01.01.2024
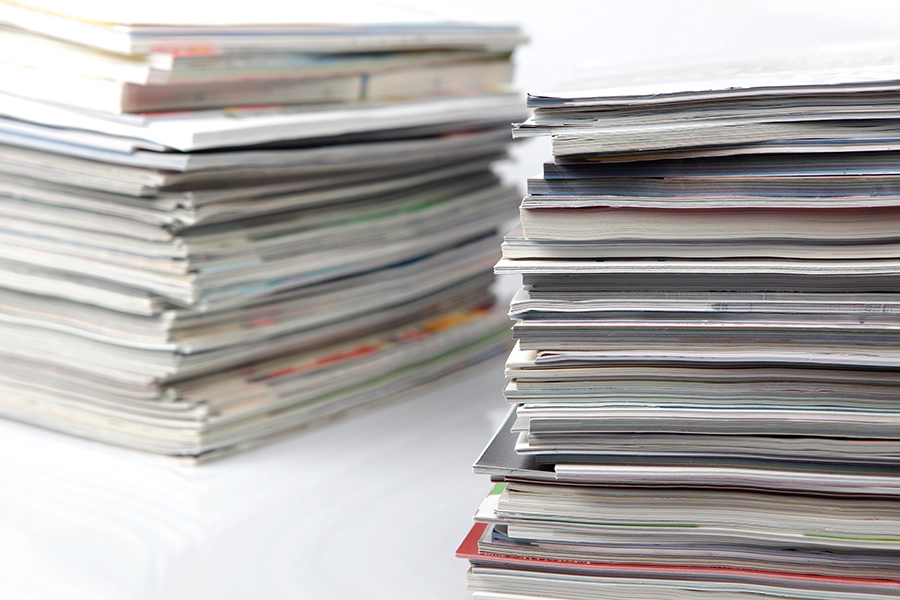
©Joachim Wendler - stock-adobe.com
88 Papers in Highly-Ranked Journals
We are happy to announce that MCML researchers are represented in 2024 with 88 papers in highly-ranked Journals. Congrats to our researchers!
Convergence of gradient descent for learning linear neural networks.
Advances in Continuous and Discrete Models 2024.23 (Jul. 2024). DOI
Abstract
We study the convergence properties of gradient descent for training deep linear neural networks, i.e., deep matrix factorizations, by extending a previous analysis for the related gradient flow. We show that under suitable conditions on the stepsizes gradient descent converges to a critical point of the loss function, i.e., the square loss in this article. Furthermore, we demonstrate that for almost all initializations gradient descent converges to a global minimum in the case of two layers. In the case of three or more layers, we show that gradient descent converges to a global minimum on the manifold matrices of some fixed rank, where the rank cannot be determined a priori.
MCML Authors
Mathematical Data Science and Artificial Intelligence
Mathematical Data Science and Artificial Intelligence
Mathematical Data Science and Artificial Intelligence
Artificial intelligence–based rapid brain volumetry substantially improves differential diagnosis in dementia.
Alzheimer’s and Dementia 16.e70037 (Oct. 2024). DOI
Abstract
This study evaluates the clinical value of a deep learning–based artificial intelligence (AI) system that performs rapid brain volumetry with automatic lobe segmentation and age- and sex-adjusted percentile comparisons.
Methods: Fifty-five patients—17 with Alzheimer’s disease (AD), 18 with frontotemporal dementia (FTD), and 20 healthy controls—underwent cranial magnetic resonance imaging scans. Two board-certified neuroradiologists (BCNR), two board-certified radiologists (BCR), and three radiology residents (RR) assessed the scans twice: first
without AI support and then with AI assistance.
Results: AI significantly improved diagnostic accuracy for AD (area under the curve −AI: 0.800, +AI: 0.926, p < 0.05), with increased correct diagnoses (p < 0.01) and reduced errors (p < 0.03). BCR and RR showed notable performance gains (BCR:
p < 0.04; RR: p < 0.02). For the diagnosis FTD, overall consensus (p < 0.01), BCNR (p < 0.02), and BCR (p < 0.05) recorded significantly more correct diagnoses.
Discussion: AI-assisted volumetry improves diagnostic performance in differentiating AD and FTD, benefiting all reader groups, including BCNR.
MCML Authors
Applied Machine Learning Using mlr3 in R.
American Statistician 79.2 (Jan. 2024). DOI
Abstract
mlr3 is an award-winning ecosystem of R packages that have been developed to enable state-of-the-art machine learning capabilities in R. Applied Machine Learning Using mlr3 in R gives an overview of flexible and robust machine learning methods, with an emphasis on how to implement them using mlr3 in R. It covers various key topics, including basic machine learning tasks, such as building and evaluating a predictive model; hyperparameter tuning of machine learning approaches to obtain peak performance; building machine learning pipelines that perform complex operations such as pre-processing followed by modelling followed by aggregation of predictions; and extending the mlr3 ecosystem with custom learners, measures, or pipeline components. The book is primarily aimed at researchers, practitioners, and graduate students who use machine learning or who are interested in using it. It can be used as a textbook for an introductory or advanced machine learning class that uses R, as a reference for people who work with machine learning methods, and in industry for exploratory experiments in machine learning.
MCML Authors
Statistical Learning and Data Science
Deep learning-based framework for city-scale rooftop solar potential estimation by considering roof superstructures.
Applied Energy 374.123839 (Nov. 2024). DOI
Abstract
Solar energy is an environmentally friendly energy source. Identifying suitable rooftops for solar panel installation contributes to not only sustainable energy plans but also carbon neutrality goals. Aerial imagery, bolstered by its growing availability, is a cost-effective data source for rooftop solar potential assessment at large scale. Existing studies generally do not take roof superstructures into account when determining how many solar panels can be installed. This procedure will lead to an overestimation of solar potential. Only several works have considered this issue, but none have devised a network that can simultaneously learn roof orientations and roof superstructures. Therefore, we devise SolarNet+, a novel framework to improve the precision of rooftop solar potential estimation. After implementing SolarNet+ on a benchmark dataset, we find that SolarNet+ outperforms other state-of-the-art approaches in both tasks — roof orientations and roof superstructure segmentation. Moreover, the SolarNet+ framework enables rooftop solar estimation at large-scale applications for investigating the correlation between urban rooftop solar potential and various local climate zone (LCZ) types. The results in the city of Brussels reveal that three specific LCZ urban types exhibit the highest rooftop solar potential efficiency: compact highrise (LCZ1), compact midrise (LCZ2), and heavy industry (LCZ10). The annual photovoltaic potential for these LCZ types is reported as 10.56 , 11.77 , and 10.70 , respectively.
MCML Authors
Deep learning for survival analysis: a review.
Artificial Intelligence Review 57.65 (Feb. 2024). DOI
Abstract
The influx of deep learning (DL) techniques into the field of survival analysis in recent years has led to substantial methodological progress; for instance, learning from unstructured or high-dimensional data such as images, text or omics data. In this work, we conduct a comprehensive systematic review of DL-based methods for time-to-event analysis, characterizing them according to both survival- and DL-related attributes. In summary, the reviewed methods often address only a small subset of tasks relevant to time-to-event data—e.g., single-risk right-censored data—and neglect to incorporate more complex settings.
MCML Authors
Statistical Learning and Data Science
Machine Learning Consulting Unit (MLCU)
GraphCompass: spatial metrics for differential analyses of cell organization across conditions.
Bioinformatics 40.Supplement 1 (Jul. 2024). DOI
Abstract
Spatial omics technologies are increasingly leveraged to characterize how disease disrupts tissue organization and cellular niches. While multiple methods to analyze spatial variation within a sample have been published, statistical and computational approaches to compare cell spatial organization across samples or conditions are mostly lacking. We present GraphCompass, a comprehensive set of omics-adapted graph analysis methods to quantitatively evaluate and compare the spatial arrangement of cells in samples representing diverse biological conditions. GraphCompass builds upon the Squidpy spatial omics toolbox and encompasses various statistical approaches to perform cross-condition analyses at the level of individual cell types, niches, and samples. Additionally, GraphCompass provides custom visualization functions that enable effective communication of results. We demonstrate how GraphCompass can be used to address key biological questions, such as how cellular organization and tissue architecture differ across various disease states and which spatial patterns correlate with a given pathological condition. GraphCompass can be applied to various popular omics techniques, including, but not limited to, spatial proteomics (e.g. MIBI-TOF), spot-based transcriptomics (e.g. 10× Genomics Visium), and single-cell resolved transcriptomics (e.g. Stereo-seq). In this work, we showcase the capabilities of GraphCompass through its application to three different studies that may also serve as benchmark datasets for further method development. With its easy-to-use implementation, extensive documentation, and comprehensive tutorials, GraphCompass is accessible to biologists with varying levels of computational expertise. By facilitating comparative analyses of cell spatial organization, GraphCompass promises to be a valuable asset in advancing our understanding of tissue function in health and disease.
MCML Authors
Explaining the optimistic performance evaluation of newly proposed methods: A cross-design validation experiment.
Biometrical Journal 66.1 (Jan. 2024). DOI
Abstract
The constant development of new data analysis methods in many fields of research is accompanied by an increasing awareness that these new methods often perform better in their introductory paper than in subsequent comparison studies conducted by other researchers. We attempt to explain this discrepancy by conducting a systematic experiment that we call “cross-design validation of methods”. In the experiment, we select two methods designed for the same data analysis task, reproduce the results shown in each paper, and then reevaluate each method based on the study design (i.e., datasets, competing methods, and evaluation criteria) that was used to show the abilities of the other method. We conduct the experiment for two data analysis tasks, namely cancer subtyping using multiomic data and differential gene expression analysis. Three of the four methods included in the experiment indeed perform worse when they are evaluated on the new study design, which is mainly caused by the different datasets. Apart from illustrating the many degrees of freedom existing in the assessment of a method and their effect on its performance, our experiment suggests that the performance discrepancies between original and subsequent papers may not only be caused by the nonneutrality of the authors proposing the new method but also by differences regarding the level of expertise and field of application. Authors of new methods should thus focus not only on a transparent and extensive evaluation but also on comprehensive method documentation that enables the correct use of their methods in subsequent studies.
MCML Authors
Biometry in Molecular Medicine
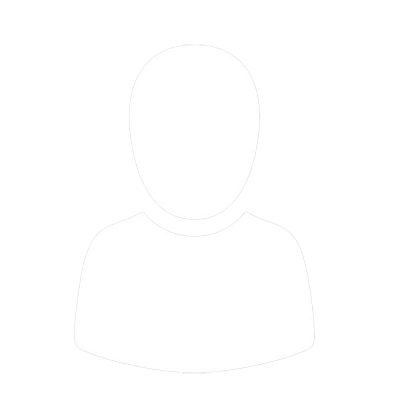
Theresa Ullmann
Dr.
* Former Member
Biometry in Molecular Medicine
Functional Data Analysis: An Introduction and Recent Developments.
Biometrical Journal 66.7 (Oct. 2024). DOI GitHub
Abstract
Functional data analysis (FDA) is a statistical framework that allows for the analysis of curves, images, or functions on higher dimensional domains. The goals of FDA, such as descriptive analyses, classification, and regression, are generally the same as for statistical analyses of scalar-valued or multivariate data, but FDA brings additional challenges due to the high- and infinite dimensionality of observations and parameters, respectively. This paper provides an introduction to FDA, including a description of the most common statistical analysis techniques, their respective software implementations, and some recent developments in the field. The paper covers fundamental concepts such as descriptives and outliers, smoothing, amplitude and phase variation, and functional principal component analysis. It also discusses functional regression, statistical inference with functional data, functional classification and clustering, and machine learning approaches for functional data analysis. The methods discussed in this paper are widely applicable in fields such as medicine, biophysics, neuroscience, and chemistry, and are increasingly relevant due to the widespread use of technologies that allow for the collection of functional data. Sparse functional data methods are also relevant for longitudinal data analysis. All presented methods are demonstrated using available software in R by analyzing a data set on human motion and motor control. To facilitate the understanding of the methods, their implementation, and hands-on application, the code for these practical examples is made available on Github.
MCML Authors
Statistics, Data Science and Machine Learning
Addressing researcher degrees of freedom through minP adjustment.
BMC Medical Research Methodology 24.152 (Jul. 2024). DOI
Abstract
When different researchers study the same research question using the same dataset they may obtain different and potentially even conflicting results. This is because there is often substantial flexibility in researchers’ analytical choices, an issue also referred to as ‘‘researcher degrees of freedom’’. Combined with selective reporting of the smallest p-value or largest effect, researcher degrees of freedom may lead to an increased rate of false positive and overoptimistic results. In this paper, we address this issue by formalizing the multiplicity of analysis strategies as a multiple testing problem. As the test statistics of different analysis strategies are usually highly dependent, a naive approach such as the Bonferroni correction is inappropriate because it leads to an unacceptable loss of power. Instead, we propose using the ‘‘minP’’ adjustment method, which takes potential test dependencies into account and approximates the underlying null distribution of the minimal p-value through a permutation-based procedure. This procedure is known to achieve more power than simpler approaches while ensuring a weak control of the family-wise error rate. We illustrate our approach for addressing researcher degrees of freedom by applying it to a study on the impact of perioperative paO2 on post-operative complications after neurosurgery. A total of 48 analysis strategies are considered and adjusted using the minP procedure. This approach allows to selectively report the result of the analysis strategy yielding the most convincing evidence, while controlling the type 1 error – and thus the risk of publishing false positive results that may not be replicable.
MCML Authors
Biometry in Molecular Medicine
Biometry in Molecular Medicine
Distributed non-disclosive validation of predictive models by a modified ROC-GLM.
BMC Medical Research Methodology 24.190 (Aug. 2024). DOI
Abstract
Distributed statistical analyses provide a promising approach for privacy protection when analyzing data distributed over several databases. Instead of directly operating on data, the analyst receives anonymous summary statistics, which are combined into an aggregated result. Further, in discrimination model (prognosis, diagnosis, etc.) development, it is key to evaluate a trained model w.r.t. to its prognostic or predictive performance on new independent data. For binary classification, quantifying discrimination uses the receiver operating characteristics (ROC) and its area under the curve (AUC) as aggregation measure. We are interested to calculate both as well as basic indicators of calibration-in-the-large for a binary classification task using a distributed and privacy-preserving approach…
MCML Authors
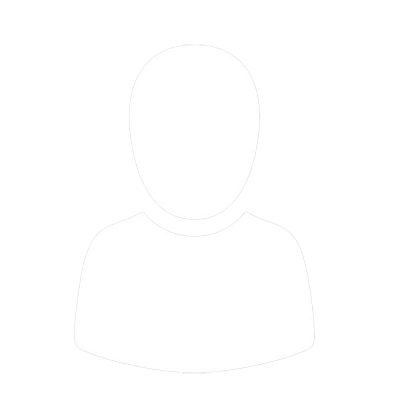
Daniel Schalk
Dr.
* Former Member
Statistical Learning and Data Science
Time-Varying Determinants of Graft Failure in Pediatric Kidney Transplantation in Europe.
Clinical Journal of the American Society of Nephrology 19.3 (Mar. 2024). DOI
Abstract
Little is known about the time-varying determinants of kidney graft failure in children. We performed a retrospective study of primary pediatric kidney transplant recipients (younger than 18 years) from the Eurotransplant registry (1990-2020). Piece-wise exponential additive mixed models were applied to analyze time-varying recipient, donor, and transplant risk factors. Primary outcome was death-censored graft failure.
MCML Authors
Machine Learning Consulting Unit (MLCU)
Influence of an allogenic collagen scaffold on implant sites with thin supracrestal tissue height: a randomized clinical trial.
