29.07.2024
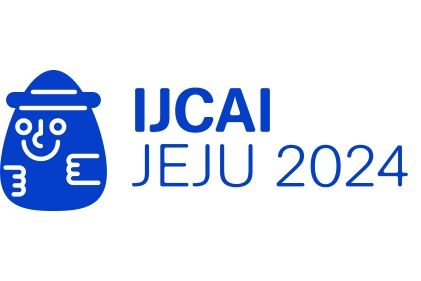
Three papers at IJCAI 2024
33rd International Joint Conference on Artificial Intelligence (IJCAI 2024). Jeju, Korea, 03.08.2024–09.08.2024
We are happy to announce that MCML researchers are represented with three papers at IJCAI 2024:
Best Arm Identification with Retroactively Increased Sampling Budget for More Resource-Efficient HPO.
IJCAI 2024 - 33rd International Joint Conference on Artificial Intelligence. Jeju, Korea, Aug 03-09, 2024. DOI
Abstract
Hyperparameter optimization (HPO) is indispensable for achieving optimal performance in machine learning tasks. A popular class of methods in this regard is based on Successive Halving (SHA), which casts HPO into a pure-exploration multi-armed bandit problem under finite sampling budget constraints. This is accomplished by considering hyperparameter configurations as arms and rewards as the negative validation losses. While enjoying theoretical guarantees as well as working well in practice, SHA comes, however, with several hyperparameters itself, one of which is the maximum budget that can be allocated to evaluate a single arm (hyperparameter configuration). Although there are already solutions to this meta hyperparameter optimization problem, such as the doubling trick or asynchronous extensions of SHA, these are either practically inefficient or lack theoretical guarantees. In this paper, we propose incremental SHA (iSHA), a synchronous extension of SHA, allowing to increase the maximum budget a posteriori while still enjoying theoretical guarantees. Our empirical analysis of HPO problems corroborates our theoretical findings and shows that iSHA is more resource-efficient than existing SHA-based approaches.
MCML Authors
Using Analogical Reasoning to Prompt LLMs for their Intuitions of Abstract Spatial Schemas.
Analogy-ANGLE @IJCAI 2024 - 1st Workshop on Analogical Abstraction in Cognition, Perception, and Language at the 33rd International Joint Conference on Artificial Intelligence (IJCAI 2024). Jeju, Korea, Aug 03-09, 2024. PDF
Abstract
Abstract notions are often comprehended through analogies, wherein there exists correspondence or partial similarity with more concrete concepts. A fundamental aspect of human cognition involves synthesising embodied experiences into spatial schemas, which profoundly influence conceptualisation and underlie language acquisition. Recent studies have demonstrated that Large Language Models (LLMs) exhibit certain spatial intuitions akin to human language. For instance, both humans and LLMs tend to associate ↑ with hope more readily than with warn. However, the nuanced partial similarities between concrete (e.g., ↑) and abstract (e.g., hope) concepts, remain insufficiently explored. Therefore, we propose a novel methodology utilising analogical reasoning to elucidate these associations and examine whether LLMs adjust their associations in response to analogy-prompts. We find that analogy-prompting is slightly increasing agreement with human choices and the answers given by models include valid explanations supported by analogies, even when in disagreement with human results.
MCML Authors
Towards Efficient Posterior Sampling in Deep Neural Networks via Symmetry Removal (Extended Abstract).
IJCAI 2024 - 33rd International Joint Conference on Artificial Intelligence. Jeju, Korea, Aug 03-09, 2024. DOI
Abstract
Bayesian inference in deep neural networks is challenging due to the high-dimensional, strongly multi-modal parameter posterior density landscape. Markov chain Monte Carlo approaches asymptotically recover the true posterior but are considered prohibitively expensive for large modern architectures. Local methods, which have emerged as a popular alternative, focus on specific parameter regions that can be approximated by functions with tractable integrals. While these often yield satisfactory empirical results, they fail, by definition, to account for the multi-modality of the parameter posterior. In this work, we argue that the dilemma between exact-but-unaffordable and cheap-but-inexact approaches can be mitigated by exploiting symmetries in the posterior landscape. Such symmetries, induced by neuron interchangeability and certain activation functions, manifest in different parameter values leading to the same functional output value. We show theoretically that the posterior predictive density in Bayesian neural networks can be restricted to a symmetry-free parameter reference set. By further deriving an upper bound on the number of Monte Carlo chains required to capture the functional diversity, we propose a straightforward approach for feasible Bayesian inference. Our experiments suggest that efficient sampling is indeed possible, opening up a promising path to accurate uncertainty quantification in deep learning.
