05.06.2022
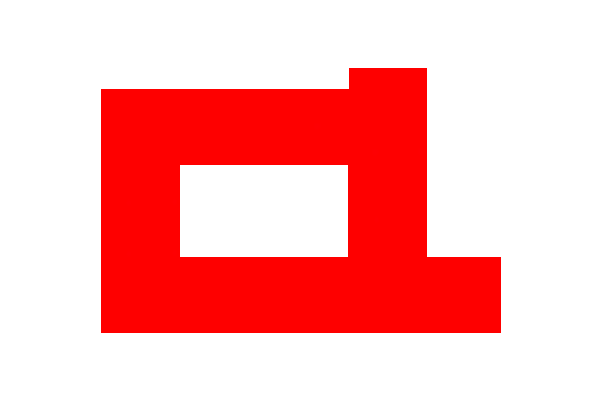
MCML Researchers With Two Papers at NAACL 2022
Annual Conference of the North American Chapter of the Association for Computational Linguistics (NAACL 2022). Seattle, WA, USA, 10.06.2022–15.06.2022
We are happy to announce that MCML researchers are represented with two papers at NAACL 2022. Congrats to our researchers!
Findings Track (2 papers)
An Information-Theoretic Approach and Dataset for Probing Gender Stereotypes in Multilingual Masked Language Models.
NAACL 2022 - Findings of the Annual Conference of the North American Chapter of the Association for Computational Linguistics. Seattle, WA, USA, Jun 10-15, 2022. DOI
Abstract
Bias research in NLP is a rapidly growing and developing field. Similar to CrowS-Pairs (Nangia et al., 2020), we assess gender bias in masked-language models (MLMs) by studying pairs of sentences with gender swapped person references.Most bias research focuses on and often is specific to English.Using a novel methodology for creating sentence pairs that is applicable across languages, we create, based on CrowS-Pairs, a multilingual dataset for English, Finnish, German, Indonesian and Thai.Additionally, we propose SJSD, a new bias measure based on Jensen–Shannon divergence, which we argue retains more information from the model output probabilities than other previously proposed bias measures for MLMs.Using multilingual MLMs, we find that SJSD diagnoses the same systematic biased behavior for non-English that previous studies have found for monolingual English pre-trained MLMs. SJSD outperforms the CrowS-Pairs measure, which struggles to find such biases for smaller non-English datasets.
MCML Authors
LMTurk: Few-Shot Learners as Crowdsourcing Workers in a Language-Model-as-a-Service Framework.
NAACL 2022 - Findings of the Annual Conference of the North American Chapter of the Association for Computational Linguistics. Seattle, WA, USA, Jun 10-15, 2022. DOI
Abstract
Vast efforts have been devoted to creating high-performance few-shot learners, i.e., large-scale pretrained language models (PLMs) that perform well with little downstream task training data. Training PLMs has incurred significant cost, but utilizing the few-shot learners is still challenging due to their enormous size. This work focuses on a crucial question: How to make effective use of these few-shot learners? We propose LMTurk, a novel approach that treats few-shotlearners as crowdsourcing workers. The rationale is that crowdsourcing workers are in fact few-shot learners: They are shown a few illustrative examples to learn about a task and then start annotating. LMTurk employs few-shot learners built upon PLMs as workers. We show that the resulting annotations can be utilized to train models that solve the task well and are small enough to be deployable in practical scenarios. Active learning is integrated into LMTurk to reduce the amount of queries made to PLMs, minimizing the computational cost of running PLM inference passes. Altogether, LMTurk is an important step towards making effective use of current PLMs.
