07.01.2025
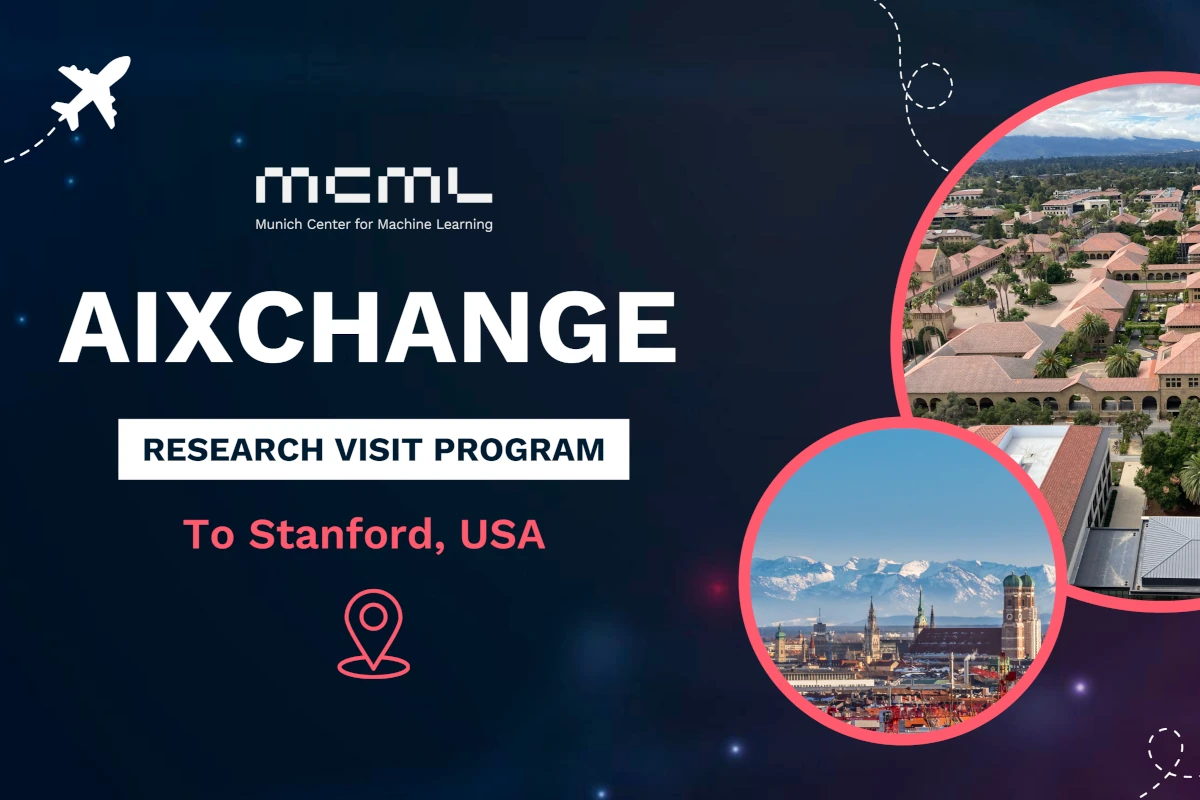
©MCML
Research Collaboration Between TUM/ MCML and Stanford University
Funded by the MCML AI X-Change Program and BaCaTeC
During the summer of 2024, I had the privilege of representing the Computer Vision Group at TUM, led by Prof. Daniel Cremers, in a collaborative research project with the Geometric Computing Group at Stanford University, headed by Prof. Leonidas Guibas. During my three-month stay at Stanford, researchers from both institutes delved deeply into the fascinating intersection of geometry and machine learning, launching research projects initiatives aimed at advancing the edge of the current state of the art. The outcomes of our ongoing efforts will be submitted for publication in leading computer vision and graphics venues. This collaboration is generously funded by the MCML AI X-change program and BaCaTeC.
Unlocking the Secrets of Shape Matching with Equivariant Networks
Non-rigid shape matching is a cornerstone task in computer vision and graphics. Whether it’s mapping the structure of ancient artifacts, analyzing medical data, or enabling robotics to understand shapes, matching deformable objects opens the door to countless applications. But this task is anything but straightforward.
Shapes in the wild often come with unique challenges—different coordinate systems, random rotations, and unknown transformations during data sharing. Historically, researchers have tackled these issues using handcrafted features, but modern neural networks have proven to be a game-changer, yielding impressive results.
