28.04.2025
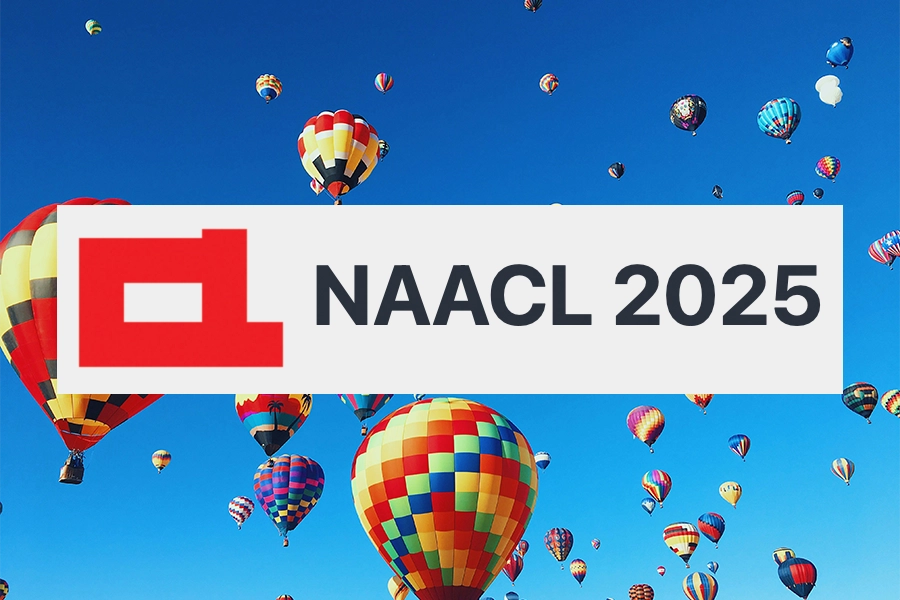
MCML Researchers With Eleven Papers at NAACL 2025
Annual Conference of the North American Chapter of the Association for Computational Linguistics (NAACL 2025). Albuquerque, NM, USA, 29.04.2025–04.05.2024
We are happy to announce that MCML researchers are represented with eleven papers at NAACL 2025. Congrats to our researchers!
Main Track (9 papers)
Fine-Grained Transfer Learning for Harmful Content Detection through Label-Specific Soft Prompt Tuning.
NAACL 2025 - Annual Conference of the North American Chapter of the Association for Computational Linguistics. Albuquerque, NM, USA, Apr 29-May 04, 2025. DOI
Abstract
The spread of harmful content online is a dynamic issue evolving over time. Existing detection models, reliant on static data, are becoming less effective and generalizable. Developing new models requires sufficient up-to-date data, which is challenging. A potential solution is to combine existing datasets with minimal new data. However, detection tasks vary—some focus on hate speech, offensive, or abusive content, which differ in the intent to harm, while others focus on identifying targets of harmful speech such as racism, sexism, etc—raising the challenge of handling nuanced class differences. To address these issues, we introduce a novel transfer learning method that leverages class-specific knowledge to enhance harmful
content detection. In our approach, we first present label-specific soft prompt tuning, which captures and represents class-level information. Secondly, we propose two approaches to transfer this fine-grained knowledge from source (existing tasks) to target (unseen and new tasks): initializing the target task prompts from source prompts and using an attention mechanism that learns and adjusts attention scores to utilize the most relevant information from source prompts. Experiments demonstrate significant improvements in harmful content detection across English and German datasets, highlighting the effectiveness of label-specific representations and knowledge transfer.
MCML Authors
Data Analytics & Statistics
Beyond Literal Token Overlap: Token Alignability for Multilinguality.