Clinical Oral Investigations 28.313 (May. 2024). DOI
Abstract
Objectives: This randomized clinical trial focused on patients with thin peri-implant soft-tissue height (STH) (≤ 2.5 mm) and investigated the impact of an allogenic collagen scaffold (aCS) on supracrestal tissue height and marginal bone loss (MBL).
Material & methods: Forty patients received bone level implants and were randomly assigned to the test group with simultaneous tissue thickening with aCS or the control group. After three months, prosthetic restoration occurred. STH measurements were taken at baseline (T0) and reopening surgery (TR), with MBL assessed at 12 months (T1). Descriptive statistics were calculated for continuous variables, and counts for categorical variables (significance level, p = 0.05).
Results: At T1, 37 patients were available. At T0, control and test groups had mean STH values of 2.3 ± 0.3 mm and 2.1 ± 0.4 mm. TR revealed mean STH values of 2.3 ± 0.2 mm (control) and 2.6 ± 0.7 mm (test), with a significant tissue thickening of 0.5 ± 0.6 mm in the test group (p < 0.03). At T1, control and test groups showed MBL mean values of 1.1 ± 0.8 mm and 1.0 ± 0.6 mm, with a moderate but significant correlation with STH thickening (-0.34), implant position (0.43), history of periodontitis (0.39), and smoking status (0.27).
Conclusion: The use of an aCS protocol resulted in soft tissue thickening but did not reach a threshold to reliably reduce MBL compared to the control group within the study’s limitations.
Clinical relevance: Peri-implant STH is crucial for maintaining peri-implant marginal bone stability. Marginal bone stability represents a crucial factor in prevention of peri-implantitis development.
MCML Authors
Statistical Learning and Data Science
A systematic review of machine learning-based tumor-infiltrating lymphocytes analysis in colorectal cancer: Overview of techniques, performance metrics, and clinical outcomes.
Computers in Biology and Medicine 173 (May. 2024). DOI
Abstract
The incidence of colorectal cancer (CRC), one of the deadliest cancers around the world, is increasing. Tissue microenvironment (TME) features such as tumor-infiltrating lymphocytes (TILs) can have a crucial impact on diagnosis or decision-making for treating patients with CRC. While clinical studies showed that TILs improve the host immune response, leading to a better prognosis, inter-observer agreement for quantifying TILs is not perfect. Incorporating machine learning (ML) based applications in clinical routine may promote diagnosis reliability. Recently, ML has shown potential for making progress in routine clinical procedures. We aim to systematically review the TILs analysis based on ML in CRC histological images. Deep learning (DL) and non-DL techniques can aid pathologists in identifying TILs, and automated TILs are associated with patient outcomes. However, a large multi-institutional CRC dataset with a diverse and multi-ethnic population is necessary to generalize ML methods.
MCML Authors
Relevance of Protein Intake for Weaning in the Mechanically Ventilated Critically Ill: Analysis of a Large International Database.
Critical Care Medicine 50.3 (Mar. 2024). DOI
Abstract
The association between protein intake and the need for mechanical ventilation (MV) is controversial. We aimed to investigate the associations between protein intake and outcomes in ventilated critically ill patients.
MCML Authors
Statistical Consulting Unit (StaBLab)
Machine Learning Consulting Unit (MLCU)
Enhancing cluster analysis via topological manifold learning.
Data Mining and Knowledge Discovery 38 (Apr. 2024). DOI
Abstract
We discuss topological aspects of cluster analysis and show that inferring the topological structure of a dataset before clustering it can considerably enhance cluster detection: we show that clustering embedding vectors representing the inherent structure of a dataset instead of the observed feature vectors themselves is highly beneficial. To demonstrate, we combine manifold learning method UMAP for inferring the topological structure with density-based clustering method DBSCAN. Synthetic and real data results show that this both simplifies and improves clustering in a diverse set of low- and high-dimensional problems including clusters of varying density and/or entangled shapes. Our approach simplifies clustering because topological pre-processing consistently reduces parameter sensitivity of DBSCAN. Clustering the resulting embeddings with DBSCAN can then even outperform complex methods such as SPECTACL and ClusterGAN. Finally, our investigation suggests that the crucial issue in clustering does not appear to be the nominal dimension of the data or how many irrelevant features it contains, but rather how separable the clusters are in the ambient observation space they are embedded in, which is usually the (high-dimensional) Euclidean space defined by the features of the data. The approach is successful because it performs the cluster analysis after projecting the data into a more suitable space that is optimized for separability, in some sense.
MCML Authors
Biometry in Molecular Medicine
Marginal Effects for Non-Linear Prediction Functions.
Data Mining and Knowledge Discovery 38 (Feb. 2024). DOI
Abstract
Beta coefficients for linear regression models represent the ideal form of an interpretable feature effect. However, for non-linear models and especially generalized linear models, the estimated coefficients cannot be interpreted as a direct feature effect on the predicted outcome. Hence, marginal effects are typically used as approximations for feature effects, either in the shape of derivatives of the prediction function or forward differences in prediction due to a change in a feature value. While marginal effects are commonly used in many scientific fields, they have not yet been adopted as a model-agnostic interpretation method for machine learning models. This may stem from their inflexibility as a univariate feature effect and their inability to deal with the non-linearities found in black box models. We introduce a new class of marginal effects termed forward marginal effects. We argue to abandon derivatives in favor of better-interpretable forward differences. Furthermore, we generalize marginal effects based on forward differences to multivariate changes in feature values. To account for the non-linearity of prediction functions, we introduce a non-linearity measure for marginal effects. We argue against summarizing feature effects of a non-linear prediction function in a single metric such as the average marginal effect. Instead, we propose to partition the feature space to compute conditional average marginal effects on feature subspaces, which serve as conditional feature effect estimates.
MCML Authors
Statistical Learning and Data Science
Statistical Learning and Data Science
Smartwatches for non-invasive hypoglycaemia detection during cognitive and psychomotor stress.
Diabetes, Obesity and Metabolism 26.3 (Mar. 2024). DOI
Abstract
Hypoglycaemia is one of the most relevant complications of diabetes1 and induces alterations in physiological parameters2, 3 that can be measured with smartwatches and detected using machine learning (ML).4 The performance of these algorithms when applied to different hypoglycaemic ranges or in situations involving cognitive and psychomotor stress remains unclear. Demanding tasks can significantly affect the physiological responses on which the wearable-based hypoglycaemia detection relies.5 The present analysis aimed to investigate ML-based hypoglycaemia detection using wearable data at different levels of hypoglycaemia during a complex task involving cognitive and psychomotor challenges (driving).
MCML Authors
Artificial Intelligence in Management
A high-resolution calving front data product for marine-terminating glaciers in Svalbard.
Earth System Science Data 16.2 (Feb. 2024). DOI
Abstract
The mass loss of glaciers outside the polar ice sheets has been accelerating during the past several decades and has been contributing to global sea-level rise. However, many of the mechanisms of this mass loss process are not well understood, especially the calving dynamics of marine-terminating glaciers, in part due to a lack of high-resolution calving front observations. Svalbard is an ideal site to study the climate sensitivity of glaciers as it is a region that has been undergoing amplified climate variability in both space and time compared to the global mean. Here we present a new high-resolution calving front dataset of 149 marine-terminating glaciers in Svalbard, comprising 124 919 glacier calving front positions during the period 1985–2023 (https://doi.org/10.5281/zenodo.10407266, Li et al., 2023). This dataset was generated using a novel automated deep-learning framework and multiple optical and SAR satellite images from Landsat, Terra-ASTER, Sentinel-2, and Sentinel-1 satellite missions. The overall calving front mapping uncertainty across Svalbard is 31 m. The newly derived calving front dataset agrees well with recent decadal calving front observations between 2000 and 2020 (Kochtitzky and Copland, 2022) and an annual calving front dataset between 2008 and 2022 (Moholdt et al., 2022). The calving fronts between our product and the latter deviate by 32±65m on average. The R2 of the glacier calving front change rates between these two products is 0.98, indicating an excellent match. Using this new calving front dataset, we identified widespread calving front retreats during the past four decades, across most regions in Svalbard except for a handful of glaciers draining the ice caps Vestfonna and Austfonna on Nordaustlandet. In addition, we identified complex patterns of glacier surging events overlaid with seasonal calving cycles. These data and findings provide insights into understanding glacier calving mechanisms and drivers. This new dataset can help improve estimates of glacier frontal ablation as a component of the integrated mass balance of marine-terminating glaciers.
MCML Authors
Does combining numerous data types in multi-omics data improve or hinder performance in survival prediction? Insights from a large-scale benchmark study.
Earth System Science Data 24.244 (Sep. 2024). DOI
Abstract
Background: Predictive modeling based on multi-omics data, which incorporates several types of omics data for the same patients, has shown potential to outperform single-omics predictive modeling. Most research in this domain focuses on incorporating numerous data types, despite the complexity and cost of acquiring them. The prevailing assumption is that increasing the number of data types necessarily improves predictive performance. However, the integration of less informative or redundant data types could potentially hinder this performance. Therefore, identifying the most effective combinations of omics data types that enhance predictive performance is critical for cost-effective and accurate predictions.
Methods: In this study, we systematically evaluated the predictive performance of all 31 possible combinations including at least one of five genomic data types (mRNA, miRNA, methylation, DNAseq, and copy number variation) using 14 cancer datasets with right-censored survival outcomes, publicly available from the TCGA database. We employed various prediction methods and up-weighted clinical data in every model to leverage their predictive importance. Harrell’s C-index and the integrated Brier Score were used as performance measures. To assess the robustness of our findings, we performed a bootstrap analysis at the level of the included datasets. Statistical testing was conducted for key results, limiting the number of tests to ensure a low risk of false positives.
Results: Contrary to expectations, we found that using only mRNA data or a combination of mRNA and miRNA data was sufficient for most cancer types. For some cancer types, the additional inclusion of methylation data led to improved prediction results. Far from enhancing performance, the introduction of more data types most often resulted in a decline in performance, which varied between the two performance measures.
Conclusions: Our findings challenge the prevailing notion that combining multiple omics data types in multi-omics survival prediction improves predictive performance. Thus, the widespread approach in multi-omics prediction of incorporating as many data types as possible should be reconsidered to avoid suboptimal prediction results and unnecessary expenditure.
MCML Authors
Biometry in Molecular Medicine
ChatGPT makes medicine easy to swallow: an exploratory case study on simplified radiology reports.
European Radiology 34 (May. 2024). DOI
Abstract
Objectives: To assess the quality of simplified radiology reports generated with the large language model (LLM) ChatGPT and to discuss challenges and chances of ChatGPT-like LLMs for medical text simplification.
Methods: In this exploratory case study, a radiologist created three fictitious radiology reports which we simplified by prompting ChatGPT with ‘Explain this medical report to a child using simple language.’’ In a questionnaire, we tasked 15 radiologists to rate the quality of the simplified radiology reports with respect to their factual correctness, completeness, and potential harm for patients. We used Likert scale analysis and inductive free-text categorization to assess the quality of the simplified reports.
Results: Most radiologists agreed that the simplified reports were factually correct, complete, and not potentially harmful to the patient. Nevertheless, instances of incorrect statements, missed relevant medical information, and potentially harmful passages were reported.
Conclusion: While we see a need for further adaption to the medical field, the initial insights of this study indicate a tremendous potential in using LLMs like ChatGPT to improve patient-centered care in radiology and other medical domains.
Clinical relevance statement: Patients have started to use ChatGPT to simplify and explain their medical reports, which is expected to affect patient-doctor interaction. This phenomenon raises several opportunities and challenges for clinical routine.
MCML Authors
Neuromechanical stabilisation of the centre of mass during running.
Gait and Posture 108 (Feb. 2024). DOI
Abstract
Background: Stabilisation of the centre of mass (COM) trajectory is thought to be important during running. There is emerging evidence of the importance of leg length and angle regulation during running, which could contribute to stability in the COM trajectory The present study aimed to understand if leg length and angle stabilises the vertical and anterior-posterior (AP) COM displacements, and if the stability alters with running speeds.
Methods: Data for this study came from an open-source treadmill running dataset (n = 28). Leg length (m) was calculated by taking the resultant distance of the two-dimensional sagittal plane leg vector (from pelvis segment to centre of pressure). Leg angle was defined by the angle subtended between the leg vector and the horizontal surface. Leg length and angle were scaled to a standard deviation of one. Uncontrolled manifold analysis (UCM) was used to provide an index of motor abundance (IMA) in the stabilisation of the vertical and AP COM displacement.
Results: IMAAP and IMAvertical were largely destabilising and always stabilising, respectively. As speed increased, the peak destabilising effect on IMAAP increased from −0.66(0.18) at 2.5 m/s to −1.12(0.18) at 4.5 m/s, and the peak stabilising effect on IMAvertical increased from 0.69 (0.19) at 2.5 m/s to 1.18 (0.18) at 4.5 m/s.
Conclusion: Two simple parameters from a simple spring-mass model, leg length and angle, can explain the control behind running. The variability in leg length and angle helped stabilise the vertical COM, whilst maintaining constant running speed may rely more on inter-limb variation to adjust the horizontal COM accelerations.
MCML Authors
Statistics, Data Science and Machine Learning
Computing-Model and Computing-Hardware Selection for ICT Under Societal and Judicial Constraints.
IEEE Access 12 (Dec. 2024). DOI
Abstract
This article discusses a formalization of aspects of Cyber-Sovereignty (CyS) for information and communication technology (ICT), linking them to technological trustworthiness and deriving an associated paradigm for hard- and software design. The upcoming 6G ICT standard is considered a keystone within modern society’s increasing interconnectedness and automatization, as it provides the necessary technological infrastructure for applications such as the Metaverse or large-scale digital twinning. Since emerging technological systems increasingly affect sensitive human goods, hard- and software manufacturers must consider a new dimension of societal and judicial constraints in the context of technological trustworthiness. This article aims to establish a formalized theory of specific aspects of CyS, providing a paradigm for hard- and software engineering in ICT. This paradigm is directly applicable in formal technology assessment and ensures that the relevant facets of CyS – specifically, the principle of Algorithmic Transparency (AgT) – are satisfied. The framework follows an axiomatic approach. Particularly, the formal basis of our theory consists of four fundamental assumptions about the general nature of physical problems and algorithmic implementations. This formal basis allows for drawing general conclusions on the relation between CyS and technological trustworthiness and entails a formal meta-thesis on AgT in digital computing.
MCML Authors
Mathematical Foundations of Artificial Intelligence
Coupling Sentiment and Arousal Analysis Towards an Affective Dialogue Manager.
IEEE Access 12 (Feb. 2024). DOI
Abstract
We present the technologies and host components developed to power a speech-based dialogue manager with affective capabilities. The overall goal is that the system adapts its response to the sentiment and arousal level of the user inferred by analysing the linguistic and paralinguistic information embedded in his or her interaction. A linguistic-based, dedicated sentiment analysis component determines the body of the system response. A paralinguistic-based, dedicated arousal recognition component adjusts the energy level to convey in the affective system response. The sentiment analysis model is trained using the CMU-MOSEI dataset and implements a hierarchical contextual attention fusion network, which scores an Unweighted Average Recall (UAR) of 79.04% on the test set when tackling the task as a binary classification problem. The arousal recognition model is trained using the MSP-Podcast corpus. This model extracts the Mel-spectrogram representations of the speech signals, which are exploited with a Convolutional Neural Network (CNN) trained from scratch, and scores a UAR of 61.11% on the test set when tackling the task as a three-class classification problem. Furthermore, we highlight two sample dialogues implemented at the system back-end to detail how the sentiment and arousal inferences are coupled to determine the affective system response. These are also showcased in a proof of concept demonstrator. We publicly release the trained models to provide the research community with off-the-shelf sentiment analysis and arousal recognition tools.
