08
Mar
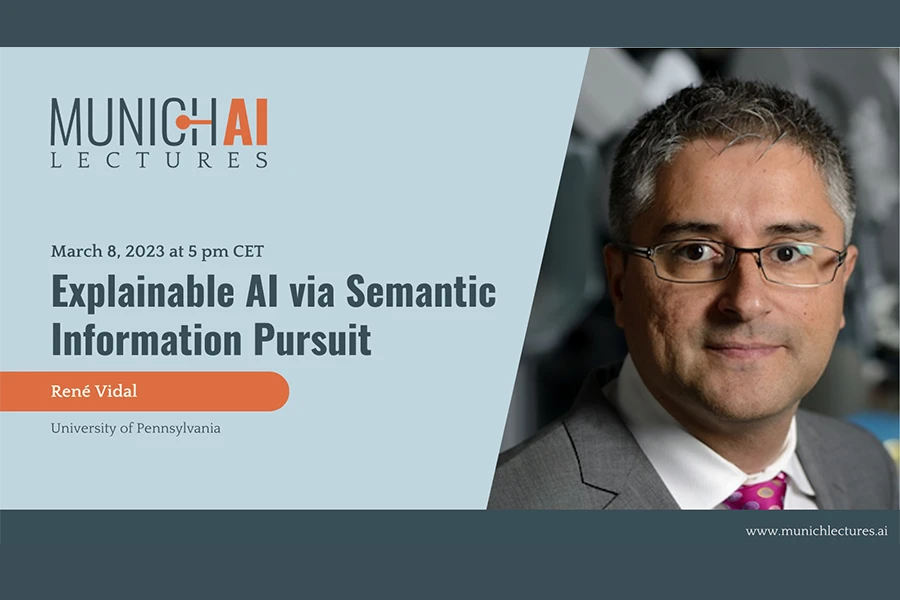
Explainable AI via Semantic Information Pursuit
René Vidal, John Hopkins University
08.03.2023
5:00 pm - 6:30 pm
Livestream on YouTube
There is a significant interest in developing ML algorithms whose final predictions can be explained in terms understandable to a human. To address this challenge, we develop a method for constructing high performance ML algorithms which are explainable by design.
Related
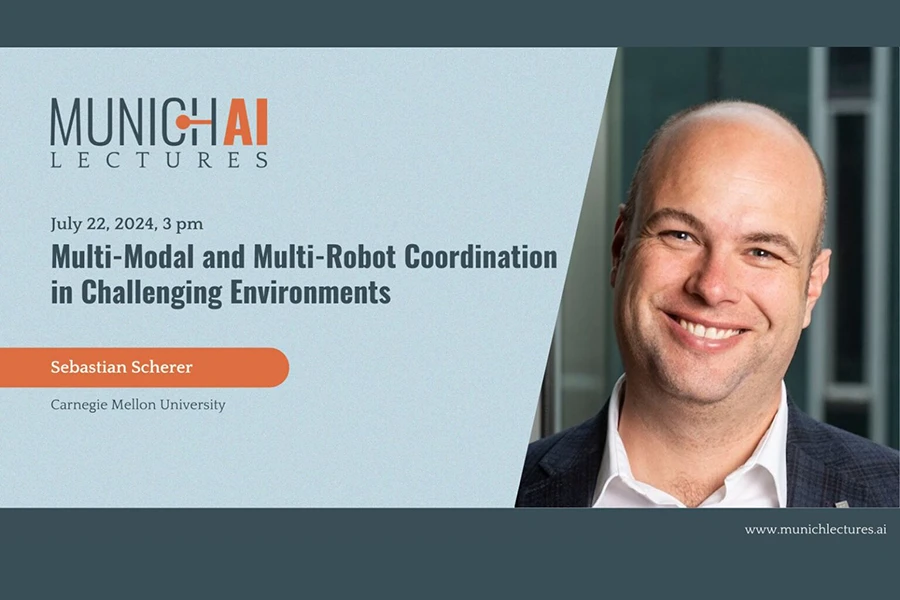
Munich AI Lectures • 22.07.2024 • TUM Garching Campus, FMI Building, Hörsaal 2 (00.04.011), Boltzmannstr. 3, 85748 Garching bei München or online via Livestream
Multi-Modal and Multi-Robot Coordination in Challenging Environments
Munich AI Lecture with Sebastian Scherer from CMU outlining some of their approaches progress, and results on multi-modal sensing.