NAACL 2025 - Annual Conference of the North American Chapter of the Association for Computational Linguistics. Albuquerque, NM, USA, Apr 29-May 04, 2025. DOI
Abstract
Previous work has considered token overlap, or even similarity of token distributions, as predictors for multilinguality and cross-lingual knowledge transfer in language models. However, these very literal metrics assign large distances to language pairs with different scripts, which can nevertheless show good cross-linguality. This limits the explanatory strength of token overlap for knowledge transfer between language pairs that use distinct scripts or follow different orthographic conventions. In this paper, we propose subword token alignability as a new way to understand the impact and quality of multilingual tokenisation. In particular, this metric predicts multilinguality much better when scripts are disparate and the overlap of literal tokens is low. We analyse this metric in the context of both encoder and decoder models, look at data size as a potential distractor, and discuss how this insight may be applied to multilingual tokenisation in future work. We recommend our subword token alignability metric for identifying optimal language pairs for cross-lingual transfer, as well as to guide the construction of better multilingual tokenisers in the future. We publish our code and reproducibility details.
MCML Authors
Data Analytics & Statistics
A Recipe of Parallel Corpora Exploitation for Multilingual Large Language Models.
NAACL 2025 - Findings of Annual Conference of the North American Chapter of the Association for Computational Linguistics. Albuquerque, NM, USA, Apr 29-May 04, 2025. DOI
Abstract
Recent studies have highlighted the potential of exploiting parallel corpora to enhance multilingual large language models, improving performance in both bilingual tasks, e.g., machine translation, and general-purpose tasks, e.g., text classification. Building upon these findings, our comprehensive study aims to identify the most effective strategies for leveraging parallel corpora. We investigate the impact of parallel corpora quality and quantity, training objectives, and model size on the performance of multilingual large language models enhanced with parallel corpora across diverse languages and tasks. Our analysis reveals several key insights: (i) filtering noisy translations is essential for effectively exploiting parallel corpora, while language identification and short sentence filtering have little effect; (ii) even a corpus containing just 10K parallel sentences can yield results comparable to those obtained from much larger datasets; (iii) employing only the machine translation objective yields the best results among various training objectives and their combinations; (iv) larger multilingual language models benefit more from parallel corpora than smaller models due to their stronger capacity for cross-task transfer. Our study offers valuable insights into the optimal utilization of parallel corpora to enhance multilingual large language models, extending the generalizability of previous findings from limited languages and tasks to a broader range of scenarios.
MCML Authors
XAMPLER: Learning to Retrieve Cross-Lingual In-Context Examples.
NAACL 2025 - Findings of Annual Conference of the North American Chapter of the Association for Computational Linguistics. Albuquerque, NM, USA, Apr 29-May 04, 2025. DOI GitHub
Abstract
Recent studies indicate that leveraging off-the-shelf or fine-tuned retrievers, capable of retrieving relevant in-context examples tailored to the input query, enhances few-shot in-context learning of English. However, adapting these methods to other languages, especially low-resource ones, poses challenges due to the scarcity of cross-lingual retrievers and annotated data. Thus, we introduce XAMPLER: Cross-Lingual Example Retrieval, a method tailored to tackle the challenge of cross-lingual in-context learning using only annotated English data. XAMPLER first trains a retriever based on Glot500, a multilingual small language model, using positive and negative English examples constructed from the predictions of a multilingual large language model, i.e., MaLA500. Leveraging the cross-lingual capacity of the retriever, it can directly retrieve English examples as few-shot examples for in-context learning of target languages. Experiments on the multilingual text classification benchmark SIB200 with 176 languages show that XAMPLER substantially improves the in-context learning performance across languages.
MCML Authors
Lost in Inference: Rediscovering the Role of Natural Language Inference for Large Language Models.
NAACL 2025 - Annual Conference of the North American Chapter of the Association for Computational Linguistics. Albuquerque, NM, USA, Apr 29-May 04, 2025. To be published. Preprint available. arXiv
Abstract
In the recent past, a popular way of evaluating natural language understanding (NLU), was to consider a model’s ability to perform natural language inference (NLI) tasks. In this paper, we investigate if NLI tasks, that are rarely used for LLM evaluation, can still be informative for evaluating LLMs. Focusing on five different NLI benchmarks across six models of different scales, we investigate if they are able to discriminate models of different size and quality and how their accuracies develop during training. Furthermore, we investigate the extent to which the softmax distributions of models align with human distributions in cases where statements are ambiguous or vague. Overall, our results paint a positive picture for the NLI tasks: we find that they are able to discriminate well between models at various stages of training, yet are not (all) saturated. Furthermore, we find that while the similarity of model distributions with human label distributions increases with scale, it is still much higher than the similarity between two populations of humans, making it a potentially interesting statistic to consider.