The Issue with Classical Approaches
Classical methods for non-rigid shape matching usually fall into two categories:
1. Methods with Pre-Alignment (Weak Supervision):
These methods rely on pre-aligning shapes to a standardized orientation (e.g., upright and facing forward). While effective, the process can be cumbersome and time-consuming, especially for large datasets. Worse yet, the performance depends heavily on how well this alignment is done—an imperfect pre-alignment often leads to subpar results.
2. Intrinsic Methods with Handcrafted Features:
Instead of requiring alignment, these approaches use intrinsic shape properties like wave kernel signatures as input to neural networks. While invariant to transformations like rotations, they struggle with real-world scenarios involving symmetric shapes, such as human or animals.
Both approaches have their merits, but they also have limitations. Pre-alignment adds unnecessary complexity, while intrinsic methods often lose valuable geometric information by relying only on simplified, handcrafted features. This makes them less suitable for tasks that require distinguishing shapes based on external factors, such as deformation or origami design.
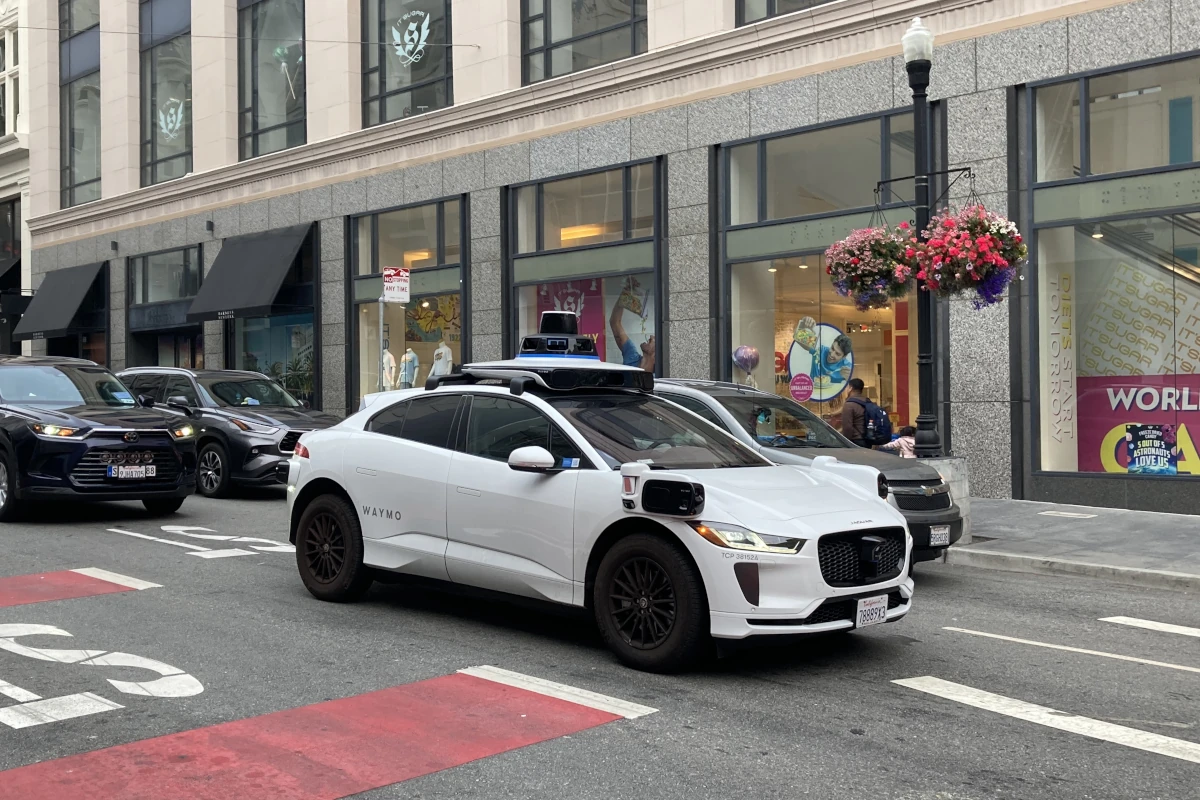
Waymo autonomous driving cars are in operation. These self-driving vehicles navigate the city's hilly terrain and bustling streets with ease, offering a ride that is both comfortable and safe.
A Shift Towards Equivariant Learning
Enter vector neurons, a groundbreaking framework introduced by Deng et al.. This SO(3)-equivariant network can learn features directly from raw shape geometry while maintaining rotational symmetry. Inspired by this approach, we propose a novel method for non-rigid shape matching, addressing the shortcomings of classical techniques.
