11.07.2022
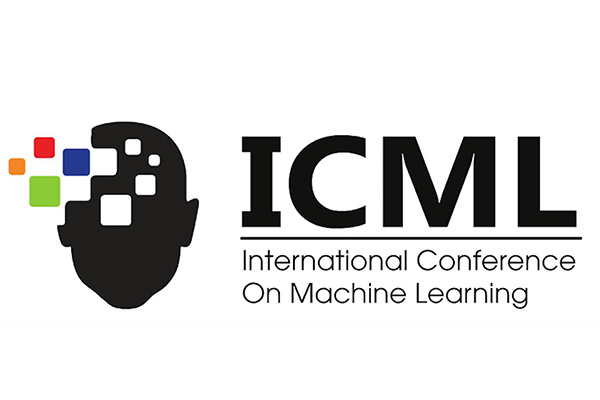
MCML Researchers With Two Papers at ICML 2022
39th International Conference on Machine Learning (ICML 2022). Baltimore, MD, USA, 17.07.2022–23.07.2023
We are happy to announce that MCML researchers are represented with two papers at ICML 2022. Congrats to our researchers!
Workshops (2 papers)
Predicting single-cell perturbation responses for unseen drugs.
MLDD @ICML 2022 - Workshop on Machine Learning for Drug Discovery at the 39th International Conference on Machine Learning (ICML 2022). Baltimore, MD, USA, Jul 17-23, 2022. URL
Abstract
Single-cell transcriptomics enabled the study of cellular heterogeneity in response to perturbations at the resolution of individual cells. However, scaling high-throughput screens (HTSs) to measure cellular responses for many drugs remains a challenge due to technical limitations and, more importantly, the cost of such multiplexed experiments. Thus, transferring information from routinely performed bulk RNA-seq HTS is required to enrich single-cell data meaningfully. We introduce a new encoder-decoder architecture to study the perturbational effects of unseen drugs. We combine the model with a transfer learning scheme and demonstrate how training on existing bulk RNA-seq HTS datasets can improve generalisation performance. Better generalisation reduces the need for extensive and costly screens at single-cell resolution. We envision that our proposed method will facilitate more efficient experiment designs through its ability to generate in-silico hypotheses, ultimately accelerating targeted drug discovery.
MCML Authors
Analyzing the Effects of Handling Data Imbalance on Learned Features from Medical Images by Looking Into the Models.
IMLH @ICML 2022 - 2nd Workshop on Interpretable Machine Learning in Healthcare at the 39th International Conference on Machine Learning (ICML 2022). Baltimore, MD, USA, Jul 17-23, 2022. arXiv
Abstract
One challenging property lurking in medical datasets is the imbalanced data distribution, where the frequency of the samples between the different classes is not balanced. Training a model on an imbalanced dataset can introduce unique challenges to the learning problem where a model is biased towards the highly frequent class. Many methods are proposed to tackle the distributional differences and the imbalanced problem. However, the impact of these approaches on the learned features is not well studied. In this paper, we look deeper into the internal units of neural networks to observe how handling data imbalance affects the learned features. We study several popular cost-sensitive approaches for handling data imbalance and analyze the feature maps of the convolutional neural networks from multiple perspectives: analyzing the alignment of salient features with pathologies and analyzing the pathology-related concepts encoded by the networks. Our study reveals differences and insights regarding the trained models that are not reflected by quantitative metrics such as AUROC and AP and show up only by looking at the models through a lens.
