19.12.2024
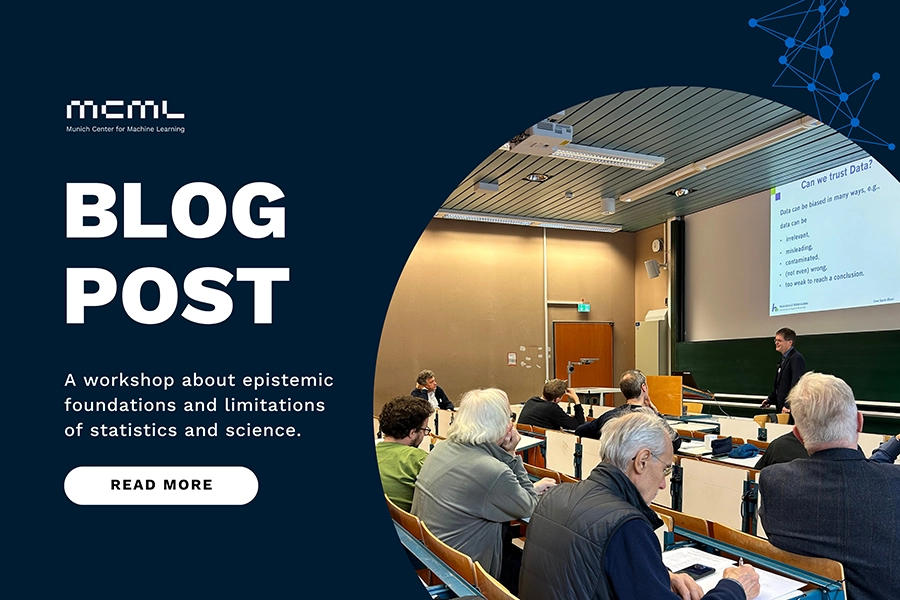
©MCML
Epistemic Foundations and Limitations of Statistics and Science
Blogpost on the Replication Crisis
The Open Science Initiative in Statistics and the MCML recently hosted a workshop about epistemic foundations and limitations of statistics and science. The event brought together researchers from diverse fields to discuss one of science’s most pressing challenges: The replication crisis. While the crisis is often attributed to systemic issues like “publish or perish” incentives, the discussions highlighted an overlooked culprit: a lack of understanding and acknowledgment of the epistemic foundations of statistics. After the workshop, our MCML members Lisa Wimmer, Moritz Herrmann and Patrick Schenk wrote a blog post with their thoughts on the topic.
Statistics suffers from a replication crisis
«The fact that Statistics, as a field, is undergoing a replication crisis might seem puzzling at first.»
Lisa Wimmer
MCML Junior Member
The fact that Statistics, as a field, is undergoing a replication crisis might seem puzzling at first. More applied disciplines like Psychology have been known for producing results that don’t replicate (i.e., prompt the same scientific conclusions). Much of this has been attributed to researchers’ misconception about complex statistical entities, such as the notorious p-value, but surely this can’t be a problem for statisticians themselves? Unfortunately, our field suffers from many of the same issues that have tripped others. For one, the pressure to publish enforces a tendency to emphasize positive results–in the sense of successful methods–while negative results, which are still valuable to the community, remain in the file drawer. A more worrying aspect is that good scientific practice has proven hard to adhere to even with the best of intentions. As the famous physicist Richard Feynmann put it: “The first principle is that you must not fool yourself— and you are the easiest person to fool.” We argue that fields like Statistics and Machine Learning need to revisit epistemic foundations and limitations, educating ourselves and others about the principles of empirical sciences.
Neither big data nor large models are going to solve the crisis
«Neither big data nor large models are going to solve the crisis.»
Lisa Wimmer
MCML Junior Member
The foundations for today’s powerful statistical models were laid in the latter half of the past century. New mathematical insights and a leap in available computing power have brought into existence AI agents that people increasingly look to as their companions. Their dazzling capabilities, however, mask the brittleness of their theoretical underpinnings. Anecdotes of, e.g., ChatGPT hallucinating to give dreadfully wrong answers, or AI turning racist, are abundant. Such undesirable effects occur due to faulty development processes: models overfitting to toy datasets, black-box algorithms picking up spurious patterns and producing surprising outcomes, or an omission to incorporate all relevant sources of uncertainty that inevitably enters the data way before they are used to build models. These examples already hint at the complexity of the endeavor–it is simply very easy to miss relevant aspects and make mistakes at some point along the way.
