adapt to a single noisy image. Experiments show that our method can improve performance under natural distribution shifts, in particular it adapts well to real-world camera and microscope noise. A competitor to our method of training and finetuning is to use a zero-shot denoiser that does not rely on training data. However, compared to state-of-the-art zero-shot denoisers, our method shows superior performance, and is much faster, suggesting that training and finetuning on the test instance is a more efficient approach to image denoising than zero-shot methods in setups where little to no data is available.
MCML Authors
Machine Learning and Information Processing
ChEX: Interactive Localization and Region Description in Chest X-rays.
ECCV 2024 - 18th European Conference on Computer Vision. Milano, Italy, Sep 29-Oct 04, 2024. DOI GitHub
Abstract
Report generation models offer fine-grained textual interpretations of medical images like chest X-rays, yet they often lack interactivity (i.e. the ability to steer the generation process through user queries) and localized interpretability (i.e. visually grounding their predictions), which we deem essential for future adoption in clinical practice. While there have been efforts to tackle these issues, they are either limited in their interactivity by not supporting textual queries or fail to also offer localized interpretability. Therefore, we propose a novel multitask architecture and training paradigm integrating textual prompts and bounding boxes for diverse aspects like anatomical regions and pathologies. We call this approach the Chest X-Ray Explainer (ChEX). Evaluations across a heterogeneous set of 9 chest X-ray tasks, including localized image interpretation and report generation, showcase its competitiveness with SOTA models while additional analysis demonstrates ChEX’s interactive capabilities.
MCML Authors
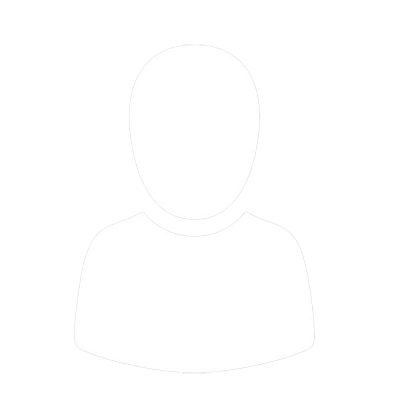
Georgios Kaissis
Dr.
* Former Member
Learning Trimodal Relation for Audio-Visual Question Answering with Missing Modality.
ECCV 2024 - 18th European Conference on Computer Vision. Milano, Italy, Sep 29-Oct 04, 2024. DOI GitHub
Abstract
Recent Audio-Visual Question Answering (AVQA) methods rely on complete visual and audio input to answer questions accurately. However, in real-world scenarios, issues such as device malfunctions and data transmission errors frequently result in missing audio or visual modality. In such cases, existing AVQA methods suffer significant performance degradation. In this paper, we propose a framework that ensures robust AVQA performance even when a modality is missing. First, we propose a Relation-aware Missing Modal (RMM) generator with Relation-aware Missing Modal Recalling (RMMR) loss to enhance the ability of the generator to recall missing modal information by understanding the relationships and context among the available modalities. Second, we design an Audio-Visual Relation-aware (AVR) diffusion model with Audio-Visual Enhancing (AVE) loss to further enhance audio-visual features by leveraging the relationships and shared cues between the audio-visual modalities. As a result, our method can provide accurate answers by effectively utilizing available information even when input modalities are missing. We believe our method holds potential applications not only in AVQA research but also in various multi-modal scenarios.
MCML Authors
Computer Aided Medical Procedures & Augmented Reality
CTRLorALTer: Conditional LoRAdapter for Efficient 0-Shot Control and Altering of T2I Models.
ECCV 2024 - 18th European Conference on Computer Vision. Milano, Italy, Sep 29-Oct 04, 2024. DOI GitHub
Abstract
Text-to-image generative models have become a prominent and powerful tool that excels at generating high-resolution realistic images. However, guiding the generative process of these models to take into account detailed forms of conditioning reflecting style and/or structure information remains an open problem. In this paper, we present. LoRAdapter, an approach that unifies both style and structure conditioning under the same formulation using a novel conditional LoRA block that enables zero-shot control. LoRAdapter is an efficient and powerful approach to condition text-to-image diffusion models, which enables fine-grained control conditioning during generation and outperforms recent state-of-the-art approaches.