MCML Authors
Statistical Learning and Data Science
Statistical Learning and Data Science
Statistical Learning and Data Science
11.07.2022
Related
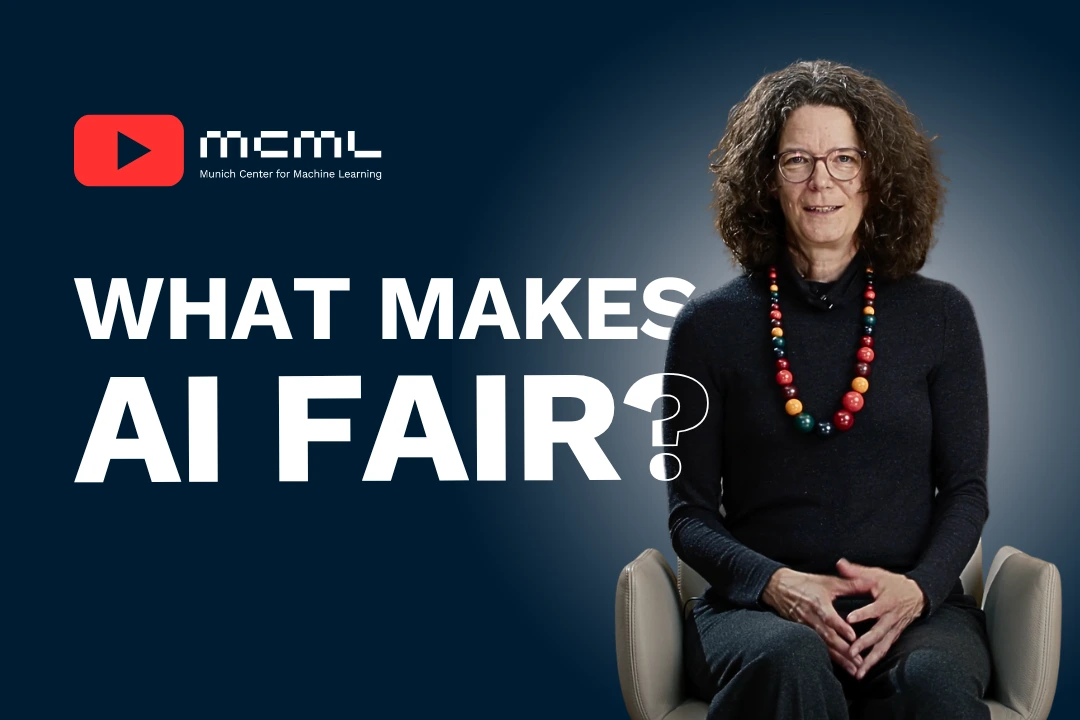
11.06.2025
Better Data, Smarter AI: Why Quality Matters – With Frauke Kreuter
In our new research film, Frauke Kreuter explains how data quality shapes fair, reliable, and socially responsible AI systems.
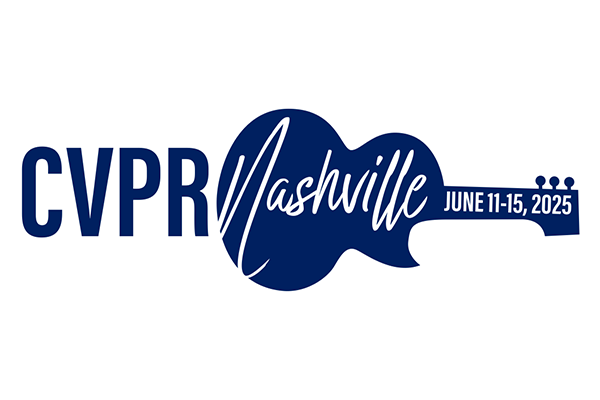
10.06.2025
MCML Researchers With 30 Papers at CVPR 2025
IEEE/CVF Conference on Computer Vision and Pattern Recognition (CVPR 2025). Nashville, TN, USA, 11.06.2025 - 15.06.2025