MCML Authors
Automatic Bird Sound Source Separation Based on Passive Acoustic Devices in Wild Environment.
IEEE Internet of Things Journal 11.9 (Jan. 2024). DOI
Abstract
The Internet of Things (IoT)-based passive acoustic monitoring (PAM) has shown great potential in large-scale remote bird monitoring. However, field recordings often contain overlapping signals, making precise bird information extraction challenging. To solve this challenge, first, the interchannel spatial feature is chosen as complementary information to the spectral feature to obtain additional spatial correlations between the sources. Then, an end-to-end model named BACPPNet is built based on Deeplabv3plus and enhanced with the polarized self-attention mechanism to estimate the spectral magnitude mask (SMM) for separating bird vocalizations. Finally, the separated bird vocalizations are recovered from SMMs and the spectrogram of mixed audio using the inverse short Fourier transform (ISTFT). We evaluate our proposed method utilizing the generated mixed data set. Experiments have shown that our method can separate bird vocalizations from mixed audio with root mean square error (RMSE), source-to-distortion ratio (SDR), source-to-interference ratio (SIR), source-to-artifact ratio (SAR), and short-time objective intelligibility (STOI) values of 2.82, 10.00 dB, 29.90 dB, 11.08 dB, and 0.66, respectively, which are better than existing methods. Furthermore, the average classification accuracy of the separated bird vocalizations drops the least. This indicates that our method outperforms other compared separation methods in bird sound separation and preserves the fidelity of the separated sound sources, which might help us better understand wild bird sound recordings.
MCML Authors
Fed-MStacking: Heterogeneous Federated Learning With Stacking Misaligned Labels for Abnormal Heart Sound Detection.
IEEE Journal of Biomedical and Health Informatics 28.9 (Jul. 2024). DOI
Abstract
Ubiquitous sensing has been widely applied in smart healthcare, providing an opportunity for intelligent heart sound auscultation. However, smart devices contain sensitive information, raising user privacy concerns. To this end, federated learning (FL) has been adopted as an effective solution, enabling decentralised learning without data sharing, thus preserving data privacy in the Internet of Health Things (IoHT). Nevertheless, traditional FL requires the same architectural models to be trained across local clients and global servers, leading to a lack of model heterogeneity and client personalisation. For medical institutions with private data clients, this study proposes Fed-MStacking, a heterogeneous FL framework that incorporates a stacking ensemble learning strategy to support clients in building their own models. The secondary objective of this study is to address scenarios involving local clients with data characterised by inconsistent labelling. Specifically, the local client contains only one case type, and the data cannot be shared within or outside the institution. To train a global multi-class classifier, we aggregate missing class information from all clients at each institution and build meta-data, which then participates in FL training via a meta-learner. We apply the proposed framework to a multi-institutional heart sound database. The experiments utilise random forests (RFs), feedforward neural networks (FNNs), and convolutional neural networks (CNNs) as base classifiers. The results show that the heterogeneous stacking of local models performs better compared to homogeneous stacking.
MCML Authors
Land Cover Classification From Sentinel-2 Images With Quantum-Classical Convolutional Neural Networks.
IEEE Journal of Selected Topics in Applied Earth Observations and Remote Sensing 17 (Jul. 2024). DOI
Abstract
Exploiting machine learning techniques to automatically classify multispectral remote sensing imagery plays a significant role in deriving changes on the Earth’s surface. However, the computation power required to manage large Earth observation data and apply sophisticated machine learning models for this analysis purpose has become an intractable bottleneck. Leveraging quantum computing provides a possibility to tackle this challenge in the future. This article focuses on land cover classification by analyzing Sentinel-2 images with quantum computing. Two hybrid quantum-classical deep learning frameworks are proposed. Both models exploit quantum computing to extract features efficiently from multispectral images and classical computing for final classification. As proof of concept, numerical simulation results on the LCZ42 dataset through the TensorFlow Quantum platform verify our models’ validity. The experiments indicate that our models can extract features more effectively compared with their classical counterparts, specifically, the convolutional neural network (CNN) model. Our models demonstrated improvements, with an average test accuracy increase of 4.5% and 3.3%, respectively, in comparison to the CNN model. In addition, our proposed models exhibit better transferability and robustness than CNN models.
MCML Authors
Uncertainty Estimation of Lake Ice Cover Maps From a Random Forest Classifier Using MODIS TOA Reflectance Data.
IEEE Journal of Selected Topics in Applied Earth Observations and Remote Sensing 18 (Dec. 2024). DOI
Abstract
This article presents a method to improve the usability of lake ice cover (LIC) maps generated from moderate resolution imaging spectroradiometer (MODIS) top-of-atmosphere reflectance data by providing estimates of aleatoric and epistemic uncertainty. We used a random forest (RF) classifier, which has been shown to have superior performance in classifying lake ice, open water, and clouds, to generate daily LIC maps with inherent (aleatoric) and model (epistemic) uncertainties. RF allows for the learning of different hypotheses (trees), producing diverse predictions that can be utilized to quantify aleatoric and epistemic uncertainty. We use a decomposition of Shannon entropy to quantify these uncertainties and apply pixel-based uncertainty estimation. Our results show that using uncertainty values to reject the classification of uncertain pixels significantly improves recall and precision. The method presented herein is under consideration for integration into the processing chain implemented for the production of daily LIC maps as part of the European Space Agency’s Climate Change Initiative (CCI+) Lakes project.
MCML Authors
Artificial Intelligence and Machine Learning
Feature Guided Masked Autoencoder for Self-Supervised Learning in Remote Sensing.
IEEE Journal of Selected Topics in Applied Earth Observations and Remote Sensing 18 (Nov. 2024). DOI
Abstract
Self-supervised learning guided by masked image modeling, such as masked autoencoder (MAE), has attracted wide attention for pretraining vision transformers in remote sensing. However, MAE tends to excessively focus on pixel details, limiting the model’s capacity for semantic understanding, particularly for noisy synthetic aperture radar (SAR) images. In this article, we explore spectral and spatial remote sensing image features as improved MAE-reconstruction targets. We first conduct a study on reconstructing various image features, all performing comparably well or better than raw pixels. Based on such observations, we propose feature guided MAE (FG-MAE): reconstructing a combination of histograms of oriented gradients (HOG) and normalized difference indices (NDI) for multispectral images, and reconstructing HOG for SAR images. Experimental results on three downstream tasks illustrate the effectiveness of FG-MAE with a particular boost for SAR imagery (e.g., up to 5% better than MAE on EuroSAT-SAR). Furthermore, we demonstrate the well-inherited scalability of FG-MAE and release a first series of pretrained vision transformers for medium-resolution SAR and multispectral images.
MCML Authors
NeRF-VO: Real-Time Sparse Visual Odometry With Neural Radiance Fields.
IEEE Robotics and Automation Letters 9.8 (Aug. 2024). DOI
Abstract
We introduce a novel monocular visual odometry (VO) system, NeRF-VO, that integrates learning-based sparse visual odometry for low-latency camera tracking and a neural radiance scene representation for fine-detailed dense reconstruction and novel view synthesis. Our system initializes camera poses using sparse visual odometry and obtains view-dependent dense geometry priors from a monocular prediction network. We harmonize the scale of poses and dense geometry, treating them as supervisory cues to train a neural implicit scene representation. NeRF-VO demonstrates exceptional performance in both photometric and geometric fidelity of the scene representation by jointly optimizing a sliding window of keyframed poses and the underlying dense geometry, which is accomplished through training the radiance field with volume rendering. We surpass SOTA methods in pose estimation accuracy, novel view synthesis fidelity, and dense reconstruction quality across a variety of synthetic and real-world datasets while achieving a higher camera tracking frequency and consuming less GPU memory.
MCML Authors
Audio-based Kinship Verification Using Age Domain Conversion.
IEEE Signal Processing Letters 32 (Dec. 2024). DOI
Abstract
Audio-based kinship verification (AKV) is important in many domains, such as home security monitoring, forensic identification, and social network analysis. A key challenge in the task arises from differences in age across samples from different individuals, which can be interpreted as a domain bias in a cross-domain verification task. To address this issue, we design the notion of an ‘age-standardised domain’ wherein we utilise the optimised CycleGAN-VC3 network to perform age-audio conversion to generate the in-domain audio. The generated audio dataset is employed to extract a range of features, which are then fed into a metric learning architecture to verify kinship. Experiments are conducted on the KAN_AV audio dataset, which contains age and kinship labels. The results demonstrate that the method markedly enhances the accuracy of kinship verification, while also offering novel insights for future kinship verification research.
MCML Authors
A Wide Evaluation of ChatGPT on Affective Computing Tasks.
IEEE Transactions on Affective Computing 15.4 (Oct. 2024). DOI
Abstract
With the rise of foundation models, a new artificial intelligence paradigm has emerged, by simply using general purpose foundation models with prompting to solve problems instead of training a separate machine learning model for each problem. Such models have been shown to have emergent properties of solving problems that they were not initially trained on. The studies for the effectiveness of such models are still quite limited. In this work, we widely study the capabilities of the ChatGPT models, namely GPT-4 and GPT-3.5, on 13 affective computing problems, namely aspect extraction, aspect polarity classification, opinion extraction, sentiment analysis, sentiment intensity ranking, emotions intensity ranking, suicide tendency detection, toxicity detection, well-being assessment, engagement measurement, personality assessment, sarcasm detection, and subjectivity detection. We introduce a framework to evaluate the ChatGPT models on regression-based problems, such as intensity ranking problems, by modelling them as pairwise ranking classification. We compare ChatGPT against more traditional NLP methods, such as end-to-end recurrent neural networks and transformers. The results demonstrate the emergent abilities of the ChatGPT models on a wide range of affective computing problems, where GPT-3.5 and especially GPT-4 have shown strong performance on many problems, particularly the ones related to sentiment, emotions, or toxicity. The ChatGPT models fell short for problems with implicit signals, such as engagement measurement and subjectivity detection.
MCML Authors
Towards Multimodal Prediction of Spontaneous Humor: A Novel Dataset and First Results.
IEEE Transactions on Affective Computing Early Access (Oct. 2024). DOI
Abstract
Humor is a substantial element of human social behavior, affect, and cognition. Its automatic understanding can facilitate a more naturalistic human-AI interaction. Current methods of humor detection have been exclusively based on staged data, making them inadequate for ‘real-world’ applications. We contribute to addressing this deficiency by introducing the novel Passau-Spontaneous Football Coach Humor (Passau-SFCH) dataset, comprising about 11 hours of recordings. The Passau-SFCH dataset is annotated for the presence of humor and its dimensions (sentiment and direction) as proposed in Martin’s Humor Style Questionnaire. We conduct a series of experiments employing pretrained Transformers, convolutional neural networks, and expert-designed features. The performance of each modality (text, audio, video) for spontaneous humor recognition is analyzed and their complementarity is investigated. Our findings suggest that for the automatic analysis of humor and its sentiment, facial expressions are most promising, while humor direction can be best modeled via text-based features. Further, we experiment with different multimodal approaches to humor recognition, including decision-level fusion and MulT, a multimodal Transformer approach. In this context, we propose a novel multimodal architecture that yields the best overall results.
MCML Authors
Heart Sound Abnormality Detection From Multi-Institutional Collaboration: Introducing a Federated Learning Framework.
IEEE Transactions on Biomedical Engineering 71.10 (May. 2024). DOI
Abstract
Objective: Early diagnosis of cardiovascular diseases is a crucial task in medical practice. With the application of computer audition in the healthcare field, artificial intelligence (AI) has been applied to clinical non-invasive intelligent auscultation of heart sounds to provide rapid and effective pre-screening. However, AI models generally require large amounts of data which may cause privacy issues. Unfortunately, it is difficult to collect large amounts of healthcare data from a single centre. Methods: In this study, we propose federated learning (FL) optimisation strategies for the practical application in multi-centre institutional heart sound databases. The horizontal FL is mainly employed to tackle the privacy problem by aligning the feature spaces of FL participating institutions without information leakage. In addition, techniques based on deep learning have poor interpretability due to their “black-box” property, which limits the feasibility of AI in real medical data. To this end, vertical FL is utilised to address the issues of model interpretability and data scarcity. Conclusion: Experimental results demonstrate that, the proposed FL framework can achieve good performance for heart sound abnormality detection by taking the personal privacy protection into account. Moreover, using the federated feature space is beneficial to balance the interpretability of the vertical FL and the privacy of the data. Significance: This work realises the potential of FL from research to clinical practice, and is expected to have extensive application in the federated smart medical system.
MCML Authors
Plug-In Channel Estimation With Dithered Quantized Signals in Spatially Non-Stationary Massive MIMO Systems.
IEEE Transactions on Communications 72.1 (Jan. 2024). DOI
Abstract
As the array dimension of massive MIMO systems increases to unprecedented levels, two problems occur. First, the spatial stationarity assumption along the antenna elements is no longer valid. Second, the large array size results in an unacceptably high power consumption if high-resolution analog-to-digital converters are used. To address these two challenges, we consider a Bussgang linear minimum mean square error (BLMMSE)-based channel estimator for large scale massive MIMO systems with one-bit quantizers and a spatially non-stationary channel. Whereas other works usually assume that the channel covariance is known at the base station, we consider a plug-in BLMMSE estimator that uses an estimate of the channel covariance and rigorously analyze the distortion produced by using an estimated, rather than the true, covariance. To cope with the spatial non-stationarity, we introduce dithering into the quantized signals and provide a theoretical error analysis. In addition, we propose an angular domain fitting procedure which is based on solving an instance of non-negative least squares. For the multi-user data transmission phase, we further propose a BLMMSE-based receiver to handle one-bit quantized data signals. Our numerical results show that the performance of the proposed BLMMSE channel estimator is very close to the oracle-aided scheme with ideal knowledge of the channel covariance matrix. The BLMMSE receiver outperforms the conventional maximum-ratio-combining and zero-forcing receivers in terms of the resulting ergodic sum rate.
MCML Authors
Mathematical Data Science and Artificial Intelligence
A First Look at Generative Artificial Intelligence Based Music Therapy for Mental Disorders.
IEEE Transactions on Consumer Electronics Early Access (Dec. 2024). DOI
Abstract
Mental disorders show a rapid increase and cause considerable harm to individuals as well as the society in recent decade. Hence, mental disorders have become a serious public health challenge in nowadays society. Timely treatment of mental disorders plays a critical role for reducing the harm of mental illness to individuals and society. Music therapy is a type of non-pharmaceutical method in treating such mental disorders. However, conventional music therapy suffers from a number of issues resulting in a lack of popularity. Thanks to the rapid development of Artificial Intelligence (AI), especially the AI Generated Content (AIGC), it provides a chance to address these issues. Nevertheless, to the best of our knowledge, there is no work investigating music therapy from AIGC and closed-loop perspective. In this paper, we summarise some universal music therapy methods and discuss their shortages. Then, we indicate some AIGC techniques, especially the music generation, for their application in music therapy. Moreover, we present a closed-loop music therapy system and introduce its implementation details. Finally, we discuss some challenges in AIGC-based music therapy with proposing further research direction, and we suggest the potential of this system to become a consumer-grade product for treating mental disorders.
MCML Authors
HyperLISTA-ABT: An Ultralight Unfolded Network for Accurate Multicomponent Differential Tomographic SAR Inversion.