MCML Authors
Alignist: CAD-Informed Orientation Distribution Estimation by Fusing Shape and Correspondences.
ECCV 2024 - 18th European Conference on Computer Vision. Milano, Italy, Sep 29-Oct 04, 2024. DOI
Abstract
Object pose distribution estimation is crucial in robotics for better path planning and handling of symmetric objects. Recent distribution estimation approaches employ contrastive learning-based approaches by maximizing the likelihood of a single pose estimate in the absence of a CAD model. We propose a pose distribution estimation method leveraging symmetry respecting correspondence distributions and shape information obtained using a CAD model. Contrastive learning-based approaches require an exhaustive amount of training images from different viewpoints to learn the distribution properly, which is not possible in realistic scenarios. Instead, we propose a pipeline that can leverage correspondence distributions and shape information from the CAD model, which are later used to learn pose distributions. Besides, having access to pose distribution based on correspondences before learning pose distributions conditioned on images, can help formulate the loss between distributions. The prior knowledge of distribution also helps the network to focus on getting sharper modes instead. With the CAD prior, our approach converges much faster and learns distribution better by focusing on learning sharper distribution near all the valid modes, unlike contrastive approaches, which focus on a single mode at a time. We achieve benchmark results on SYMSOL-I and T-Less datasets.
MCML Authors
Computer Aided Medical Procedures & Augmented Reality
Computer Aided Medical Procedures & Augmented Reality
Decoupling Common and Unique Representations for Multimodal Self-supervised Learning.
ECCV 2024 - 18th European Conference on Computer Vision. Milano, Italy, Sep 29-Oct 04, 2024. DOI GitHub
Abstract
The increasing availability of multi-sensor data sparks wide interest in multimodal self-supervised learning. However, most existing approaches learn only common representations across modalities while ignoring intra-modal training and modality-unique representations. We propose Decoupling Common and Unique Representations (DeCUR), a simple yet effective method for multimodal self-supervised learning. By distinguishing inter- and intra-modal embeddings through multimodal redundancy reduction, DeCUR can integrate complementary information across different modalities. We evaluate DeCUR in three common multimodal scenarios (radar-optical, RGB-elevation, and RGB-depth), and demonstrate its consistent improvement regardless of architectures and for both multimodal and modality-missing settings. With thorough experiments and comprehensive analysis, we hope this work can provide valuable insights and raise more interest in researching the hidden relationships of multimodal representations.
MCML Authors
Power Variable Projection for Initialization-Free Large-Scale Bundle Adjustment.
ECCV 2024 - 18th European Conference on Computer Vision. Milano, Italy, Sep 29-Oct 04, 2024. DOI
Abstract
Most Bundle Adjustment (BA) solvers like the Levenberg-Marquardt algorithm require a good initialization. Instead, initialization-free BA remains a largely uncharted territory. The under-explored Variable Projection algorithm (VarPro) exhibits a wide convergence basin even without initialization. Coupled with object space error formulation, recent works have shown its ability to solve small-scale initialization-free bundle adjustment problem. To make such initialization-free BA approaches scalable, we introduce Power Variable Projection (PoVar), extending a recent inverse expansion method based on power series. Importantly, we link the power series expansion to Riemannian manifold optimization. This projective framework is crucial to solve large-scale bundle adjustment problems without initialization. Using the real-world BAL dataset, we experimentally demonstrate that our solver achieves state-of-the-art results in terms of speed and accuracy. To our knowledge, this work is the first to address the scalability of BA without initialization opening new venues for initialization-free structure-from-motion.
MCML Authors
Boosting 3D Single Object Tracking with 2D Matching Distillation and 3D Pre-training.
