30.04.2025
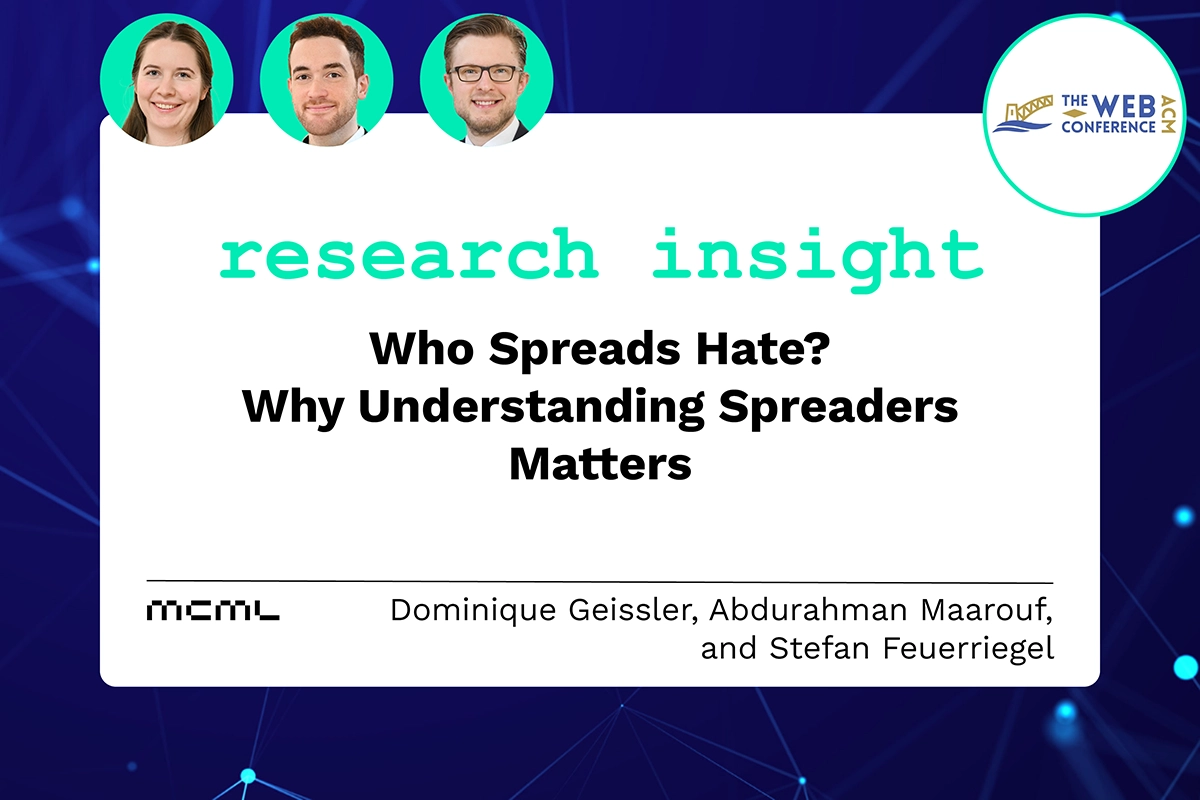
Who Spreads Hate?
MCML Research Insight – With Dominique Geissler, Abdurahman Maarouf, and Stefan Feuerriegel
Hate speech on social media isn’t just offensive - it’s dangerous. It spreads quickly, harms mental health, and can even contribute to real-world violence. While many studies have focused on identifying hate speech or profiling those who create it, a key piece of the puzzle remained missing: Who reshares hate speech?
The team at MCML - Dominique Geissler, Abdurahman Maarouf, and PI Stefan Feuerriegel - explored this question with their latest work: "Analyzing User Characteristics of Hate Speech Spreaders on Social Media".
Why Understanding Spreaders Matters
«Understanding the factors that drive users to share hate speech is crucial for detecting individuals at risk of engaging in harmful behavior and for designing effective mitigation strategies»
Dominique Geissler et al.