MCML Authors
Taxi1500: A Dataset for Multilingual Text Classification in 1500 Languages.
NAACL 2025 - Annual Conference of the North American Chapter of the Association for Computational Linguistics. Albuquerque, NM, USA, Apr 29-May 04, 2025. URL
Abstract
While natural language processing tools have been developed extensively for some of the world’s languages, a significant portion of the world’s over 7000 languages are still neglected. One reason for this is that evaluation datasets do not yet cover a wide range of languages, including low-resource and endangered ones. We aim to address this issue by creating a text classification dataset encompassing a large number of languages, many of which currently have little to no annotated data available. We leverage parallel translations of the Bible to construct such a dataset by first developing applicable topics and employing a crowdsourcing tool to collect annotated data. By annotating the English side of the data and projecting the labels onto other languages through aligned verses, we generate text classification datasets for more than 1500 languages. We extensively benchmark several existing multilingual language models using our dataset. To facilitate the advancement of research in this area, we will release our dataset and code.
MCML Authors
Modern Models, Medieval Texts: A POS Tagging Study of Old Occitan.
NAACL 2025 - Annual Conference of the North American Chapter of the Association for Computational Linguistics. Albuquerque, NM, USA, Apr 29-May 04, 2025. To be published. Preprint available. arXiv
Abstract
Large language models (LLMs) have demonstrated remarkable capabilities in natural language processing, yet their effectiveness in handling historical languages remains largely unexplored. This study examines the performance of open-source LLMs in part-of-speech (POS) tagging for Old Occitan, a historical language characterized by non-standardized orthography and significant diachronic variation. Through comparative analysis of two distinct corpora-hagiographical and medical texts-we evaluate how current models handle the inherent challenges of processing a low-resource historical language. Our findings demonstrate critical limitations in LLM performance when confronted with extreme orthographic and syntactic variability. We provide detailed error analysis and specific recommendations for improving model performance in historical language processing. This research advances our understanding of LLM capabilities in challenging linguistic contexts while offering practical insights for both computational linguistics and historical language studies.
MCML Authors
Statistical Learning and Data Science
Statistical Learning and Data Science
Dialetto, ma Quanto Dialetto? Transcribing and Evaluating Dialects on a Continuum.
NAACL 2025 - Annual Conference of the North American Chapter of the Association for Computational Linguistics. Albuquerque, NM, USA, Apr 29-May 04, 2025. To be published. Preprint available.
Abstract
There is increasing interest in looking at dialects in NLP. However, most work to date still treats dialects as discrete categories. For instance, evaluative work in variation-oriented NLP for English often works with Indian English or African-American Venacular English as homogeneous categories (Faisal et al., 2024; Ziems et al., 2023), yet even within one variety there is substantial variation. We examine within-dialect variation and show that performance critically varies within categories. We measure speech-to-text performance on Italian dialects, and empirically observe a geographical performance disparity. This disparity correlates substantially (-0.5) with linguistic similarity to the highest performing dialect variety. We cross-examine our results against dialectometry methods, and interpret the performance disparity to be due to a bias towards dialects that are more similar to the standard variety in the speech-to-text model examined. We additionally leverage geostatistical methods to predict zero-shot performance at unseen sites, and find the incorporation of geographical information to substantially improve prediction performance, indicating there to be geographical structure in the performance distribution.
MCML Authors
Adaptive Prompting: Ad-hoc Prompt Composition for Social Bias Detection.
