29.04.2025
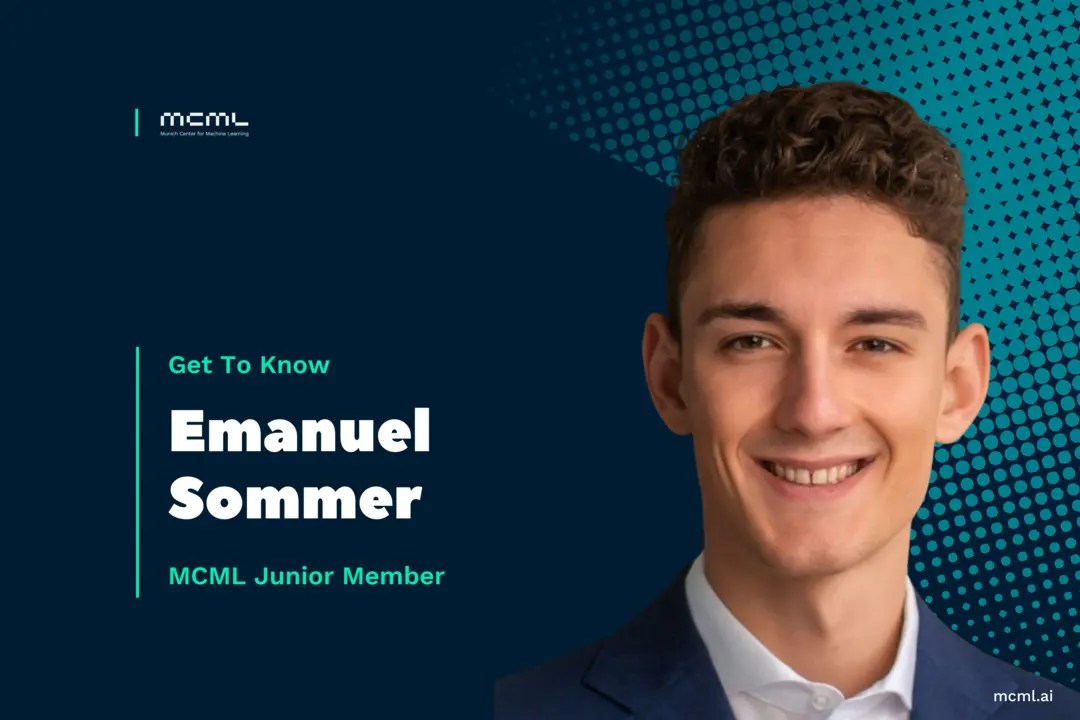
How Certain Is AI? An Introduction to Bayesian Deep Learning
Researcher in Focus: Emanuel Sommer
MCML Junior Member Emanuel Sommer is a PhD-student at the Munich Uncertainty Quantification AI Lab at LMU Munich supervised by David Rügamer. His research focuses on Scalable and Reliable (Bayesian) Deep Learning.
What is your research about?
My research focuses on improving how we estimate and measure uncertainty in modern deep learning models. In simple terms, I’m asking: How certain can we be about a model’s predictions? And more importantly: Are there other reasonable answers it might have missed?
To tackle these questions, I work within the field of Bayesian Deep Learning. The key idea is to move beyond a single prediction and instead consider a whole range of plausible alternatives—like gathering multiple expert opinions before making a decision. This makes AI systems not only smarter but also more trustworthy, especially in high-stakes environments. I also develop efficient software tools to ensure these ideas are usable in real-world applications.
«I’m asking: How certain can we be about a model’s predictions? And more importantly: Are there other reasonable answers it might have missed?»
Emanuel Sommer
MCML Junior Member
Could you explain what Bayesian Deep Learning is and why it is important?
A traditional deep learning model learns a single “opinion” about a prediction—say, a weather app that gives you one prediction—like “it will have 30° Celsius”—based on past data. While this opinion might often be correct, it lacks a sense of certainty about its correctness. Bayesian Deep Learning identifies alternative but still plausible opinions and by considering them it allows to quantify this uncertainty. So instead of just saying “it will be 30° Celsius”, it might report, “most likely the temperature tomorrow will be between 26° and 31° Celsius”.
Ideally, we would like to consider all possible explanations that fit the data and assess their likelihood. This matters because real-world data is often messy, incomplete, or ambiguous. With this broader perspective, predictions are no longer based on a single rigid hypothesis but rather on a weighted combination of many—akin to a voting system where each “expert opinion” contributes based on its credibility. This leads to more robust and reliable predictions, which is particularly important as AI takes on an increasing role in decision-making.
«Bayesian Deep Learning identifies alternative but still plausible opinions and by considering them it allows to quantify this uncertainty. »
Emanuel Sommer
MCML Junior Member
In your opinion, what are the disadvantages of Bayesian Deep Learning methods?