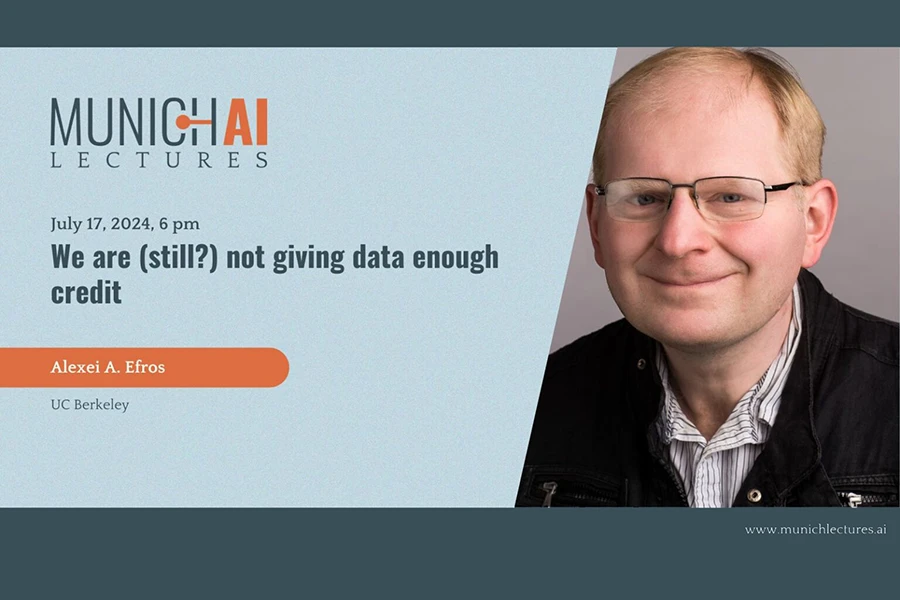
Munich AI Lectures • 17.07.2024 • Bayerische Akademie der Wissenschaften, Plenarsaal, 1. Stock, Alfons-Goppel-Straße 11, 80539 München
We are (still?) not giving data enough credit
Munich AI Highlight Lecture with Alexei A. Efros from UC Berkeley, emphasizing the critical role of data in Computer Vision with historical examples and recent work.
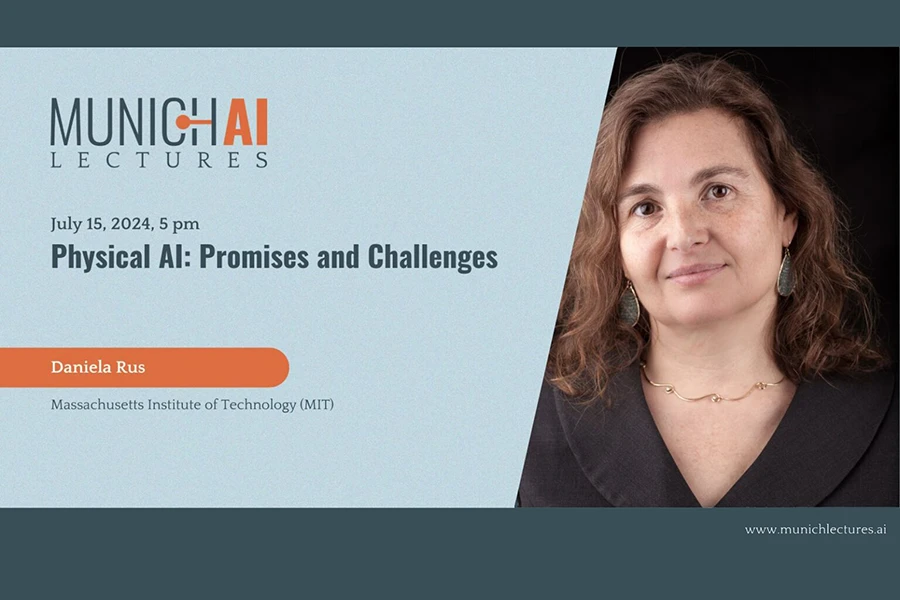
Munich AI Lectures • 15.07.2024 • TU Munich, Institute for Advanced Study, Auditorium (Ground floor), Lichtenbergstraße 2a, 85748 Garching
Physical AI: Promises and Challenges
Munich AI Lecture with Daniela Rus from MIT discussing recent developments in ML and robotics.