ECCV 2024 - 18th European Conference on Computer Vision. Milano, Italy, Sep 29-Oct 04, 2024. DOI
Abstract
3D single object tracking (SOT) is an essential task in autonomous driving and robotics. However, learning robust 3D SOT trackers remains challenging due to the limited category-specific point cloud data and the inherent sparsity and incompleteness of LiDAR scans. To tackle these issues, we propose a unified 3D SOT framework that leverages 3D generative pre-training and learns robust 3D matching abilities from 2D pre-trained foundation trackers. Our framework features a consistent target-matching architecture with the widely used 2D trackers, facilitating the transfer of 2D matching knowledge. Specifically, we first propose a lightweight Target-Aware Projection (TAP) module, allowing the pre-trained 2D tracker to work well on the projected point clouds without further fine-tuning. We then propose a novel IoU-guided matching-distillation framework that utilizes the powerful 2D pre-trained trackers to guide 3D matching learning in the 3D tracker, i.e., the 3D template-to-search matching should be consistent with its corresponding 2D template-to-search matching obtained from 2D pre-trained trackers. Our designs are applied to two mainstream 3D SOT frameworks: memory-less Siamese and contextual memory-based approaches, which are respectively named SiamDisst and MemDisst. Extensive experiments show that SiamDisst and MemDisst achieve state-of-the-art performance on KITTI, Waymo Open Dataset and nuScenes benchmarks, while running at above real-time speed of 25 and 90 FPS on a RTX3090 GPU.
MCML Authors
MICDrop: Masking Image and Depth Features via Complementary Dropout for Domain-Adaptive Semantic Segmentation.
ECCV 2024 - 18th European Conference on Computer Vision. Milano, Italy, Sep 29-Oct 04, 2024. DOI GitHub
Abstract
Unsupervised Domain Adaptation (UDA) is the task of bridging the domain gap between a labeled source domain, e.g., synthetic data, and an unlabeled target domain. We observe that current UDA methods show inferior results on fine structures and tend to oversegment objects with ambiguous appearance. To address these shortcomings, we propose to leverage geometric information, i.e., depth predictions, as depth discontinuities often coincide with segmentation boundaries. We show that naively incorporating depth into current UDA methods does not fully exploit the potential of this complementary information. To this end, we present MICDrop, which learns a joint feature representation by masking image encoder features while inversely masking depth encoder features. With this simple yet effective complementary masking strategy, we enforce the use of both modalities when learning the joint feature representation. To aid this process, we propose a feature fusion module to improve both global as well as local information sharing while being robust to errors in the depth predictions. We show that our method can be plugged into various recent UDA methods and consistently improve results across standard UDA benchmarks, obtaining new state-of-the-art performances.
MCML Authors
EchoScene: Indoor Scene Generation via Information Echo over Scene Graph Diffusion.
ECCV 2024 - 18th European Conference on Computer Vision. Milano, Italy, Sep 29-Oct 04, 2024. DOI
Abstract
We present EchoScene, an interactive and controllable generative model that generates 3D indoor scenes on scene graphs. EchoScene leverages a dual-branch diffusion model that dynamically adapts to scene graphs. Existing methods struggle to handle scene graphs due to varying numbers of nodes, multiple edge combinations, and manipulator-induced node-edge operations. EchoScene overcomes this by associating each node with a denoising process and enables collaborative information exchange, enhancing controllable and consistent generation aware of global constraints. This is achieved through an information echo scheme in both shape and layout branches. At every denoising step, all processes share their denoising data with an information exchange unit that combines these updates using graph convolution. The scheme ensures that the denoising processes are influenced by a holistic understanding of the scene graph, facilitating the generation of globally coherent scenes. The resulting scenes can be manipulated during inference by editing the input scene graph and sampling the noise in the diffusion model. Extensive experiments validate our approach, which maintains scene controllability and surpasses previous methods in generation fidelity. Moreover, the generated scenes are of high quality and thus directly compatible with off-the-shelf texture generation. Our code and models are open-sourced.
MCML Authors
Computer Aided Medical Procedures & Augmented Reality
Computer Aided Medical Procedures & Augmented Reality
Workshops (6 papers)
PRISM: Progressive Restoration for Scene Graph-Based Image Manipulation.