IEEE Transactions on Geoscience and Remote Sensing 62 (Apr. 2024). DOI
Abstract
Deep neural networks based on unrolled iterative algorithms have achieved remarkable success in sparse reconstruction applications, such as synthetic aperture radar (SAR) tomographic inversion (TomoSAR). However, the currently available deep learning-based TomoSAR algorithms are limited to 3-D reconstruction. The extension of deep learning-based algorithms to 4-D imaging, i.e., differential TomoSAR (D-TomoSAR) applications, is impeded mainly due to the high-dimensional weight matrices required by the network designed for D-TomoSAR inversion, which typically contain millions of freely trainable parameters. Learning such huge number of weights requires an enormous number of training samples, resulting in a large memory burden and excessive time consumption. To tackle this issue, we propose an efficient and accurate algorithm called HyperLISTA-ABT. The weights in HyperLISTA-ABT are determined in an analytical way according to a minimum coherence criterion, trimming the model down to an ultra-light one with only three hyperparameters. Additionally, HyperLISTA-ABT improves the global thresholding by utilizing an adaptive blockwise thresholding (ABT) scheme, which applies block-coordinate techniques and conducts thresholding in local blocks, so that weak expressions and local features can be retained in the shrinkage step layer by layer. Simulations were performed and demonstrated the effectiveness of our approach, showing that HyperLISTA-ABT achieves superior computational efficiency with no significant performance degradation compared to the state-of-the-art methods. Real data experiments showed that a high-quality 4-D point cloud could be reconstructed over a large area by the proposed HyperLISTA-ABT with affordable computational resources and in a fast time.
MCML Authors
PixelDINO: Semi-Supervised Semantic Segmentation for Detecting Permafrost Disturbances in the Arctic.
IEEE Transactions on Geoscience and Remote Sensing 62 (Aug. 2024). DOI
Abstract
Arctic permafrost is facing significant changes due to global climate change. As these regions are largely inaccessible, remote sensing plays a crucial rule in better understanding the underlying processes across the Arctic. In this study, we focus on the remote detection of retrogressive thaw slumps (RTSs), a permafrost disturbance comparable to slow landslides. For such remote sensing tasks, deep learning has become an indispensable tool, but limited labeled training data remains a challenge for training accurate models. We present PixelDINO, a semi-supervised learning approach, to improve model generalization across the Arctic with a limited number of labels. PixelDINO leverages unlabeled data by training the model to define its own segmentation categories (pseudoclasses), promoting consistent structural learning across strong data augmentations. This allows the model to extract structural information from unlabeled data, supplementing the learning from labeled data. PixelDINO surpasses both supervised baselines and existing semi-supervised methods, achieving average intersection-over-union (IoU) of 30.2 and 39.5 on the two evaluation sets, representing significant improvements of 13% and 21%, respectively, over the strongest existing models. This highlights the potential for training robust models that generalize well to regions that were not included in the training data.
MCML Authors
Multimodal Co-Learning for Building Change Detection: A Domain Adaptation Framework Using VHR Images and Digital Surface Models.
IEEE Transactions on Geoscience and Remote Sensing 62 (Feb. 2024). DOI
Abstract
In this article, we propose a multimodal co-learning framework for building change detection. This framework can be adopted to jointly train a Siamese bitemporal image network and a height difference (HDiff) network with labeled source data and unlabeled target data pairs. Three co-learning combinations (vanilla co-learning, fusion co-learning, and detached fusion co-learning) are proposed and investigated with two types of co-learning loss functions within our framework. Our experimental results demonstrate that the proposed methods are able to take advantage of unlabeled target data pairs and, therefore, enhance the performance of single-modal neural networks on the target data. In addition, our synthetic-to-real experiments demonstrate that the recently published synthetic dataset, Simulated Multimodal Aerial Remote Sensing (SMARS), is feasible to be used in real change detection scenarios, where the optimal result is with the F1 score of 79.29%.
MCML Authors
Few-Shot Object Detection in Remote Sensing: Lifting the Curse of Incompletely Annotated Novel Objects.
IEEE Transactions on Geoscience and Remote Sensing 62 (Jan. 2024). DOI GitHub
Abstract
Object detection (OD) is an essential and fundamental task in computer vision (CV) and satellite image processing. Existing deep learning methods have achieved impressive performance thanks to the availability of large-scale annotated datasets. Yet, in real-world applications, the availability of labels is limited. In this article, few-shot OD (FSOD) has emerged as a promising direction, which aims at enabling the model to detect novel objects with only few of them annotated. However, many existing FSOD algorithms overlook a critical issue: when an input image contains multiple novel objects and only a subset of them are annotated, the unlabeled objects will be considered as background during training. This can cause confusions and severely impact the model’s ability to recall novel objects. To address this issue, we propose a self-training-based FSOD (ST-FSOD) approach, which incorporates the self-training mechanism into the few-shot fine-tuning process. ST-FSOD aims to enable the discovery of novel objects that are not annotated and take them into account during training. On the one hand, we devise a two-branch region proposal networks (RPNs) to separate the proposal extraction of base and novel objects. On the another hand, we incorporate the student-teacher mechanism into RPN and the region-of-interest (RoI) head to include those highly confident yet unlabeled targets as pseudolabels. Experimental results demonstrate that our proposed method outperforms the state of the art in various FSOD settings by a large margin.
MCML Authors
Self-Supervised Pretraining With Monocular Height Estimation for Semantic Segmentation.
IEEE Transactions on Geoscience and Remote Sensing 62 (Jul. 2024). DOI GitHub
Abstract
Monocular height estimation (MHE) is key for generating 3-D city models, essential for swift disaster response. Moving beyond the traditional focus on performance enhancement, our study breaks new ground by probing the interpretability of MHE networks. We have pioneeringly discovered that neurons within MHE models demonstrate selectivity for both height and semantic classes. This insight sheds light on the complex inner workings of MHE models and inspires innovative strategies for leveraging elevation data more effectively. Informed by this insight, we propose a pioneering framework that employs MHE as a self-supervised pretraining method for remote sensing (RS) imagery. This approach significantly enhances the performance of semantic segmentation tasks. Furthermore, we develop a disentangled latent transformer (DLT) module that leverages explainable deep representations from pretrained MHE networks for unsupervised semantic segmentation. Our method demonstrates the significant potential of MHE tasks in developing foundation models for sophisticated pixel-level semantic analyses. Additionally, we present a new dataset designed to benchmark the performance of both semantic segmentation and height estimation tasks.
MCML Authors
MaskCD: A Remote Sensing Change Detection Network Based on Mask Classification.
IEEE Transactions on Geoscience and Remote Sensing 62 (Jul. 2024). DOI GitHub
Abstract
Change detection (CD) from remote sensing (RS) images using deep learning has been widely investigated in the literature. It is typically regarded as a pixelwise labeling task that aims to classify each pixel as changed or unchanged. Although per-pixel classification networks in encoder-decoder structures have shown dominance, they still suffer from imprecise boundaries and incomplete object delineation at various scenes. For high-resolution RS images, partly or totally changed objects are more worthy of attention rather than a single pixel. Therefore, we revisit the CD task from the mask prediction and classification perspective and propose mask classification-based CD (MaskCD) to detect changed areas by adaptively generating categorized masks from input image pairs. Specifically, it utilizes a cross-level change representation perceiver (CLCRP) to learn multiscale change-aware representations and capture spatiotemporal relations from encoded features by exploiting deformable multihead self-attention (DeformMHSA). Subsequently, a masked cross-attention-based detection transformers (MCA-DETRs) decoder is developed to accurately locate and identify changed objects based on masked cross-attention and self-attention (SA) mechanisms. It reconstructs the desired changed objects by decoding the pixelwise representations into learnable mask proposals and making final predictions from these candidates. Experimental results on five benchmark datasets demonstrate the proposed approach outperforms other state-of-the-art models.
MCML Authors
A Review of Building Extraction From Remote Sensing Imagery: Geometrical Structures and Semantic Attributes.
IEEE Transactions on Geoscience and Remote Sensing 62 (Mar. 2024). DOI
Abstract
In the remote sensing community, extracting buildings from remote sensing imagery has triggered great interest. While many studies have been conducted, a comprehensive review of these approaches that are applied to optical and synthetic aperture radar (SAR) imagery is still lacking. Therefore, we provide an in-depth review of both early efforts and recent advances, which are aimed at extracting geometrical structures or semantic attributes of buildings, including building footprint generation, building facade segmentation, roof segment and superstructure segmentation, building height retrieval, building-type classification, building change detection, and annotation data correction. Furthermore, a list of corresponding benchmark datasets is given. Finally, challenges and outlooks of existing approaches as well as promising applications are discussed to enhance comprehension within this realm of research.
MCML Authors
RRSIS: Referring Remote Sensing Image Segmentation.
IEEE Transactions on Geoscience and Remote Sensing 62 (Mar. 2024). DOI GitHub
Abstract
Localizing desired objects from remote sensing images is of great use in practical applications. Referring image segmentation, which aims at segmenting out the objects to which a given expression refers, has been extensively studied in natural images. However, almost no research attention is given to this task of remote sensing imagery. Considering its potential for real-world applications, in this article, we introduce referring remote sensing image segmentation (RRSIS) to fill in this gap and make some insightful explorations. Specifically, we created a new dataset, called RefSegRS, for this task, enabling us to evaluate different methods. Afterward, we benchmark referring image segmentation methods of natural images on the RefSegRS dataset and find that these models show limited efficacy in detecting small and scattered objects. To alleviate this issue, we propose a language-guided cross-scale enhancement (LGCE) module that utilizes linguistic features to adaptively enhance multiscale visual features by integrating both deep and shallow features. The proposed dataset, benchmarking results, and the designed LGCE module provide insights into the design of a better RRSIS model.
MCML Authors
Attention-ConvNet Network for Ocean-Front Prediction via Remote Sensing SST Images.
IEEE Transactions on Geoscience and Remote Sensing 62 (Nov. 2024). DOI GitHub
Abstract
Ocean front is one typical geophysical phenomenon acting as oases in the ocean for fishes and marine mammals. Accurate ocean-front prediction is critical for fishery and navigation safety. However, the formation and evolution of ocean fronts are inherently nonlinear and are influenced by various factors such as ocean currents, wind fields, and temperature changes, making ocean-front prediction a considerable challenge. This study proposes a temporal-sensitive network named Attention-ConvNet to address this challenge. Ocean fronts exhibit significant multiscale characteristics, requiring analysis and prediction across various temporal and spatial scales. The proposed network designs a hierarchical attention mechanism (HAM) that efficiently prioritizes relevant spatial and temporal information to meet the specific requirement. What is more, the proposed network uses a complex hierarchical branching convolutional network (HBCNet) architecture, which allows our network to leverage the complementary strengths of spatial and temporal information, effectively capturing the dynamic and complex variations in ocean fronts. In general, the network prioritizes and focuses on the most relevant information of front dynamics, which ensures its ability to effectively predict the ocean front. External experiments demonstrate that our network significantly outperforms conventional methods, confirming its capability for precise ocean-front prediction.
MCML Authors
MineNetCD: A Benchmark for Global Mining Change Detection on Remote Sensing Imagery.
IEEE Transactions on Geoscience and Remote Sensing 62 (Nov. 2024). DOI
Abstract
Monitoring land changes triggered by mining activities is crucial for industrial control, environmental management, and regulatory compliance, yet it poses significant challenges due to the vast and often remote locations of mining sites. Remote sensing technologies have increasingly become indispensable to detect and analyze these changes over time. We thus introduce MineNetCD, a comprehensive benchmark designed for global mining change detection using remote sensing imagery. The benchmark comprises three key contributions. First, we establish a global mining change detection dataset featuring more than 70k paired patches of bitemporal high-resolution remote sensing images and pixel-level annotations from 100 mining sites worldwide. Second, we develop a novel baseline model based on a change-aware fast Fourier transform (ChangeFFT) module, which enhances various backbones by leveraging essential spectrum components within features in the frequency domain and capturing the channelwise correlation of bitemporal feature differences to learn change-aware representations. Third, we construct a unified change detection (UCD) framework that currently integrates 20 change detection methods. This framework is designed for streamlined and efficient processing, using the cloud platform hosted by HuggingFace. Extensive experiments have been conducted to demonstrate the superiority of the proposed baseline model compared with 19 state-of-the-art change detection approaches. Empirical studies on modularized backbones comprehensively confirm the efficacy of different representation learners on change detection. This benchmark represents significant advancements in the field of remote sensing and change detection, providing a robust resource for future research and applications in global mining monitoring.
MCML Authors
Multilabel-Guided Soft Contrastive Learning for Efficient Earth Observation Pretraining.
IEEE Transactions on Geoscience and Remote Sensing 62 (Oct. 2024). DOI GitHub
Abstract
Self-supervised pretraining on large-scale satellite data has raised great interest in building Earth observation (EO) foundation models. However, many important resources beyond pure satellite imagery, such as land-cover-land-use products that provide free global semantic information, as well as vision foundation models that hold strong knowledge of the natural world, are not widely studied. In this work, we show these free additional resources not only help resolve common contrastive learning bottlenecks but also significantly boost the efficiency and effectiveness of EO pretraining. Specifically, we first propose soft contrastive learning (SoftCon) that optimizes cross-scene soft similarity based on land-cover-generated multilabel supervision, naturally solving the issue of multiple positive samples and too strict positive matching in complex scenes. Second, we revisit and explore cross-domain continual pretraining for both multispectral and synthetic aperture radar (SAR) imagery, building efficient EO foundation models from strongest vision models such as DINOv2. Adapting simple weight-initialization and Siamese masking strategies into our SoftCon framework, we demonstrate impressive continual pretraining performance even when the input modalities are not aligned. Without prohibitive training, we produce multispectral and SAR foundation models that achieve significantly better results in 10 out of 11 downstream tasks than most existing SOTA models. For example, our ResNet50/ViT-S achieve 84.8/85.0 linear probing mAP scores on BigEarthNet-10%, which are better than most existing ViT-L models; under the same setting, our ViT-B sets a new record of 86.8 in multispectral, and 82.5 in SAR, the latter even better than many multispectral models.
MCML Authors
Computability of Optimizers.
IEEE Transactions on Information Theory 70.4 (Apr. 2024). DOI
Abstract
Optimization problems are a staple of today’s scientific and technical landscape. However, at present, solvers of such problems are almost exclusively run on digital hardware. Using Turing machines as a mathematical model for any type of digital hardware, in this paper, we analyze fundamental limitations of this conceptual approach of solving optimization problems. Since in most applications, the optimizer itself is of significantly more interest than the optimal value of the corresponding function, we will focus on computability of the optimizer. In fact, we will show that in various situations the optimizer is unattainable on Turing machines and consequently on digital computers. Moreover, even worse, there does not exist a Turing machine, which approximates the optimizer itself up to a certain constant error. We prove such results for a variety of well-known problems from very different areas, including artificial intelligence, financial mathematics, and information theory, often deriving the even stronger result that such problems are not Banach-Mazur computable, also not even in an approximate sense.
MCML Authors
Mathematical Foundations of Artificial Intelligence
An Immersive and Interactive VR Dataset to Elicit Emotions.
IEEE Transactions on Visualization and Computer Graphics 30.11 (Sep. 2024). DOI
Abstract
Images and videos are widely used to elicit emotions; however, their visual appeal differs from real-world experiences. With virtual reality becoming more realistic, immersive, and interactive, we envision virtual environments to elicit emotions effectively, rapidly, and with high ecological validity. This work presents the first interactive virtual reality dataset to elicit emotions. We created five interactive virtual environments based on corresponding validated 360° videos and validated their effectiveness with 160 participants. Our results show that our virtual environments successfully elicit targeted emotions. Compared with the existing methods using images or videos, our dataset allows virtual reality researchers and practitioners to integrate their designs effectively with emotion elicitation settings in an immersive and interactive way.
MCML Authors
Adaptive FEM with quasi-optimal overall cost for nonsymmetric linear elliptic PDEs.
