20.08.2024
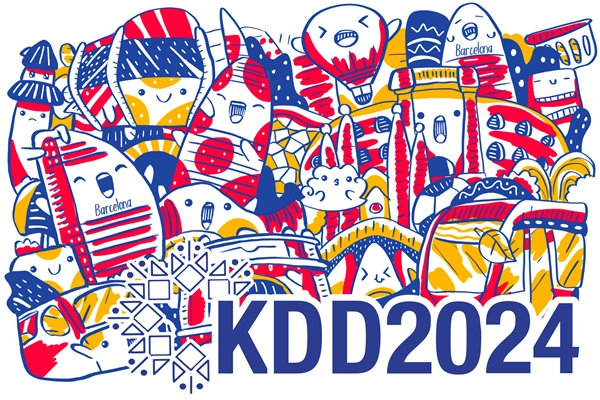
MCML Researchers With Two Papers at KDD 2024
30th ACM SIGKDD International Conference on Knowledge Discovery and Data (KDD 2024). Barcelona, Spain, 25.08.2024–29.08.2024
We are happy to announce that MCML researchers are represented with two papers at KDD 2024. Congrats to our researchers!
Main Track (2 papers)
Explanatory Model Monitoring to Understand the Effects of Feature Shifts on Performance.
KDD 2024 - 30th ACM SIGKDD International Conference on Knowledge Discovery and Data Mining. Barcelona, Spain, Aug 25-29, 2024. DOI
Abstract
Monitoring and maintaining machine learning models are among the most critical challenges in translating recent advances in the field into real-world applications. However, current monitoring methods lack the capability of provide actionable insights answering the question of why the performance of a particular model really degraded. In this work, we propose a novel approach to explain the behavior of a black-box model under feature shifts by attributing an estimated performance change to interpretable input characteristics. We refer to our method that combines concepts from Optimal Transport and Shapley Values as Explanatory Performance Estimation (XPE). We analyze the underlying assumptions and demonstrate the superiority of our approach over several baselines on different data sets across various data modalities such as images, audio, and tabular data. We also indicate how the generated results can lead to valuable insights, enabling explanatory model monitoring by revealing potential root causes for model deterioration and guiding toward actionable countermeasures.
MCML Authors
Causal Machine Learning for Cost-Effective Allocation of Development Aid.
KDD 2024 - 30th ACM SIGKDD International Conference on Knowledge Discovery and Data Mining. Barcelona, Spain, Aug 25-29, 2024. DOI
Abstract
The Sustainable Development Goals (SDGs) of the United Nations provide a blueprint of a better future by ’leaving no one behind’, and, to achieve the SDGs by 2030, poor countries require immense volumes of development aid. In this paper, we develop a causal machine learning framework for predicting heterogeneous treatment effects of aid disbursements to inform effective aid allocation. Specifically, our framework comprises three components: (i) a balancing autoencoder that uses representation learning to embed high-dimensional country characteristics while addressing treatment selection bias; (ii) a counterfactual generator to compute counterfactual outcomes for varying aid volumes to address small sample-size settings; and (iii) an inference model that is used to predict heterogeneous treatment-response curves. We demonstrate the effectiveness of our framework using data with official development aid earmarked to end HIV/AIDS in 105 countries, amounting to more than USD 5.2 billion. For this, we first show that our framework successfully computes heterogeneous treatment-response curves using semi-synthetic data. Then, we demonstrate our framework using real-world HIV data. Our framework points to large opportunities for a more effective aid allocation, suggesting that the total number of new HIV infections could be reduced by up to 3.3% (~50,000 cases) compared to the current allocation practice.