Workshop @ECCV 2024 - Computer Vision Workshop at the 18th European Conference on Computer Vision (ECCV 2024). Milano, Italy, Sep 29-Oct 04, 2024. DOI
Abstract
Scene graphs have emerged as accurate semantic descriptions for image generation and manipulation tasks; however, their complexity and diversity of the shapes and relations of objects in data make it challenging to incorporate them into the models and generate high-quality results. To address these challenges, we propose PRISM, a novel progressive multi-head image manipulation approach to improve the accuracy of the manipulation of target regions in the scene. Our image manipulation framework is trained using an end-to-end denoising masked reconstruction proxy task, where the masked regions are progressively unmasked from the outer regions to the inner part. We take advantage of the outer part of the masked area as they have a direct correlation with the context of the scene. Moreover, our multi-head architecture simultaneously generates detailed object-specific regions in addition to the entire image to produce higher-quality images. Our model is evaluated against methods in the semantic image manipulation task on the CLEVR and Visual Genome datasets. Our results demonstrate the potential of our approach for enhancing the quality and precision of scene graph-based image manipulation.
MCML Authors
Computer Aided Medical Procedures & Augmented Reality
Computer Aided Medical Procedures & Augmented Reality
Coloring the Past: Neural Historical Monuments Reconstruction from Archival Photography.
Wild3D @ECCV 2024 - Workshop 3D Modeling, Reconstruction, and Generation in the Wild at the 18th European Conference on Computer Vision (ECCV 2024). Milano, Italy, Sep 29-Oct 04, 2024. URL
Abstract
Historical monuments are a treasure and milestone of cultural heritage. Reconstructing the 3D models of these buildings holds significant value. The rapid development of neural rendering methods makes it possible to recover the original 3D shape exclusively based on archival photographs. However, this task presents considerable challenges due to the properties of available color images. Historical pictures are often limited in number and the scenes in these photos might have altered over time. The radiometric quality of these images is often sub-optimal for using automatic methods. To address these challenges, we introduce an approach to reconstruct the geometry of historical buildings from limited input images. We leverage dense point clouds as a geometric prior and introduce a color appearance embedding loss in volumetric rendering to recover the color of the building. We aim for our work to spark increased interest and focus on preserving historic buildings. Together with the proposed method, we introduce a new historical dataset of the Hungarian National Theater, providing a new benchmark for 3D reconstruction.
MCML Authors
Enhancing Surface Neural Implicits with Curvature-Guided Sampling and Uncertainty-Augmented Representations.
Wild3D @ECCV 2024 - Workshop 3D Modeling, Reconstruction, and Generation in the Wild at the 18th European Conference on Computer Vision (ECCV 2024). Milano, Italy, Sep 29-Oct 04, 2024. URL
Abstract
Neural implicits are a widely used surface presentation because they offer an adaptive resolution and support arbitrary topology changes. While previous works rely on ground truth point clouds or meshes, they often do not discuss the data acquisition and ignore the effect of input quality and sampling methods during reconstruction. In this paper, we introduce a sampling method with an uncertainty-augmented surface implicit representation that employs a sampling technique that considers the geometric characteristics of inputs. To this end, we introduce a strategy that efficiently computes differentiable geometric features, namely, mean curvatures, to guide the sampling phase during the training period. The uncertainty augmentation offers insights into the occupancy and reliability of the output signed distance value, thereby expanding representation capabilities into open surfaces. Finally, we demonstrate that our method improves the reconstruction of both synthetic and real-world data.
MCML Authors
Progressive Optimization of Camera Pose and 4D Radiance Fields for long Endoscopic Videos.
NeuralBCC @ECCV 2024 - 1st Workshop on Neural Fields Beyond Conventional Cameras at the 18th European Conference on Computer Vision (ECCV 2024). Milano, Italy, Sep 29-Oct 04, 2024. PDF
Abstract
Reconstructing endoscopic scenes is vital for medical purposes, such as post-operative assessments and educational training. Recently, neural rendering has emerged as a promising method for reconstructing endoscopic scenes involving tissue deformation. Yet, current techniques exhibit major limitations, such as reliance on static endoscopes, limited deformation, or the need for external tracking devices to obtain camera pose data. In this paper we introduce a novel solution that can tackle these challenges posed by
a moving stereo endoscope in a highly deformable setting. Our method divides the scene into multiple overlapping 4D neural radiance fields (NeRFs) and uses a progressive optimization approach via optical flow and geometry supervision for simultaneous reconstruction and camera pose estimation. Tested on videos of up to fifteen times longer than what prior work experiment on, our method greatly improves usability, extending detailed reconstruction to much longer surgical videos without external tracking. Comprehensive evaluations using the StereoMIS dataset show that our method substantially enhances novel view synthesis quality while maintaining competitive pose accuracy.
