24.02.2025
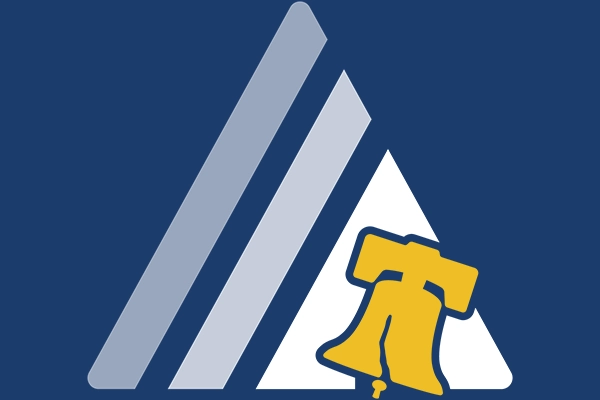
MCML Researchers With Ten Papers at AAAI 2025
39th Conference on Artificial Intelligence (AAAI 2025). Philadelphia, PA, USA, 25.02.2025–04.03.2024
We are happy to announce that MCML researchers are represented with ten papers at AAAI 2025. Congrats to our researchers!
Main Track (10 papers)
FedPop: Federated Population-based Hyperparameter Tuning.
AAAI 2025 - 39th Conference on Artificial Intelligence. Philadelphia, PA, USA, Feb 25-Mar 04, 2025. DOI
Abstract
Federated Learning (FL) is a distributed machine learning (ML) paradigm, in which multiple clients collaboratively train ML models without centralizing their local data. Similar to conventional ML pipelines, the client local optimization and server aggregation procedure in FL are sensitive to the hyperparameter (HP) selection. Despite extensive research on tuning HPs for centralized ML, these methods yield suboptimal results when employed in FL. This is mainly because their ’training-after-tuning’ framework is unsuitable for FL with limited client computation power. While some approaches have been proposed for HP-Tuning in FL, they are limited to the HPs for client local updates. In this work, we propose a novel HP-tuning algorithm, called Federated Population-based Hyperparameter Tuning (FedPop), to address this vital yet challenging problem. FedPop employs population-based evolutionary algorithms to optimize the HPs, which accommodates various HP types at both the client and server sides. Compared with prior tuning methods, FedPop employs an online ’tuning-while-training’ framework, offering computational efficiency and enabling the exploration of a broader HP search space. Our empirical validation on the common FL benchmarks and complex real-world FL datasets, including full-sized Non-IID ImageNet-1K, demonstrates the effectiveness of the proposed method, which substantially outperforms the concurrent state-of-the-art HP-tuning methods in FL.
MCML Authors
CAGE: Unsupervised Visual Composition and Animation for Controllable Video Generation.
AAAI 2025 - 39th Conference on Artificial Intelligence. Philadelphia, PA, USA, Feb 25-Mar 04, 2025. DOI GitHub
Abstract
In this work we propose a novel method for unsupervised controllable video generation. Once trained on a dataset of unannotated videos, at inference our model is capable of both composing scenes of predefined object parts and animating them in a plausible and controlled way. This is achieved by conditioning video generation on a randomly selected subset of local pre-trained self-supervised features during training. We call our model CAGE for visual Composition and Animation for video GEneration. We conduct a series of experiments to demonstrate capabilities of CAGE in various settings.
MCML Authors
DUO: Diverse, Uncertain, On-Policy Query Generation and Selection for Reinforcement Learning from Human Feedback.
AAAI 2025 - 39th Conference on Artificial Intelligence. Philadelphia, PA, USA, Feb 25-Mar 04, 2025. DOI
Abstract
Defining a reward function is usually a challenging but critical task for the system designer in reinforcement learning, especially when specifying complex behaviors. Reinforcement learning from human feedback (RLHF) emerges as a promising approach to circumvent this. In RLHF, the agent typically learns a reward function by querying a human teacher using pairwise comparisons of trajectory segments. A key question in this domain is how to reduce the number of queries necessary to learn an informative reward function since asking a human teacher too many queries is impractical and costly. To tackle this question, we propose DUO, a novel method for diverse, uncertain, on-policy query generation and selection in RLHF. Our method produces queries that are (1) more relevant for policy training (via an on-policy criterion), (2) more informative (via a principled measure of epistemic uncertainty), and (3) diverse (via a clustering-based filter). Experimental results on a variety of locomotion and robotic manipulation tasks demonstrate that our method can outperform state-of-the-art RLHF methods given the same total budget of queries, while being robust to possibly irrational teachers.