MCML Junior Members
Resharing on Social Media can propagate hate speech far beyond its origin. However, not much is known about the people who click the “share” button. To address this gap, the team developed a method to analyse resharing behaviour across different types of hate - such as political, racist, or misogynistic content.
Using large language models and debiasing techniques from causal inference, they were able to pinpoint which user characteristics correlate with hate speech resharing - without falling into the trap of biased social media data.
How the Model Works
The study follows a three-step strategy:
- Clustering Hate: First, hate speech posts are grouped by topic using BERTopic and labeled with LLAMA-3.
- Past Latent Vulnerability: Next, the model estimates how vulnerable a user is to hate content - how likely they are to see and engage with it - using reweighted, debiased click data.
- Modeling Behavior: Finally, the model uses an explainable boosting machine to predict which users are more likely to reshare hate, based on features like follower count, posting activity, and account age.
«We find that, all else equal, users with fewer followers, fewer friends, fewer posts, and older accounts share more hate speech.»
Dominique Geissler et al.
MCML Junior Members
Key Takeaways
- Low Influence, High Harm: Surprisingly, users with low social influence are the primary spreaders of most hate speech.
- Not All Hate Is the Same: Racist and misogynistic hate is spread mostly by users with little social influence. In contrast, political anti-Trump and anti-right-wing hate is reshared by users with larger social influence.
- Feature Spotlight: A feature importance analysis revealed that the number of posts was the strongest predictor of hate speech resharing, followed by the number of followers.
Why It Matters
Identifying hate speech is only half the battle. Understanding who spreads it opens the door for smarter moderation, better platform design, and more effective interventions.
Curious what the authors suggest to reduce the probability of resharing hate speech? Then read the full paper that will be presented at WWW 2025 - The A* ACM Web Conference in Sidney, one of the most prestigious venues in web and internet-related research.
Analyzing User Characteristics of Hate Speech Spreaders on Social Media.
WWW 2025 - ACM Web Conference. Sydney, Australia, Apr 28-May 02, 2025. To be published. Preprint available. arXiv
Abstract
Hate speech on social media threatens the mental and physical well-being of individuals and contributes to real-world violence. Resharing is an important driver behind the spread of hate speech on social media. Yet, little is known about who reshares hate speech and what their characteristics are. In this paper, we analyze the role of user characteristics in hate speech resharing across different types of hate speech (e.g., political hate). For this, we proceed as follows: First, we cluster hate speech posts using large language models to identify different types of hate speech. Then we model the effects of user attributes on users’ probability to reshare hate speech using an explainable machine learning model. To do so, we apply debiasing to control for selection bias in our observational social media data and further control for the latent vulnerability of users to hate speech. We find that, all else equal, users with fewer followers, fewer friends, fewer posts, and older accounts share more hate speech. This shows that users with little social influence tend to share more hate speech. Further, we find substantial heterogeneity across different types of hate speech. For example, racist and misogynistic hate is spread mostly by users with little social influence. In contrast, political anti-Trump and anti-right-wing hate is reshared by users with larger social influence. Overall, understanding the factors that drive users to share hate speech is crucial for detecting individuals at risk of engaging in harmful behavior and for designing effective mitigation strategies.
MCML Authors
Artificial Intelligence in Management
Artificial Intelligence in Management
Artificial Intelligence in Management
Share Your Research!
Get in touch with us!
Are you an MCML Junior Member and interested in showcasing your research on our blog?
We’re happy to feature your work—get in touch with us to present your paper.