MCML Authors
Computer Aided Medical Procedures & Augmented Reality
Computer Aided Medical Procedures & Augmented Reality
SABER-6D: Shape Representation Based Implicit Object Pose Estimation.
Workshop @ECCV 2024 - Computer Vision Workshop at the 18th European Conference on Computer Vision (ECCV 2024). Milano, Italy, Sep 29-Oct 04, 2024. DOI
Abstract
In this paper, we propose a novel encoder-decoder architecture, named SABER, to learn the 6D pose of the object in the embedding space by learning shape representation at a given pose. This model enables us to learn pose by performing shape representation at a target pose from RGB image input. We perform shape representation as an auxiliary task which helps us in learning rotations space for an object based on 2D images. An image encoder predicts the rotation in the embedding space and the DeepSDF based decoder learns to represent the object’s shape at the given pose. As our approach is shape based, the pipeline is suitable for any type of object irrespective of the symmetry. Moreover, we need only a CAD model of the objects to train SABER. Our pipeline is synthetic data based and can also handle symmetric objects without symmetry labels and, thus, no additional labeled training data is needed. The experimental evaluation shows that our method achieves close to benchmark results for both symmetric objects and asymmetric objects on Occlusion-LineMOD, and T-LESS datasets.
MCML Authors
Computer Aided Medical Procedures & Augmented Reality
Beyond Ultra-NeRF: Explainable Neural Fields for Ultrasound.
NeuralBCC @ECCV 2024 - 1st Workshop on Neural Fields Beyond Conventional Cameras at the 18th European Conference on Computer Vision (ECCV 2024). Milano, Italy, Sep 29-Oct 04, 2024. URL
Abstract