IMA Journal of Numerical Analysis 44.3 (May. 2024). DOI
Abstract
We consider a general nonsymmetric second-order linear elliptic partial differential equation in the framework of the Lax–Milgram lemma. We formulate and analyze an adaptive finite element algorithm with arbitrary polynomial degree that steers the adaptive meshrefinement and the inexact iterative solution of the arising linear systems. More precisely, the iterative solver employs, as an outer loop, the so-called Zarantonello iteration to symmetrize the system and, as an inner loop, a uniformly contractive algebraic solver, for example, an optimally preconditioned conjugate gradient method or an optimal geometric multigrid algorithm. We prove that the proposed inexact adaptive iteratively symmetrized finite element method leads to full linear convergence and, for sufficiently small adaptivity parameters, to optimal convergence rates with respect to the overall computational cost, i.e., the total computational time. Numerical experiments underline the theory.
MCML Authors
Interpretable deep learning for consistent large-scale urban population estimation using Earth observation data.
International Journal of Applied Earth Observation and Geoinformation 128 (Apr. 2024). DOI
Abstract
Accurate and up-to-date mapping of the human population is fundamental for a wide range of disciplines, from effective governance and establishing policies to disaster management and crisis dilution. The traditional method of gathering population data through census is costly and time-consuming. Recently, with the availability of large amounts of Earth observation data sets, deep learning methods have been explored for population estimation; however, they are either limited by census data availability, inter-regional evaluations, or transparency. In this paper, we present an end-to-end interpretable deep learning framework for large-scale population estimation at a resolution of 1 km that uses only the publicly available data sets and does not rely on census data for inference. The architecture is based on a modification of the common ResNet-50 architecture tailored to analyze both image-like and vector-like data. Our best model outperforms the baseline random forest model by improving the RMSE by around 9% and also surpasses the community standard product, GHS-POP, thus yielding promising results. Furthermore, we improve the transparency of the proposed model by employing an explainable AI technique that identified land use information to be the most relevant feature for population estimation. We expect the improved interpretation of the model outcome will inspire both academic and non-academic end users, particularly those investigating urbanization or sub-urbanization trends, to have confidence in the deep learning methods for population estimation.
MCML Authors
Data Science in Earth Observation
Learning decision catalogues for situated decision making: The case of scoring systems.
International Journal of Approximate Reasoning 171 (Aug. 2024). DOI
Abstract
In this paper, we formalize the problem of learning coherent collections of decision models, which we call decision catalogues, and illustrate it for the case where models are scoring systems. This problem is motivated by the recent rise of algorithmic decision-making and the idea to improve human decision-making through machine learning, in conjunction with the observation that decision models should be situated in terms of their complexity and resource requirements: Instead of constructing a single decision model and using this model in all cases, different models might be appropriate depending on the decision context. Decision catalogues are supposed to support a seamless transition from very simple, resource-efficient to more sophisticated but also more demanding models. We present a general algorithmic framework for inducing such catalogues from training data, which tackles the learning task as a problem of searching the space of candidate catalogues systematically and, to this end, makes use of heuristic search methods. We also present a concrete instantiation of this framework as well as empirical studies for performance evaluation, which, in a nutshell, show that greedy search is an efficient and hard-to-beat strategy for the construction of catalogues of scoring systems.
MCML Authors
Artificial Intelligence and Machine Learning
Radiological age assessment based on clavicle ossification in CT: enhanced accuracy through deep learning.
International Journal of Legal Medicine (Jan. 2024). DOI
Abstract
Background: Radiological age assessment using reference studies is inherently limited in accuracy due to a finite number of assignable skeletal maturation stages. To overcome this limitation, we present a deep learning approach for continuous age assessment based on clavicle ossification in computed tomography (CT).
Methods: Thoracic CT scans were retrospectively collected from the picture archiving and communication system. Individuals aged 15.0 to 30.0 years examined in routine clinical practice were included. All scans were automatically cropped around the medial clavicular epiphyseal cartilages. A deep learning model was trained to predict a person’s chronological age based on these scans. Performance was evaluated using mean absolute error (MAE). Model performance was compared to an optimistic human reader performance estimate for an established reference study method.
Results: The deep learning model was trained on 4,400 scans of 1,935 patients (training set: mean age =
24.2 years ± 4.0, 1132 female) and evaluated on 300 scans of 300 patients with a balanced age and sex distribution (test set: mean age = 22.5 years ± 4.4, 150 female). Model MAE was 1.65 years, and the highest absolute error was 6.40 years for females and 7.32 years for males. However, performance could be attributed to norm-variants or pathologic disorders. Human reader estimate MAE was 1.84 years and the highest absolute error was 3.40 years for females and 3.78 years for males.
Conclusions: We present a deep learning approach for continuous age predictions using CT volumes highlighting the medial clavicular epiphyseal cartilage with performance comparable to the human reader estimate.
MCML Authors
Continent-wide urban tree canopy fine-scale mapping and coverage assessment in South America with high-resolution satellite images.
ISPRS Journal of Photogrammetry and Remote Sensing 212 (Jun. 2024). DOI
Abstract
Urban development in South America has experienced significant growth and transformation over the past few decades. South America’s urban development and trees are closely interconnected, and tree cover within cities plays a vital role in shaping sustainable and resilient urban landscapes. However, knowledge of urban tree canopy (UTC) coverage in the South American continent remains limited. In this study, we used high-resolution satellite images and developed a semi-supervised deep learning method to create UTC data for 888 South American cities. The proposed semi-supervised method can leverage both labeled and unlabeled data during training. By incorporating labeled data for guidance and utilizing unlabeled data to explore underlying patterns, the algorithm enhances model robustness and generalization for urban tree canopy detection across South America, with an average overall accuracy of 94.88% for the tested cities. Based on the created UTC products, we successfully assessed the UTC coverage for each city. Statistical results showed that the UTC coverage in South America is between 0.76% and 69.53%, and the average UTC coverage is approximately 19.99%. Among the 888 cities, only 357 cities that accommodate approximately 48.25% of the total population have UTC coverage greater than 20%, while the remaining 531 cities that accommodate approximately 51.75% of the total population have UTC coverage less than 20%. Natural factors (climatic and geographical) play a very important role in determining UTC coverage, followed by human activity factors (economy and urbanization level). We expect that the findings of this study and the created UTC dataset will help formulate policies and strategies to promote sustainable urban forestry, thus further improving the quality of life of residents in South America.
MCML Authors
Decouple and weight semi-supervised semantic segmentation of remote sensing images.
ISPRS Journal of Photogrammetry and Remote Sensing 212 (Jun. 2024). DOI GitHub
Abstract
Semantic understanding of high-resolution remote sensing (RS) images is of great value in Earth observation, however, it heavily depends on numerous pixel-wise manually-labeled data, which is laborious and thereby limits its practical application. Semi-supervised semantic segmentation (SSS) of RS images would be a promising solution, which uses both limited labeled data and dominant unlabeled data to train segmentation models, significantly mitigating the annotation burden. The current mainstream methods of remote sensing semi-supervised semantic segmentation (RS-SSS) utilize the hard or soft pseudo-labels of unlabeled data for model training and achieve excellent performance. Nevertheless, their performance is bottlenecked because of two inherent problems: irreversible wrong pseudo-labels and long-tailed distribution among classes. Aiming at them, we propose a decoupled weighting learning (DWL) framework for RS-SSS in this study, which consists of two novel modules, decoupled learning and ranking weighting, corresponding to the above two problems, respectively. During training, the decoupled learning module separates the predictions of the labeled and unlabeled data to decrease the negative impact of the self-training of the wrongly pseudo-labeled unlabeled data on the supervised training of the labeled data. Furthermore, the ranking weighting module tries to adaptively weight each pseudo-label of the unlabeled data according to its relative confidence ranking in its pseudo-class to alleviate model bias to majority classes as a result of the long-tailed distribution. To verify the effectiveness of the proposed DWL framework, extensive experiments are conducted on three widely-used RS semantic segmentation datasets in the semi-supervised setting. The experimental results demonstrate the superiority of our method to some state-of-the-art SSS methods.
MCML Authors
CloudSeg: A multi-modal learning framework for robust land cover mapping under cloudy conditions.
ISPRS Journal of Photogrammetry and Remote Sensing 214 (Aug. 2024). DOI GitHub
Abstract
Cloud coverage poses a significant challenge to optical image interpretation, degrading ground information on Earth’s surface. Synthetic aperture radar (SAR), with its ability to penetrate clouds, provides supplementary information to optical data. However, existing optical-SAR fusion methods predominantly focus on cloud-free scenarios, neglecting the practical challenge of semantic segmentation under cloudy conditions. To tackle this issue, we propose CloudSeg, a novel framework tailored for land cover mapping in the presence of clouds. It addresses the challenges posed by cloud cover from two aspects: reducing semantic ambiguity in areas of the cloudy image that are obscured by clouds and enhancing effective information in the unobstructed portions. Specifically, CloudSeg employs a multi-task learning strategy to simultaneously handle low-level visual task and high-level semantic understanding task, mitigating the semantic ambiguity caused by cloud cover by acquiring discriminative features through an auxiliary cloud removal task. Additionally, CloudSeg incorporates a knowledge distillation strategy, which utilizes the knowledge learned by the teacher network under cloud-free conditions to guide the student network to overcome the interference of cloud-covered areas, enhancing the valuable information from the unobstructed parts of cloud-covered images. Extensive experiments conducted on two datasets, M3M-CR and WHU-OPT-SAR, demonstrate the effectiveness and superiority of the proposed CloudSeg method for land cover mapping under cloudy conditions. Specifically, CloudSeg outperforms the state-of-the-art competitors by 3.16% in terms of mIoU on M3M-CR and by 5.56% on WHU-OPT-SAR, highlighting its substantial advantages for analyzing regions frequently obscured by clouds.
MCML Authors
PolyGNN: Polyhedron-based graph neural network for 3D building reconstruction from point clouds.
ISPRS Journal of Photogrammetry and Remote Sensing 218.A (Dec. 2024). DOI GitHub
Abstract
We present PolyGNN, a polyhedron-based graph neural network for 3D building reconstruction from point clouds. PolyGNN learns to assemble primitives obtained by polyhedral decomposition via graph node classification, achieving a watertight and compact reconstruction. To effectively represent arbitrary-shaped polyhedra in the neural network, we propose a skeleton-based sampling strategy to generate polyhedron-wise queries. These queries are then incorporated with inter-polyhedron adjacency to enhance the classification. PolyGNN is end-to-end optimizable and is designed to accommodate variable-size input points, polyhedra, and queries with an index-driven batching technique. To address the abstraction gap between existing city-building models and the underlying instances, and provide a fair evaluation of the proposed method, we develop our method on a large-scale synthetic dataset with well-defined ground truths of polyhedral labels. We further conduct a transferability analysis across cities and on real-world point clouds. Both qualitative and quantitative results demonstrate the effectiveness of our method, particularly its efficiency for large-scale reconstructions.
MCML Authors
Can Fairness be Automated? Guidelines and Opportunities for Fairness-aware AutoML.
Journal of Artificial Intelligence Research 79 (Feb. 2024). DOI
Abstract
The field of automated machine learning (AutoML) introduces techniques that automate parts of the development of machine learning (ML) systems, accelerating the process and reducing barriers for novices. However, decisions derived from ML models can reproduce, amplify, or even introduce unfairness in our societies, causing harm to (groups of) individuals. In response, researchers have started to propose AutoML systems that jointly optimize fairness and predictive performance to mitigate fairness-related harm. However, fairness is a complex and inherently interdisciplinary subject, and solely posing it as an optimization problem can have adverse side effects. With this work, we aim to raise awareness among developers of AutoML systems about such limitations of fairness-aware AutoML, while also calling attention to the potential of AutoML as a tool for fairness research. We present a comprehensive overview of different ways in which fairness-related harm can arise and the ensuing implications for the design of fairness-aware AutoML. We conclude that while fairness cannot be automated, fairness-aware AutoML can play an important role in the toolbox of ML practitioners. We highlight several open technical challenges for future work in this direction. Additionally, we advocate for the creation of more user-centered assistive systems designed to tackle challenges encountered in fairness work.
MCML Authors
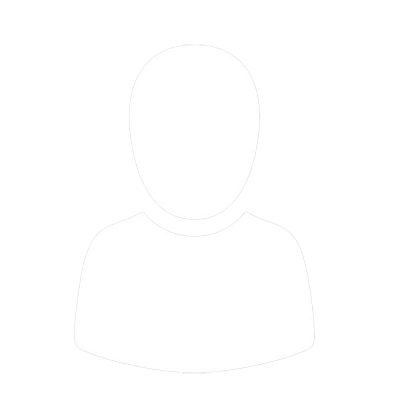
Florian Pfisterer
Dr.
* Former Member
Statistical Learning and Data Science
Statistical Learning and Data Science
Strategies to optimise machine learning classification performance when using biomechanical features.
Journal of Biomechanics 165 (Mar. 2024). DOI
Abstract
Building prediction models using biomechanical features is challenging because such models may require large sample sizes. However, collecting biomechanical data on large sample sizes is logistically very challenging. This study aims to investigate if modern machine learning algorithms can help overcome the issue of limited sample sizes on developing prediction models. This was a secondary data analysis two biomechanical datasets – a walking dataset on 2295 participants, and a countermovement jump dataset on 31 participants. The input features were the three-dimensional ground reaction forces (GRFs) of the lower limbs. The outcome was the orthopaedic disease category (healthy, calcaneus, ankle, knee, hip) in the walking dataset, and healthy vs people with patellofemoral pain syndrome in the jump dataset. Different algorithms were compared: multinomial/LASSO regression, XGBoost, various deep learning time-series algorithms with augmented data, and with transfer learning. For the outcome of weighted multiclass area under the receiver operating curve (AUC) in the walking dataset, the three models with the best performance were InceptionTime with x12 augmented data (0.810), XGBoost (0.804), and multinomial logistic regression (0.800). For the jump dataset, the top three models with the highest AUC were the LASSO (1.00), InceptionTime with x8 augmentation (0.750), and transfer learning (0.653). Machine-learning based strategies for managing the challenging issue of limited sample size for biomechanical ML-based problems, could benefit the development of alternative prediction models in healthcare, especially when time-series data are involved.
MCML Authors
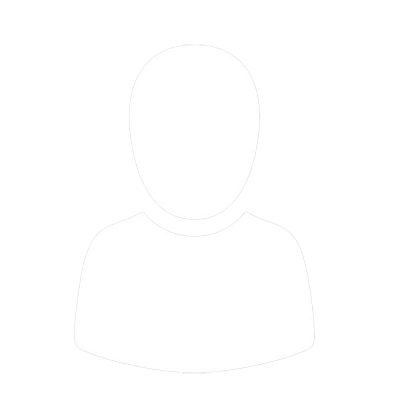
Florian Pfisterer
Dr.
* Former Member
Statistics, Data Science and Machine Learning
AMLB: an AutoML Benchmark.
Journal of Machine Learning Research 25.101 (Feb. 2024). URL
Abstract
Comparing different AutoML frameworks is notoriously challenging and often done incorrectly. We introduce an open and extensible benchmark that follows best practices and avoids common mistakes when comparing AutoML frameworks. We conduct a thorough comparison of 9 well-known AutoML frameworks across 71 classification and 33 regression tasks. The differences between the AutoML frameworks are explored with a multi-faceted analysis, evaluating model accuracy, its trade-offs with inference time, and framework failures. We also use Bradley-Terry trees to discover subsets of tasks where the relative AutoML framework rankings differ. The benchmark comes with an open-source tool that integrates with many AutoML frameworks and automates the empirical evaluation process end-to-end: from framework installation and resource allocation to in-depth evaluation. The benchmark uses public data sets, can be easily extended with other AutoML frameworks and tasks, and has a website with up-to-date results.