30.04.2025
Related
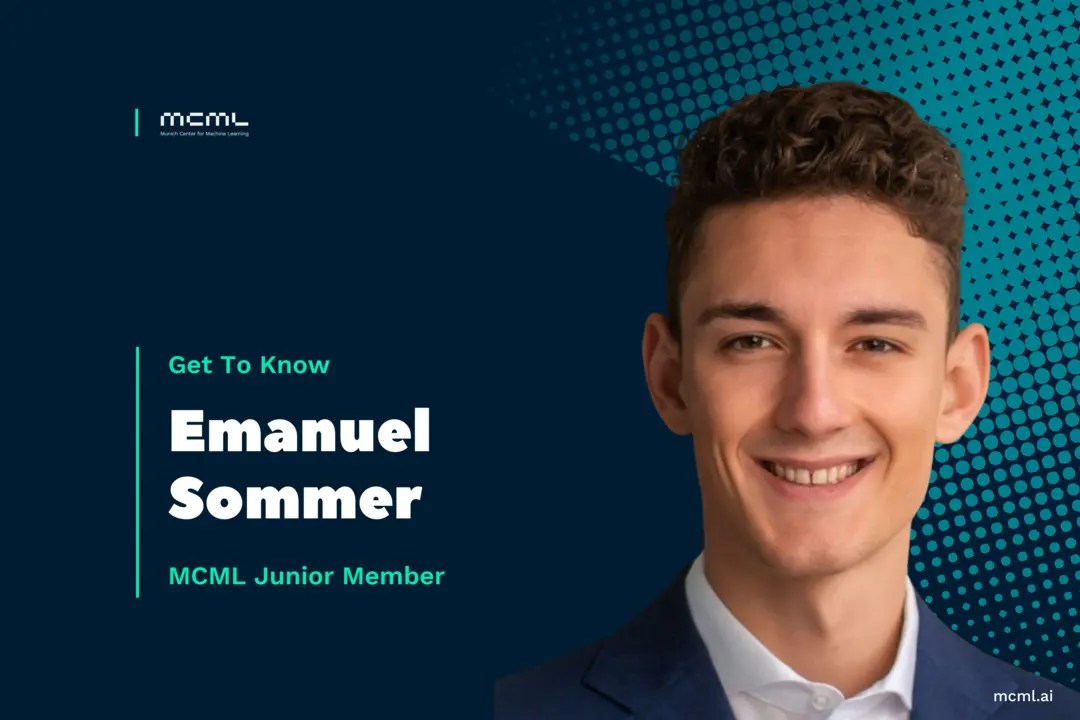
29.04.2025
How Certain Is AI? an Introduction to Bayesian Deep Learning
Researcher in Focus Emanuel Sommer improves uncertainty estimation in deep learning using scalable Bayesian methods for more trustworthy AI.
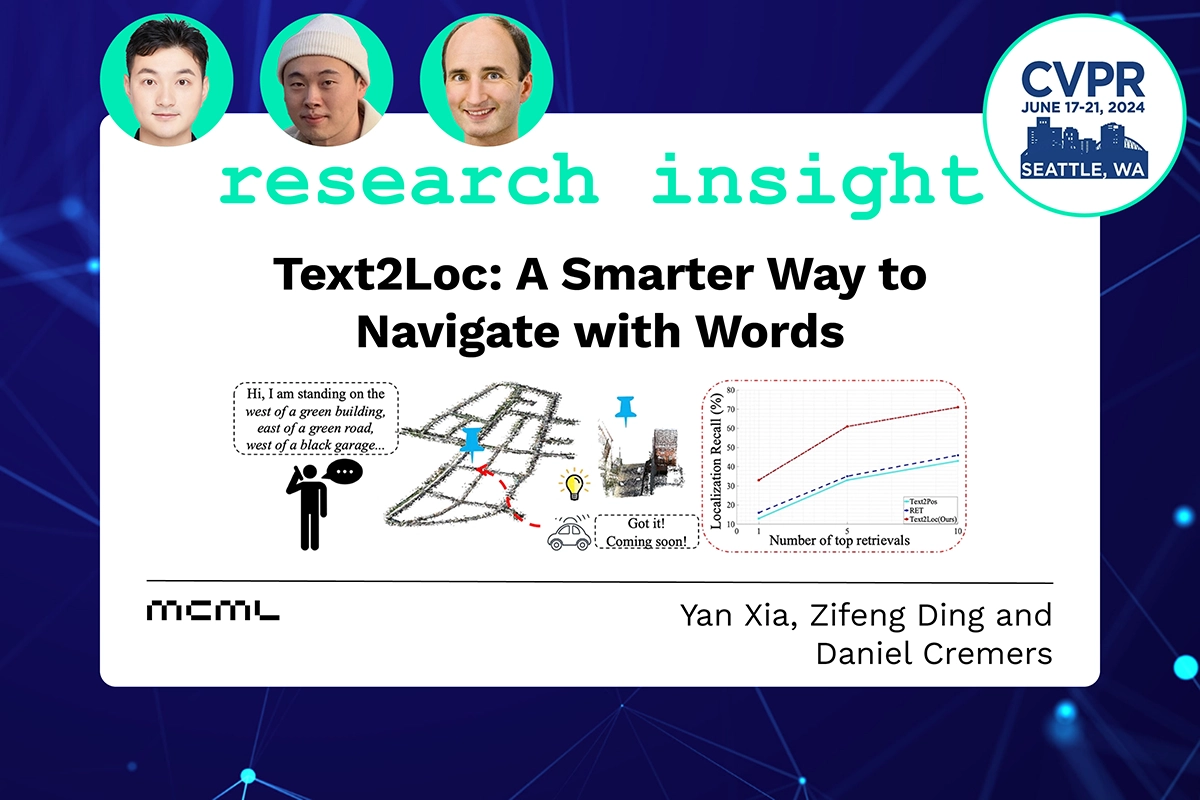
10.04.2025
Text2Loc: A Smarter Way to Navigate With Words
