20.10.2022
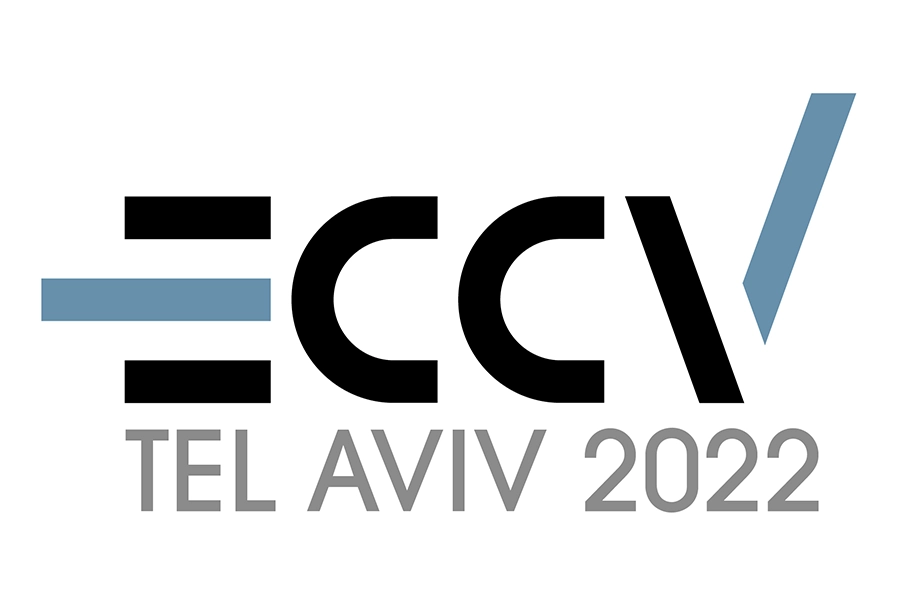
MCML Researchers With Two Papers at ECCV 2022
17th European Conference on Computer Vision (ECCV 2022). Tel Aviv, Israel, 23.10.2022–27.10.2022
We are happy to announce that MCML researchers are represented with two papers at ECCV 2022. Congrats to our researchers!
Main Track (2 papers)
Relationformer: A Unified Framework for Image-to-Graph Generation.
ECCV 2022 - 17th European Conference on Computer Vision. Tel Aviv, Israel, Oct 23-27, 2022. DOI GitHub
Abstract
A comprehensive representation of an image requires understanding objects and their mutual relationship, especially in image-to-graph generation, e.g., road network extraction, blood-vessel network extraction, or scene graph generation. Traditionally, image-to-graph generation is addressed with a two-stage approach consisting of object detection followed by a separate relation prediction, which prevents simultaneous object-relation interaction. This work proposes a unified one-stage transformer-based framework, namely Relationformer that jointly predicts objects and their relations. We leverage direct set-based object prediction and incorporate the interaction among the objects to learn an object-relation representation jointly. In addition to existing [obj]-tokens, we propose a novel learnable token, namely [rln]-token. Together with [obj]-tokens, [rln]-token exploits local and global semantic reasoning in an image through a series of mutual associations. In combination with the pair-wise [obj]-token, the [rln]-token contributes to a computationally efficient relation prediction. We achieve state-of-the-art performance on multiple, diverse and multi-domain datasets that demonstrate our approach’s effectiveness and generalizability.
MCML Authors
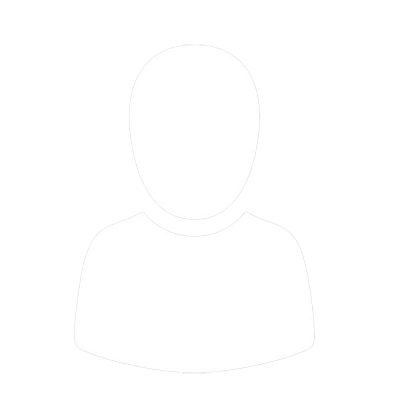
Georgios Kaissis
Dr.
* Former Member
Parameterized Temperature Scaling for Boosting the Expressive Power in Post-Hoc Uncertainty Calibration.
ECCV 2022 - 17th European Conference on Computer Vision. Tel Aviv, Israel, Oct 23-27, 2022. DOI GitHub
Abstract
We address the problem of uncertainty calibration and introduce a novel calibration method, Parametrized Temperature Scaling (PTS). Standard deep neural networks typically yield uncalibrated predictions, which can be transformed into calibrated confidence scores using post-hoc calibration methods. In this contribution, we demonstrate that the performance of accuracy-preserving state-of-the-art post-hoc calibrators is limited by their intrinsic expressive power. We generalize temperature scaling by computing prediction-specific temperatures, parameterized by a neural network. We show with extensive experiments that our novel accuracy-preserving approach consistently outperforms existing algorithms across a large number of model architectures, datasets and metrics.