MCML Authors
Artificial Intelligence and Machine Learning
DepthFM: Fast Generative Monocular Depth Estimation with Flow Matching.
AAAI 2025 - 39th Conference on Artificial Intelligence. Philadelphia, PA, USA, Feb 25-Mar 04, 2025. Oral Presentation. DOI
Abstract
Current discriminative depth estimation methods often produce blurry artifacts, while generative approaches suffer from slow sampling due to curvatures in the noise-to-depth transport. Our method addresses these challenges by framing depth estimation as a direct transport between image and depth distributions. We are the first to explore flow matching in this field, and we demonstrate that its interpolation trajectories enhance both training and sampling efficiency while preserving high performance. While generative models typically require extensive training data, we mitigate this dependency by integrating external knowledge from a pre-trained image diffusion model, enabling effective transfer even across differing objectives. To further boost our model performance, we employ synthetic data and utilize image-depth pairs generated by a discriminative model on an in-the-wild image dataset. As a generative model, our model can reliably estimate depth confidence, which provides an additional advantage. Our approach achieves competitive zero-shot performance on standard benchmarks of complex natural scenes while improving sampling efficiency and only requiring minimal synthetic data for training.
MCML Authors
Mind the Uncertainty in Human Disagreement: Evaluating Discrepancies between Model Predictions and Human Responses in VQA.
AAAI 2025 - 39th Conference on Artificial Intelligence. Philadelphia, PA, USA, Feb 25-Mar 04, 2025. DOI
Abstract
Large vision-language models struggle to accurately predict responses provided by multiple human annotators, particularly when those responses exhibit high uncertainty. In this study, we focus on a Visual Question Answering (VQA) task and comprehensively evaluate how well the output of the state-of-the-art vision-language model correlates with the distribution of human responses. To do so, we categorize our samples based on their levels (low, medium, high) of human uncertainty in disagreement (HUD) and employ, not only accuracy, but also three new human-correlated metrics for the first time in VQA, to investigate the impact of HUD. We also verify the effect of common calibration and human calibration (Baan et al. 2022) on the alignment of models and humans. Our results show that even BEiT3, currently the best model for this task, struggles to capture the multi-label distribution inherent in diverse human responses. Additionally, we observe that the commonly used accuracy-oriented calibration technique adversely affects BEiT3’s ability to capture HUD, further widening the gap between model predictions and human distributions. In contrast, we show the benefits of calibrating models towards human distributions for VQA, to better align model confidence with human uncertainty. Our findings highlight that for VQA, the alignment between human responses and model predictions is understudied and is an important target for future studies.
MCML Authors
Whole Genome Transformer for Gene Interaction Effects in Microbiome Habitat Specificity.
AAAI 2025 - 39th Conference on Artificial Intelligence. Philadelphia, PA, USA, Feb 25-Mar 04, 2025. DOI
Abstract
Leveraging the vast genetic diversity within microbiomes offers unparalleled insights into complex phenotypes, yet the task of accurately predicting and understanding such traits from genomic data remains challenging. We propose a framework taking advantage of existing large models for gene vectorization to predict habitat specificity from entire microbial genome sequences. Based on our model, we develop attribution techniques to elucidate gene interaction effects that drive microbial adaptation to diverse environments. We train and validate our approach on a large dataset of high quality microbiome genomes from different habitats. We not only demonstrate solid predictive performance, but also how sequence-level information of entire genomes allows us to identify gene associations underlying complex phenotypes. Our attribution recovers known important interaction networks and proposes new candidates for experimental follow up.
MCML Authors
Ethics in Systems Design and Machine Learning
Does VLM Classification Benefit from LLM Description Semantics?