MCML Authors
05.06.2022
Related
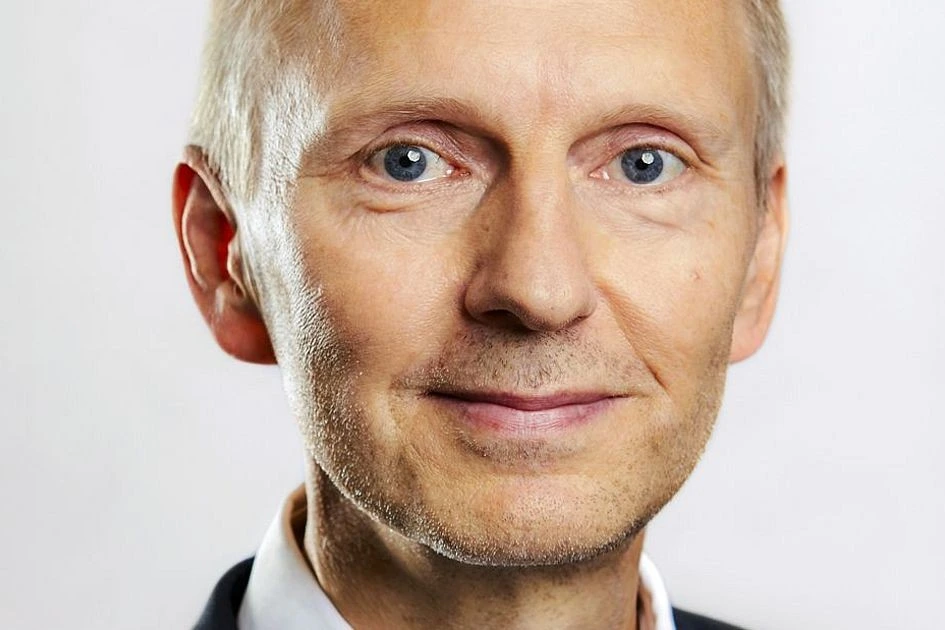
22.07.2025
Eyke Hüllermeier to Lead New DFG-Funded Research Training Group METEOR
MCML PI Eyke Hüllermeier to lead new DFG-funded RTG METEOR, uniting ML and control theory to build robust, explainable AI systems.
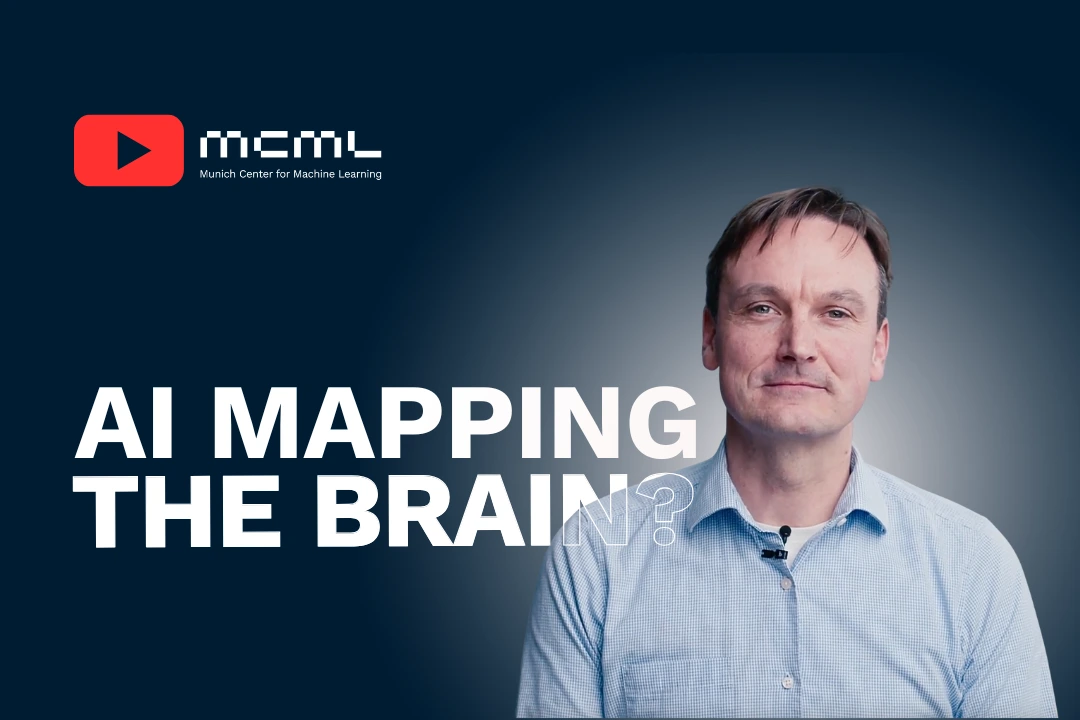
16.07.2025
AI-Powered Cortical Mapping for Neurodegenerative Disease Diagnoses - With Christian Wachinger
Research film with Christian Wachinger shows how AI maps the brain’s cortex to support diagnoses of neurodegenerative diseases.