Our approach leverages equivariant networks to learn vector-valued feature fields—an upgrade from traditional scalar features. By integrating these advanced features with orientation-aware representation of correspondences, we can handle transformations like rotations and symmetries naturally without pre-alignment required.
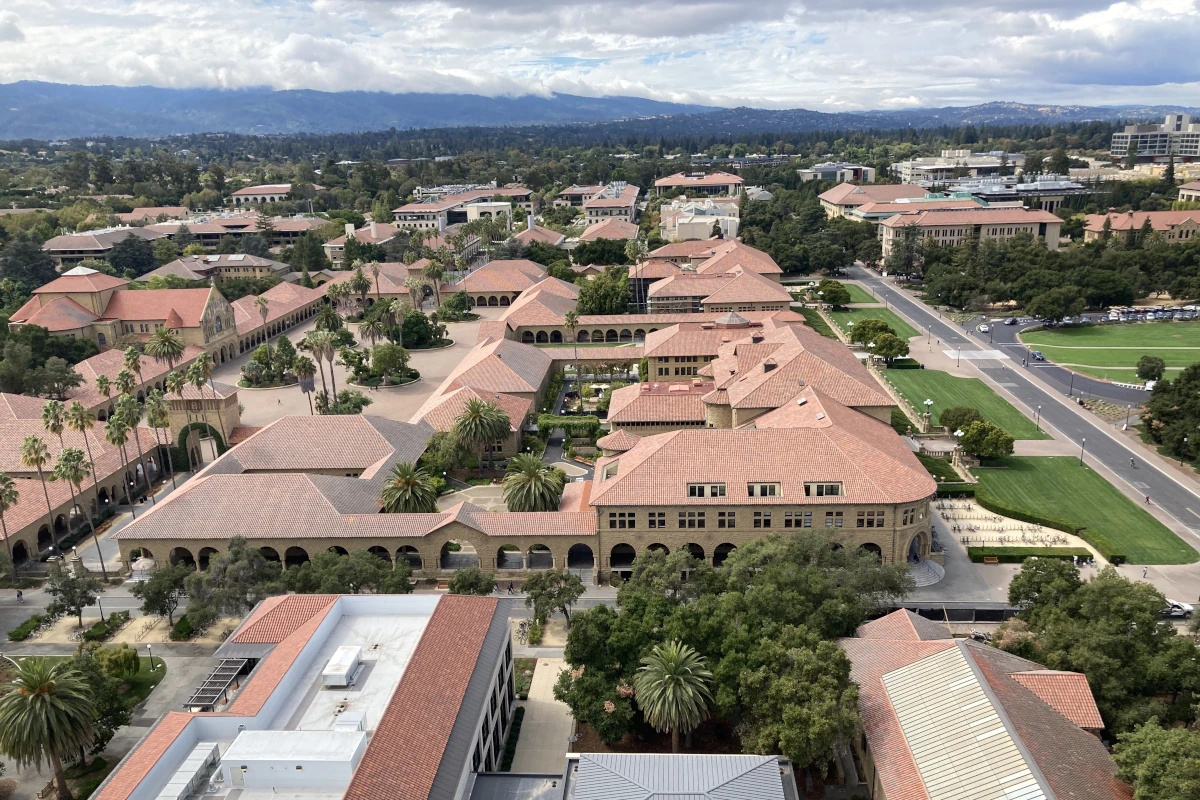
The main quad of Stanford University from the Hoover tower. Every building with a signature red-tiled roof is part of the Stanford campus
Why This Matters
By leveraging equivariant learning and bridging intrinsic and extrinsic methods, our approach tackles a broader range of challenges:
- It enables learning from in-the-wild data without shape pre-alignment and can disambiguate intrinsic shape symmetries. Together with an unsupervised loss, it is the first method, which can explore and learn in the large corpus of available 3D data.
- It enables applications beyond shape matching, such as deformation analysis, classification, and even creative fields like origami design.
What’s Next?
Our method demonstrates the power of equivariant learning and the importance of combining intrinsic and extrinsic features, paving the way for new possibilities in shape analysis. Whether you’re exploring medical applications, robotics, or even creative design, these advancements could redefine how we think about shape understanding.