AAAI 2025 - 39th Conference on Artificial Intelligence. Philadelphia, PA, USA, Feb 25-Mar 04, 2025. Invited talk. DOI
Abstract
Accurately describing images with text is a foundation of explainable AI. Vision-Language Models (VLMs) like CLIP have recently addressed this by aligning images and texts in a shared embedding space, expressing semantic similarities between vision and language embeddings. VLM classification can be improved with descriptions generated by Large Language Models (LLMs). However, it is difficult to determine the contribution of actual description semantics, as the performance gain may also stem from a semantic-agnostic ensembling effect, where multiple modified text prompts act as a noisy test-time augmentation for the original one. We propose an alternative evaluation scenario to decide if a performance boost of LLM-generated descriptions is caused by such a noise augmentation effect or rather by genuine description semantics. The proposed scenario avoids noisy test-time augmentation and ensures that genuine, distinctive descriptions cause the performance boost. Furthermore, we propose a training-free method for selecting discriminative descriptions that work independently of classname-ensembling effects. Our approach identifies descriptions that effectively differentiate classes within a local CLIP label neighborhood, improving classification accuracy across seven datasets. Additionally, we provide insights into the explainability of description-based image classification with VLMs.
MCML Authors
MPTSNet: Integrating Multiscale Periodic Local Patterns and Global Dependencies for Multivariate Time Series Classification.
AAAI 2025 - 39th Conference on Artificial Intelligence. Philadelphia, PA, USA, Feb 25-Mar 04, 2025. DOI
Abstract
Multivariate Time Series Classification (MTSC) is crucial in extensive practical applications, such as environmental monitoring, medical EEG analysis, and action recognition. Real-world time series datasets typically exhibit complex dynamics. To capture this complexity, RNN-based, CNN-based, Transformer-based, and hybrid models have been proposed. Unfortunately, current deep learning-based methods often neglect the simultaneous construction of local features and global dependencies at different time scales, lacking sufficient feature extraction capabilities to achieve satisfactory classification accuracy. To address these challenges, we propose a novel Multiscale Periodic Time Series Network (MPTSNet), which integrates multiscale local patterns and global correlations to fully exploit the inherent information in time series. Recognizing the multi-periodicity and complex variable correlations in time series, we use the Fourier transform to extract primary periods, enabling us to decompose data into multiscale periodic segments. Leveraging the inherent strengths of CNN and attention mechanism, we introduce the PeriodicBlock, which adaptively captures local patterns and global dependencies while offering enhanced interpretability through attention integration across different periodic scales. The experiments on UEA benchmark datasets demonstrate that the proposed MPTSNet outperforms 21 existing advanced baselines in the MTSC tasks.
MCML Authors
Medical Multimodal Model Stealing Attacks via Adversarial Domain Alignment.
AAAI 2025 - 39th Conference on Artificial Intelligence. Philadelphia, PA, USA, Feb 25-Mar 04, 2025. DOI
Abstract
Medical multimodal large language models (MLLMs) are becoming an instrumental part of healthcare systems, assisting medical personnel with decision making and results analysis. Models for radiology report generation are able to interpret medical imagery, thus reducing the workload of radiologists. As medical data is scarce and protected by privacy regulations, medical MLLMs represent valuable intellectual property. However, these assets are potentially vulnerable to model stealing, where attackers aim to replicate their functionality via black-box access. So far, model stealing for the medical domain has focused on classification; however, existing attacks are not effective against MLLMs. In this paper, we introduce Adversarial Domain Alignment (ADA-STEAL), the first stealing attack against medical MLLMs. ADA-STEAL relies on natural images, which are public and widely available, as opposed to their medical counterparts. We show that data augmentation with adversarial noise is sufficient to overcome the data distribution gap between natural images and the domain-specific distribution of the victim MLLM. Experiments on the IU X-RAY and MIMIC-CXR radiology datasets demonstrate that Adversarial Domain Alignment enables attackers to steal the medical MLLM without any access to medical data.
MCML Authors
WebPilot: A Versatile and Autonomous Multi-Agent System for Web Task Execution with Strategic Exploration.