MCML Authors
Artificial Intelligence in Management
Artificial Intelligence in Management
20.08.2024
Related
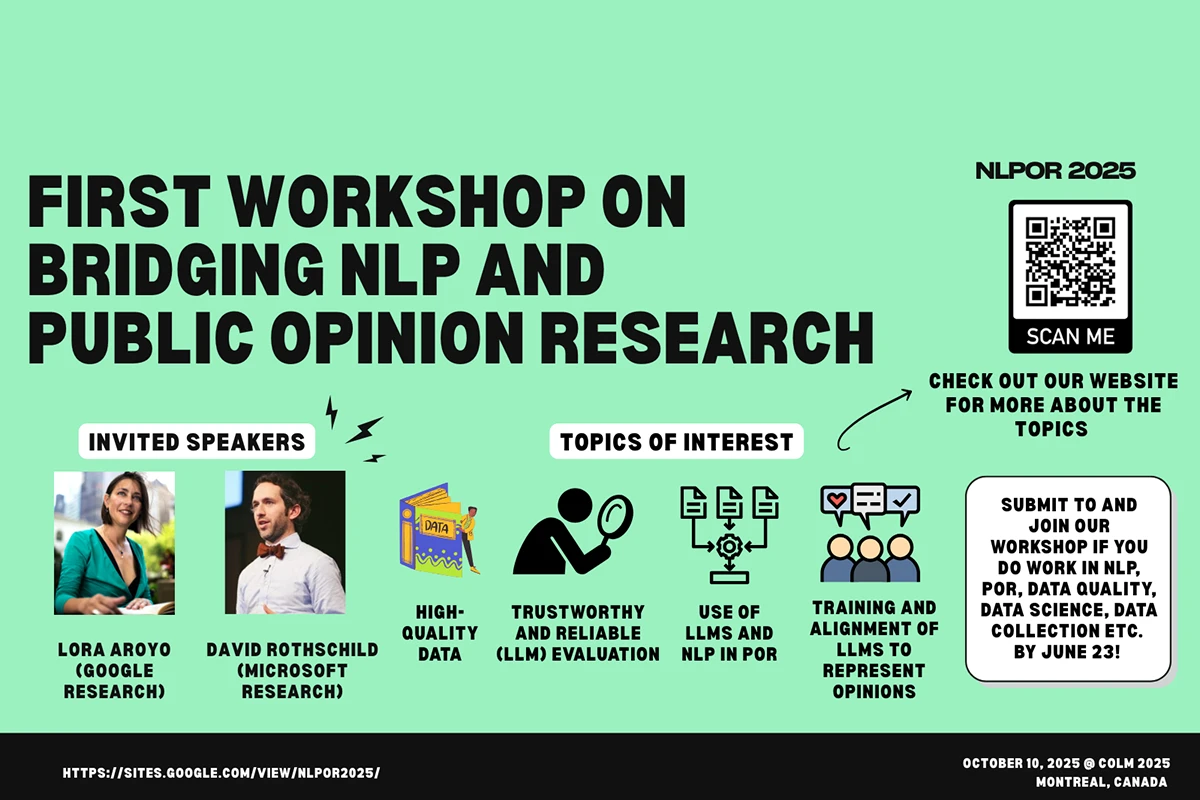
20.05.2025
Call for Papers for NLPOR - First Workshop on Bridging NLP and Public Opinion Research
This interdisciplinary workshop explores the powerful connections between Natural Language Processing (NLP) and Public Opinion Research (POR).
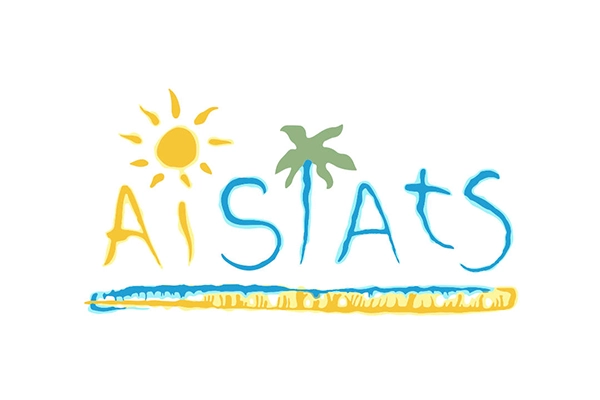
02.05.2025
MCML Researchers With Five Papers at AISTATS 2025