Current ultrasound image synthesis techniques often fall short in semantic accuracy and physical realism or produce images with a significant domain gap. Ultra-NeRF addresses these issues by creating a Neural Field from reconstructed acoustic properties via pose-annotated B-mode images and shows that it can be used for novel view synthesis of B-mode images. While Ultra-NeRF generates plausible results, it lacks explainability in the acoustic parameter space. In this paper, we revisit neural fields for ultrasound and introduce the Sonographic Neural Reflection Field (SuRF), which adheres to the physical properties of acoustic ultrasound. By redesigning Ultra-NeRF’s differentiable forward synthesis model and incorporating physics-inspired regularizations, we ensure the interpretability of learned acoustic parameters. Tested on the Ultra-NeRF in-silico dataset and a new multi-view ex-vivo 3D ultrasound dataset, our method demonstrates enhanced reconstruction and interpretation across various tissue types, including fat, muscle, and bone.
MCML Authors
Computer Aided Medical Procedures & Augmented Reality
Computer Aided Medical Procedures & Augmented Reality
Computer Aided Medical Procedures & Augmented Reality
Computer Aided Medical Procedures & Augmented Reality
26.09.2024
Related
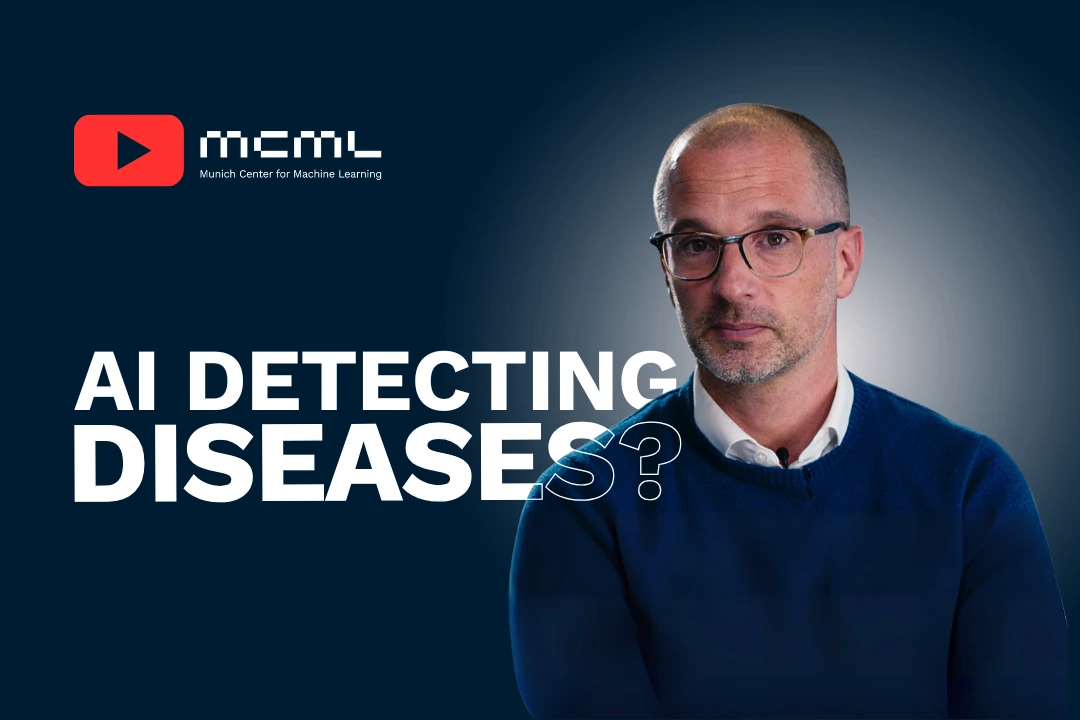
25.06.2025
When Clinical Expertise Meets AI Innovation – With Michael Ingrisch
The new research film features Michael Ingrisch, who shows how AI and clinical expertise can solve real challenges in radiology together.
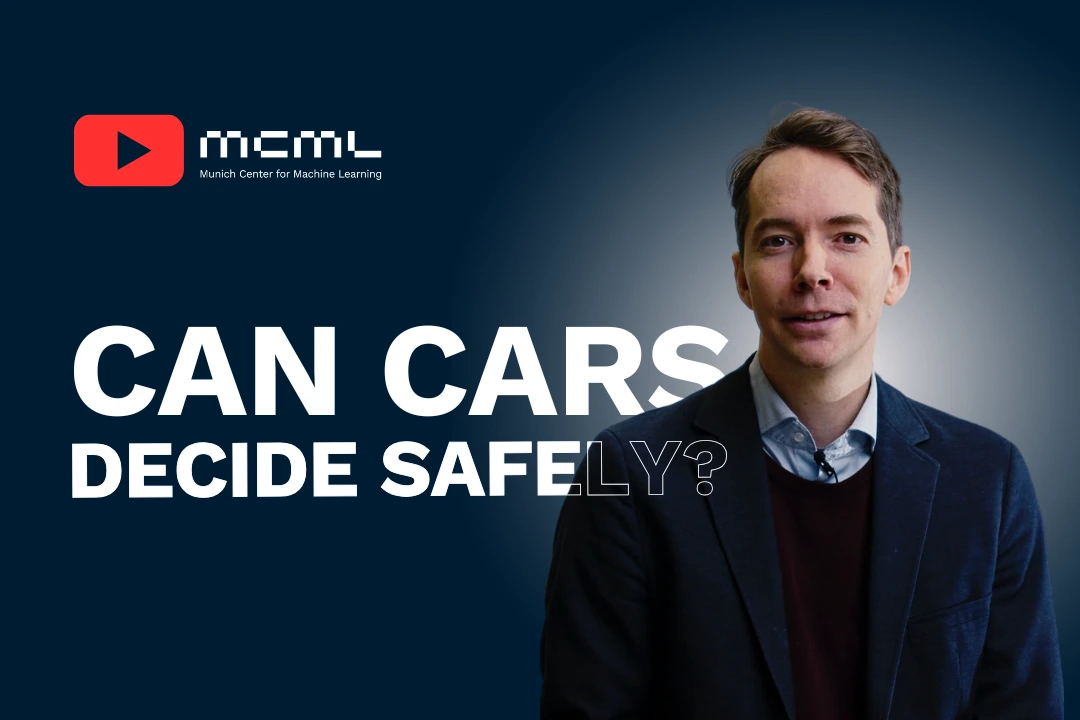
23.06.2025
Autonomous Driving: From Infinite Possibilities to Safe Decisions— With Matthias Althoff
The new research film features Matthias Althoff explaining how his team verifies autonomous vehicle safety using EDGAR and rigorous testing.