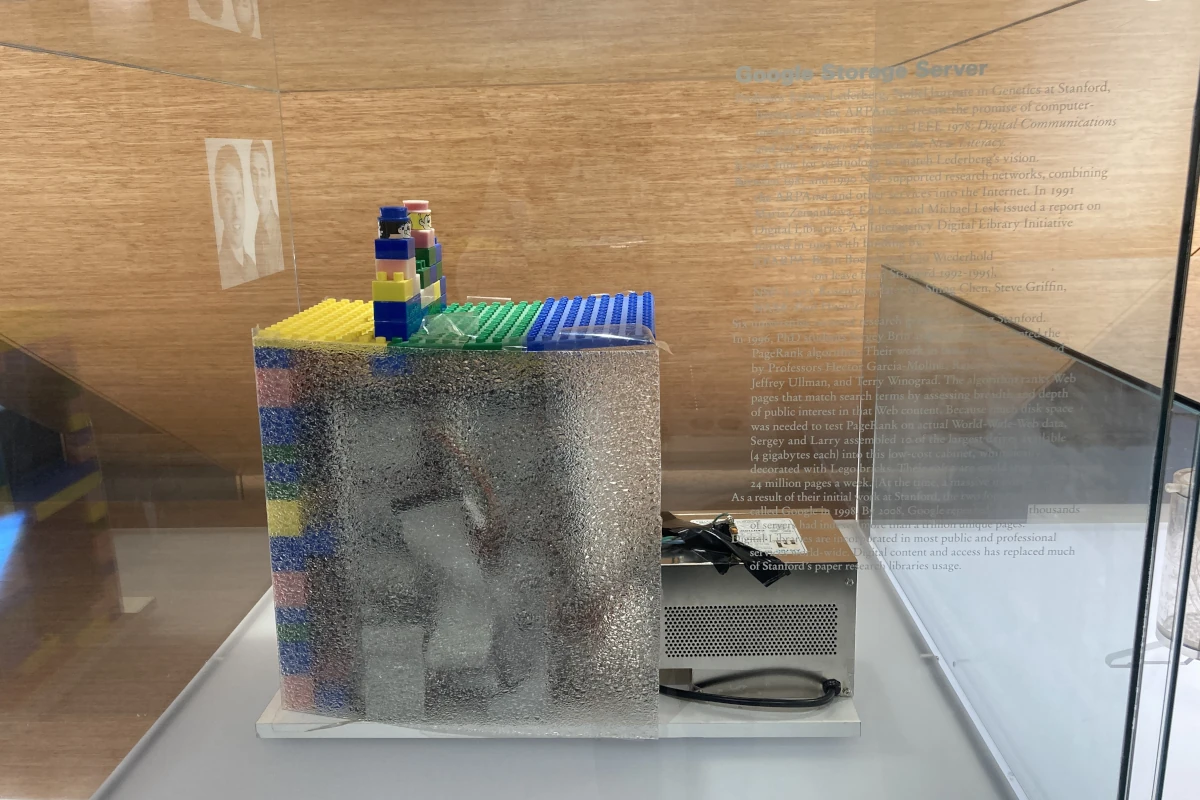
The birth of Google traces back to Stanford University, where Larry Page and Sergey Brin built the first Google server during their PhD studies in computer science. The server, a revolutionary creation, laid the foundation for what would become one of the world's most influential companies. Due to Google's immense success, Page and Brin discontinued their PhD to focus on building the company. This pivotal piece of tech history is now exhibited in the basement of the Jen-Hsun Huang Engineering Center at Stanford.