MCML Authors
Statistical Learning and Data Science
Decomposing Global Feature Effects Based on Feature Interactions.
Journal of Machine Learning Research 25.381 (Dec. 2024). URL
Abstract
Global feature effect methods, such as partial dependence plots, provide an intelligible visualization of the expected marginal feature effect. However, such global feature effect methods can be misleading, as they do not represent local feature effects of single observations well when feature interactions are present. We formally introduce generalized additive decomposition of global effects (GADGET), which is a new framework based on recursive partitioning to find interpretable regions in the feature space such that the interaction-related heterogeneity of local feature effects is minimized. We provide a mathematical foundation of the framework and show that it is applicable to the most popular methods to visualize marginal feature effects, namely partial dependence, accumulated local effects, and Shapley additive explanations (SHAP) dependence. Furthermore, we introduce and validate a new permutation-based interaction detection procedure that is applicable to any feature effect method that fits into our proposed framework. We empirically evaluate the theoretical characteristics of the proposed methods based on various feature effect methods in different experimental settings. Moreover, we apply our introduced methodology to three real-world examples to showcase their usefulness.
MCML Authors
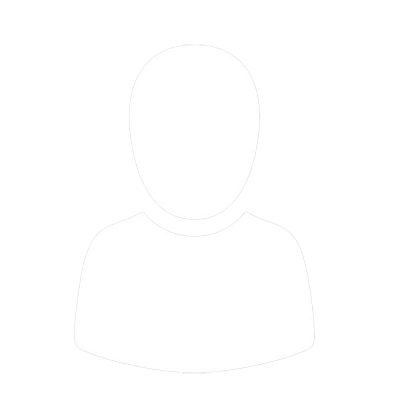
Julia Herbinger
Dr.
* Former Member
Computational Statistics & Data Science
Statistical Learning and Data Science
Statistical Learning and Data Science
Estimating Conditional Distributions with Neural Networks Using R Package deeptrafo.
Journal of Statistical Software 111.10 (2024). DOI
Abstract
Contemporary empirical applications frequently require flexible regression models for complex response types and large tabular or non-tabular, including image or text, data. Classical regression models either break down under the computational load of processing such data or require additional manual feature extraction to make these problems tractable. Here, we present deeptrafo, a package for fitting flexible regression models for conditional distributions using a tensorflow backend with numerous additional processors, such as neural networks, penalties, and smoothing splines. Package deeptrafo implements deep conditional transformation models (DCTMs) for binary, ordinal, count, survival, continuous, and time series responses, potentially with uninformative censoring. Unlike other available methods, DCTMs do not assume a parametric family of distributions for the response. Further, the data analyst may trade off interpretability and flexibility by supplying custom neural network architectures and smoothers for each term in an intuitive formula interface. We demonstrate how to set up, fit, and work with DCTMs for several response types. We further showcase how to construct ensembles of these models, evaluate models using inbuilt cross-validation, and use other convenience functions for DCTMs in several applications. Lastly, we discuss DCTMs in light of other approaches to regression with non-tabular data.
MCML Authors
Statistics, Data Science and Machine Learning
Testing many constraints in possibly irregular models using incomplete U-statistics.
Journal of the Royal Statistical Society. Series B (Statistical Methodology) 86.4 (Mar. 2024). DOI
Abstract
We consider the problem of testing a null hypothesis defined by equality and inequality constraints on a statistical parameter. Testing such hypotheses can be challenging because the number of relevant constraints may be on the same order or even larger than the number of observed samples. Moreover, standard distributional approximations may be invalid due to irregularities in the null hypothesis. We propose a general testing methodology that aims to circumvent these difficulties. The constraints are estimated by incomplete U-statistics, and we derive critical values by Gaussian multiplier bootstrap. We show that the bootstrap approximation of incomplete U-statistics is valid for kernels that we call mixed degenerate when the number of combinations used to compute the incomplete U-statistic is of the same order as the sample size. It follows that our test controls type I error even in irregular settings. Furthermore, the bootstrap approximation covers high-dimensional settings making our testing strategy applicable for problems with many constraints. The methodology is applicable, in particular, when the constraints to be tested are polynomials in U-estimable parameters. As an application, we consider goodness-of-fit tests of latent-tree models for multivariate data.
MCML Authors
Mathematical Statistics
Fusing structure from motion and simulation-augmented pose regression from optical flow for challenging indoor environments.
Journal of Visual Communication and Image Representation 103 (Aug. 2024). DOI
Abstract
The localization of objects is essential in many applications, such as robotics, virtual and augmented reality, and warehouse logistics. Recent advancements in deep learning have enabled localization using monocular cameras. Traditionally, structure from motion (SfM) techniques predict an object’s absolute position from a point cloud, while absolute pose regression (APR) methods use neural networks to understand the environment semantically. However, both approaches face challenges from environmental factors like motion blur, lighting changes, repetitive patterns, and featureless areas. This study addresses these challenges by incorporating additional information and refining absolute pose estimates with relative pose regression (RPR) methods. RPR also struggles with issues like motion blur. To overcome this, we compute the optical flow between consecutive images using the Lucas–Kanade algorithm and use a small recurrent convolutional network to predict relative poses. Combining absolute and relative poses is difficult due to differences between global and local coordinate systems. Current methods use pose graph optimization (PGO) to align these poses. In this work, we propose recurrent fusion networks to better integrate absolute and relative pose predictions, enhancing the accuracy of absolute pose estimates. We evaluate eight different recurrent units and create a simulation environment to pre-train the APR and RPR networks for improved generalization. Additionally, we record a large dataset of various scenarios in a challenging indoor environment resembling a warehouse with transportation robots. Through hyperparameter searches and experiments, we demonstrate that our recurrent fusion method outperforms PGO in effectiveness.
MCML Authors
Statistics, Data Science and Machine Learning
Statistical Learning and Data Science
High-resolution satellite images reveal the prevalent positive indirect impact of urbanization on urban tree canopy coverage in South America.
Landscape and Urban Planning 247 (Apr. 2024). DOI
Abstract
Trees in urban areas act as carbon sinks and provide ecosystem services for residents. However, the impact of urbanization on tree coverage in South America remains poorly understood. Here, we make use of very high resolution satellite imagery to derive urban tree coverage for 882 cities in South America and developed a tree coverage impacted (TCI) coefficient to quantify the direct and indirect impacts of urbanization on urban tree canopy (UTC) coverage. The direct effect refers to the change in tree cover due to the rise in urban intensity compared to scenarios with extremely low levels of urbanization, while the indirect impact refers to the change in tree coverage resulting from human management practices and alterations in urban environments. Our study revealed the negative direct impacts and prevalent positive indirect impacts of urbanization on UTC coverage. In South America, 841 cities exhibit positive indirect impacts, while only 41 cities show negative indirect impacts. The prevalent positive indirect effects can offset approximately 48% of the direct loss of tree coverage due to increased urban intensity, with full offsets achieved in Argentinian and arid regions of South America. In addition, human activity factors play the most important role in determining the indirect effects of urbanization on UTC coverage, followed by climatic and geographic factors. These findings will help us understand the impact of urbanization on UTC coverage along the urban intensity gradient and formulate policies and strategies to promote sustainable urban development in South America.
MCML Authors
CoMadOut—a robust outlier detection algorithm based on CoMAD.
Machine Learning 113 (May. 2024). DOI
Abstract
Unsupervised learning methods are well established in the area of anomaly detection and achieve state of the art performances on outlier datasets. Outliers play a significant role, since they bear the potential to distort the predictions of a machine learning algorithm on a given dataset. Especially among PCA-based methods, outliers have an additional destructive potential regarding the result: they may not only distort the orientation and translation of the principal components, they also make it more complicated to detect outliers. To address this problem, we propose the robust outlier detection algorithm CoMadOut, which satisfies two required properties: (1) being robust towards outliers and (2) detecting them. Our CoMadOut outlier detection variants using comedian PCA define, dependent on its variant, an inlier region with a robust noise margin by measures of in-distribution (variant CMO) and optimized scores by measures of out-of-distribution (variants CMO*), e.g. kurtosis-weighting by CMO+k. These measures allow distribution based outlier scoring for each principal component, and thus, an appropriate alignment of the degree of outlierness between normal and abnormal instances. Experiments comparing CoMadOut with traditional, deep and other comparable robust outlier detection methods showed that the performance of the introduced CoMadOut approach is competitive to well established methods related to average precision (AP), area under the precision recall curve (AUPRC) and area under the receiver operating characteristic (AUROC) curve. In summary our approach can be seen as a robust alternative for outlier detection tasks.
MCML Authors
Neural deformation fields for template-based reconstruction of cortical surfaces from MRI.
Medical Image Analysis 93 (Jan. 2024). DOI
Abstract
The reconstruction of cortical surfaces is a prerequisite for quantitative analyses of the cerebral cortex in magnetic resonance imaging (MRI). Existing segmentation-based methods separate the surface registration from the surface extraction, which is computationally inefficient and prone to distortions. We introduce Vox2Cortex-Flow (V2C-Flow), a deep mesh-deformation technique that learns a deformation field from a brain template to the cortical surfaces of an MRI scan. To this end, we present a geometric neural network that models the deformation-describing ordinary differential equation in a continuous manner. The network architecture comprises convolutional and graph-convolutional layers, which allows it to work with images and meshes at the same time. V2C-Flow is not only very fast, requiring less than two seconds to infer all four cortical surfaces, but also establishes vertex-wise correspondences to the template during reconstruction. In addition, V2C-Flow is the first approach for cortex reconstruction that models white matter and pial surfaces jointly, therefore avoiding intersections between them. Our comprehensive experiments on internal and external test data demonstrate that V2C-Flow results in cortical surfaces that are state-of-the-art in terms of accuracy. Moreover, we show that the established correspondences are more consistent than in FreeSurfer and that they can directly be utilized for cortex parcellation and group analyses of cortical thickness.
MCML Authors
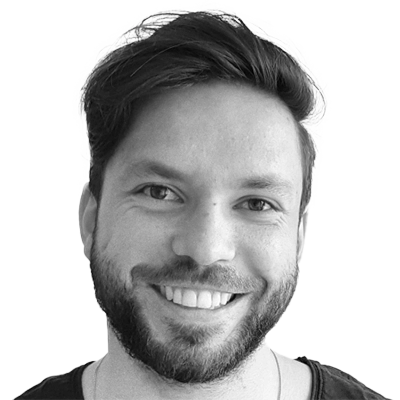
Fabian Bongratz
Artificial Intelligence in Medical Imaging
Benchmarking deep learning-based low-dose CT image denoising algorithms.
Medical Physics 51 (Sep. 2024). DOI
Abstract
Background: Long-lasting efforts have been made to reduce radiation dose and thus the potential radiation risk to the patient for computed tomography (CT) acquisitions without severe deterioration of image quality. To this end, various techniques have been employed over the years including iterative reconstruction methods and noise reduction algorithms.
Purpose: Recently, deep learning-based methods for noise reduction became increasingly popular and a multitude of papers claim ever improving performance both quantitatively and qualitatively. However, the lack of a standardized benchmark setup and inconsistencies in experimental design across studies hinder the verifiability and reproducibility of reported results.
Methods: In this study, we propose a benchmark setup to overcome those flaws and improve reproducibility and verifiability of experimental results in the field. We perform a comprehensive and fair evaluation of several state-of-the-art methods using this standardized setup.
Results: Our evaluation reveals that most deep learning-based methods show statistically similar performance, and improvements over the past years have been marginal at best.
Conclusions: This study highlights the need for a more rigorous and fair evaluation of novel deep learning-based methods for low-dose CT image denoising. Our benchmark setup is a first and important step towards this direction and can be used by future researchers to evaluate their algorithms.
MCML Authors
Retinal small vessel pathology is associated with disease burden in multiple sclerosis.
Multiple Sclerosis Journal 30.7 (Jun. 2024). DOI
Abstract
Background: Alterations of the superficial retinal vasculature are commonly observed in multiple sclerosis (MS) and can be visualized through optical coherence tomography angiography (OCTA).
Objectives: This study aimed to examine changes in the retinal vasculature during MS and to integrate findings into current concepts of the underlying pathology.
Methods: In this cross-sectional study, including 259 relapsing–remitting MS patients and 78 healthy controls, we analyzed OCTAs using deep-learning-based segmentation algorithm tools.
Results: We identified a loss of small-sized vessels (diameter < 10 µm) in the superficial vascular complex in all MS eyes, irrespective of their optic neuritis (ON) history. This alteration was associated with MS disease burden and appears independent of retinal ganglion cell loss. In contrast, an observed reduction of medium-sized vessels (diameter 10–20 µm) was specific to eyes with a history of ON and was closely linked to ganglion cell atrophy.
Conclusion: These findings suggest distinct atrophy patterns in retinal vessels in patients with MS. Further studies are necessary to investigate retinal vessel alterations and their underlying pathology in MS.
MCML Authors
Integration of variant annotations using deep set networks boosts rare variant association testing.
Nature Genetics 56 (Sep. 2024). DOI
Abstract
Rare genetic variants can have strong effects on phenotypes, yet accounting for rare variants in genetic analyses is statistically challenging due to the limited number of allele carriers and the burden of multiple testing. While rich variant annotations promise to enable well-powered rare variant association tests, methods integrating variant annotations in a data-driven manner are lacking. Here we propose deep rare variant association testing (DeepRVAT), a model based on set neural networks that learns a trait-agnostic gene impairment score from rare variant annotations and phenotypes, enabling both gene discovery and trait prediction. On 34 quantitative and 63 binary traits, using whole-exome-sequencing data from UK Biobank, we find that DeepRVAT yields substantial gains in gene discoveries and improved detection of individuals at high genetic risk. Finally, we demonstrate how DeepRVAT enables calibrated and computationally efficient rare variant tests at biobank scale, aiding the discovery of genetic risk factors for human disease traits.
MCML Authors
Causal machine learning for predicting treatment outcomes.
Nature Medicine 30 (Apr. 2024). DOI
Abstract
Causal machine learning (ML) offers flexible, data-driven methods for predicting treatment outcomes including efficacy and toxicity, thereby supporting the assessment and safety of drugs. A key benefit of causal ML is that it allows for estimating individualized treatment effects, so that clinical decision-making can be personalized to individual patient profiles. Causal ML can be used in combination with both clinical trial data and real-world data, such as clinical registries and electronic health records, but caution is needed to avoid biased or incorrect predictions. In this Perspective, we discuss the benefits of causal ML (relative to traditional statistical or ML approaches) and outline the key components and steps. Finally, we provide recommendations for the reliable use of causal ML and effective translation into the clinic.
MCML Authors
Artificial Intelligence in Management
Artificial Intelligence in Management
Artificial Intelligence in Management
Artificial Intelligence in Management
Artificial Intelligence in Management
Transformers in single-cell omics: a review and new perspectives.
Nature Methods 21 (Aug. 2024). DOI
Abstract
Recent efforts to construct reference maps of cellular phenotypes have expanded the volume and diversity of single-cell omics data, providing an unprecedented resource for studying cell properties. Despite the availability of rich datasets and their continued growth, current single-cell models are unable to fully capitalize on the information they contain. Transformers have become the architecture of choice for foundation models in other domains owing to their ability to generalize to heterogeneous, large-scale datasets. Thus, the question arises of whether transformers could set off a similar shift in the field of single-cell modeling. Here we first describe the transformer architecture and its single-cell adaptations and then present a comprehensive review of the existing applications of transformers in single-cell analysis and critically discuss their future potential for single-cell biology. By studying limitations and technical challenges, we aim to provide a structured outlook for future research directions at the intersection of machine learning and single-cell biology.
MCML Authors
Ethics in Systems Design and Machine Learning
Probe set selection for targeted spatial transcriptomics.
