22.11.2022
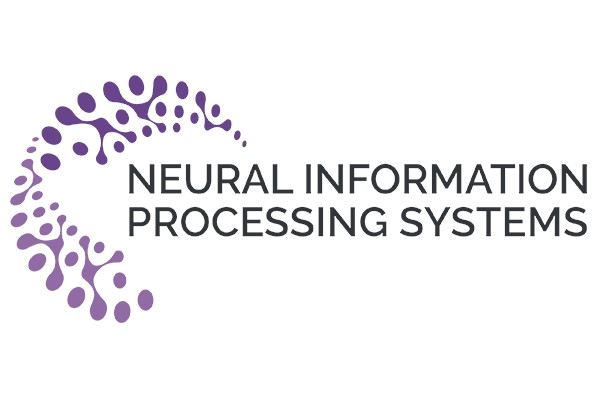
Eleven papers at NeurIPS 2022
36th Conference on Neural Information Processing Systems (NeurIPS 2022). New Orleans, LA, USA, 28.11.2022–09.12.2022
We are happy to announce that MCML researchers are represented with eleven papers at NeurIPS 2022:
Pitfalls of Epistemic Uncertainty Quantification through Loss Minimisation.
NeurIPS 2022 - 36th Conference on Neural Information Processing Systems. New Orleans, LA, USA, Nov 28-Dec 09, 2022. URL
Abstract
Uncertainty quantification has received increasing attention in machine learning in the recent past. In particular, a distinction between aleatoric and epistemic uncertainty has been found useful in this regard. The latter refers to the learner’s (lack of) knowledge and appears to be especially difficult to measure and quantify. In this paper, we analyse a recent proposal based on the idea of a second-order learner, which yields predictions in the form of distributions over probability distributions. While standard (first-order) learners can be trained to predict accurate probabilities, namely by minimising suitable loss functions on sample data, we show that loss minimisation does not work for second-order predictors: The loss functions proposed for inducing such predictors do not incentivise the learner to represent its epistemic uncertainty in a faithful way.
MCML Authors
Retrieval-Augmented Diffusion Models.
NeurIPS 2022 - 36th Conference on Neural Information Processing Systems. New Orleans, LA, USA, Nov 28-Dec 09, 2022. URL
Abstract
Novel architectures have recently improved generative image synthesis leading to excellent visual quality in various tasks. Much of this success is due to the scalability of these architectures and hence caused by a dramatic increase in model complexity and in the computational resources invested in training these models. Our work questions the underlying paradigm of compressing large training data into ever growing parametric representations. We rather present an orthogonal, semi-parametric approach. We complement comparably small diffusion or autoregressive models with a separate image database and a retrieval strategy. During training we retrieve a set of nearest neighbors from this external database for each training instance and condition the generative model on these informative samples. While the retrieval approach is providing the (local) content, the model is focusing on learning the composition of scenes based on this content. As demonstrated by our experiments, simply swapping the database for one with different contents transfers a trained model post-hoc to a novel domain. The evaluation shows competitive performance on tasks which the generative model has not been trained on, such as class-conditional synthesis, zero-shot stylization or text-to-image synthesis without requiring paired text-image data. With negligible memory and computational overhead for the external database and retrieval we can significantly reduce the parameter count of the generative model and still outperform the state-of-the-art.
MCML Authors
Finding optimal arms in non-stochastic combinatorial bandits with semi-bandit feedback and finite budget.
NeurIPS 2022 - 36th Conference on Neural Information Processing Systems. New Orleans, LA, USA, Nov 28-Dec 09, 2022. URL
Abstract
We consider the combinatorial bandits problem with semi-bandit feedback under finite sampling budget constraints, in which the learner can carry out its action only for a limited number of times specified by an overall budget. The action is to choose a set of arms, whereupon feedback for each arm in the chosen set is received. Unlike existing works, we study this problem in a non-stochastic setting with subset-dependent feedback, i.e., the semi-bandit feedback received could be generated by an oblivious adversary and also might depend on the chosen set of arms. In addition, we consider a general feedback scenario covering both the numerical-based as well as preference-based case and introduce a sound theoretical framework for this setting guaranteeing sensible notions of optimal arms, which a learner seeks to find. We suggest a generic algorithm suitable to cover the full spectrum of conceivable arm elimination strategies from aggressive to conservative. Theoretical questions about the sufficient and necessary budget of the algorithm to find the best arm are answered and complemented by deriving lower bounds for any learning algorithm for this problem scenario.