The team of MCML Director Daniel Cremers introduce Text2Loc, a solution designed for city-scale position localization using textual descriptions.
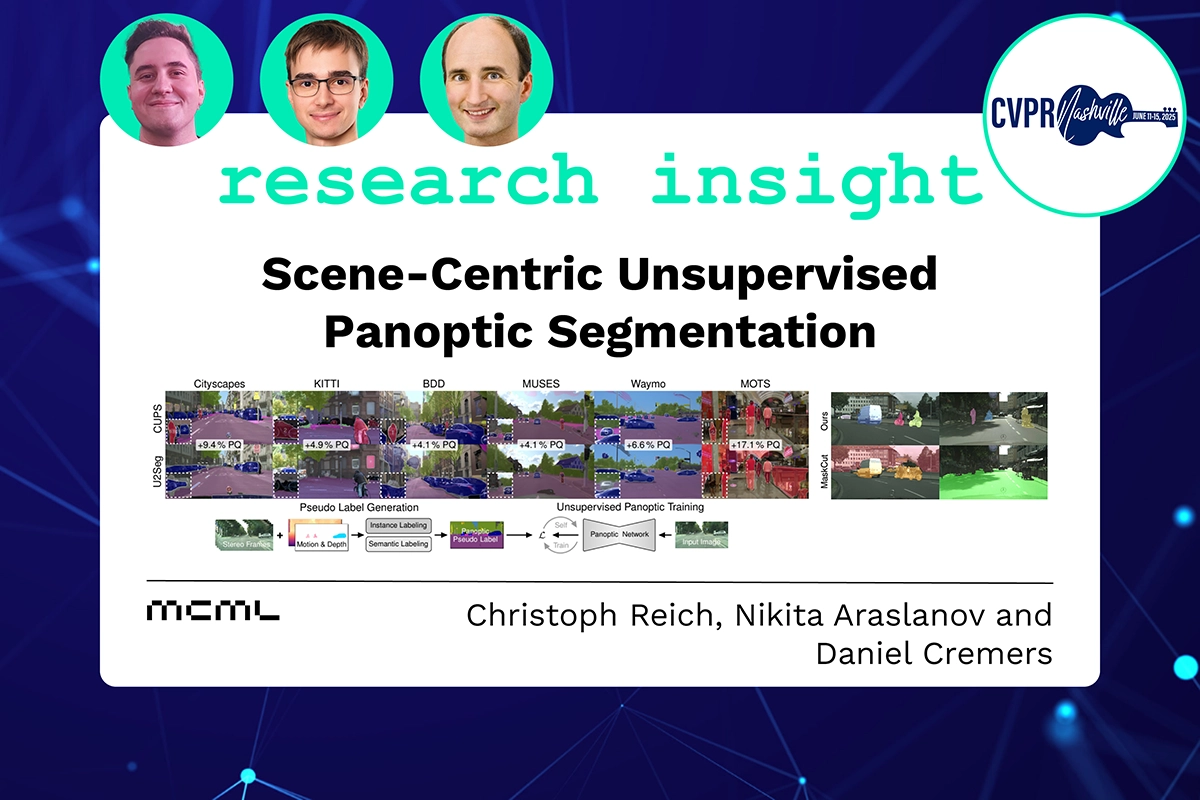
03.04.2025
CUPS: Teaching AI to Understand Scenes Without Human Labels
The team of MCML Director Daniel Cremers introduces CUPS, proving that AI can learn to understand entire scenes - without any human labels.