In this conundrum, some turn to Big Data as the savior of us all. Can’t we create an appropriate representation of the world if we just feed our models enough data? Sadly, the answer is no for two reasons at least. First, a well-established result of learning theory states that there can be no learning without inductive biases, i.e., some assumptions we are willing to make about the nature of the data-generating process (otherwise, we could build one model to rule them all and abolish the field of Statistics altogether). Second, it can be shown that data pooled from multiple sources–as is the case in many instances of Big Data–rarely give rise to a well-defined joint probability distribution. In other words, data cobbled together from different corners of the internet don’t tell a coherent story. This may be exacerbated in the future by the incestuous evolution of training data that is to be expected from addressing the perennial data shortage with AI-generated imitations.
With so many unresolved issues, society risks being carried away on an enthusiastic wave of adopting technological progress when the foundations of this progress remain shaky. All this means that our field must continue to strive for excellence in scientific principles – sometimes this includes taking a step back and thinking about whether we too have been swept into the wrong direction. Science is a cumulative endeavor in which researchers ought to be able to rely on previous results. We can only achieve this by holding ourselves to the highest-possible standards. Otherwise, we’re building a house of cards.
We need clarity about concepts more urgently than procedures and formalism
«What we actually need is conceptual clarity.»
Lisa Wimmer
MCML Junior Member
Alas, scientists (and perhaps statisticians in particular) are prone to get bogged down in discussions about methodological details. What we actually need is conceptual clarity. Take the example of reproducibility. Our field broadly seems to consider computational reproducibility, i.e., the guarantee to produce the exact same numerical results when re-running experiment code, necessary and sufficient to tick off replicability. While computational reproducibility is frequently desirable, making it the sole yardstick falls desperately short of good scientific practice. Program code typically stands at the end of a long succession of design choices. Decisions about research questions (which often conflate exploratory and confirmatory endeavors), model classes, datasets, evaluation criteria, etc. heavily influence the scientific conclusions we can draw. Any two studies about the same research questions must be expected to differ due to assumptions in varying degree of violation alone.
It doesn’t help that scientific progress is often judged by one-dimensional metrics. “When a measure becomes a target, it ceases to be a good measure” has become known as Goodhart’s law. If p-values below 5 % or above-baseline values of accuracy signal scientific quality, it’s not surprising that researchers work towards those indicators more than actual knowledge gain. The abstraction provided by quantitative methods is actually a core virtue of Statistics, with numbers as a lingua franca for people from any scientific (or social, geographical, temporal) background. We need to make sure, however, that quantification doesn’t lead to oversimplification, decontextualization, and measure hacking.
«Statistics and Machine Learning need to revisit epistemic foundations and limitations, educating ourselves and others about the principles of empirical sciences.»
Lisa Wimmer
MCML Junior Member
This is all the more important in our current geopolitical landscape. Contrary to what naive realism or positivism would have us believe, Statistics doesn’t operate in a vacuum devoid of social processes. We have a responsibility to take into account the circumstances under which data have been generated, the personal perspectives shaping our scientific work, and, ultimately, the implications of employing our models in decision-making.
From our discussions we infer a number of opportunities to save our field from the looming replication crisis. Rather than measure-hack our way forward, we should discern more clearly between exploratory research (in which not every idea can be a winner) and confirmatory research (with proper scientific hypotheses). Better infrastructure can further discourage bad practices: well-maintained software and well-curated, well-understood datasets ensure that promising results don’t depend on lucky experimental settings. Besides getting the incentives right, we all need more education–ignorance can’t be an excuse for questionable science. We hope that initiatives like our workshop are steps into the right direction. So, statisticians, roll up your sleeves, there’s a crisis to be solved (freely adapted from Seibold et al., 2021).
Lisa Wimmer
Moritz Herrmann and Patrick Schenk as Co-Organizers of the Workshop
For our article, we drew from discussions and talks in our workshop. We emphasize that the above arguments reflect our own interpretation and not participants’ opinions.