Bayesian methods are computationally more expensive because maintaining and evaluating multiple hypotheses requires significantly more resources than relying on a single point estimate. Furthermore, they are not as well understood as standard deep learning approaches, which have benefited from extensive theoretical and empirical research. Nonetheless, ongoing advances in software and methodology, including my own work, are making Bayesian approaches more scalable and practical.
What practical applications do you envision for Bayesian Deep Learning?
Bayesian Deep Learning is particularly valuable in safety-critical applications, where reliable uncertainty estimates are crucial. In the medical field, predictive models used for diagnosis or treatment recommendations must indicate when they are uncertain, as incorrect predictions can have severe consequences. Similarly, in finance, risk modeling depends on accurate uncertainty quantification to support investment and lending decisions. More broadly, any predictive model benefits from understanding and communicating its own uncertainty, making Bayesian approaches relevant across a wide range of applications.
«Improved communication of (un)certainty will foster trust in data-driven systems and consequently encourage widespread adoption of data-driven decision making.»
Emanuel Sommer
MCML Junior Member
How could your research contribute to improving decision-making under uncertainty?
By improving uncertainty quantification for deep neural networks, my research enhances decision-making in any domain where such predictive models play a role and uncertainty matters. While fully Bayesian methods remain computationally challenging for extremely large models like GPT4, many complex models in use today could already benefit from Bayesian inference techniques. As Bayesian methods become more efficient and accessible, they have the potential to significantly improve reliability. Moreover, I am convinced that improved communication of (un)certainty will foster trust in data-driven systems and consequently encourage widespread adoption of data-driven decision making.
What would the success of your work look like in a few years’ time?
If everything goes according to plan, Bayesian Deep Learning will become the default for many applications—efficient, reliable, and easy to use in practice. My goal is to make principled uncertainty quantification an affordable standard component of modern machine learning workflows. At the same time, I hope this progress encourages other researchers to engage with remaining practical challenges that, if solved, would make Bayesian methods truly mainstream. Further, I am convinced that the Bayesian perspective will lead to a better understanding of what and how modern machine learning systems, which are often perceived as black-box oracles, learn “under the hood”.
When you are not researching, how do you spend your time?
I love cooking, eating, and being active—especially hiking/skiing in the mountains. Fortunately, these interests complement each other quite well.
29.04.2025
Related
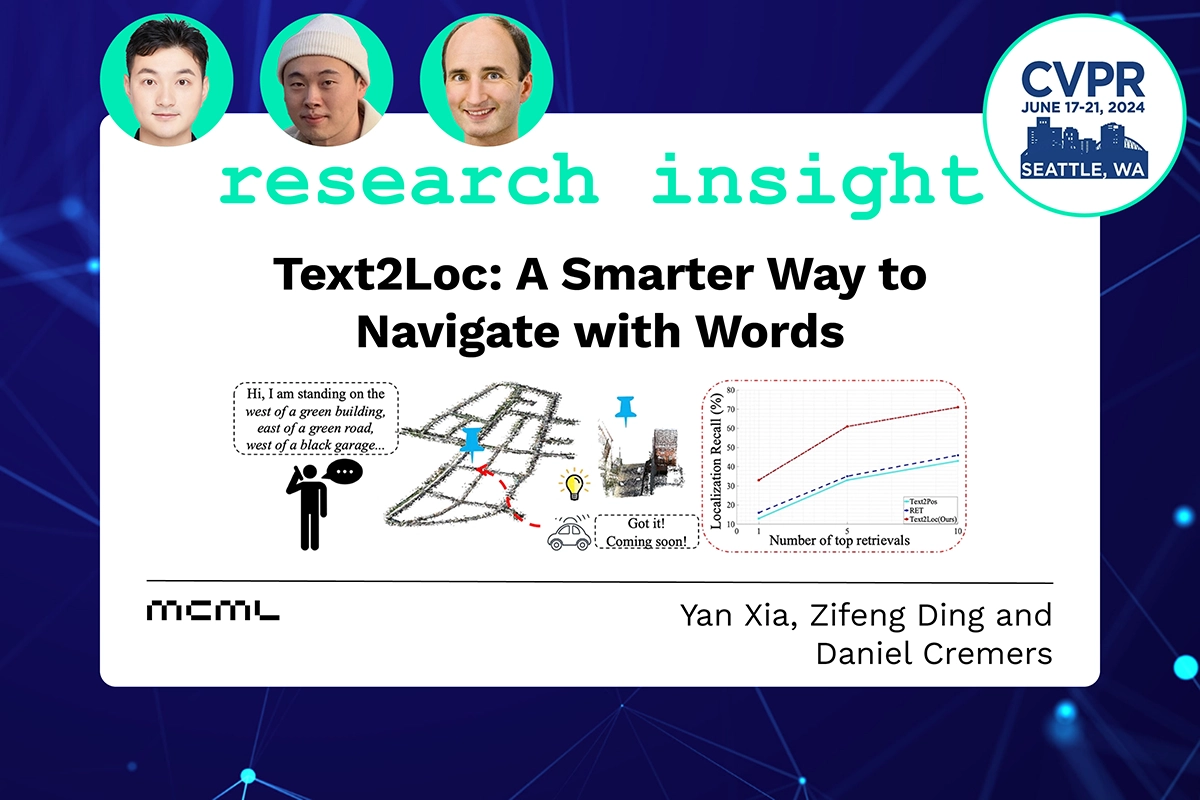
10.04.2025
Text2Loc: A Smarter Way to Navigate With Words
The team of MCML Director Daniel Cremers introduce Text2Loc, a solution designed for city-scale position localization using textual descriptions.