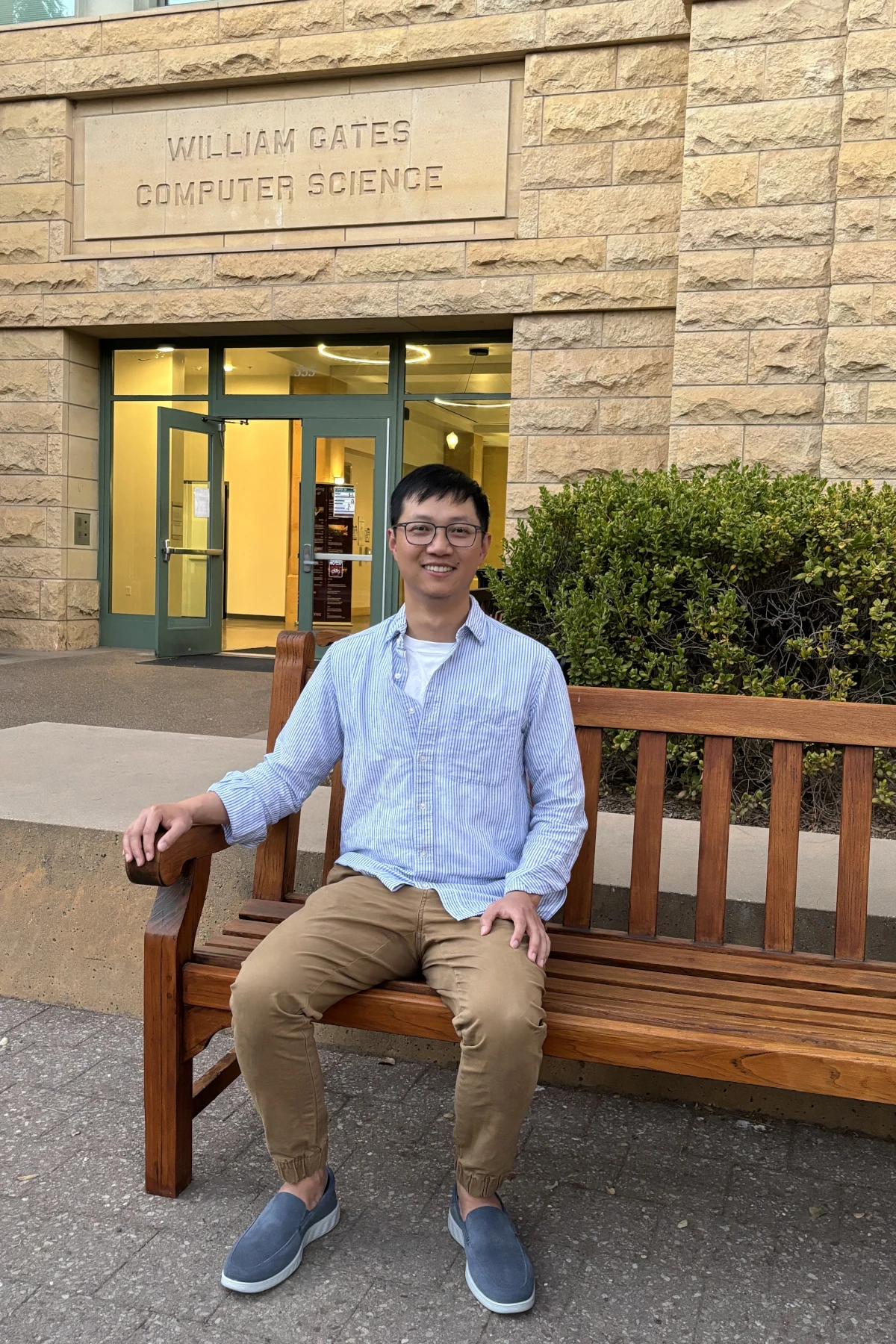
Maolin Gao in front of the Gates Building, home to Stanford University’s renowned Computer Science Department. Completed in 1996, the building was named after Bill Gates, co-founder of Microsoft Corporation, in recognition of his $6 million contribution to the project.
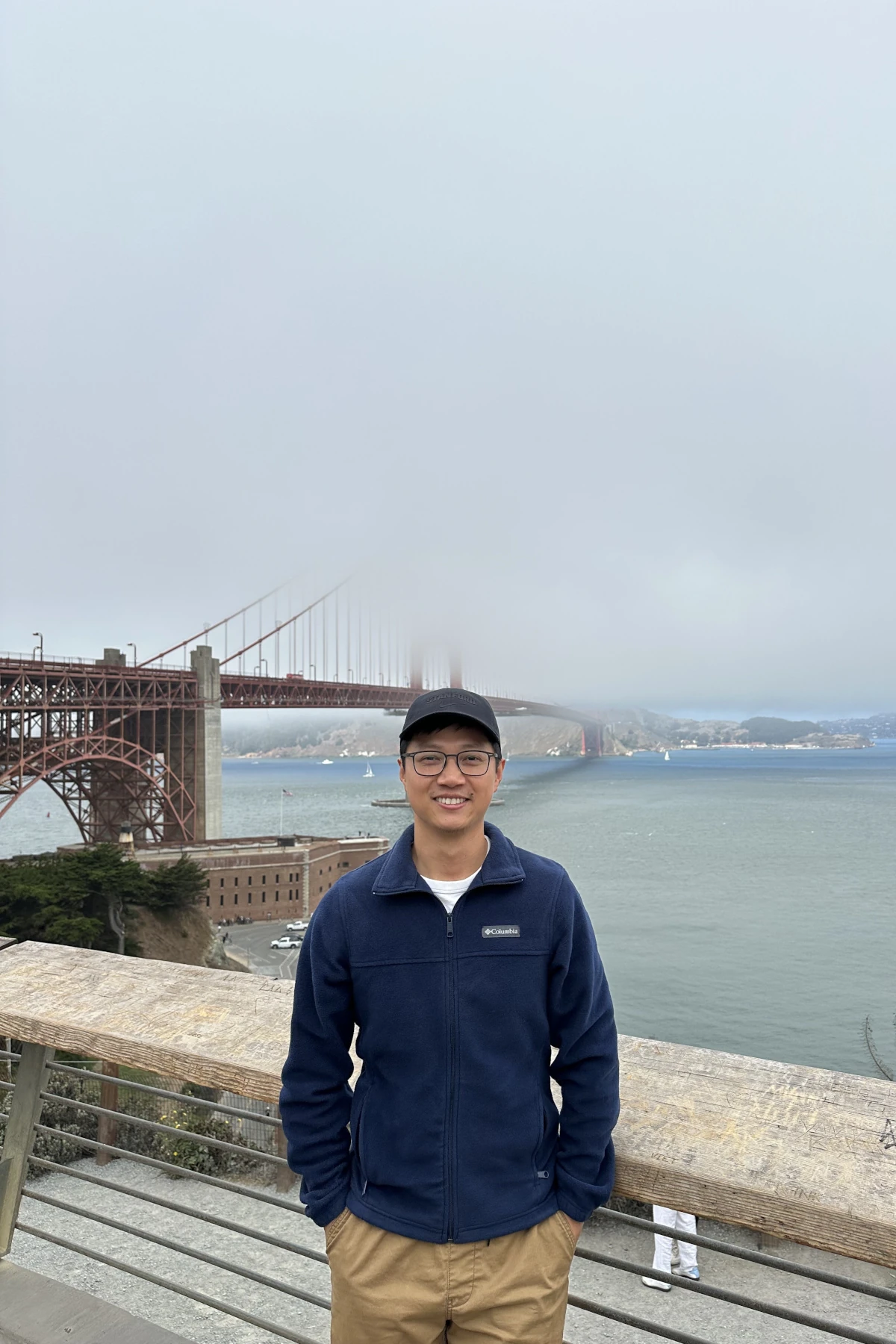