MCML Authors
Predicting Cellular Responses to Novel Drug Perturbations at a Single-Cell Resolution.
NeurIPS 2022 - 36th Conference on Neural Information Processing Systems. New Orleans, LA, USA, Nov 28-Dec 09, 2022. URL
Abstract
Single-cell transcriptomics enabled the study of cellular heterogeneity in response to perturbations at the resolution of individual cells. However, scaling high-throughput screens (HTSs) to measure cellular responses for many drugs remains a challenge due to technical limitations and, more importantly, the cost of such multiplexed experiments. Thus, transferring information from routinely performed bulk RNA HTS is required to enrich single-cell data meaningfully.We introduce chemCPA, a new encoder-decoder architecture to study the perturbational effects of unseen drugs. We combine the model with an architecture surgery for transfer learning and demonstrate how training on existing bulk RNA HTS datasets can improve generalisation performance. Better generalisation reduces the need for extensive and costly screens at single-cell resolution. We envision that our proposed method will facilitate more efficient experiment designs through its ability to generate in-silico hypotheses, ultimately accelerating drug discovery.
MCML Authors
A Graph Is More Than Its Nodes: Towards Structured Uncertainty-Aware Learning on Graphs.
NeurIPS 2022 - Workshop on New Frontiers in Graph Learning at the 36th Conference on Neural Information Processing Systems. New Orleans, LA, USA, Nov 28-Dec 09, 2022. URL
Abstract
Current graph neural networks (GNNs) that tackle node classification on graphs tend to only focus on nodewise scores and are solely evaluated by nodewise metrics. This limits uncertainty estimation on graphs since nodewise marginals do not fully characterize the joint distribution given the graph structure. In this work, we propose novel edgewise metrics, namely the edgewise expected calibration error (ECE) and the agree/disagree ECEs, which provide criteria for uncertainty estimation on graphs beyond the nodewise setting. Our experiments demonstrate that the proposed edgewise metrics can complement the nodewise results and yield additional insights. Moreover, we show that GNN models which consider the structured prediction problem on graphs tend to have better uncertainty estimations, which illustrates the benefit of going beyond the nodewise setting.
MCML Authors
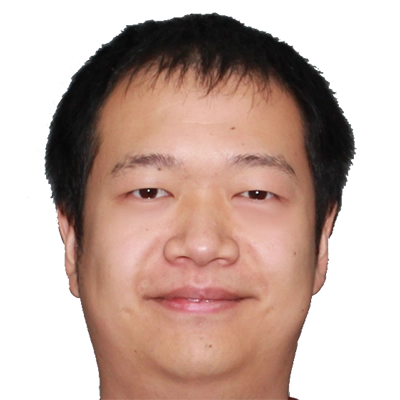
Yuesong Shen
Computer Vision & Artificial Intelligence
What Makes Graph Neural Networks Miscalibrated?.
NeurIPS 2022 - 36th Conference on Neural Information Processing Systems. New Orleans, LA, USA, Nov 28-Dec 09, 2022. URL
Abstract
Given the importance of getting calibrated predictions and reliable uncertainty estimations, various post-hoc calibration methods have been developed for neural networks on standard multi-class classification tasks. However, these methods are not well suited for calibrating graph neural networks (GNNs), which presents unique challenges such as accounting for the graph structure and the graph-induced correlations between the nodes. In this work, we conduct a systematic study on the calibration qualities of GNN node predictions. In particular, we identify five factors which influence the calibration of GNNs: general under-confident tendency, diversity of nodewise predictive distributions, distance to training nodes, relative confidence level, and neighborhood similarity. Furthermore, based on the insights from this study, we design a novel calibration method named Graph Attention Temperature Scaling (GATS), which is tailored for calibrating graph neural networks. GATS incorporates designs that address all the identified influential factors and produces nodewise temperature scaling using an attention-based architecture. GATS is accuracy-preserving, data-efficient, and expressive at the same time. Our experiments empirically verify the effectiveness of GATS, demonstrating that it can consistently achieve state-of-the-art calibration results on various graph datasets for different GNN backbones.