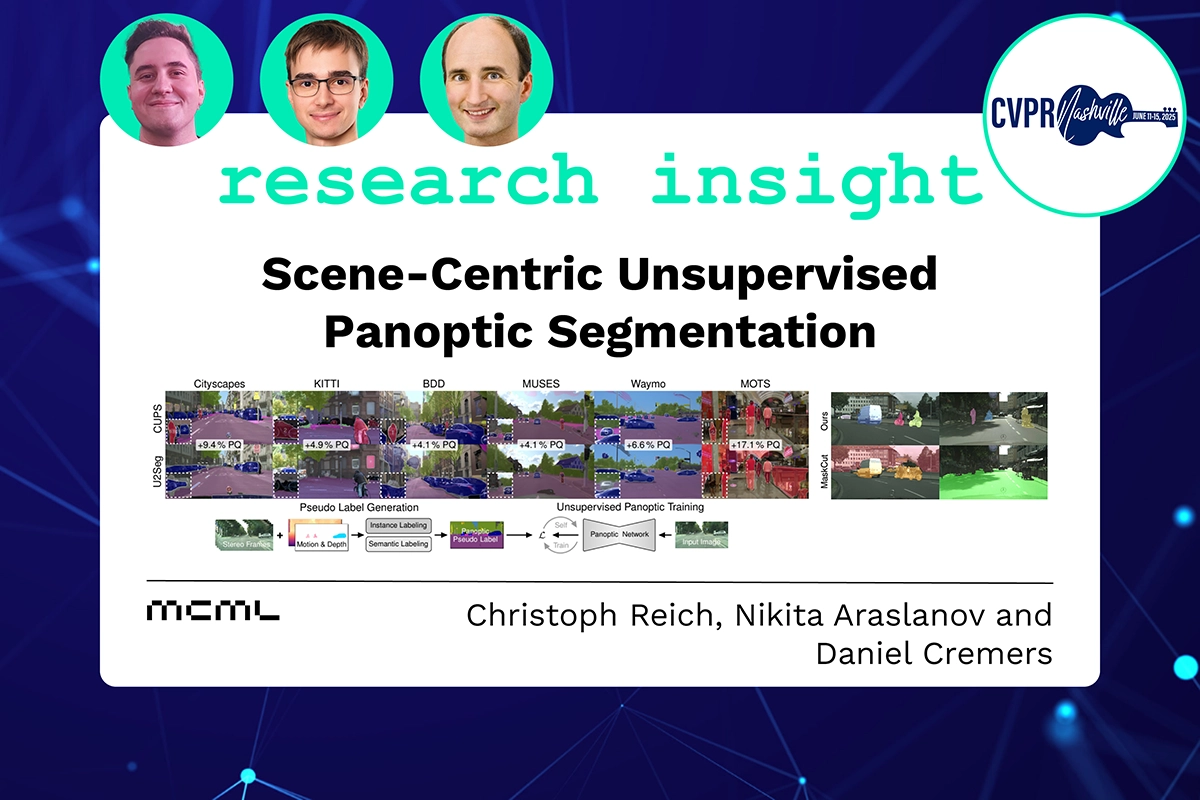
03.04.2025
CUPS: Teaching AI to Understand Scenes Without Human Labels
The team of MCML Director Daniel Cremers introduces CUPS, proving that AI can learn to understand entire scenes - without any human labels.