NAACL 2025 - Annual Conference of the North American Chapter of the Association for Computational Linguistics. Albuquerque, NM, USA, Apr 29-May 04, 2025. DOI
Abstract
Recent advances on instruction fine-tuning have led to the development of various prompting techniques for large language models, such as explicit reasoning steps. However, the success of techniques depends on various parameters, such as the task, language model, and context provided. Finding an effective prompt is, therefore, often a trial-and-error process. Most existing approaches to automatic prompting aim to optimize individual techniques instead of compositions of techniques and their dependence on the input. To fill this gap, we propose an adaptive prompting approach that predicts the optimal prompt composition ad-hoc for a given input. We apply our approach to social bias detection, a highly context-dependent task that requires semantic understanding. We evaluate it with three large language models on three datasets, comparing compositions to individual techniques and other baselines. The results underline the importance of finding an effective prompt composition. Our approach robustly ensures high detection performance, and is best in several settings. Moreover, first experiments on other tasks support its generalizability.
MCML Authors
Artificial Intelligence and Machine Learning
Workshops (2 papers)
Privacy-Preserving Federated Learning for Hate Speech Detection.
SRW @NAACL 2025 - Student Research Workshop at the Annual Conference of the North American Chapter of the Association for Computational Linguistics (NAACL 2025). Albuquerque, NM, USA, Apr 29-May 04, 2025. DOI
Abstract
This paper presents a federated learning system with differential privacy for hate speech detection, tailored to low-resource languages. By fine-tuning pre-trained language models, ALBERT emerged as the most effective option for balancing performance and privacy. Experiments demonstrated that federated learning with differential privacy performs adequately in low-resource settings, though datasets with fewer than 20 sentences per client struggled due to excessive noise. Balanced datasets and augmenting hateful data with non-hateful examples proved critical for improving model utility. These findings offer a scalable and privacy-conscious framework for integrating hate speech detection into social media platforms and browsers, safeguarding user privacy while addressing online harm.
MCML Authors
Computational Linguistics
Can Large Language Models Advance Crosswalks? The Case of Danish Occupation Codes.
SRW @NAACL 2025 - Student Research Workshop at the Annual Conference of the North American Chapter of the Association for Computational Linguistics (NAACL 2025). Albuquerque, NM, USA, Apr 29-May 04, 2025. URL
Abstract
Crosswalks, which map one classification system to another, are critical tools for harmonizing data across time, countries, or frameworks. However, constructing crosswalks is labor-intensive and often requires domain expertise. This paper investigates the potential of Large Language Models (LLMs) to assist in creating crosswalks, focusing on two Danish occupational classification systems from different time periods as a case study. We propose a two-stage, prompt-based framework for this task, where LLMs perform similarity assessments between classification codes and identify final mappings through a guided decision process. Using four instruction-tuned LLMs and comparing them against an embedding-based baseline, we evaluate the performance of different models in crosswalks. Our results highlight the strengths of LLMs in crosswalk creation compared to the embedding-based baseline, showing the effectiveness of the interactive prompt-based framework for conducting crosswalks by LLMs. Furthermore, we analyze the impact of model combinations across two interactive rounds, highlighting the importance of model selection and consistency. This work contributes to the growing field of NLP applications for domain-specific knowledge mapping and demonstrates the potential of LLMs in advancing crosswalk methodologies.