28th International Conference on Artificial Intelligence and Statistics (AISTATS 2025). Mai Khao, Thailand, 29.04.2025 - 05.05.2024
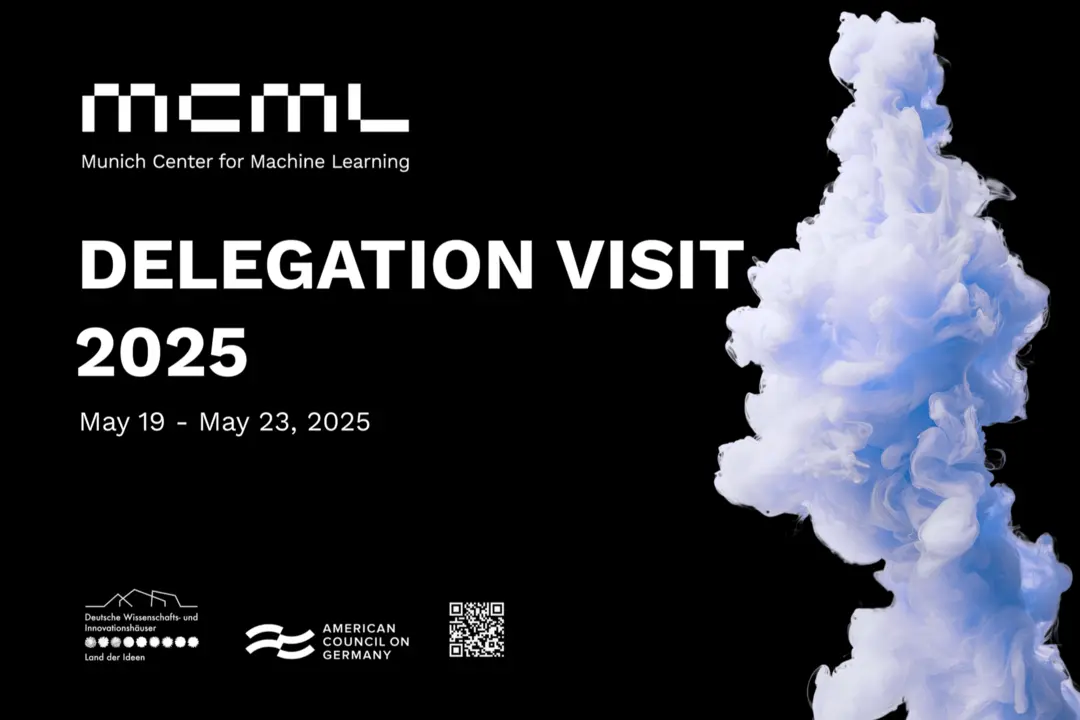
28.04.2025
MCML Delegation Visit to the USA
MCML delegation visits top US institutions to foster AI research collaborations in Generative and Medical AI, May 19–23, 2025.
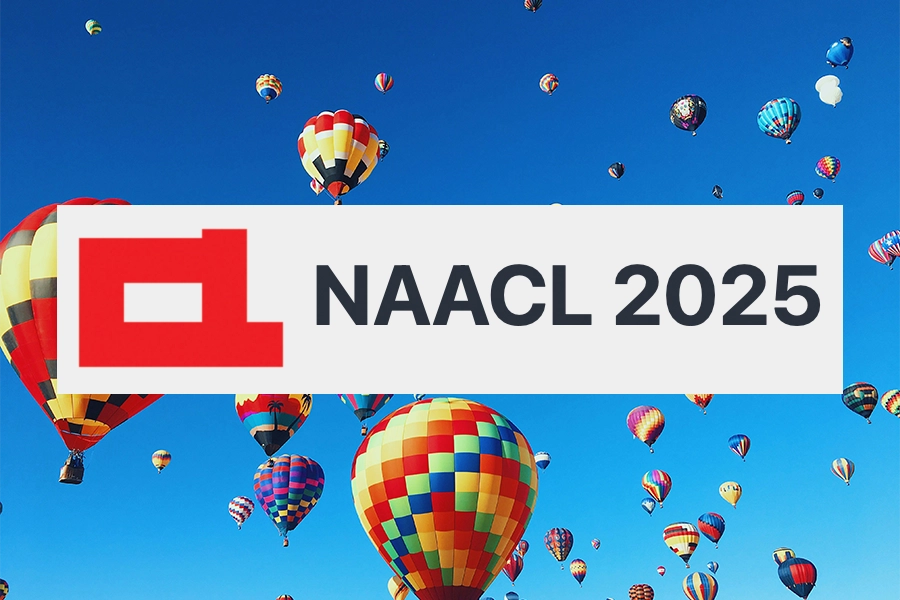
28.04.2025
MCML Researchers With Eleven Papers at NAACL 2025
Annual Conference of the North American Chapter of the Association for Computational Linguistics (NAACL 2025). Albuquerque, NM, USA, 29.04.2025 - 04.05.2024