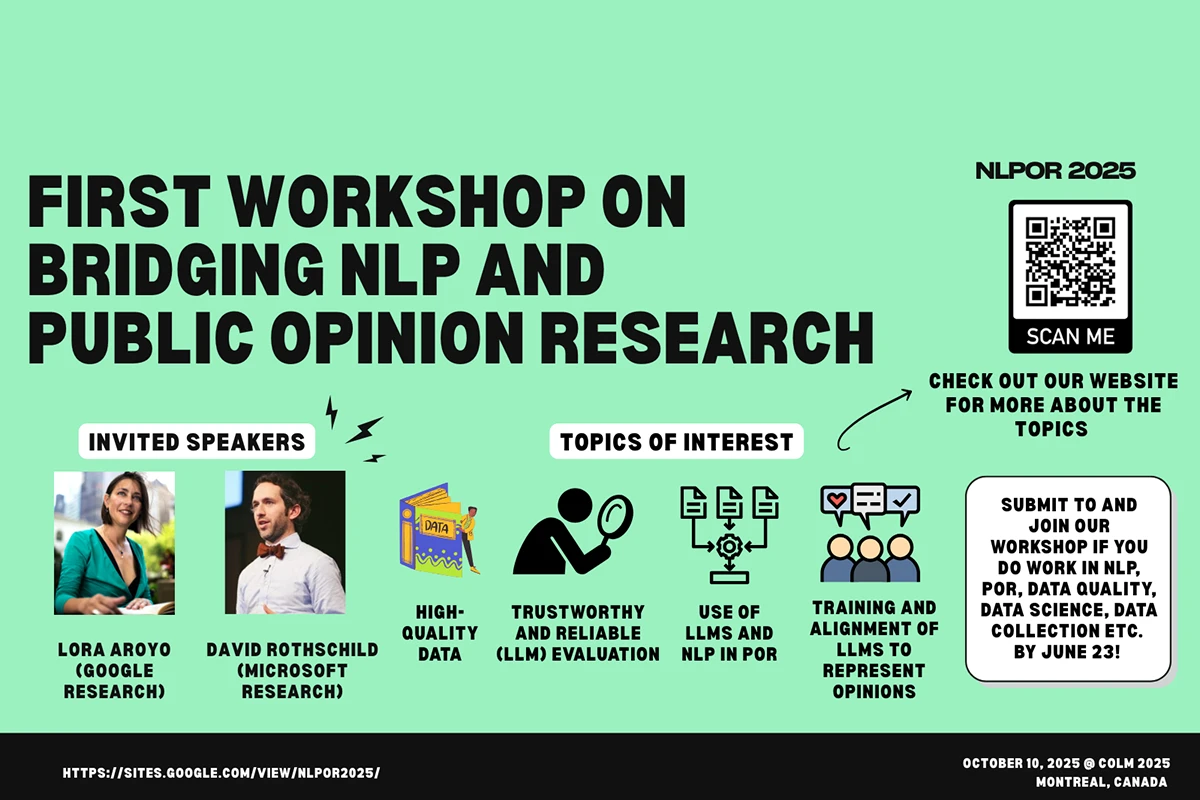
20.05.2025
Call for Papers for NLPOR - First Workshop on Bridging NLP and Public Opinion Research
This interdisciplinary workshop explores the powerful connections between Natural Language Processing (NLP) and Public Opinion Research (POR).
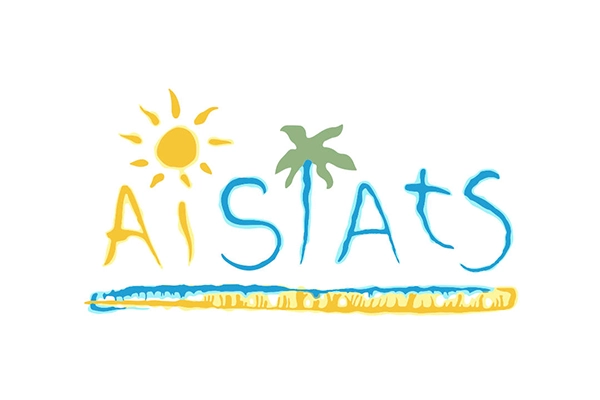
02.05.2025
MCML Researchers With Five Papers at AISTATS 2025
28th International Conference on Artificial Intelligence and Statistics (AISTATS 2025). Mai Khao, Thailand, 29.04.2025 - 05.05.2024