MCML Authors
Social Data Science and AI
28.04.2025
Related
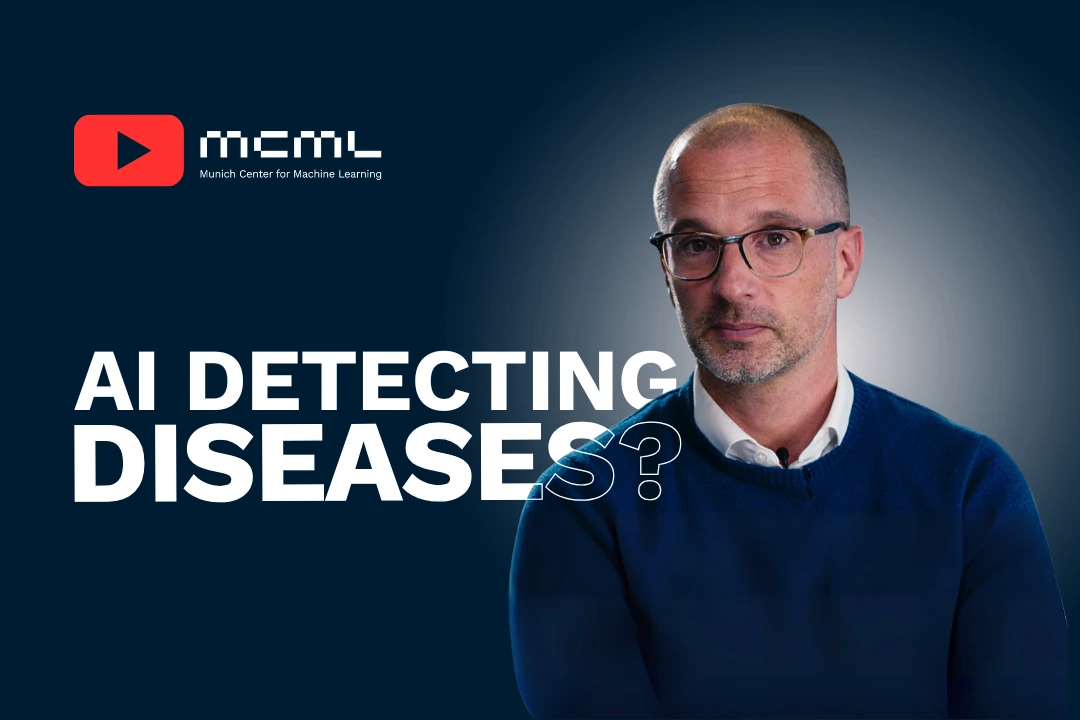
25.06.2025
When Clinical Expertise Meets AI Innovation – With Michael Ingrisch
The new research film features Michael Ingrisch, who shows how AI and clinical expertise can solve real challenges in radiology together.
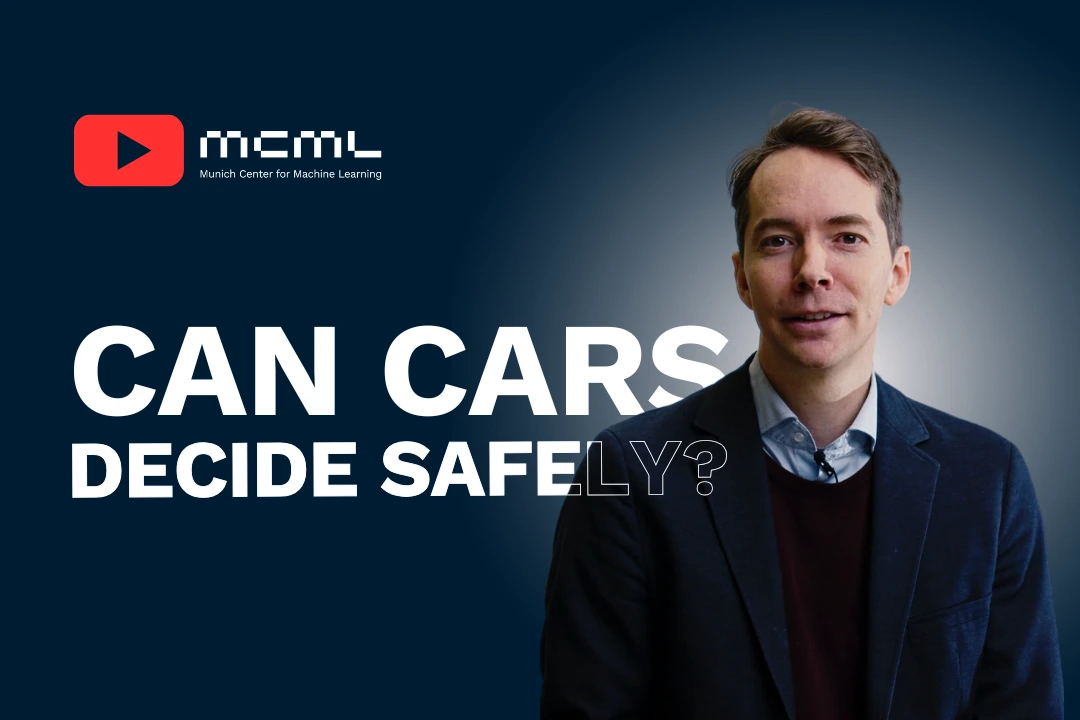
23.06.2025
Autonomous Driving: From Infinite Possibilities to Safe Decisions— With Matthias Althoff
The new research film features Matthias Althoff explaining how his team verifies autonomous vehicle safety using EDGAR and rigorous testing.