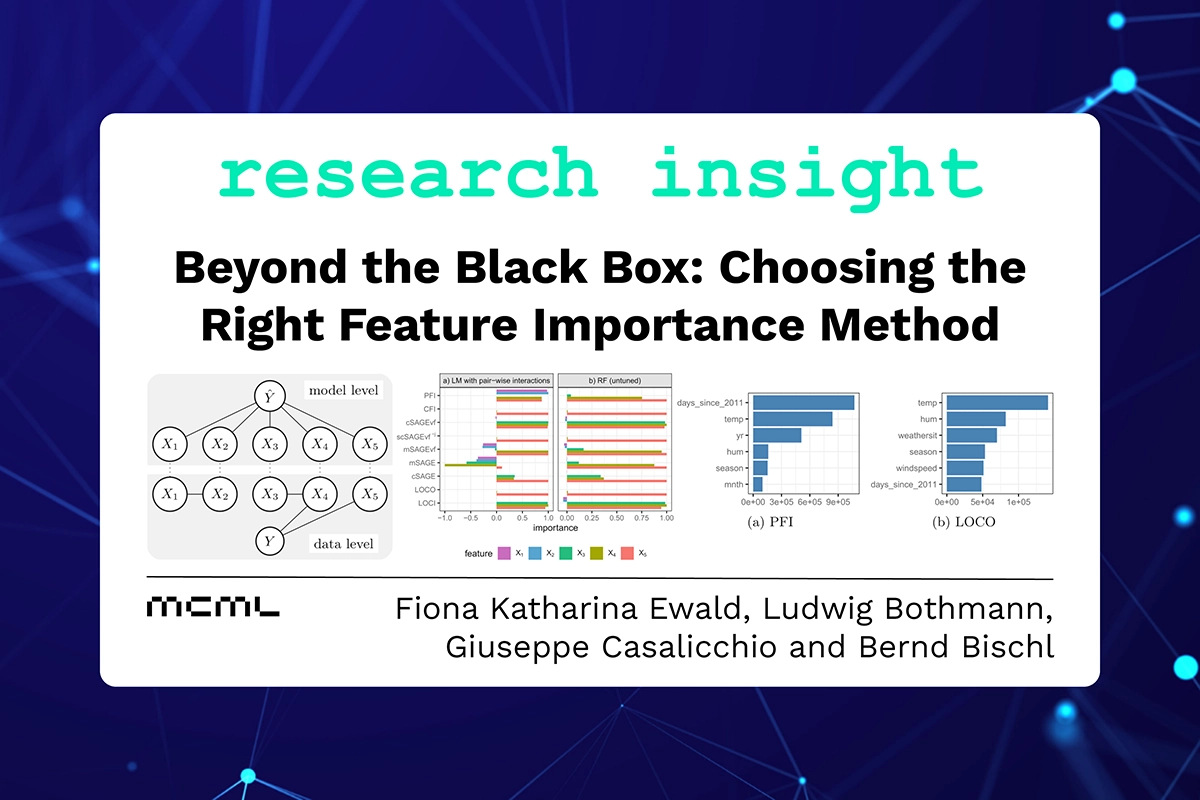
27.03.2025
Beyond the Black Box: Choosing the Right Feature Importance Method
The team of Bernd Bischl created a clear guide to feature importance methods, helping researchers and practitioners interpret AI models effectively.
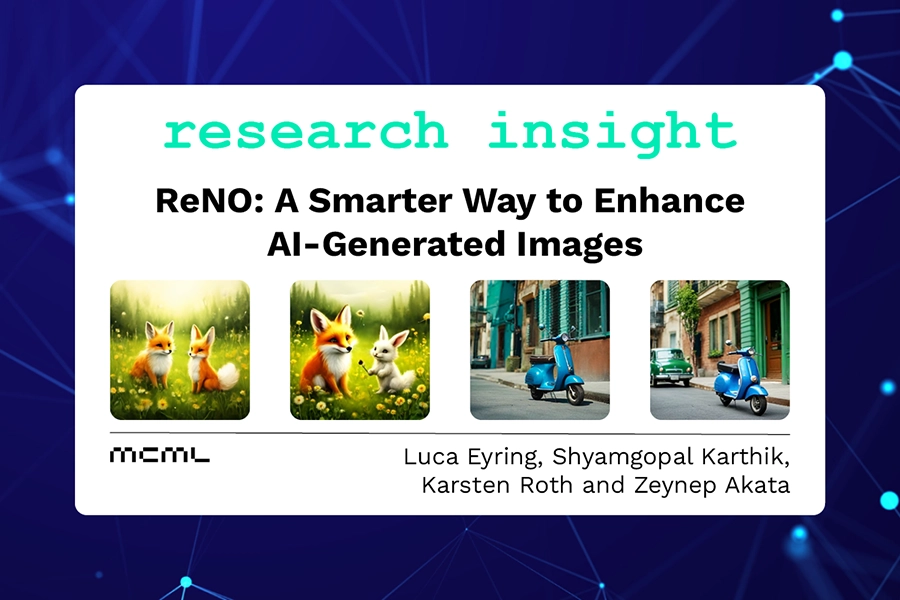
13.03.2025
ReNO: A Smarter Way to Enhance AI-Generated Images
The team of MCML PI Zeynep Akata developed ReNO that enhances image generation without requiring expensive model retraining.