MCML Authors
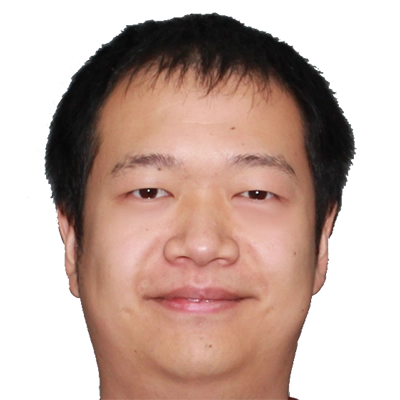
Yuesong Shen
Computer Vision & Artificial Intelligence
Transformer Model for Genome Sequence Analysis.
LMRL @NeurIPS 2022 - Workshop on Learning Meaningful Representations of Life at the 36th Conference on Neural Information Processing Systems (NeurIPS 2022). New Orleans, LA, USA, Nov 28-Dec 09, 2022. URL
Abstract
One major challenge of applying machine learning in genomics is the scarcity of labeled data, which often requires expensive and time-consuming physical experimentation under laboratory conditions to obtain. However, the advent of high throughput sequencing has made large quantities of unlabeled genome data available. This can be used to apply semi-supervised learning methods through representation learning. In this paper, we investigate the impact of a popular and well-established language model, namely BERT [Devlin et al., 2018], for sequence genome analysis. Specifically, we adapt DNABERT [Ji et al., 2021] to GenomeNet-BERT in order to produce useful representations for downstream tasks such as classification and semi10 supervised learning. We explore different pretraining setups and compare their performance on a virus genome classification task to strictly supervised training and baselines on different training set size setups. The conducted experiments show that this architecture provides an increase in performance compared to existing methods at the cost of more resource-intensive training.
MCML Authors
This joke is [MASK]: Recognizing Humor and Offense with Prompting.
TL4NLP @NeurIPS 2022 - 1st Transfer Learning for Natural Language Processing Workshop at the 36th Conference on Neural Information Processing Systems (NeurIPS 2022). New Orleans, LA, USA, Nov 28-Dec 09, 2022. URL
Abstract
Humor is a magnetic component in everyday human interactions and communications. Computationally modeling humor enables NLP systems to entertain and engage with users. We investigate the effectiveness of prompting, a new transfer learning paradigm for NLP, for humor recognition. We show that prompting performs similarly to finetuning when numerous annotations are available, but gives stellar performance in low-resource humor recognition. The relationship between humor and offense is also inspected by applying influence functions to prompting; we show that models could rely on offense to determine humor during transfer.
MCML Authors
Randomized Message-Interception Smoothing: Gray-box Certificates for Graph Neural Networks.
NeurIPS 2022 - 36th Conference on Neural Information Processing Systems. New Orleans, LA, USA, Nov 28-Dec 09, 2022. URL
Abstract
Randomized smoothing is one of the most promising frameworks for certifying the adversarial robustness of machine learning models, including Graph Neural Networks (GNNs). Yet, existing randomized smoothing certificates for GNNs are overly pessimistic since they treat the model as a black box, ignoring the underlying architecture. To remedy this, we propose novel gray-box certificates that exploit the message-passing principle of GNNs: We randomly intercept messages and carefully analyze the probability that messages from adversarially controlled nodes reach their target nodes. Compared to existing certificates, we certify robustness to much stronger adversaries that control entire nodes in the graph and can arbitrarily manipulate node features. Our certificates provide stronger guarantees for attacks at larger distances, as messages from farther-away nodes are more likely to get intercepted. We demonstrate the effectiveness of our method on various models and datasets. Since our gray-box certificates consider the underlying graph structure, we can significantly improve certifiable robustness by applying graph sparsification.
MCML Authors
Deep Combinatorial Aggregation.
NeurIPS 2022 - 36th Conference on Neural Information Processing Systems. New Orleans, LA, USA, Nov 28-Dec 09, 2022. URL
Abstract
Neural networks are known to produce poor uncertainty estimations, and a variety of approaches have been proposed to remedy this issue. This includes deep ensemble, a simple and effective method that achieves state-of-the-art results for uncertainty-aware learning tasks. In this work, we explore a combinatorial generalization of deep ensemble called deep combinatorial aggregation (DCA). DCA creates multiple instances of network components and aggregates their combinations to produce diversified model proposals and predictions. DCA components can be defined at different levels of granularity. And we discovered that coarse-grain DCAs can outperform deep ensemble for uncertainty-aware learning both in terms of predictive performance and uncertainty estimation. For fine-grain DCAs, we discover that an average parameterization approach named deep combinatorial weight averaging (DCWA) can improve the baseline training. It is on par with stochastic weight averaging (SWA) but does not require any custom training schedule or adaptation of BatchNorm layers. Furthermore, we propose a consistency enforcing loss that helps the training of DCWA and modelwise DCA. We experiment on in-domain, distributional shift, and out-of-distribution image classification tasks, and empirically confirm the effectiveness of DCWA and DCA approaches.