Maolin Gao at the Golden Gate Bridge, an iconic landmark of San Francisco that connects San Francisco Bay to the Pacific Ocean. Completed in 1937, it was a marvel of engineering at the time and held the title of the longest suspension bridge in the world. Its striking design and historical significance continue to make it a symbol of innovation and beauty.
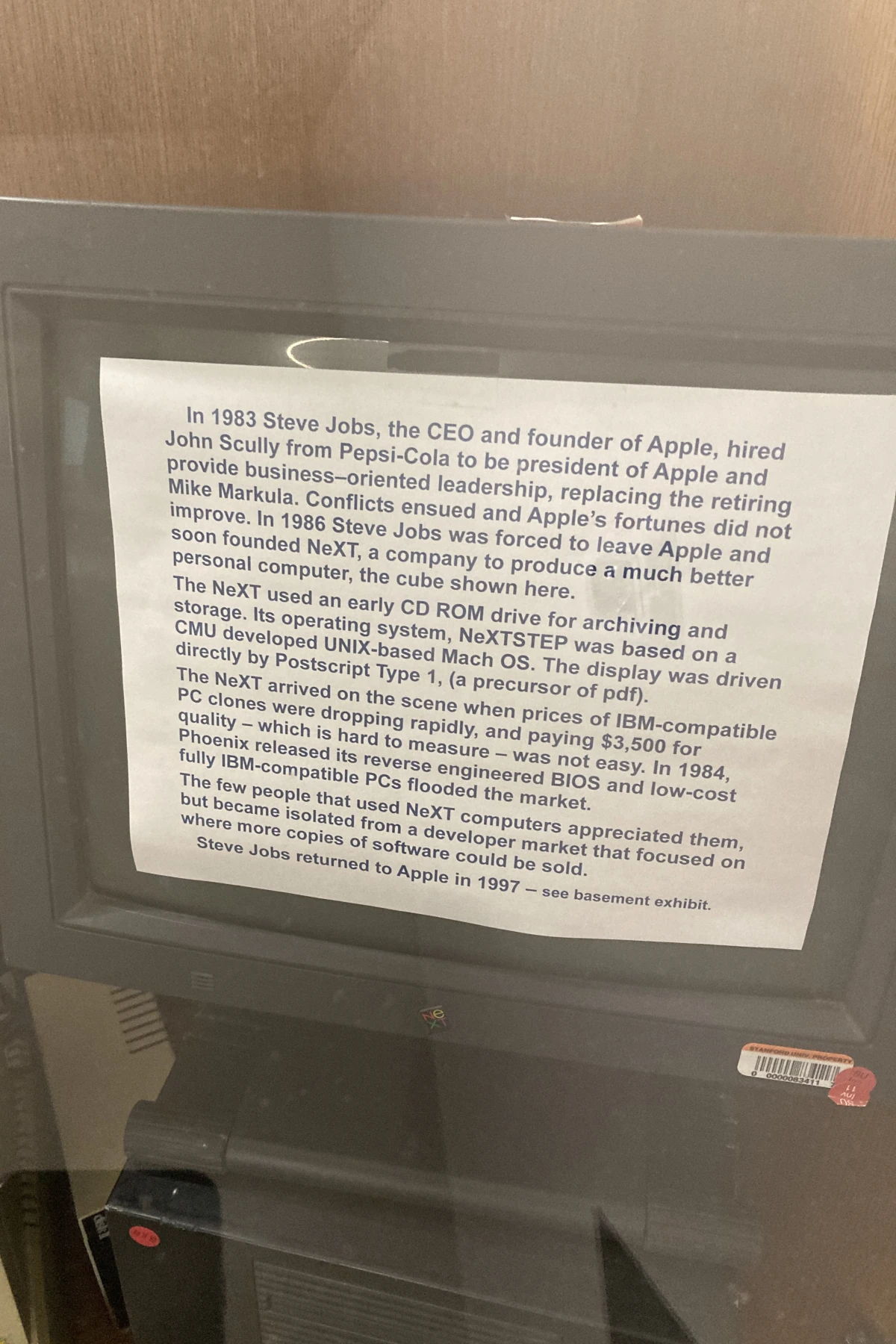
A NeXT computer is on display in the basement of the Gates