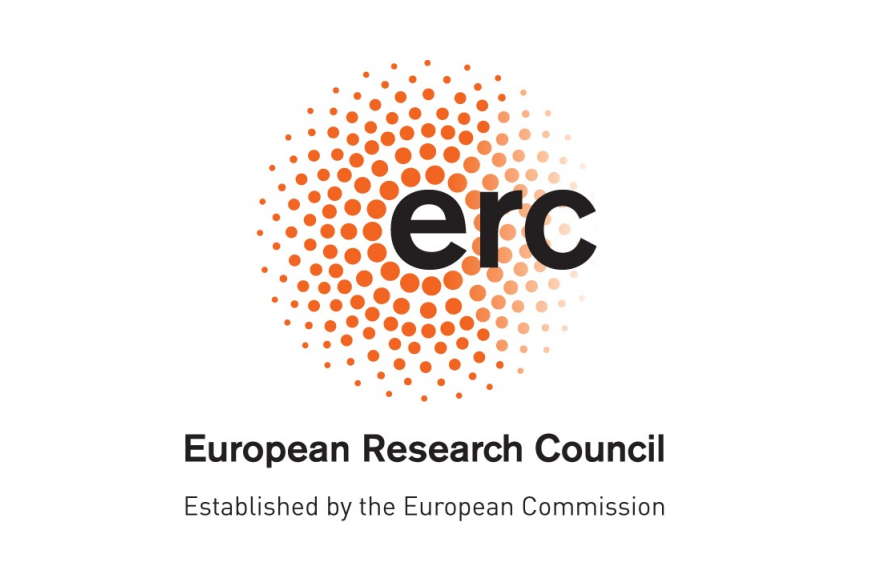
20.06.2025
ERC Advanced Grant for Massimo Fornasier
Massimo Fornasier was awarded ERC Advanced Grant to develop advanced algorithms for solving complex nonconvex optimization problems.
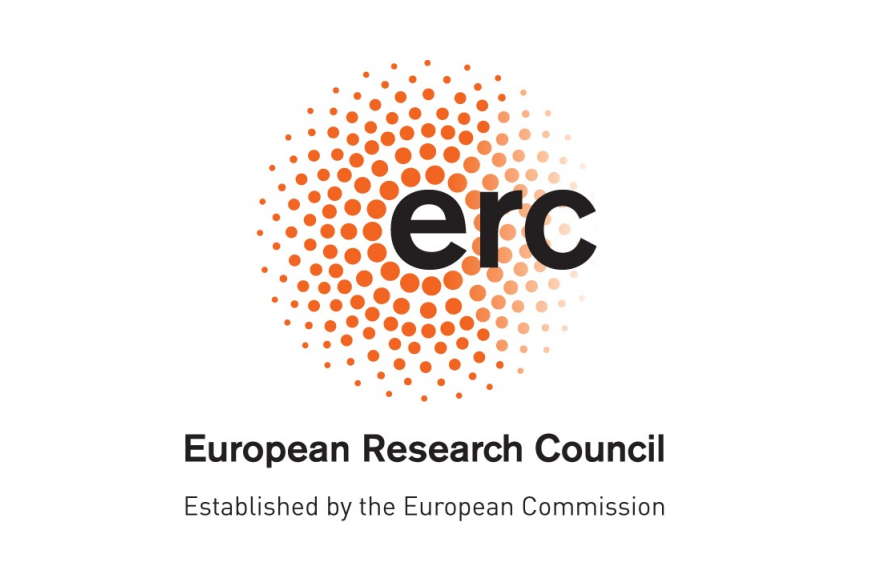
18.06.2025
ERC Advanced Grant for Albrecht Schmidt
Albrecht Schmidt receives ERC Advanced Grant for research on personalized generative AI to support memory, planning, and creativity.