AAAI 2025 - 39th Conference on Artificial Intelligence. Philadelphia, PA, USA, Feb 25-Mar 04, 2025. DOI
Abstract
LLM-based autonomous agents often fail to execute complex web tasks that require dynamic interaction due to the inherent uncertainty and complexity of these environments. Existing LLM-based web agents typically rely on rigid, expert-designed policies specific to certain states and actions, which lack the flexibility and generalizability needed to adapt to unseen tasks. In contrast, humans excel by exploring unknowns, continuously adapting strategies, and resolving ambiguities through exploration. To emulate human-like adaptability, web agents need strategic exploration and complex decision-making. Monte Carlo Tree Search (MCTS) is well-suited for this, but classical MCTS struggles with vast action spaces, unpredictable state transitions, and incomplete information in web tasks. In light of this, we develop WebPilot, a multi-agent system with a dual optimization strategy that improves MCTS to better handle complex web environments. Specifically, the Global Optimization phase involves generating a high-level plan by breaking down tasks into manageable subtasks and continuously refining this plan, thereby focusing the search process and mitigating the challenges posed by vast action spaces in classical MCTS. Subsequently, the Local Optimization phase executes each subtask using a tailored MCTS designed for complex environments, effectively addressing uncertainties and managing incomplete information. Experimental results on WebArena and MiniWoB++ demonstrate the effectiveness of WebPilot. Notably, on WebArena, WebPilot achieves SOTA performance with GPT-4, achieving a 93% relative increase in success rate over the concurrent tree search-based method. WebPilot marks a significant advancement in general autonomous agent capabilities, paving the way for more advanced and reliable decision-making in practical environments.
MCML Authors
24.02.2025
Related
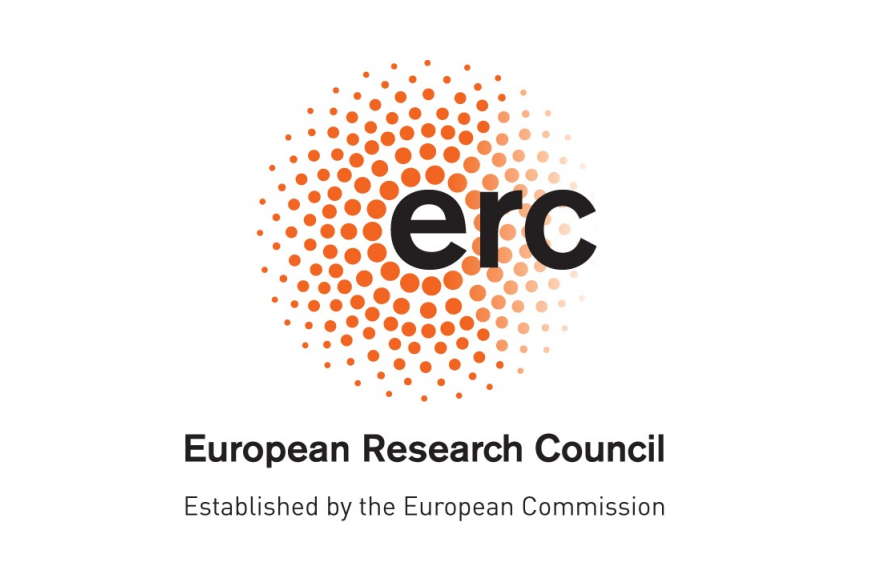
20.06.2025
ERC Advanced Grant for Massimo Fornasier
Massimo Fornasier was awarded ERC Advanced Grant to develop advanced algorithms for solving complex nonconvex optimization problems.
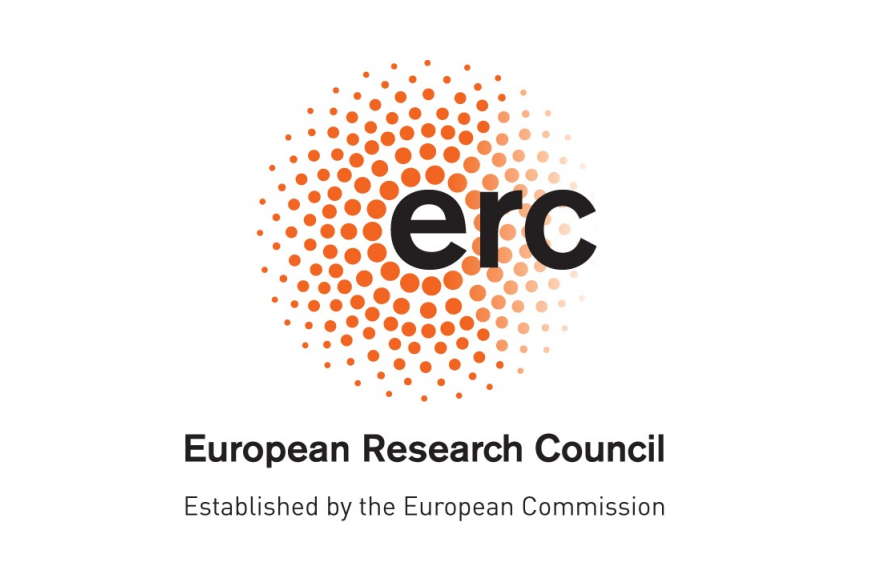
18.06.2025
ERC Advanced Grant for Albrecht Schmidt
Albrecht Schmidt receives ERC Advanced Grant for research on personalized generative AI to support memory, planning, and creativity.
