03.02.2023
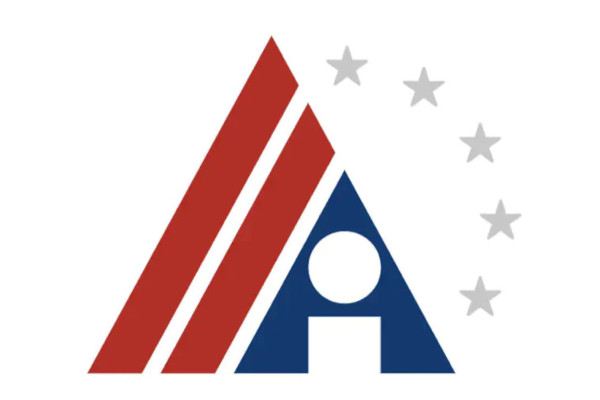
MCML researchers with four papers at AAAI 2023
37th Conference on Artificial Intelligence (AAAI 2023). Washington, DC, USA, 07.02.2023–14.02.2023
We are happy to announce that MCML researchers are represented with four papers at AAAI 2023:
AC-Band: A Combinatorial Bandit-Based Approach to Algorithm Configuration.
37th Conference on Artificial Intelligence (AAAI 2023). Washington, DC, USA, Feb 07-14, 2023. DOI.
Abstract
We study the algorithm configuration (AC) problem, in which one seeks to find an optimal parameter configuration of a given target algorithm in an automated way. Although this field of research has experienced much progress recently regarding approaches satisfying strong theoretical guarantees, there is still a gap between the practical performance of these approaches and the heuristic state-of-the-art approaches. Recently, there has been significant progress in designing AC approaches that satisfy strong theoretical guarantees. However, a significant gap still remains between the practical performance of these approaches and state-of-the-art heuristic methods. To this end, we introduce AC-Band, a general approach for the AC problem based on multi-armed bandits that provides theoretical guarantees while exhibiting strong practical performance. We show that AC-Band requires significantly less computation time than other AC approaches providing theoretical guarantees while still yielding high-quality configurations.
MCML Authors
Estimating Average Causal Effects from Patient Trajectories.
37th Conference on Artificial Intelligence (AAAI 2023). Washington, DC, USA, Feb 07-14, 2023. DOI.
Abstract
In medical practice, treatments are selected based on the ex- pected causal effects on patient outcomes. Here, the gold standard for estimating causal effects are randomized con- trolled trials; however, such trials are costly and sometimes even unethical. Instead, medical practice is increasingly interested in estimating causal effects among patient (sub)groups from electronic health records, that is, observational data. In this paper, we aim at estimating the average causal effect (ACE) from observational data (patient trajectories) that are collected over time. For this, we propose DeepACE: an end-to-end deep learning model. DeepACE leverages the iterative G-computation formula to adjust for the bias induced by time-varying confounders. Moreover, we develop a novel sequential targeting procedure which ensures that DeepACE has favorable theoretical properties, i. e., is doubly robust and asymptotically efficient. To the best of our knowledge, this is the first work that proposes an end-to-end deep learning model tailored for estimating time-varying ACEs. We com- pare DeepACE in an extensive number of experiments, confirming that it achieves state-of-the-art performance. We further provide a case study for patients suffering from low back pain to demonstrate that DeepACE generates important and meaningful findings for clinical practice. Our work enables practitioners to develop effective treatment recommendations based on population effects.
MCML Authors
InstanceFormer: An Online Video Instance Segmentation Framework.
37th Conference on Artificial Intelligence (AAAI 2023). Washington, DC, USA, Feb 07-14, 2023. DOI. GitHub.