MCML Authors
Computer Vision & Artificial Intelligence
20.10.2022
Related
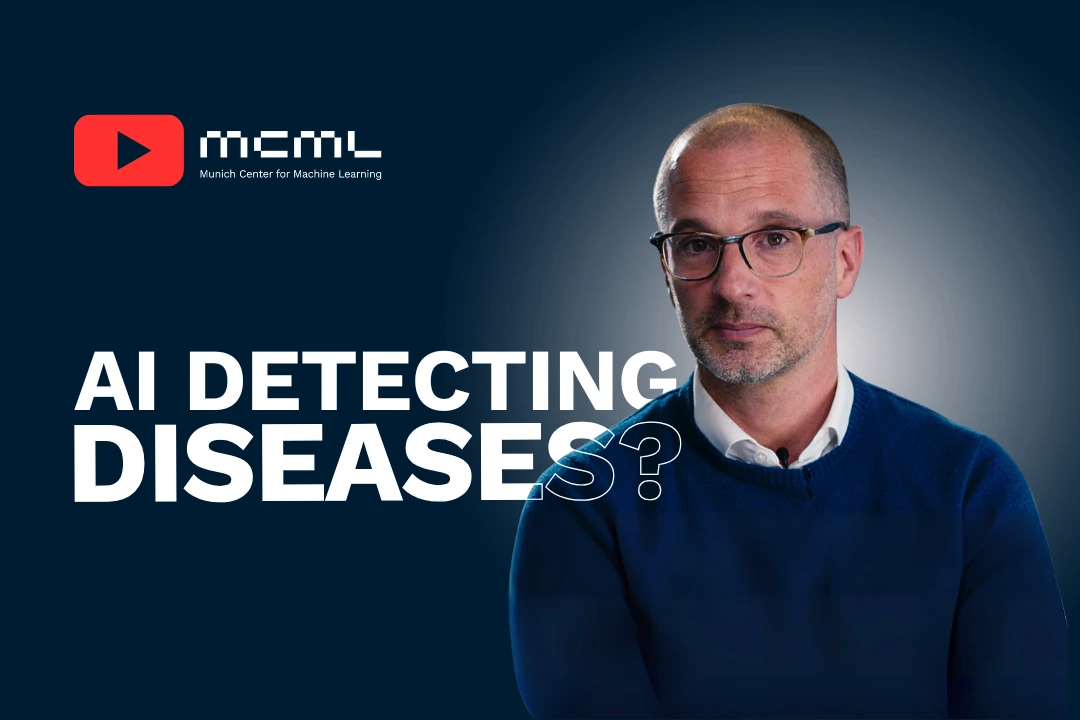
25.06.2025
When Clinical Expertise Meets AI Innovation – With Michael Ingrisch
The new research film features Michael Ingrisch, who shows how AI and clinical expertise can solve real challenges in radiology together.
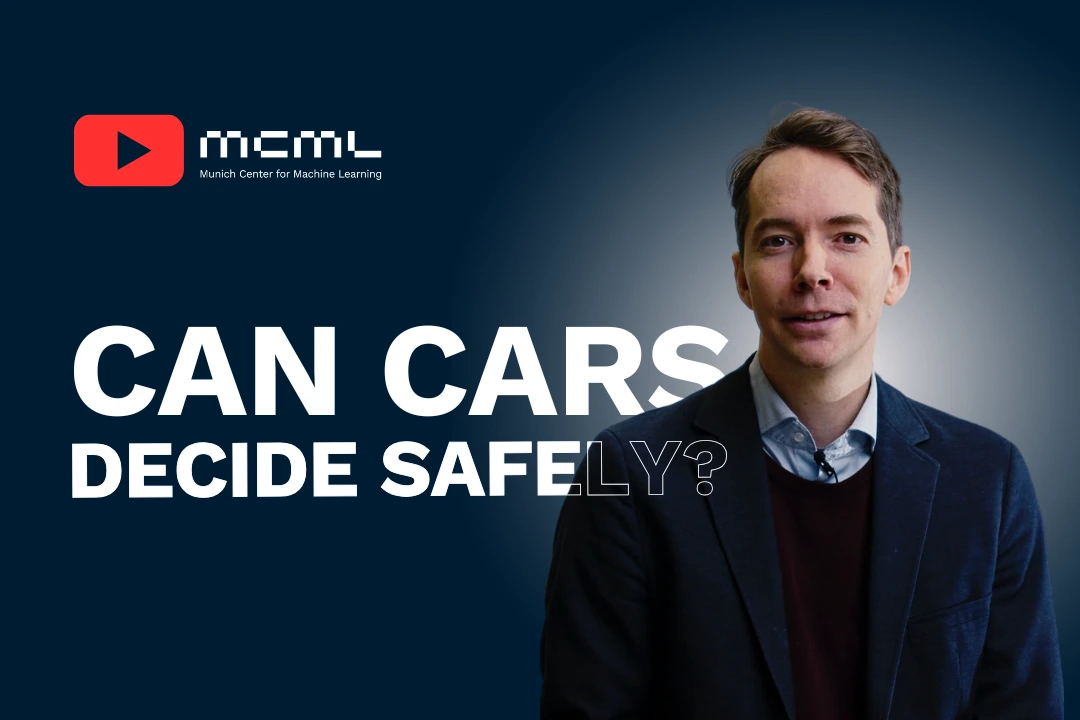
23.06.2025
Autonomous Driving: From Infinite Possibilities to Safe Decisions— With Matthias Althoff
The new research film features Matthias Althoff explaining how his team verifies autonomous vehicle safety using EDGAR and rigorous testing.