Building, serving as a fascinating piece of computing history and a tribute to the innovative legacy of the NeXT platform.
07.01.2025
Related
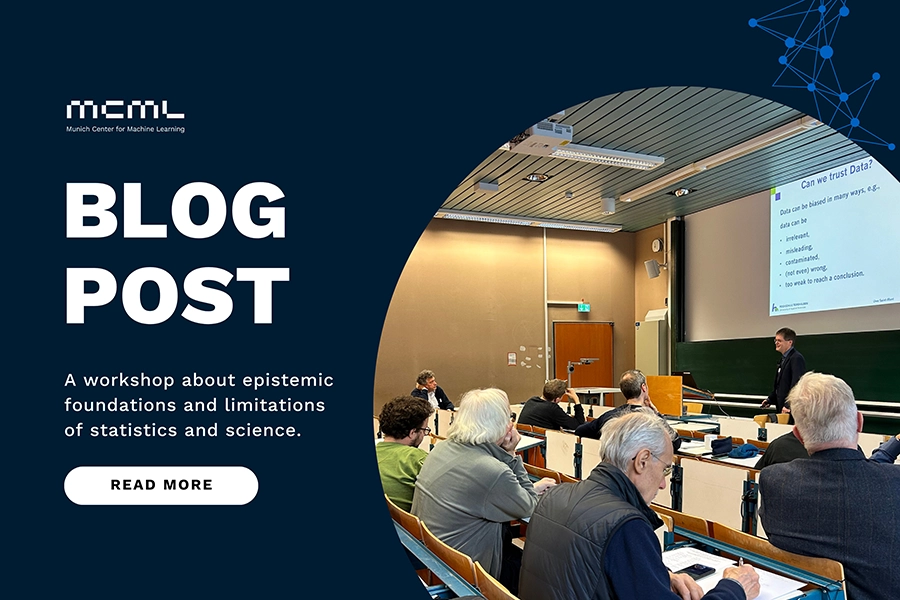
©MCML
19.12.2024
Epistemic Foundations and Limitations of Statistics and Science
Statisticians, philosophers, and scientists tackled the replication crisis, exploring limitations, biases, and paths forward in statistics and AI.
