26.04.2023
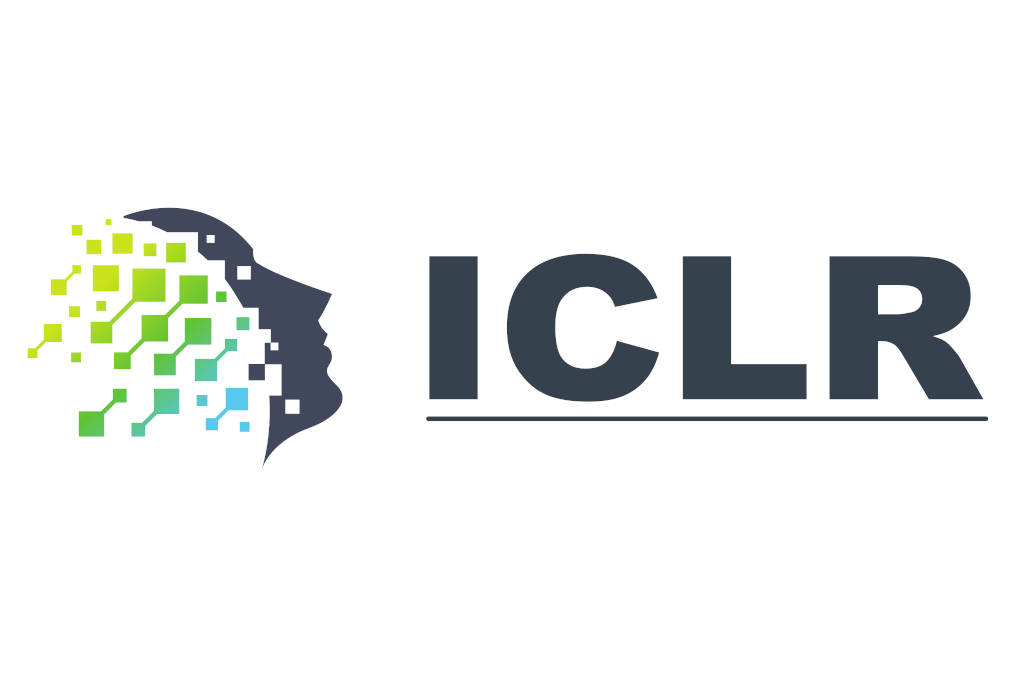
MCML Researchers With Three Papers at ICLR 2023
11th International Conference on Learning Representations (ICLR 2023). Vienna, Austria, 01.05.2023–05.05.2023
We are happy to announce that MCML researchers are represented with three papers at ICLR 2023. Congrats to our researchers!
Main Track (3 papers)
Estimating individual treatment effects under unobserved confounding using binary instruments.
ICLR 2023 - 11th International Conference on Learning Representations. Kigali, Rwanda, May 01-05, 2023. URL
Abstract
Estimating conditional average treatment effects (CATEs) from observational data is relevant in many fields such as personalized medicine. However, in practice, the treatment assignment is usually confounded by unobserved variables and thus introduces bias. A remedy to remove the bias is the use of instrumental variables (IVs). Such settings are widespread in medicine (e.g., trials where the treatment assignment is used as binary IV). In this paper, we propose a novel, multiply robust machine learning framework, called MRIV, for estimating CATEs using binary IVs and thus yield an unbiased CATE estimator. Different from previous work for binary IVs, our framework estimates the CATE directly via a pseudo outcome regression. (1)~We provide a theoretical analysis where we show that our framework yields multiple robust convergence rates: our CATE estimator achieves fast convergence even if several nuisance estimators converge slowly. (2)~We further show that our framework asymptotically outperforms state-of-the-art plug-in IV methods for CATE estimation, in the sense that it achieves a faster rate of convergence if the CATE is smoother than the individual outcome surfaces. (3)~We build upon our theoretical results and propose a tailored deep neural network architecture called MRIV-Net for CATE estimation using binary IVs. Across various computational experiments, we demonstrate empirically that our MRIV-Net achieves state-of-the-art performance. To the best of our knowledge, our MRIV is the first multiply robust machine learning framework tailored to estimating CATEs in the binary IV setting.
MCML Authors
Artificial Intelligence in Management
Artificial Intelligence in Management
Unveiling the Sampling Density in Non-Uniform Geometric Graphs.
