12.06.2022
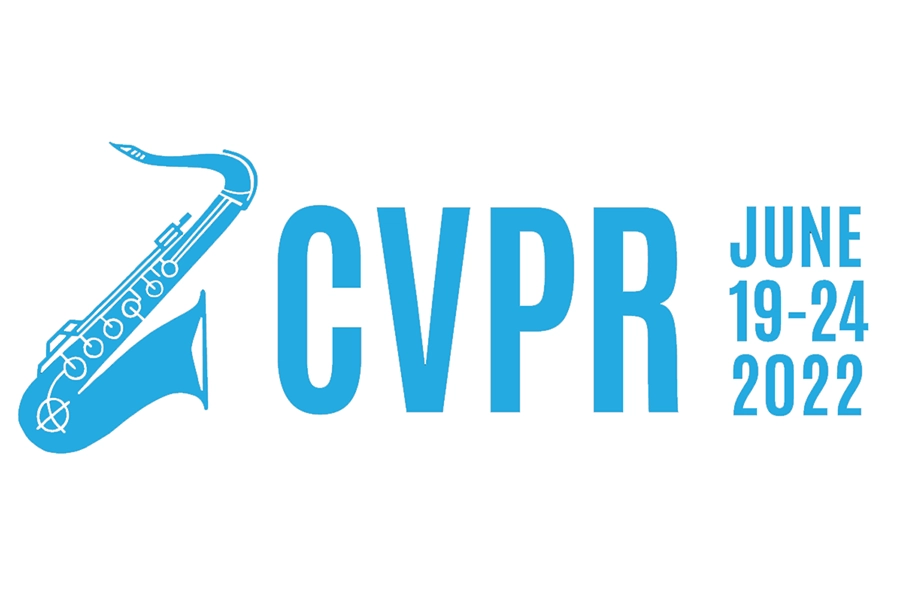
MCML Researchers With Two Papers at CVPR 2022
IEEE/CVF Conference on Computer Vision and Pattern Recognition (CVPR 2022). Vancouver, Canada, 19.06.2022–24.06.2022
We are happy to announce that MCML researchers are represented with two papers at CVPR 2022. Congrats to our researchers!
Main Track (2 papers)
Do Explanations Explain? Model Knows Best.
CVPR 2022 - IEEE/CVF Conference on Computer Vision and Pattern Recognition. New Orleans, LA, USA, Jun 19-24, 2022. DOI GitHub
Abstract
It is a mystery which input features contribute to a neural network’s output. Various explanation (feature attribution) methods are proposed in the literature to shed light on the problem. One peculiar observation is that these explanations (attributions) point to different features as being important. The phenomenon raises the question, which explanation to trust? We propose a framework for evaluating the explanations using the neural network model itself. The framework leverages the network to generate input features that impose a particular behavior on the output. Using the generated features, we devise controlled experimental setups to evaluate whether an explanation method conforms to an axiom. Thus we propose an empirical framework for axiomatic evaluation of explanation methods. We evaluate well-known and promising explanation solutions using the proposed framework. The framework provides a toolset to reveal properties and drawbacks within existing and future explanation solutions
MCML Authors
The Probabilistic Normal Epipolar Constraint for Frame-To-Frame Rotation Optimization under Uncertain Feature Positions.
CVPR 2022 - IEEE/CVF Conference on Computer Vision and Pattern Recognition. New Orleans, LA, USA, Jun 19-24, 2022. DOI
Abstract
The estimation of the relative pose of two camera views is a fundamental problem in computer vision. Kneip et al. proposed to solve this problem by introducing the normal epipolar constraint (NEC). However, their approach does not take into account uncertainties, so that the accuracy of the estimated relative pose is highly dependent on accurate feature positions in the target frame. In this work, we introduce the probabilistic normal epipolar constraint (PNEC) that overcomes this limitation by accounting for anisotropic and inhomogeneous uncertainties in the feature positions. To this end, we propose a novel objective function, along with an efficient optimization scheme that effectively minimizes our objective while maintaining real-time performance. In experiments on synthetic data, we demonstrate that the novel PNEC yields more accurate rotation estimates than the original NEC and several popular relative rotation estimation algorithms. Furthermore, we integrate the proposed method into a state-of-the-art monocular rotation-only odometry system and achieve consistently improved results for the real-world KITTI dataset.