Our speakers and their insightful topics
- Rudolf Seising: An Interwoven History of AI and Statistics
- Uwe Saint-Mont: How Feynman Predicted the Replication Crisis
- Jürgen Landes: Data Aggregation of Big Data Is Not Enough
- Walter Radermacher: Epistemology and Sociology of Quantification Based on Convention Theory
- Sabina Leonelli: What Reproducibility Can’t Solve
- Moritz Herrmann: When Measures Become Targets
- Sabine Hoffmann: How Foundational Assumptions about Probability, Uncertainty, and Subjectivity Jeopardize the Replicability of Research Findings
- Michael Schomaker: Replicability When Considering Unconditional Interpretations and Gradations of Evidence
A list of references from our speakers
- Rudolf Seising (Ed.): Geschichten der Künstlichen Intelligenz in der Bundesrepublik Deutschland [in German]
- Uwe Saint-Mont: Statistik im Forschungsprozess - Eine Philosophie der Statistik als Baustein einer integrativen Wissenschaftstheorie [in German]
- Jürgen Landes (et al.): Objective Bayesian Nets for Integrating Consistent Datasets
- Walter Radermacher: Official Statistics 4.0 - Verified Facts for People in the 21st Century
- Sabina Leonelli: Philosophy of Open Science
- Moritz Herrmann (et al.): Position: Why We Must Rethink Empirical Research in Machine Learning
- Sabine Hoffmann (et al.): A Bayesian hierarchical approach to account for evidence and uncertainty in the modeling of infectious diseases: An application to COVID‐19
- Michael Schomaker (et al.): Introduction to Statistics and Data Analysis - With Exercises, Solutions and Applications in R (Second updated and extended edition)
A far from exhaustive list of further references
- Hybrid theory: Or “Cargo Cult Science” and its “Statistical Rituals”
- Cargo Cult Science (Feynman, 1974)
- Misinterpretations of significance: A problem students share with their teachers (Haller & Krauss, 2002)
- A dirty dozen: Twelve P-value misconceptions (Goodman, 2008)
- Mindless statistics (Gigerenzer, 2004)
- Surrogate Science: The Idol of a Universal Method for Scientific Inference (Gigerenzer & Marewski, 2014)
- Statistical rituals: The replication delusion and how we got there (Gigerenzer, 2018)
- Null hypothesis significance tests. A mix-up of two different theories: the basis for widespread confusion and numerous misinterpretations (Schneider, 2015)
- Connecting simple and precise P-values to complex and ambiguous realities(Greenland, 2023)
- The number of the beast: Or “The earth is round (p < .05)"
- The earth is round (p < .05) (Cohen, 1994)
- The difference between ‘‘significant’’ and ‘‘not significant’’ is not itself statistically significant (Gelman & Stern, 2012)
- The problem with p-values are not just p-values (Gelman, 2016)
- The ASA Statement on p-Values: Context, Process, and Purpose (Wasserstein & Lazar, 2016)
- Moving to a World Beyond “p < 0.05”(Wasserstein, Schirm & Lazar, 2019)
- A new look at p-values for randomized clinical trials (van Zwet et al, 2023)
- Difficult to cure: Or “Significance tests die hard”
- Tests of significance considered as evidence (Berkson, 1942)
- The case against statistical significance testing (Carver, 1978)
- Statistical analysis and the illusion of objectivity (Berger & Berry, 1988)
- The superego, the ego, and the id in statistical reasoning (Gigerenzer, Keren & Lewis, 1993)
- Significance tests die hard: The Amazing Persistence of a Probabilistic Misconception (Falk and Greenbaum, 1997)
- Needed: A ban on the significance test. (Hunter, 1997)
- Scientific versus statistical inference (Dixon & O’Reilly, 1999)
- The p-value Statement, Five Years On (Matthews, 2021)
- By the way: Or “Why most published research findings are false” but “Fair coins tend to land on the same side they started”
- Why most published research findings are false (Ioannidis, 2005)
- A problem in theory (Muthukrishna & Henrich, 2019)
- Understanding the Exploratory/Confirmatory Data Analysis Continuum: Moving Beyond the “Replication Crisis” (Fife and Rogers, 2022)
- Same data, different analysts: variation in effect sizes due to analytical decisions in ecology and evolutionary biology (Gould et al, 2023)
- The many analysts problem in the social sciences: Auspurg & Brüderl (2023, 2021)
- Fair coins tend to land on the same side they started: Evidence from 350,757 flips (Bartoš et al, 2023)
19.12.2024
Related
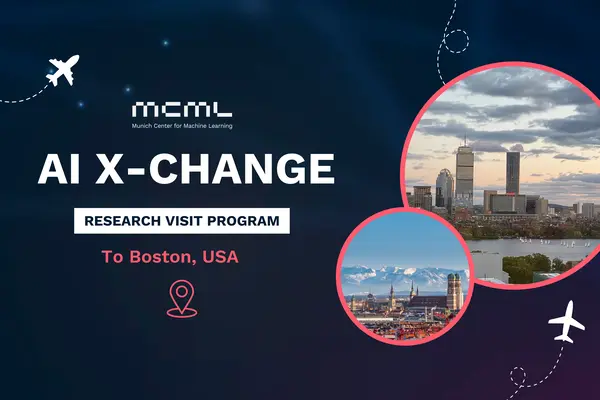
©MCML
04.03.2025
Research at EWCS at the Broad Institute of MIT and Harvard
Research stay at Broad Institute: Exploring causality in biology, microbial interactions, and ML applications through MCML AI X-change program.