Abstract
Recent transformer-based offline video instance segmentation (VIS) approaches achieve encouraging results and significantly outperform online approaches. However, their reliance on the whole video and the immense computational complexity caused by full Spatio-temporal attention limit them in real-life applications such as processing lengthy videos. In this paper, we propose a single-stage transformer-based efficient online VIS framework named InstanceFormer, which is especially suitable for long and challenging videos. We propose three novel components to model short-term and long-term dependency and temporal coherence. First, we propagate the representation, location, and semantic information of prior instances to model short-term changes. Second, we propose a novel memory cross-attention in the decoder, which allows the network to look into earlier instances within a certain temporal window. Finally, we employ a temporal contrastive loss to impose coherence in the representation of an instance across all frames. Memory attention and temporal coherence are particularly beneficial to long-range dependency modeling, including challenging scenarios like occlusion. The proposed InstanceFormer outperforms previous online benchmark methods by a large margin across multiple datasets. Most importantly, InstanceFormer surpasses offline approaches for challenging and long datasets such as YouTube-VIS-2021 and OVIS.
MCML Authors
Improvement-focused causal recourse (ICR).
37th Conference on Artificial Intelligence (AAAI 2023). Washington, DC, USA, Feb 07-14, 2023. DOI.
Abstract
Algorithmic recourse recommendations, such as Karimi et al.’s (2021) causal recourse (CR), inform stakeholders of how to act to revert unfavorable decisions. However, there are ac- tions that lead to acceptance (i.e., revert the model’s deci- sion) but do not lead to improvement (i.e., may not revert the underlying real-world state). To recommend such actions is to recommend fooling the predictor. We introduce a novel method, Improvement-Focused Causal Recourse (ICR), which involves a conceptual shift: Firstly, we require ICR recommen- dations to guide toward improvement. Secondly, we do not tailor the recommendations to be accepted by a specific predic- tor. Instead, we leverage causal knowledge to design decision systems that predict accurately pre- and post-recourse. As a result, improvement guarantees translate into acceptance guar- antees. We demonstrate that given correct causal knowledge ICR, in contrast to existing approaches, guides toward both acceptance and improvement.
MCML Authors
03.02.2023
Related
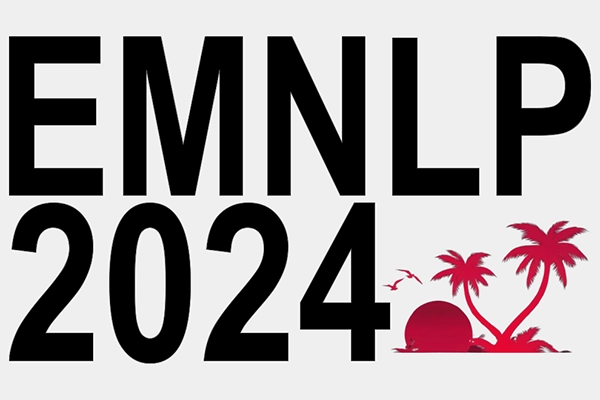
06.11.2024
MCML researchers with 20 papers at EMNLP 2024
Conference on Empirical Methods in Natural Language Processing (EMNLP 2024). Miami, FL, USA, 12.11.2024 - 16.11.2024
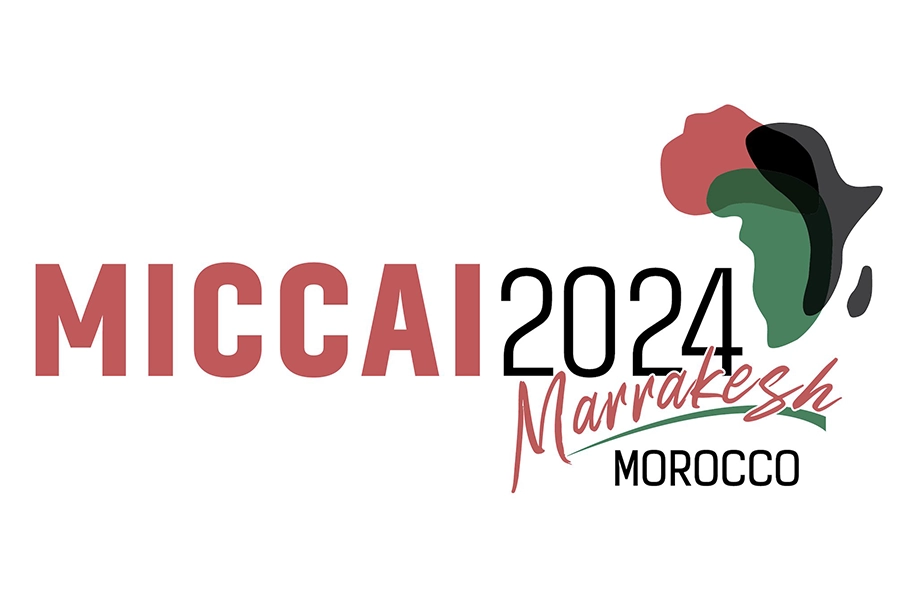
01.10.2024
MCML researchers with 16 papers at MICCAI 2024
27th International Conference on Medical Image Computing and Computer Assisted Intervention (MICCAI 2024). Marrakesh, Morocco, 06.10.2024 - 10.10.2024
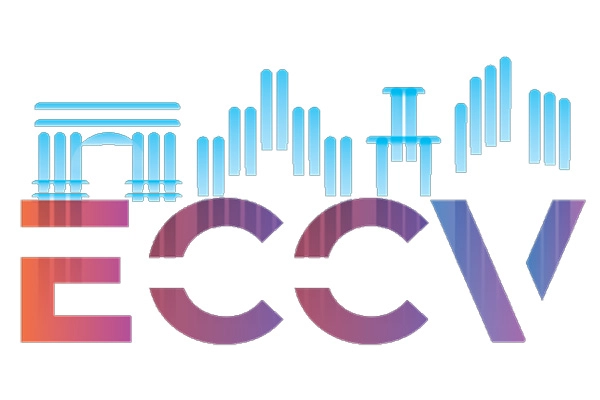
26.09.2024
MCML researchers with 18 papers at ECCV 2024
18th European Conference on Computer Vision (ECCV 2024). Milano, Italy, 29.09.2024 - 04.10.2024
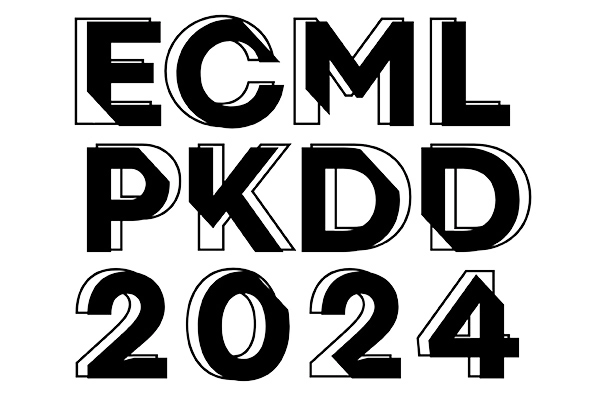
10.09.2024
MCML at ECML-PKDD 2024
We are happy to announce that MCML researchers are represented at ECML-PKDD 2024.
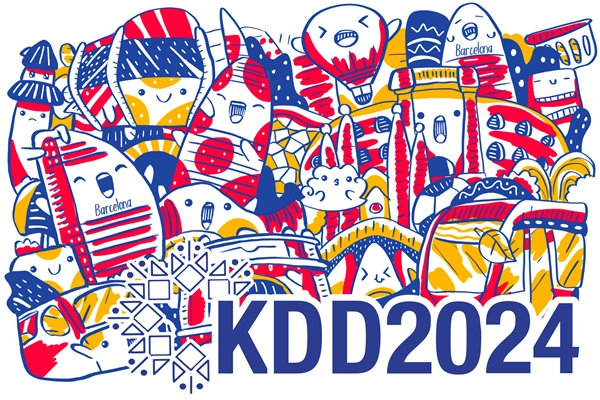
20.08.2024
MCML researchers with two papers at KDD 2024
30th ACM SIGKDD International Conference on Knowledge Discovery and Data (KDD 2024). Barcelona, Spain, 25.08.2024 - 29.08.2024