ICLR 2023 - 11th International Conference on Learning Representations. Kigali, Rwanda, May 01-05, 2023. URL
Abstract
A powerful framework for studying graphs is to consider them as geometric graphs: nodes are randomly sampled from an underlying metric space, and any pair of nodes is connected if their distance is less than a specified neighborhood radius. Currently, the literature mostly focuses on uniform sampling and constant neighborhood radius. However, real-world graphs are likely to be better represented by a model in which the sampling density and the neighborhood radius can both vary over the latent space. For instance, in a social network communities can be modeled as densely sampled areas, and hubs as nodes with larger neighborhood radius. In this work, we first perform a rigorous mathematical analysis of this (more general) class of models, including derivations of the resulting graph shift operators. The key insight is that graph shift operators should be corrected in order to avoid potential distortions introduced by the non-uniform sampling. Then, we develop methods to estimate the unknown sampling density in a self-supervised fashion. Finally, we present exemplary applications in which the learnt density is used to 1) correct the graph shift operator and improve performance on a variety of tasks, 2) improve pooling, and 3) extract knowledge from networks. Our experimental findings support our theory and provide strong evidence for our model.
MCML Authors
Mathematical Foundations of Artificial Intelligence
Mathematical Foundations of Artificial Intelligence
Approximate Bayesian Inference with Stein Functional Variational Gradient Descent.
ICLR 2023 - 11th International Conference on Learning Representations. Kigali, Rwanda, May 01-05, 2023. URL
Abstract
We propose a general-purpose variational algorithm that forms a natural analogue of Stein variational gradient descent (SVGD) in function space. While SVGD successively updates a set of particles to match a target density, the method introduced here of Stein functional variational gradient descent (SFVGD) updates a set of particle functions to match a target stochastic process (SP). The update step is found by minimizing the functional derivative of the Kullback-Leibler divergence between SPs. SFVGD can either be used to train Bayesian neural networks (BNNs) or for ensemble gradient boosting. We show the efficacy of training BNNs with SFVGD on various real-world datasets.
MCML Authors
Statistical Learning and Data Science
Statistical Learning and Data Science
Statistics, Data Science and Machine Learning
26.04.2023
Related
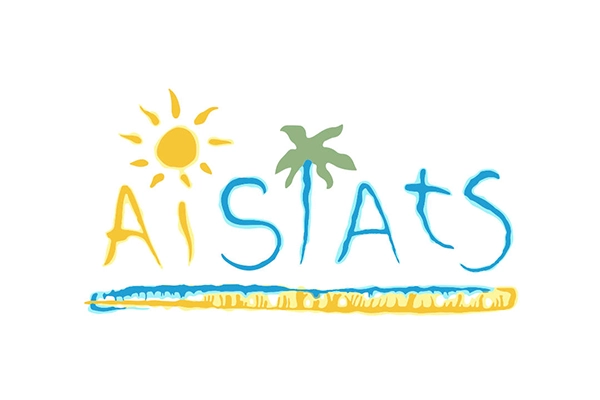
02.05.2025
MCML Researchers With Five Papers at AISTATS 2025
28th International Conference on Artificial Intelligence and Statistics (AISTATS 2025). Mai Khao, Thailand, 29.04.2025 - 05.05.2024
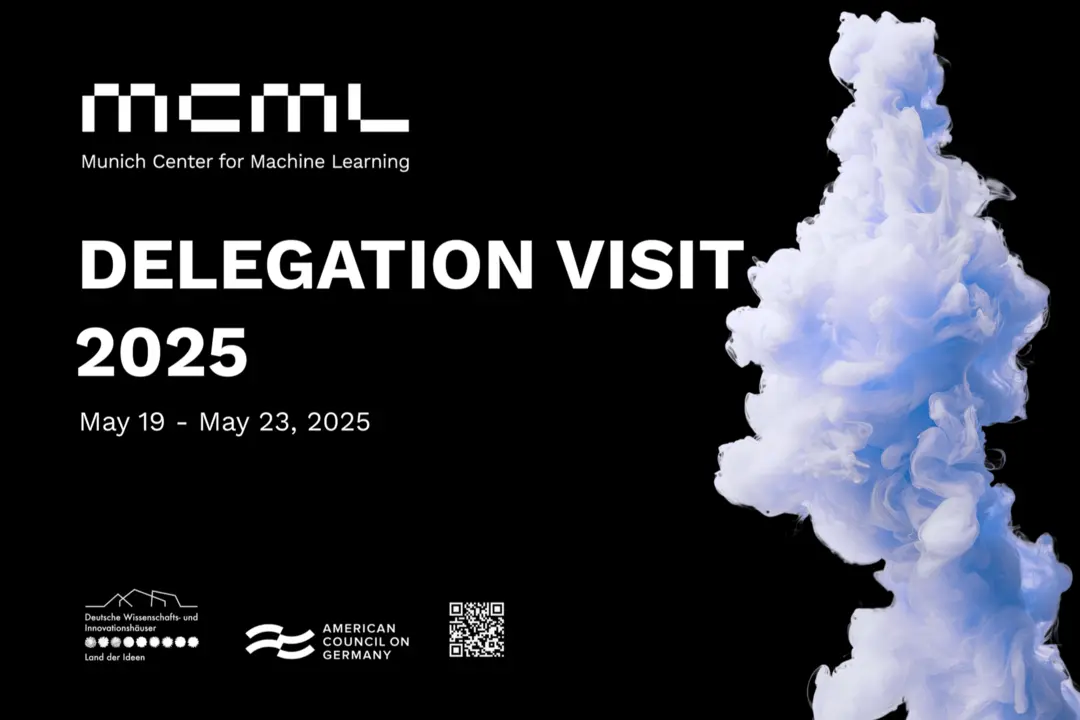
28.04.2025
MCML Delegation Visit to the USA
MCML delegation visits top US institutions to foster AI research collaborations in Generative and Medical AI, May 19–23, 2025.