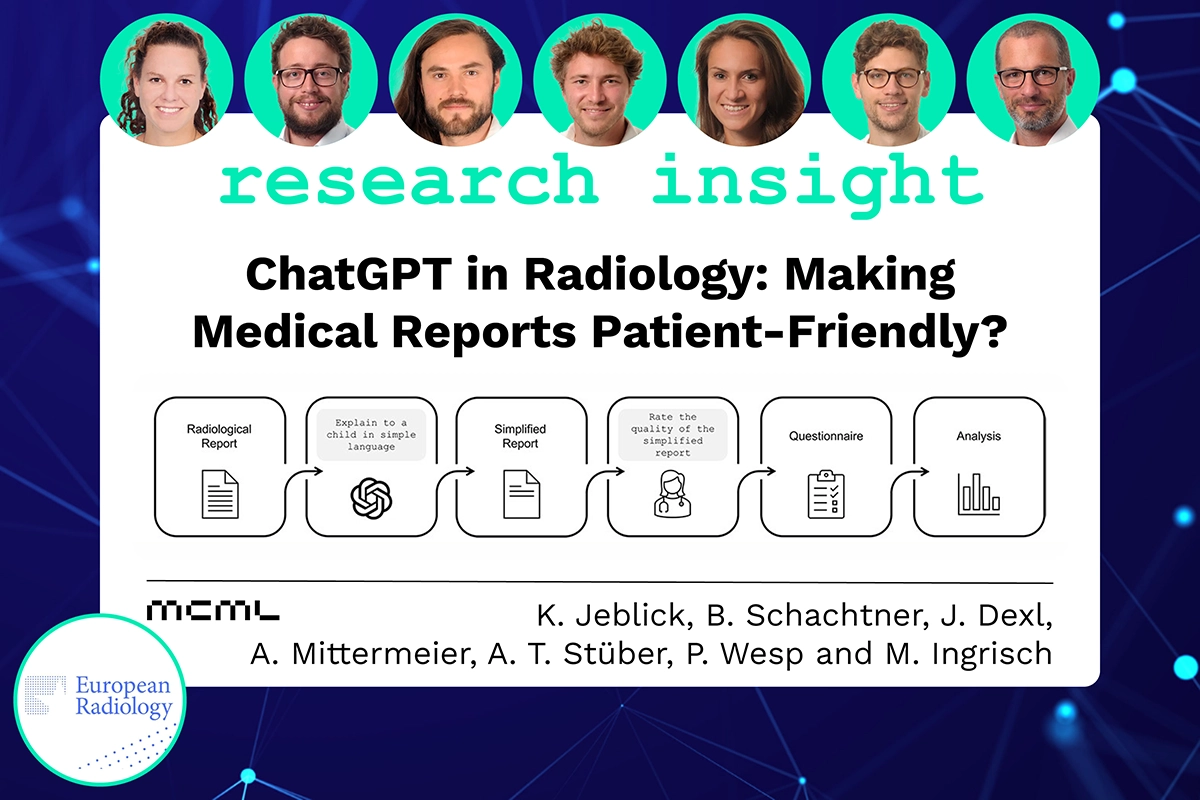
23.02.2025
ChatGPT in Radiology: Making Medical Reports Patient-Friendly?