MCML Authors
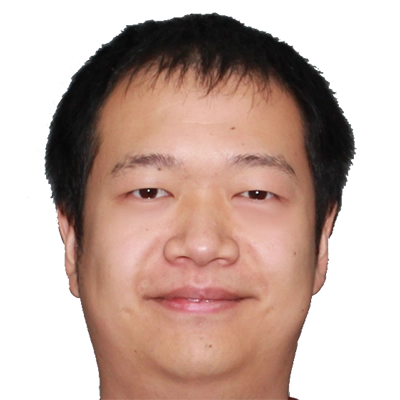
Yuesong Shen
Computer Vision & Artificial Intelligence
What cleaves? Is proteasomal cleavage prediction reaching a ceiling?.
LMRL @NeurIPS 2022 - Workshop on Learning Meaningful Representations of Life at the 36th Conference on Neural Information Processing Systems (NeurIPS 2022). New Orleans, LA, USA, Nov 28-Dec 09, 2022. URL
Abstract
Epitope vaccines are a promising direction to enable precision treatment for cancer, autoimmune diseases, and allergies. Effectively designing such vaccines requires accurate prediction of proteasomal cleavage in order to ensure that the epitopes in the vaccine are presented to T cells by the major histocompatibility complex (MHC). While direct identification of proteasomal cleavage in vitro is cumbersome and low throughput, it is possible to implicitly infer cleavage events from the termini of MHC-presented epitopes, which can be detected in large amounts thanks to recent advances in high-throughput MHC ligandomics. Inferring cleavage events in such a way provides an inherently noisy signal which can be tackled with new developments in the field of deep learning that supposedly make it possible to learn predictors from noisy labels. Inspired by such innovations, we sought to modernize proteasomal cleavage predictors by benchmarking a wide range of recent methods, including LSTMs, transformers, CNNs, and denoising methods, on a recently introduced cleavage dataset. We found that increasing model scale and complexity appeared to deliver limited