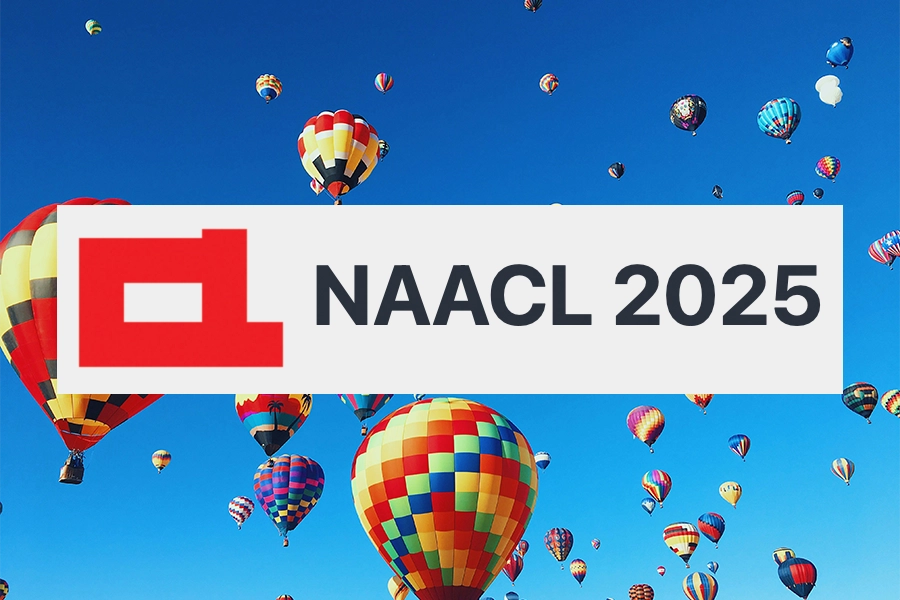
28.04.2025
MCML Researchers With Eleven Papers at NAACL 2025
Annual Conference of the North American Chapter of the Association for Computational Linguistics (NAACL 2025). Albuquerque, NM, USA, 29.04.2025 - 04.05.2024
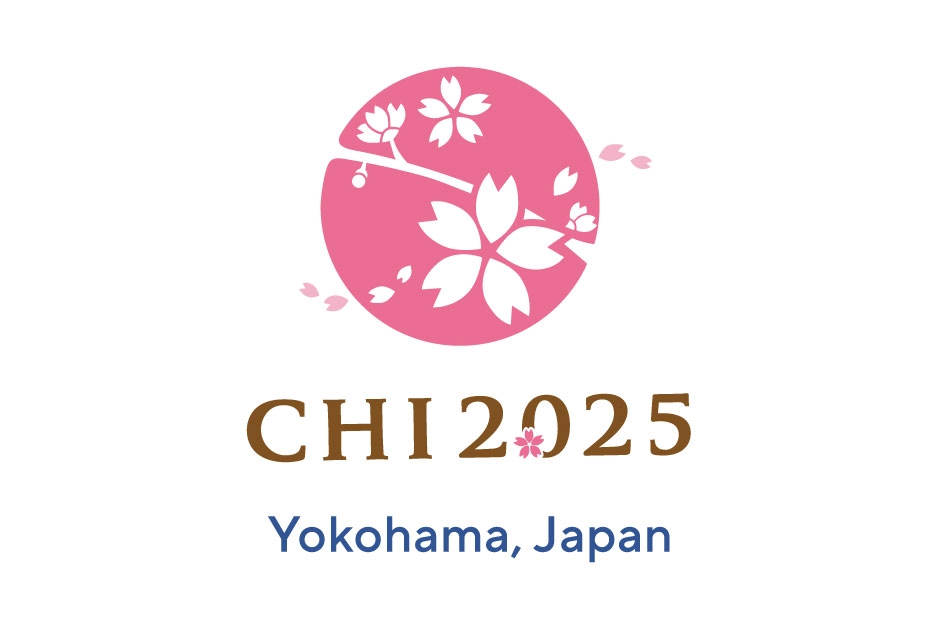
25.04.2025
MCML Researchers With Seven Papers at CHI 2025
Conference on Human Factors in Computing Systems (CHI 2025). Yokohama, Japan, 26.04.2025 - 01.05.2024