MCML Authors
29.07.2024
Related
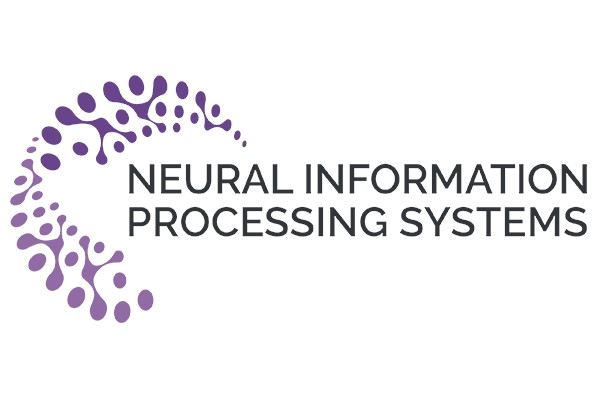
05.12.2024
26 papers at NeurIPS 2024
38th Conference on Neural Information Processing Systems (NeurIPS 2024). Vancouver, Canada, 10.12.2024 - 15.12.2024
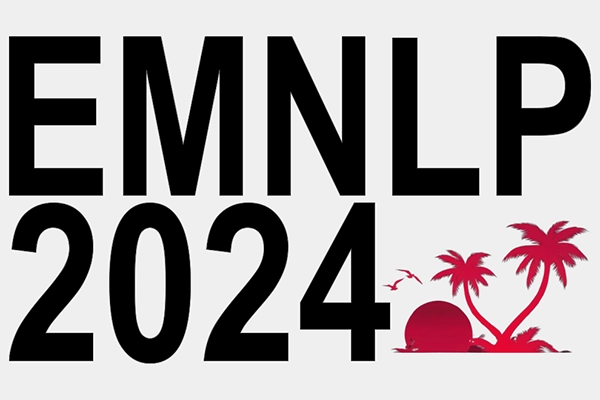
06.11.2024
20 papers at EMNLP 2024
Conference on Empirical Methods in Natural Language Processing (EMNLP 2024). Miami, FL, USA, 12.11.2024 - 16.11.2024
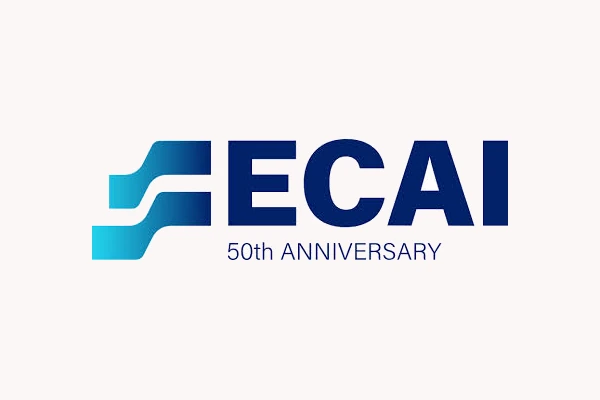
18.10.2024
Three papers at ECAI 2024
27th European Conference on Artificial Intelligence (ECAI 2024). Santiago de Compostela, Spain, 19.10.2024 - 24.10.2024
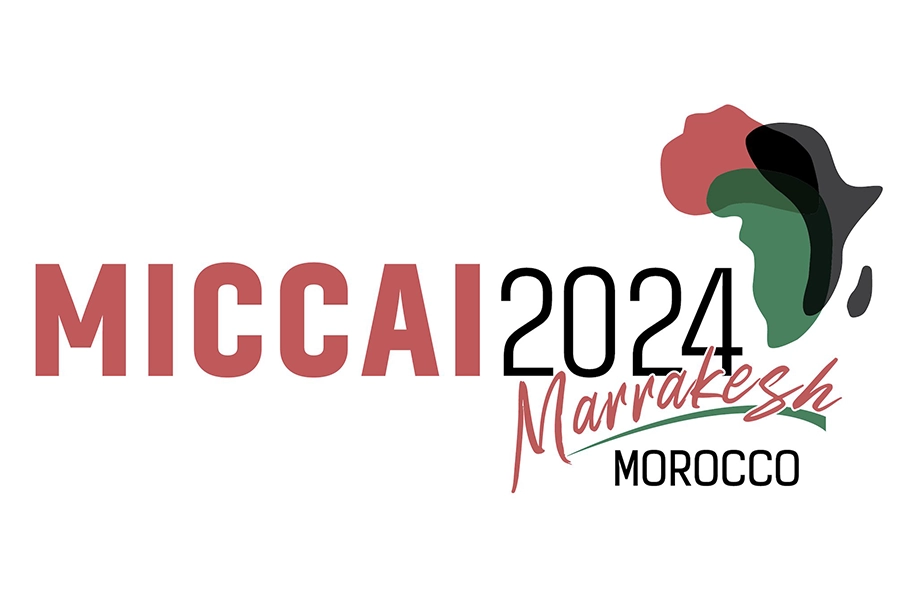
01.10.2024
16 papers at MICCAI 2024
27th International Conference on Medical Image Computing and Computer Assisted Intervention (MICCAI 2024). Marrakesh, Morocco, 06.10.2024 - 10.10.2024
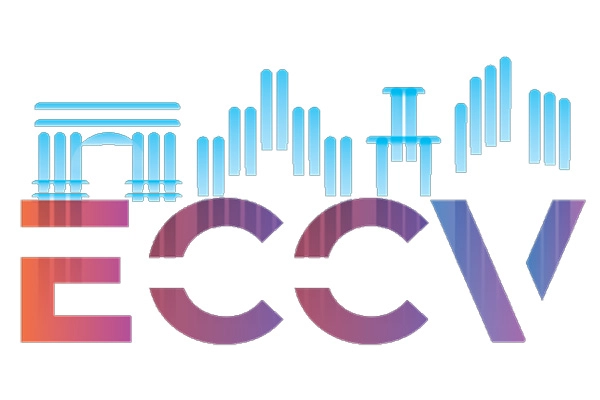
26.09.2024
20 papers at ECCV 2024
18th European Conference on Computer Vision (ECCV 2024). Milano, Italy, 29.09.2024 - 04.10.2024