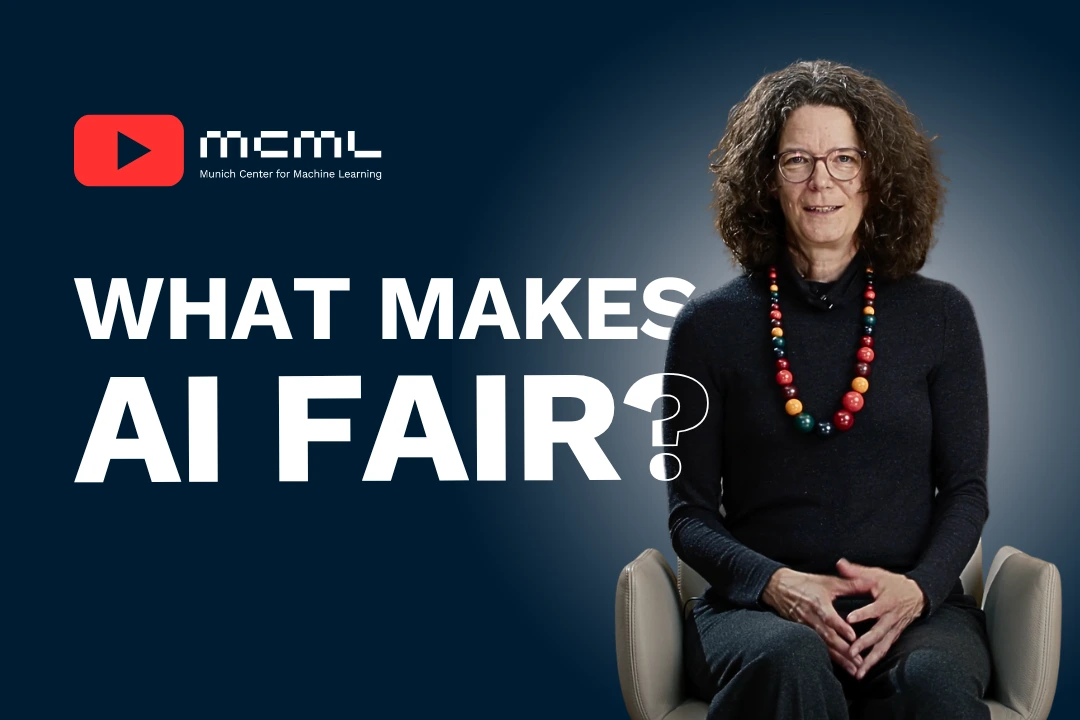
11.06.2025
Better Data, Smarter AI: Why Quality Matters – With Frauke Kreuter
In our new research film, Frauke Kreuter explains how data quality shapes fair, reliable, and socially responsible AI systems.
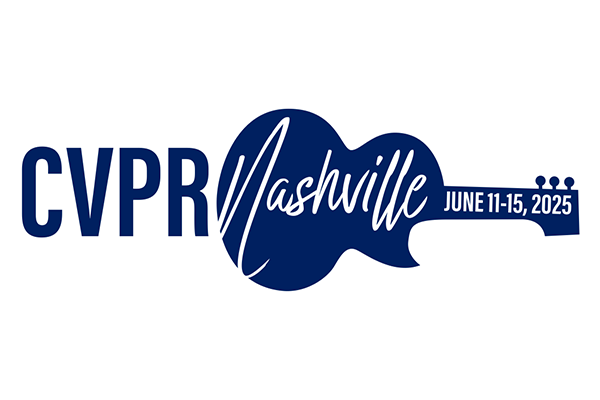
10.06.2025
MCML Researchers With 30 Papers at CVPR 2025
IEEE/CVF Conference on Computer Vision and Pattern Recognition (CVPR 2025). Nashville, TN, USA, 11.06.2025 - 15.06.2025