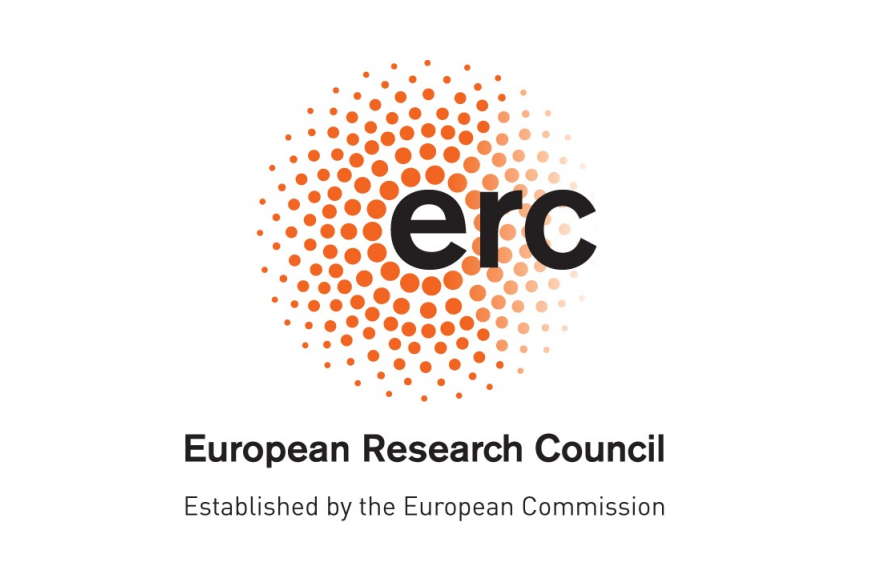
20.06.2025
ERC Advanced Grant for Massimo Fornasier
Massimo Fornasier was awarded ERC Advanced Grant to develop advanced algorithms for solving complex nonconvex optimization problems.
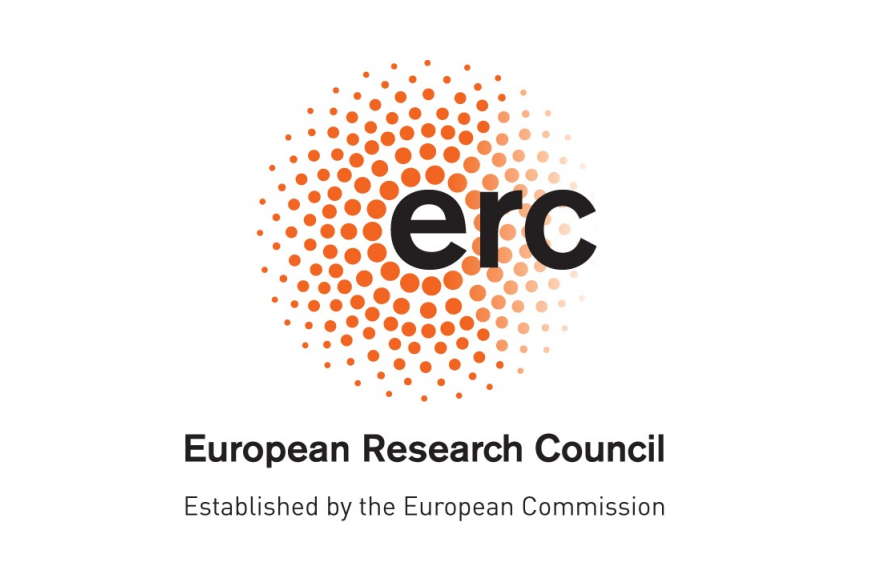
18.06.2025
ERC Advanced Grant for Albrecht Schmidt
Albrecht Schmidt receives ERC Advanced Grant for research on personalized generative AI to support memory, planning, and creativity.