Nature Methods 21 (Dec. 2024). DOI
Abstract
Targeted spatial transcriptomic methods capture the topology of cell types and states in tissues at single-cell and subcellular resolution by measuring the expression of a predefined set of genes. The selection of an optimal set of probed genes is crucial for capturing the spatial signals present in a tissue. This requires selecting the most informative, yet minimal, set of genes to profile (gene set selection) for which it is possible to build probes (probe design). However, current selections often rely on marker genes, precluding them from detecting continuous spatial signals or new states. We present Spapros, an end-to-end probe set selection pipeline that optimizes both gene set specificity for cell type identification and within-cell type expression variation to resolve spatially distinct populations while considering prior knowledge as well as probe design and expression constraints. We evaluated Spapros and show that it outperforms other selection approaches in both cell type recovery and recovering expression variation beyond cell types. Furthermore, we used Spapros to design a single-cell resolution in situ hybridization on tissues (SCRINSHOT) experiment of adult lung tissue to demonstrate how probes selected with Spapros identify cell types of interest and detect spatial variation even within cell types.
MCML Authors
Deep generative modeling of transcriptional dynamics for RNA velocity analysis in single cells.
Nature Methods 21 (Jan. 2024). DOI
Abstract
RNA velocity has been rapidly adopted to guide interpretation of transcriptional dynamics in snapshot single-cell data; however, current approaches for estimating RNA velocity lack effective strategies for quantifying uncertainty and determining the overall applicability to the system of interest. Here, we present veloVI (velocity variational inference), a deep generative modeling framework for estimating RNA velocity. veloVI learns a gene-specific dynamical model of RNA metabolism and provides a transcriptome-wide quantification of velocity uncertainty. We show that veloVI compares favorably to previous approaches with respect to goodness of fit, consistency across transcriptionally similar cells and stability across preprocessing pipelines for quantifying RNA abundance. Further, we demonstrate that veloVI’s posterior velocity uncertainty can be used to assess whether velocity analysis is appropriate for a given dataset. Finally, we highlight veloVI as a flexible framework for modeling transcriptional dynamics by adapting the underlying dynamical model to use time-dependent transcription rates.
MCML Authors
Multi-vehicle trajectory prediction and control at intersections using state and intention information.
Neurocomputing 574 (Jan. 2024). DOI GitHub
Abstract
Traditional deep learning approaches for prediction of future trajectory of multiple road agents rely on knowing information about their past trajectory. In contrast, this work utilizes information of only the current state and intended direction to predict the future trajectory of multiple vehicles at intersections. Incorporating intention information has two distinct advantages: (1) It allows to not just predict the future trajectory but also control the multiple vehicles. (2) By manipulating the intention, the interaction among the vehicles is adapted accordingly to achieve desired behavior. Both these advantages would otherwise not be possible using only past trajectory information Our model utilizes message passing of information between the vehicle nodes for a more holistic overview of the environment, resulting in better trajectory prediction and control of the vehicles. This work also provides a thorough investigation and discussion into the disparity between offline and online metrics for the task of multi-agent control. We particularly show why conducting only offline evaluation would not suffice, thereby necessitating online evaluation. We demonstrate the superiority of utilizing intention information rather than past trajectory in online scenarios. Lastly, we show the capability of our method in adapting to different domains through experiments conducted on two distinct simulation platforms i.e. SUMO and CARLA.
MCML Authors
Energy Expenditure Prediction in Preschool Children: A Machine Learning Approach Using Accelerometry and External Validation.
Physiological Measurement 45.9 (Sep. 2024). DOI
Abstract
Objective. This study aimed to develop convolutional neural networks (CNNs) models to predict the energy expenditure (EE) of children from raw accelerometer data. Additionally, this study sought to external validation of the CNN models in addition to the linear regression (LM), random forest (RF), and full connected neural network (FcNN) models published in Steenbock et al (2019 J. Meas. Phys. Behav. 2 94–102). Approach. Included in this study were 41 German children (3.0–6.99 years) for the training and internal validation who were equipped with GENEActiv, GT3X+, and activPAL accelerometers. The external validation dataset consisted of 39 Canadian children (3.0–5.99 years) that were equipped with OPAL, GT9X, GENEActiv, and GT3X+ accelerometers. EE was recorded simultaneously in both datasets using a portable metabolic unit. The protocols consisted of a semi-structured activities ranging from low to high intensities. The root mean square error (RMSE) values were calculated and used to evaluate model performances. Main results. (1) The CNNs outperformed the LM (13.17%–23.81% lower mean RMSE values), FcNN (8.13%–27.27% lower RMSE values) and the RF models (3.59%–18.84% lower RMSE values) in the internal dataset. (2) In contrast, it was found that when applied to the external Canadian dataset, the CNN models had consistently higher RMSE values compared to the LM, FcNN, and RF. Significance. Although CNNs can enhance EE prediction accuracy, their ability to generalize to new datasets and accelerometer brands/models, is more limited compared to LM, RF, and FcNN models.
MCML Authors
Statistical Learning and Data Science
Raising awareness of uncertain choices in empirical data analysis: A teaching concept toward replicable research practices.
PLOS Computational Biology 20.3 (2024). DOI
Abstract
Throughout their education and when reading the scientific literature, students may get the impression that there is a unique and correct analysis strategy for every data analysis task and that this analysis strategy will always yield a significant and noteworthy result. This expectation conflicts with a growing realization that there is a multiplicity of possible analysis strategies in empirical research, which will lead to overoptimism and nonreplicable research findings if it is combined with result-dependent selective reporting. Here, we argue that students are often ill-equipped for real-world data analysis tasks and unprepared for the dangers of selectively reporting the most promising results. We present a seminar course intended for advanced undergraduates and beginning graduate students of data analysis fields such as statistics, data science, or bioinformatics that aims to increase the awareness of uncertain choices in the analysis of empirical data and present ways to deal with these choices through theoretical modules and practical hands-on sessions.
MCML Authors
Biometry in Molecular Medicine
Biometry in Molecular Medicine
Simulation Studies for Methodological Research in Psychology: A Standardized Template for Planning, Preregistration, and Reporting.
Psychological Methods Advance online publication (2024). DOI
Abstract
Simulation studies are widely used for evaluating the performance of statistical methods in psychology. However, the quality of simulation studies can vary widely in terms of their design, execution, and reporting. In order to assess the quality of typical simulation studies in psychology, we reviewed 321 articles published in Psychological Methods, Behavior Research Methods, and Multivariate Behavioral Research in 2021 and 2022, among which 100/321 = 31.2% report a simulation study. We find that many articles do not provide complete and transparent information about key aspects of the study, such as justifications for the number of simulation repetitions, Monte Carlo uncertainty estimates, or code and data to reproduce the simulation studies. To address this problem, we provide a summary of the ADEMP (aims, data-generating mechanism, estimands and other targets, methods, performance measures) design and reporting framework from Morris et al. (2019) adapted to simulation studies in psychology. Based on this framework, we provide ADEMP-PreReg, a step-by-step template for researchers to use when designing, potentially preregistering, and reporting their simulation studies. We give formulae for estimating common performance measures, their Monte Carlo standard errors, and for calculating the number of simulation repetitions to achieve a desired Monte Carlo standard error. Finally, we give a detailed tutorial on how to apply the ADEMP framework in practice using an example simulation study on the evaluation of methods for the analysis of pre–post measurement experiments. (PsycInfo Database Record (c) 2024 APA, all rights reserved)
MCML Authors
Biometry in Molecular Medicine
MRI-based ventilation and perfusion imaging to predict radiation-induced pneumonitis in lung tumor patients at a 0.35T MR-Linac.
Radiotherapy and Oncology (Aug. 2024). DOI
Abstract
Radiation-induced pneumonitis (RP), diagnosed 6–12 weeks after treatment, is a complication of lung tumor radiotherapy. So far, clinical and dosimetric parameters have not been reliable in predicting RP. We propose using non-contrast enhanced magnetic resonance imaging (MRI) based functional parameters acquired over the treatment course for patient stratification for improved follow-up.
MCML Authors
A satellite imagery-driven framework for rapid resource allocation in flood scenarios to enhance loss and damage fund effectiveness.
Scientific Reports 14.19290 (Aug. 2024). DOI
Abstract
The impact of climate change and urbanization has increased the risk of flooding. During the UN Climate Change Conference 28 (COP 28), an agreement was reached to establish “The Loss and Damage Fund” to assist low-income countries impacted by climate change. However, allocating the resources required for post-flood reconstruction and reimbursement is challenging due to the limited availability of data and the absence of a comprehensive tool. Here, we propose a novel resource allocation framework based on remote sensing and geospatial data near the flood peak, such as buildings and population. The quantification of resource distribution utilizes an exposure index for each municipality, which interacts with various drivers, including flood hazard drivers, buildings exposure, and population exposure. The proposed framework asses the flood extension using pre- and post-flood Sentinel-1 Synthetic Aperture Radar (SAR) data. To demonstrate the effectiveness of this framework, an analysis was conducted on the flood that occurred in the Thessaly region of Greece in September 2023. The study revealed that the municipality of Palamas has the highest need for resource allocation, with an exposure index rating of 5/8. Any government can use this framework for rapid decision-making and to expedite post-flood recovery.
MCML Authors
Explainable AI improves task performance in human–AI collaboration.
Scientific Reports 14.31150 (Dec. 2024). DOI
Abstract
Artificial intelligence (AI) provides considerable opportunities to assist human work. However, one crucial challenge of human-AI collaboration is that many AI algorithms operate in a black-box manner where the way how the AI makes predictions remains opaque. This makes it difficult for humans to validate a prediction made by AI against their own domain knowledge. For this reason, we hypothesize that augmenting humans with explainable AI as a decision aid improves task performance in human-AI collaboration. To test this hypothesis, we analyze the effect of augmenting domain experts with explainable AI in the form of visual heatmaps. We then compare participants that were either supported by (a) black-box AI or (b) explainable AI, where the latter supports them to follow AI predictions when the AI is accurate or overrule the AI when the AI predictions are wrong. We conducted two preregistered experiments with representative, real-world visual inspection tasks from manufacturing and medicine. The first experiment was conducted with factory workers from an electronics factory, who performed N=9,600 assessments of whether electronic products have defects. The second experiment was conducted with radiologists, who performed N=5,650 assessments of chest X-ray images to identify lung lesions. The results of our experiments with domain experts performing real-world tasks show that task performance improves when participants are supported by explainable AI instead of black-box AI. For example, in the manufacturing setting, we find that augmenting participants with explainable AI (as opposed to black-box AI) leads to a five-fold decrease in the median error rate of human decisions, which gives a significant improvement in task performance.
MCML Authors
Artificial Intelligence in Management
Artificial Intelligence in Management
Consensus-Based Optimization Methods Converge Globally.
SIAM Journal on Optimization 34.3 (Jul. 2024). DOI
Abstract
In this paper we study consensus-based optimization (CBO), which is a multiagent metaheuristic derivative-free optimization method that can globally minimize nonconvex nonsmooth functions and is amenable to theoretical analysis. Based on an experimentally supported intuition that, on average, CBO performs a gradient descent of the squared Euclidean distance to the global minimizer, we devise a novel technique for proving the convergence to the global minimizer in mean-field law for a rich class of objective functions. The result unveils internal mechanisms of CBO that are responsible for the success of the method. In particular, we prove that CBO performs a convexification of a large class of optimization problems as the number of optimizing agents goes to infinity. Furthermore, we improve prior analyses by requiring mild assumptions about the initialization of the method and by covering objectives that are merely locally Lipschitz continuous. As a core component of this analysis, we establish a quantitative nonasymptotic Laplace principle, which may be of independent interest. From the result of CBO convergence in mean-field law, it becomes apparent that the hardness of any global optimization problem is necessarily encoded in the rate of the mean-field approximation, for which we provide a novel probabilistic quantitative estimate. The combination of these results allows us to obtain probabilistic global convergence guarantees of the numerical CBO method.
MCML Authors
Heterogeneous treatment effect estimation for observational data using model-based forests.
Statistical Methods in Medical Research 33.3 (Mar. 2024). DOI
Abstract
The estimation of heterogeneous treatment effects has attracted considerable interest in many disciplines, most prominently in medicine and economics. Contemporary research has so far primarily focused on continuous and binary responses where heterogeneous treatment effects are traditionally estimated by a linear model, which allows the estimation of constant or heterogeneous effects even under certain model misspecifications. More complex models for survival, count, or ordinal outcomes require stricter assumptions to reliably estimate the treatment effect. Most importantly, the noncollapsibility issue necessitates the joint estimation of treatment and prognostic effects. Model-based forests allow simultaneous estimation of covariate-dependent treatment and prognostic effects, but only for randomized trials. In this paper, we propose modifications to model-based forests to address the confounding issue in observational data. In particular, we evaluate an orthogonalization strategy originally proposed by Robinson (1988, Econometrica) in the context of model-based forests targeting heterogeneous treatment effect estimation in generalized linear models and transformation models. We found that this strategy reduces confounding effects in a simulated study with various outcome distributions. We demonstrate the practical aspects of heterogeneous treatment effect estimation for survival and ordinal outcomes by an assessment of the potentially heterogeneous effect of Riluzole on the progress of Amyotrophic Lateral Sclerosis.
MCML Authors
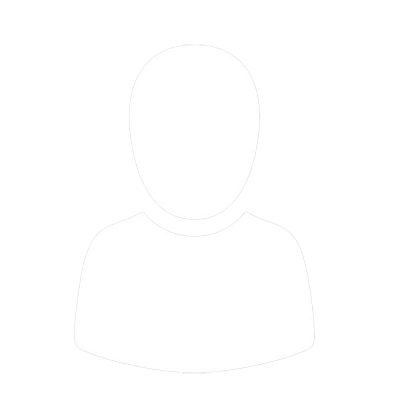
Susanne Dandl
Dr.
* Former Member
Machine Learning Consulting Unit (MLCU)
Privacy-Preserving and Lossless Distributed Estimation of High-Dimensional Generalized Additive Mixed Models.
Statistics and Computing 34.31 (Feb. 2024). DOI
Abstract
Various privacy-preserving frameworks that respect the individual’s privacy in the analysis of data have been developed in recent years. However, available model classes such as simple statistics or generalized linear models lack the flexibility required for a good approximation of the underlying data-generating process in practice. In this paper, we propose an algorithm for a distributed, privacy-preserving, and lossless estimation of generalized additive mixed models (GAMM) using component-wise gradient boosting (CWB). Making use of CWB allows us to reframe the GAMM estimation as a distributed fitting of base learners using the $L_2$-loss. In order to account for the heterogeneity of different data location sites, we propose a distributed version of a row-wise tensor product that allows the computation of site-specific (smooth) effects. Our adaption of CWB preserves all the important properties of the original algorithm, such as an unbiased feature selection and the feasibility to fit models in high-dimensional feature spaces, and yields equivalent model estimates as CWB on pooled data. Next to a derivation of the equivalence of both algorithms, we also showcase the efficacy of our algorithm on a distributed heart disease data set and compare it with state-of-the-art methods.
MCML Authors
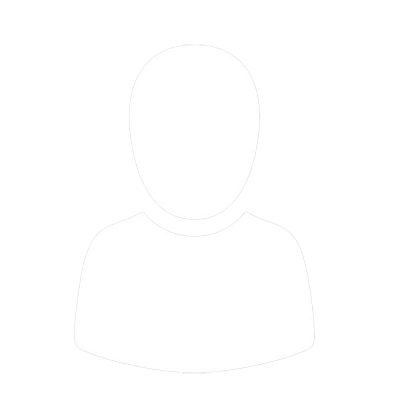
Daniel Schalk
Dr.