performance gains, as several methods reached about 88.5% AUC on C-terminal and 79.5% AUC on N-terminal cleavage prediction. This suggests that the noise and/or complexity of proteasomal cleavage and the subsequent biological processes of the antigen processing pathway are the major limiting factors for predictive performance rather than the specific modeling approach used. While biological complexity can be tackled by more data and better models, noise and randomness inherently limit the maximum achievable predictive performance.
MCML Authors
22.11.2022
Related
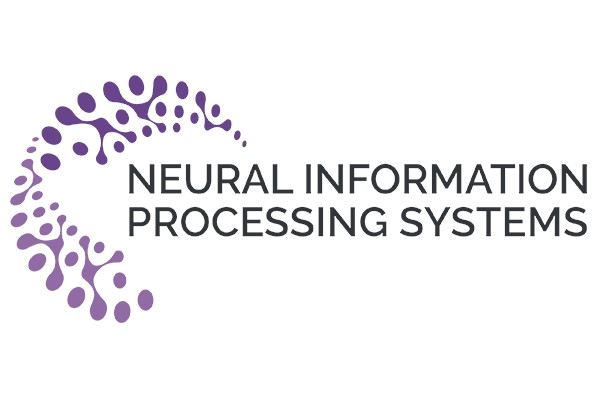
05.12.2024
26 papers at NeurIPS 2024
38th Conference on Neural Information Processing Systems (NeurIPS 2024). Vancouver, Canada, 10.12.2024 - 15.12.2024
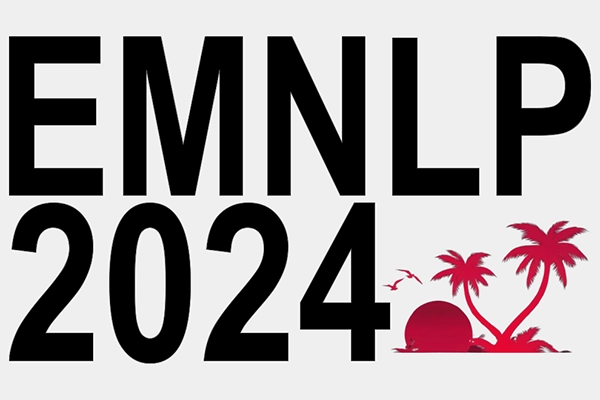
06.11.2024
20 papers at EMNLP 2024
Conference on Empirical Methods in Natural Language Processing (EMNLP 2024). Miami, FL, USA, 12.11.2024 - 16.11.2024
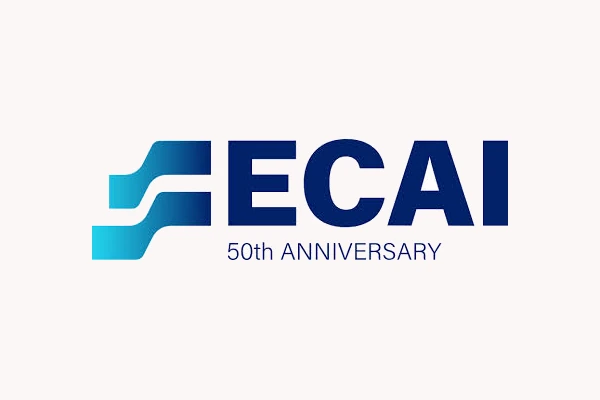
18.10.2024
Three papers at ECAI 2024
27th European Conference on Artificial Intelligence (ECAI 2024). Santiago de Compostela, Spain, 19.10.2024 - 24.10.2024
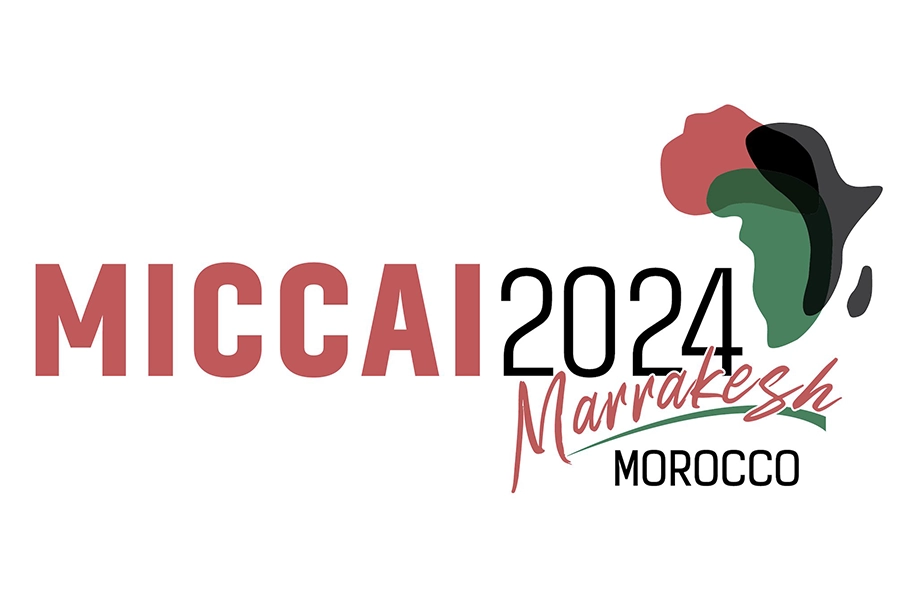
01.10.2024
16 papers at MICCAI 2024
27th International Conference on Medical Image Computing and Computer Assisted Intervention (MICCAI 2024). Marrakesh, Morocco, 06.10.2024 - 10.10.2024
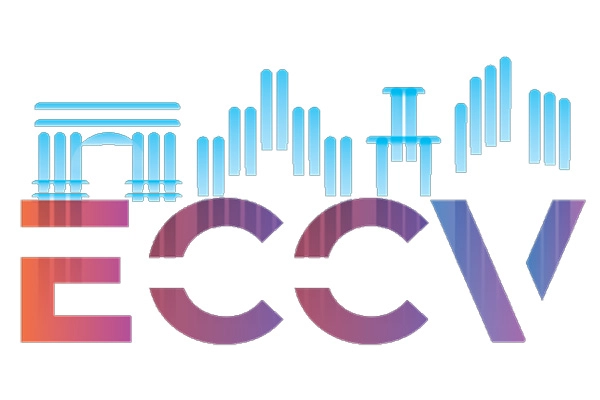
26.09.2024
20 papers at ECCV 2024
18th European Conference on Computer Vision (ECCV 2024). Milano, Italy, 29.09.2024 - 04.10.2024