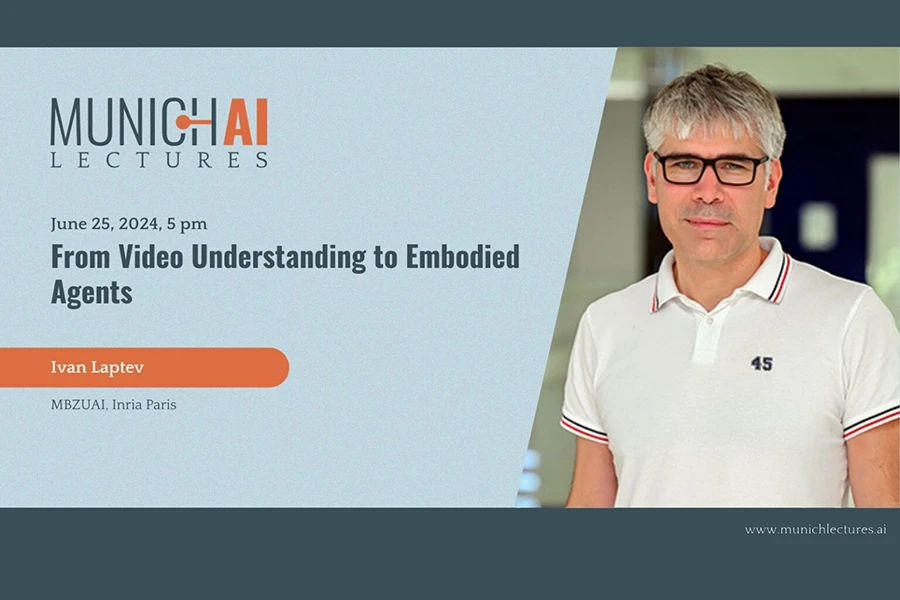
Munich AI Lectures • 25.06.2024 • TUM Campus Munich, Room 0790, Arcisstraße 21, 80333 München
From Video Understanding to Embodied Agents
The MCML invites you to the Munich AI Lectures with Ivan Laptev discussing AI models that make reliable predictions from explanatory videos.
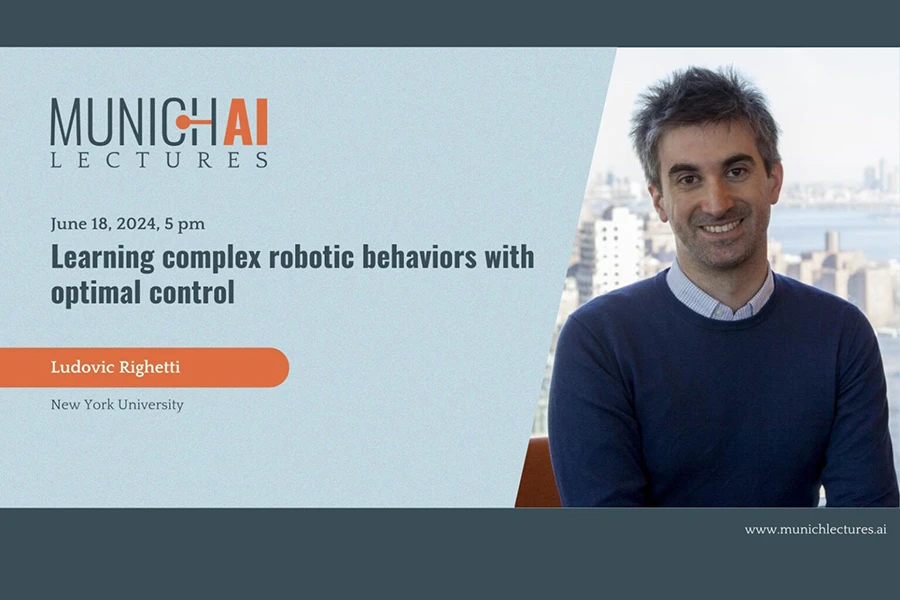
Munich AI Lectures • 18.06.2024 • TUM, Arcisstr. 21, 80333 Munich, Room 0790 (ground floor)
Learning complex robotic behaviors with optimal control
Munich AI Lecture with Ludovic Righetti from NYU presenting their recent work with a particular eye towards unifying learning and numerical optimal control.