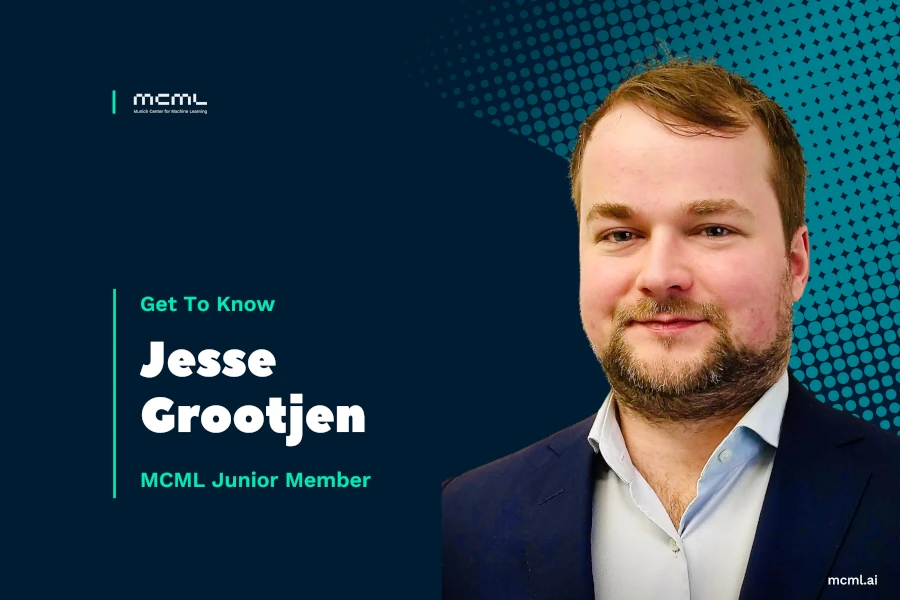
11.12.2024
Understanding Vision Loss and the Need for Early Treatment
Researcher in focus: Jesse Grootjen is writing his doctoral thesis at LMU, focusing on enhancing human abilities through digital technologies.
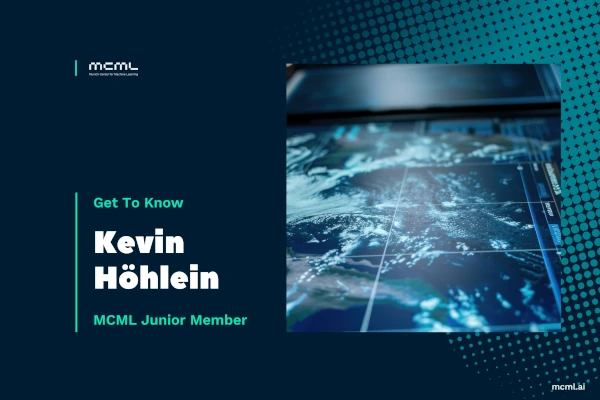
04.12.2024
AI and Weather Predictions
Researcher in focus: Kevin Höhlein, PhD student at TUM, applies data science and machine learning to analyze meteorological data.
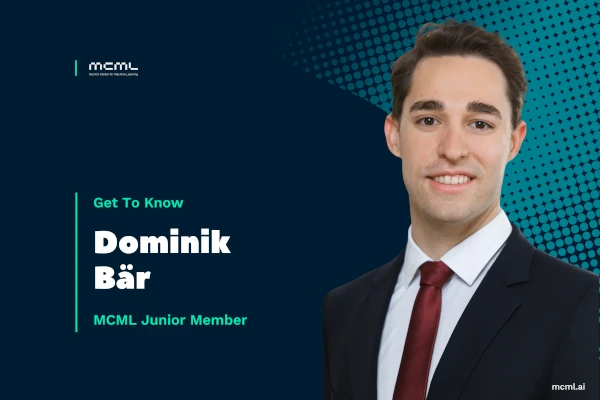
28.11.2024
Enhancing the Integrity of Social Media With AI
Researcher in focus: Dominik Bär, PhD at LMU’s Institute of AI in Management, focuses on social media analytics in Stefan Feuerriegel's group.
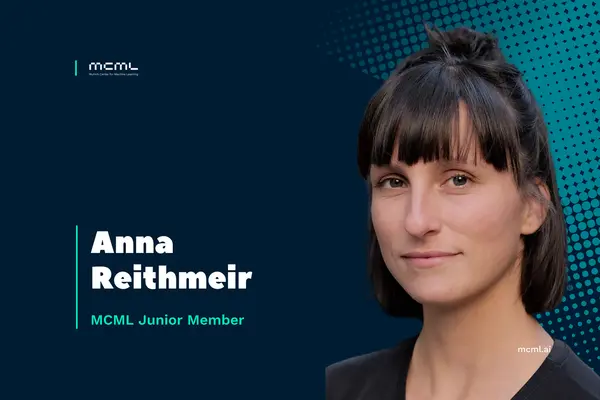
12.11.2024
Exploring the Impact of AI in Medicine
Researcher in focus: Anna Reithmeir specializes in image alignment research to track anatomical changes like tumor growth.