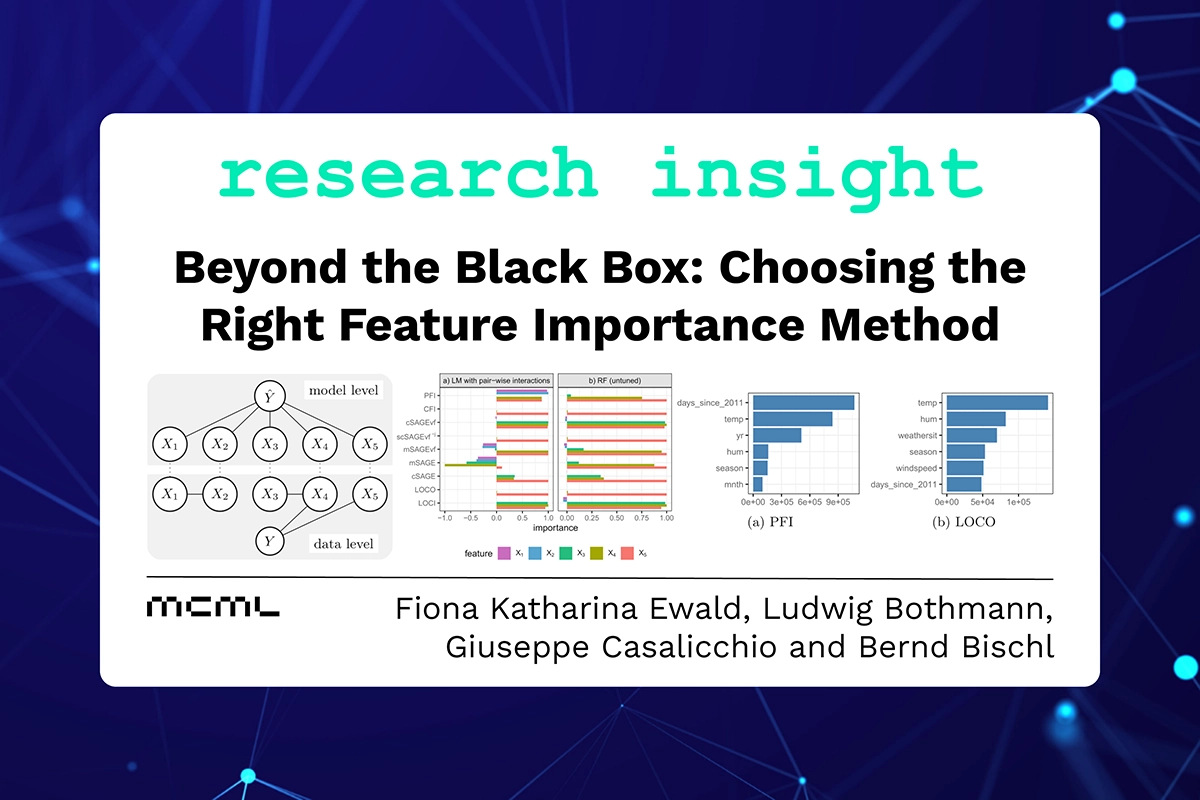
27.03.2025
Beyond the Black Box: Choosing the Right Feature Importance Method
The team of Bernd Bischl created a clear guide to feature importance methods, helping researchers and practitioners interpret AI models effectively.