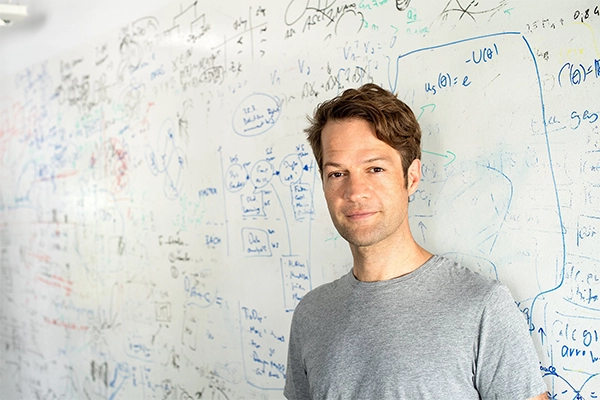
©TUM
15.07.2025
ERC Proof of Concept Grants for Fabian Theis
Fabian Theis receives ERC Proof of Concept Grants for his project on deep learning methods for dynamic single-cell data analysis.
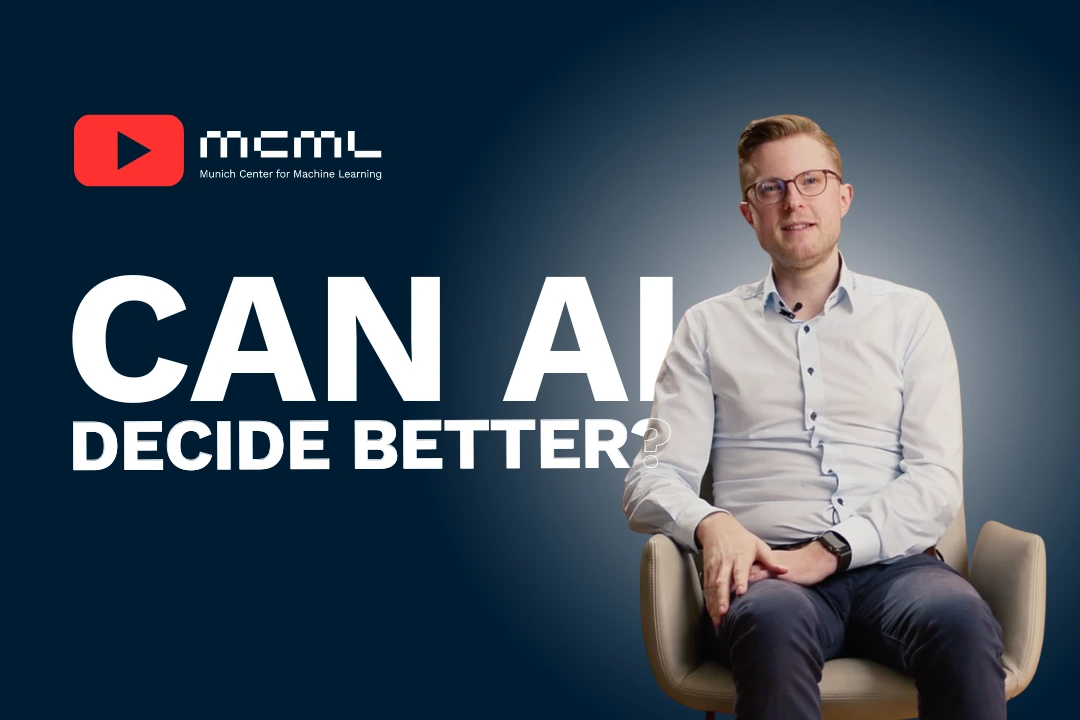
10.07.2025
Beyond Prediction: How Causal AI Enables Better Decision-Making - With Stefan Feuerriegel
Stefan Feuerriegel in our new film shows how Causal AI helps pick better actions by predicting outcomes for each possible decision.