MCML Authors
12.06.2022
Related
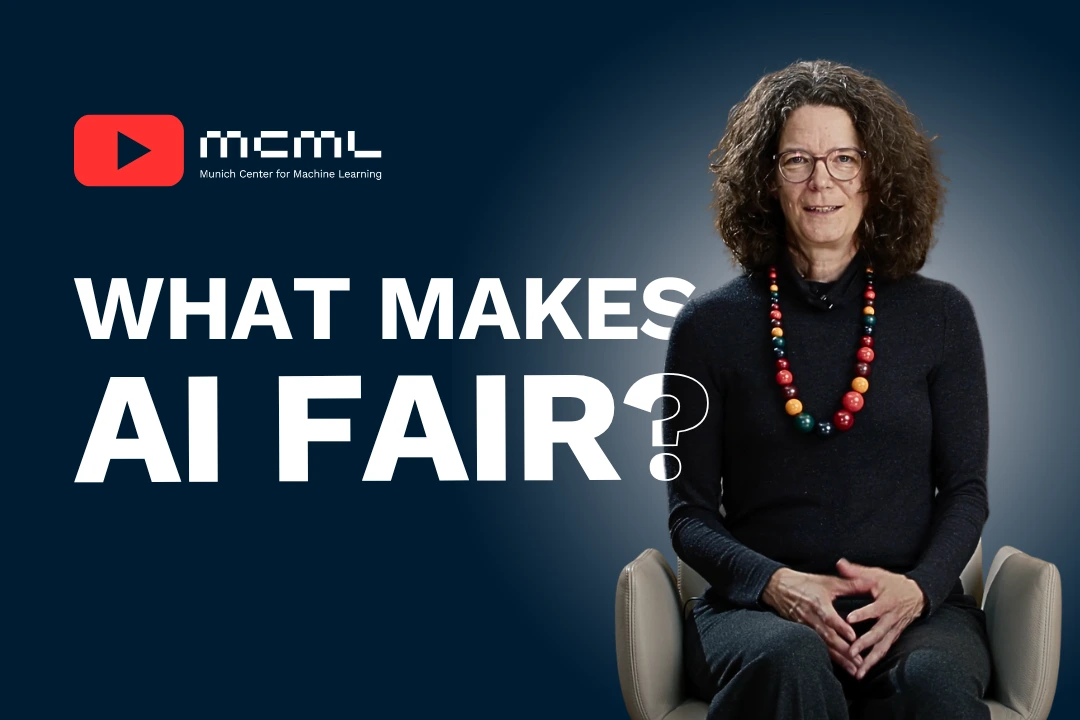
11.06.2025
Better Data, Smarter AI: Why Quality Matters – With Frauke Kreuter
In our new research film, Frauke Kreuter explains how data quality shapes fair, reliable, and socially responsible AI systems.
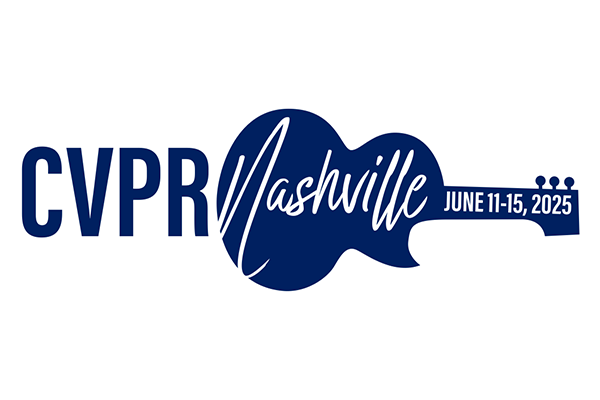
10.06.2025
MCML Researchers With 30 Papers at CVPR 2025
IEEE/CVF Conference on Computer Vision and Pattern Recognition (CVPR 2025). Nashville, TN, USA, 11.06.2025 - 15.06.2025