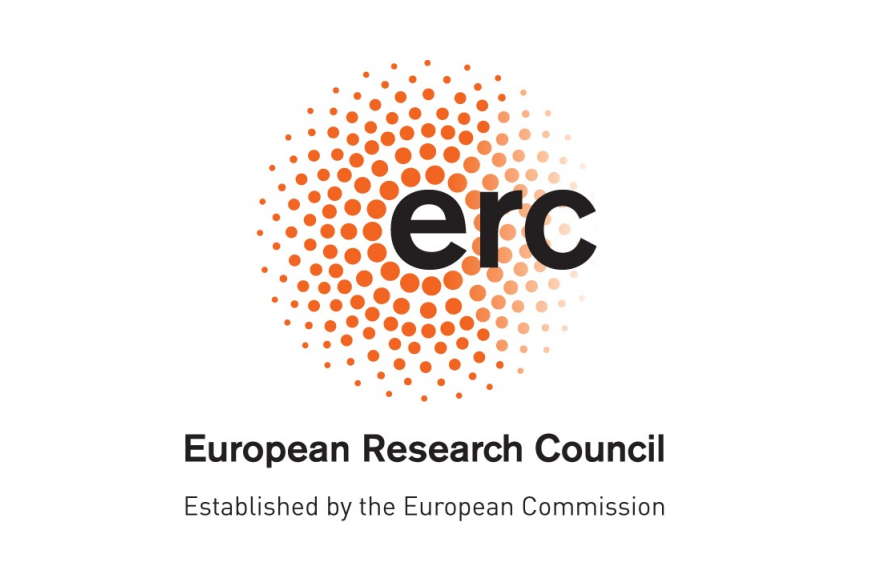
20.06.2025
ERC Advanced Grant for Massimo Fornasier
Massimo Fornasier was awarded ERC Advanced Grant to develop advanced algorithms for solving complex nonconvex optimization problems.
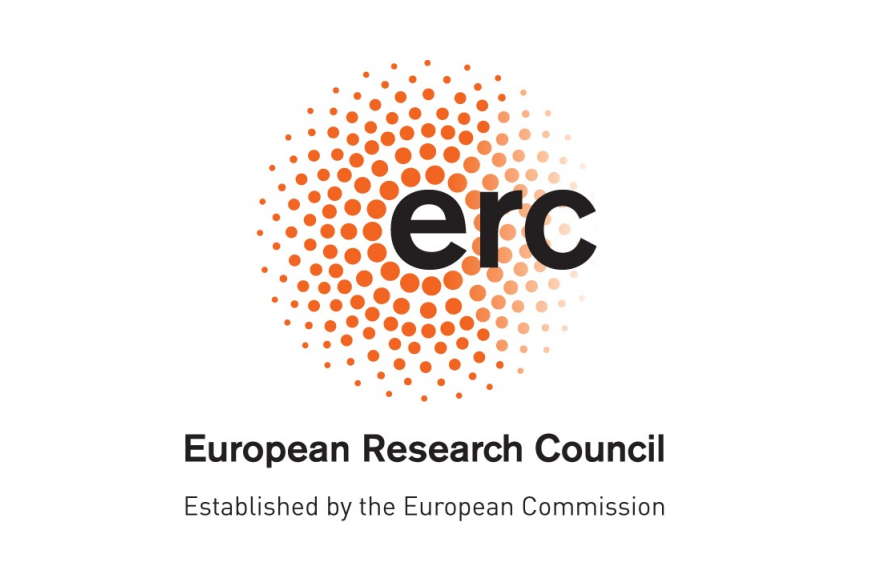
18.06.2025
ERC Advanced Grant for Albrecht Schmidt
Albrecht Schmidt receives ERC Advanced Grant for research on personalized generative AI to support memory, planning, and creativity.