* Former Member
Statistical Learning and Data Science
Statistics, Data Science and Machine Learning
Deep learning for autosegmentation for radiotherapy treatment planning: State-of-the-art and novel perspectives.
Strahlentherapie und Onkologie 201 (Aug. 2024). DOI GitHub
Abstract
The rapid development of artificial intelligence (AI) has gained importance, with many tools already entering our daily lives. The medical field of radiation oncology is also subject to this development, with AI entering all steps of the patient journey. In this review article, we summarize contemporary AI techniques and explore the clinical applications of AI-based automated segmentation models in radiotherapy planning, focusing on delineation of organs at risk (OARs), the gross tumor volume (GTV), and the clinical target volume (CTV). Emphasizing the need for precise and individualized plans, we review various commercial and freeware segmentation tools and also state-of-the-art approaches. Through our own findings and based on the literature, we demonstrate improved efficiency and consistency as well as time savings in different clinical scenarios. Despite challenges in clinical implementation such as domain shifts, the potential benefits for personalized treatment planning are substantial. The integration of mathematical tumor growth models and AI-based tumor detection further enhances the possibilities for refining target volumes. As advancements continue, the prospect of one-stop-shop segmentation and radiotherapy planning represents an exciting frontier in radiotherapy, potentially enabling fast treatment with enhanced precision and individualization.
MCML Authors
The impact of intelligent decision-support systems on humans' ethical decision-making: A systematic literature review and an integrated framework.
Technological Forecasting and Social Change 204.123403 (Jul. 2024). DOI
Abstract
With the rise and public accessibility of AI-enabled decision-support systems, individuals outsource increasingly more of their decisions, even those that carry ethical dimensions. Considering this trend, scholars have highlighted that uncritical deference to these systems would be problematic and consequently called for investigations of the impact of pertinent technology on humans’ ethical decision-making. To this end, this article conducts a systematic review of existing scholarship and derives an integrated framework that demonstrates how intelligent decision-support systems (IDSSs) shape humans’ ethical decision-making. In particular, we identify resulting consequences on an individual level (i.e., deliberation enhancement, motivation enhancement, autonomy enhancement and action enhancement) and on a societal level (i.e., moral deskilling, restricted moral progress and moral responsibility gaps). We carve out two distinct methods/operation types (i.e., process-oriented and outcome-oriented navigation) that decision-support systems can deploy and postulate that these determine to what extent the previously stated consequences materialize. Overall, this study holds important theoretical and practical implications by establishing clarity in the conceptions, underlying mechanisms and (directions of) influences that can be expected when using particular IDSSs for ethical decisions.
MCML Authors
TRIPOD+AI statement: updated guidance for reporting clinical prediction models that use regression or machine learning methods.
The BMJ 385.e078378 (Apr. 2024). DOI
Abstract
The TRIPOD (Transparent Reporting of a multivariable prediction model for Individual Prognosis Or Diagnosis) statement was published in 2015 to provide the minimum reporting recommendations for studies developing or evaluating the performance of a prediction model. Methodological advances in the field of prediction have since included the widespread use of artificial intelligence (AI) powered by machine learning methods to develop prediction models. An update to the TRIPOD statement is thus needed. TRIPOD+AI provides harmonised guidance for reporting prediction model studies, irrespective of whether regression modelling or machine learning methods have been used. The new checklist supersedes the TRIPOD 2015 checklist, which should no longer be used. This article describes the development of TRIPOD+AI and presents the expanded 27 item checklist with more detailed explanation of each reporting recommendation, and the TRIPOD+AI for Abstracts checklist. TRIPOD+AI aims to promote the complete, accurate, and transparent reporting of studies that develop a prediction model or evaluate its performance. Complete reporting will facilitate study appraisal, model evaluation, and model implementation.
MCML Authors
Biometry in Molecular Medicine
Understanding the Omicron Variant Impact in Healthcare Workers: Insights from the Prospective COVID-19 Post-Immunization Serological Cohort in Munich (KoCo-Impf) on Risk Factors for Breakthrough and Reinfections.
Viruses 16.10 (Sep. 2024). DOI
Abstract
This study analyzes immune responses to SARS-CoV-2 vaccination and infection, including asymptomatic cases, focusing on infection risks during the Omicron wave, particularly among high-risk healthcare workers. In the KoCo-Impf study, we monitored 6088 vaccinated participants in Munich aged 18 and above. From 13 May to 31 July 2022, 2351 participants were follow-uped. Logistic regression models evaluated primary, secondary, and breakthrough infections (BTIs). Roche Elecsys® Anti-SARS-CoV-2 assays detected prior infections (via anti-Nucleocapsid antibodies) and assessed vaccination/infection impact (via anti-Spike antibodies) using dried blood spots. Our findings revealed an anti-Nucleocapsid seroprevalence of 44.1%. BTIs occurred in 38.8% of participants, with reinfections in 48.0%. Follow-up participation was inversely associated with current smoking and non-vaccination, while significantly increasing with age and receipt of three vaccine doses. Larger household sizes and younger age increased infection risks, whereas multiple vaccinations and older age reduced them. Household size and specific institutional subgroups were risk factors for BTIs. The anti-Nucleocapsid value prior to the second infection was significantly associated with reinfection risk. Institutional subgroups influenced all models, underscoring the importance of tailored outbreak responses. The KoCo-Impf study underscores the importance of vaccination, demographic factors, and institutional settings in understanding SARS-CoV-2 infection risks during the Omicron wave.
MCML Authors
Statistical Consulting Unit (StaBLab)
Large-scale flood modeling and forecasting with FloodCast.
Water Research 264 (Oct. 2024). DOI
Abstract
Large-scale hydrodynamic models generally rely on fixed-resolution spatial grids and model parameters as well as incurring a high computational cost. This limits their ability to accurately forecast flood crests and issue time-critical hazard warnings. In this work, we build a fast, stable, accurate, resolution-invariant, and geometry-adaptive flood modeling and forecasting framework that can perform at large scales, namely FloodCast. The framework comprises two main modules: multi-satellite observation and hydrodynamic modeling. In the multi-satellite observation module, a real-time unsupervised change detection method and a rainfall processing and analysis tool are proposed to harness the full potential of multi-satellite observations in large-scale flood prediction. In the hydrodynamic modeling module, a geometry-adaptive physics-informed neural solver (GeoPINS) is introduced, benefiting from the absence of a requirement for training data in physics-informed neural networks (PINNs) and featuring a fast, accurate, and resolution-invariant architecture with Fourier neural operators. To adapt to complex river geometries, we reformulate PINNs in a geometry-adaptive space. GeoPINS demonstrates impressive performance on popular partial differential equations across regular and irregular domains. Building upon GeoPINS, we propose a sequence-to-sequence GeoPINS model to handle long-term temporal series and extensive spatial domains in large-scale flood modeling. This model employs sequence-to-sequence learning and hard-encoding of boundary conditions. Next, we establish a benchmark dataset in the 2022 Pakistan flood using a widely accepted finite difference numerical solution to assess various flood simulation methods. Finally, we validate the model in three dimensions - flood inundation range, depth, and transferability of spatiotemporal downscaling - utilizing SAR-based flood data, traditional hydrodynamic benchmarks, and concurrent optical remote sensing images. Traditional hydrodynamics and sequence-to-sequence GeoPINS exhibit exceptional agreement during high water levels, while comparative assessments with SAR-based flood depth data show that sequence-to-sequence GeoPINS outperforms traditional hydrodynamics, with smaller simulation errors. The experimental results for the 2022 Pakistan flood demonstrate that the proposed method enables high-precision, large-scale flood modeling with an average MAPE of 14.93% and an average Mean Absolute Error (MAE) of 0.0610 m for 14-day water depth simulations while facilitating real-time flood hazard forecasting using reliable precipitation data.
MCML Authors
01.01.2024
Related
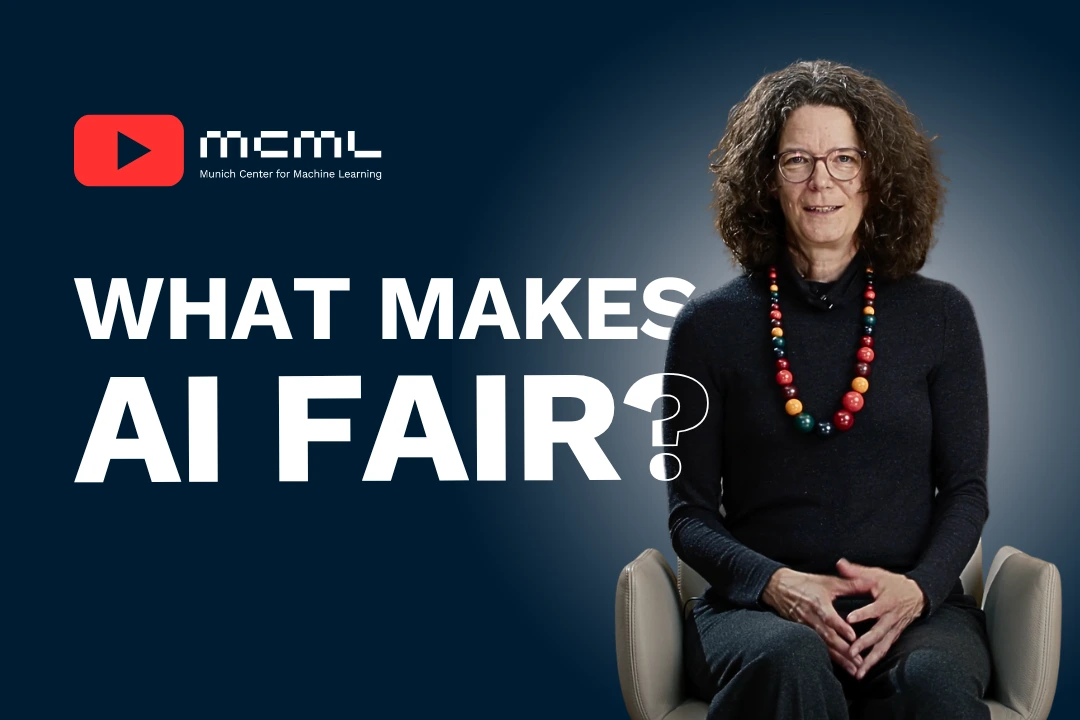
11.06.2025
Better Data, Smarter AI: Why Quality Matters – With Frauke Kreuter
In our new research film, Frauke Kreuter explains how data quality shapes fair, reliable, and socially responsible AI systems.
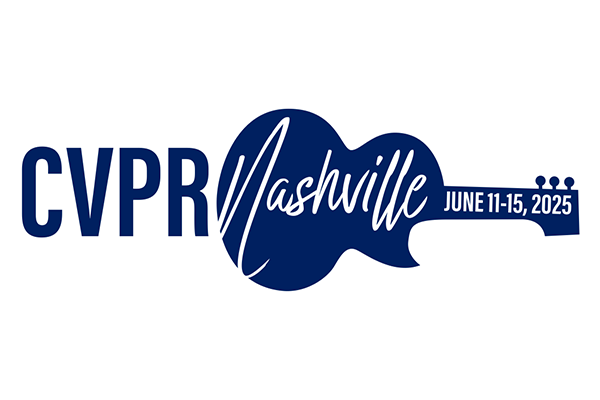
10.06.2025
MCML Researchers With 29 Papers at CVPR 2025
IEEE/CVF Conference on Computer Vision and Pattern Recognition (CVPR 2025). Nashville, TN, USA, 11.06.2025 - 15.06.2025
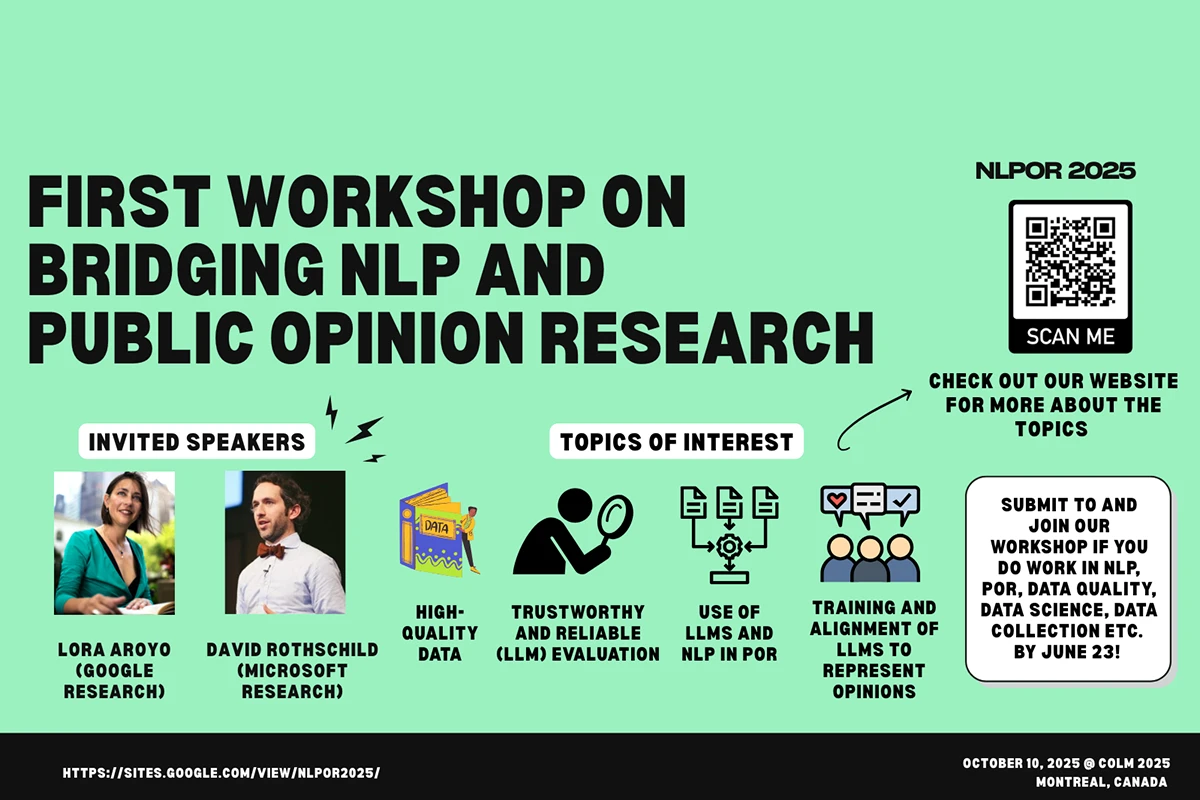
20.05.2025
Call for Papers for NLPOR - First Workshop on Bridging NLP and Public Opinion Research
This interdisciplinary workshop explores the powerful connections between Natural Language Processing (NLP) and Public Opinion Research (POR).
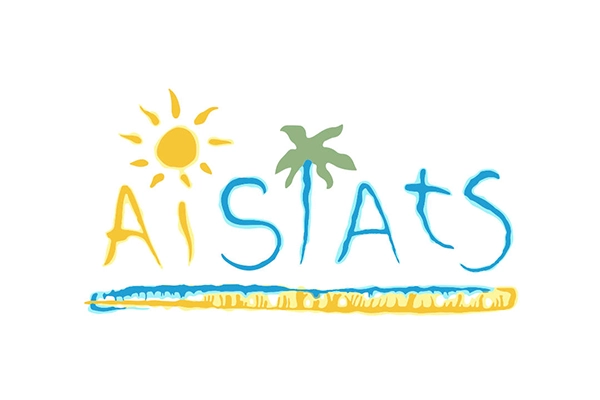
02.05.2025
MCML Researchers With Five Papers at AISTATS 2025
28th International Conference on Artificial Intelligence and Statistics (AISTATS 2025). Mai Khao, Thailand, 29.04.2025 - 05.05.2024