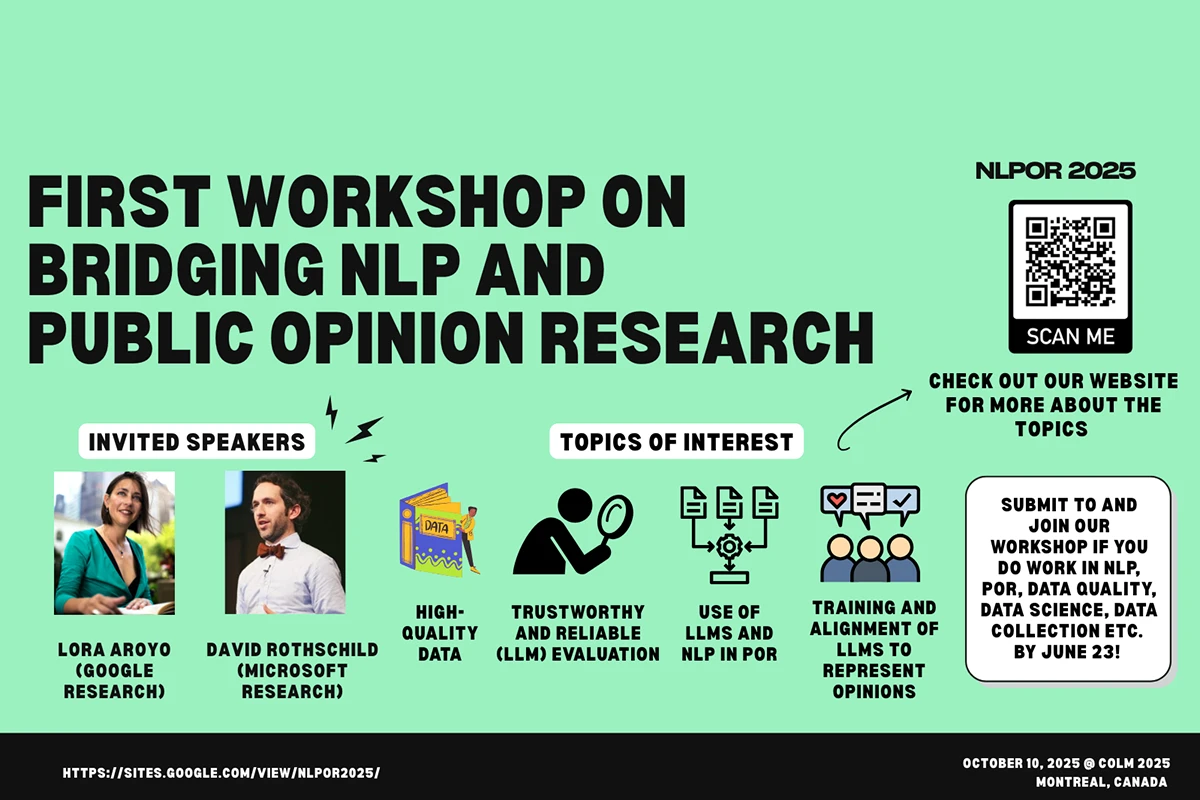
20.05.2025
Call for Papers for NLPOR - First Workshop on Bridging NLP and Public Opinion Research
This interdisciplinary workshop explores the powerful connections between Natural Language Processing (NLP) and Public Opinion Research (POR).
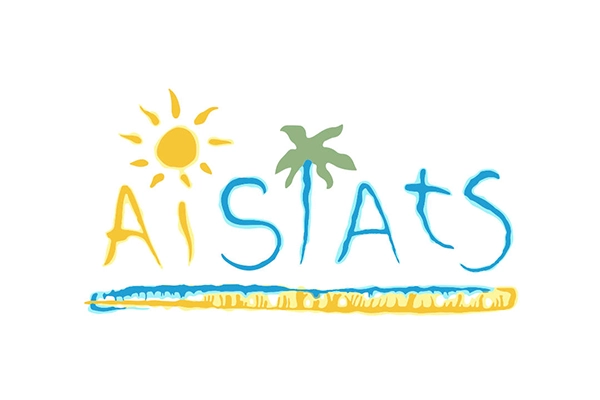
02.05.2025
MCML Researchers With Five Papers at AISTATS 2025
28th International Conference on Artificial Intelligence and Statistics (AISTATS 2025). Mai Khao, Thailand, 29.04.2025 - 05.05.2024