The team of MCML PI Michael Ingrisch explored whether ChatGPT could effectively simplify radiology reports while preserving factual accuracy.
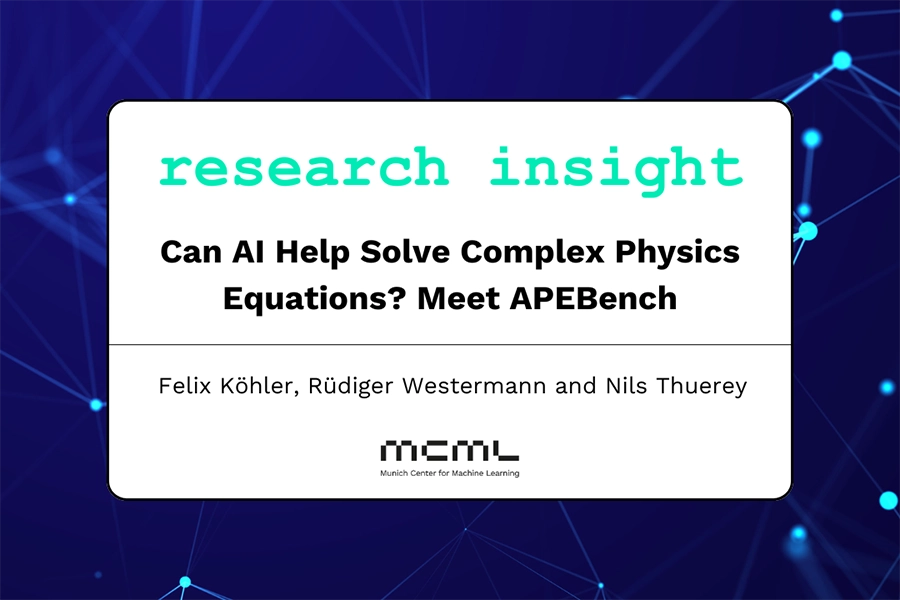
11.02.2025
Can AI Help Solve Complex Physics Equations? Meet APEBench
Our Junior Member Felix Köhler, together with our PIs Rüdiger Westermann and Nils Thuerey have introduced APEBench, an innovative benchmark suite.
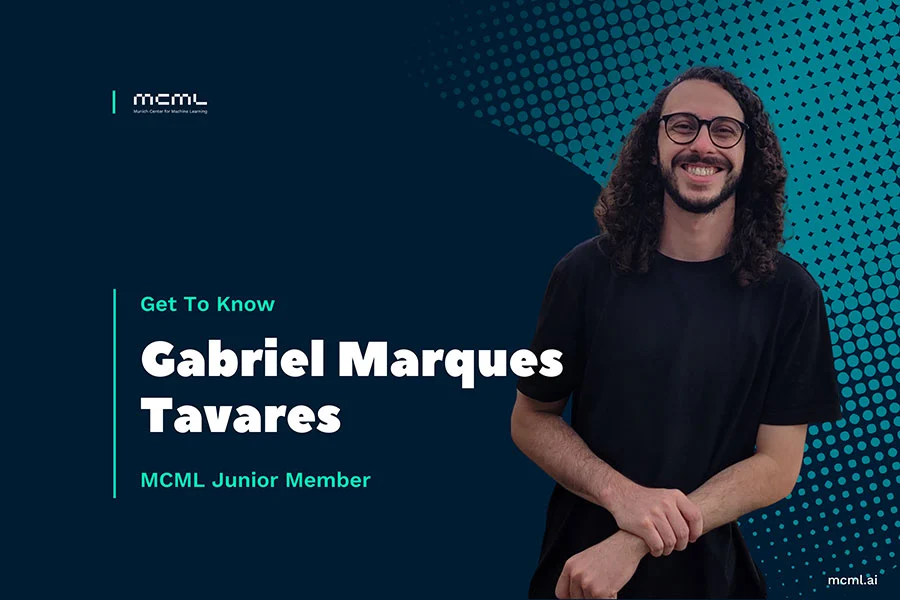
06.02.2025
Improving Business Processes
Gabriel Marques Tavares, MCML Junior Member and LMU PostDoc, explores Process Mining to optimize workflows and promote data-driven decisions.