04.12.2024
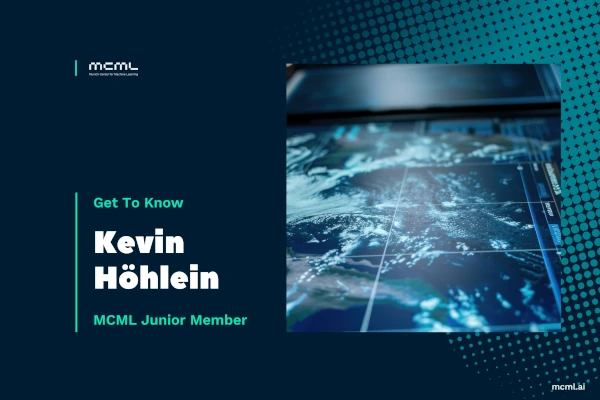
AI and Weather Predictions
Researcher in Focus: Kevin Höhlein
MCML Junior Member Kevin Höhlein is a PhD student at the Chair of Computer Graphics and Visualization (TUM), researching applications of data science and machine learning techniques in the context of meteorological data analysis.
What is the focus of your research?
I work on data-driven methods for analyzing and improving the quality of weather predictions. My research focuses mainly on applying machine learning and deep learning techniques.
What are current challenges in weather prediction, and where can AI help solve them?
«I work on data-driven methods for analyzing and improving the quality of weather predictions.»
Kevin Höhlein
MCML Junior Member
Weather prediction is challenging because it involves many different kinds of physical phenomena and uncertainties. Meteorologists have come very far with building numerical weather models, which can accurately simulate future weather. Nevertheless, there is always room for improvements, e.g., concerning simulation accuracy and a better understanding of the limits of the current methods. AI models, especially those based on deep learning, open a whole new toolbox with opportunities to fix shortcomings of the current models and develop new applications. For example, AI models can help to build faster and more detailed weather models and can be used to correct statistical errors in the current models predictions.
How has deep learning influenced the field of weather prediction and meteorological data analysis? Can you share specific examples from your
research?
«One of my projects addressed the resolution enhancement of weather simulations, i.e., deriving high-resolution local weather forecasts based on coarser simulation outputs.»
Kevin Höhlein
MCML Junior Member
Weather prediction has relied on data-driven methods and machine learning for quite a long time. However, deep learning has opened new research directions that were difficult or unfeasible with previous methods. For example, several research groups have recently developed deep-learning models that can produce more accurate weather predictions than traditional numerical weather models in specific prediction scenarios. With the adoption of deep learning methodologies, meteorology has also attracted interest from a large new research community, AI researchers and computer scientists, who bring in new ideas and accelerate the pace of developments. This interdisciplinary exchange was also the basic idea behind my research. Weather prediction datasets have a specific physical and meteorological interpretation, but the underlying data items are structurally similar to those encountered in computer science. For example, the output of weather simulations looks very similar to digital image or video data in terms of data types and representations. Such similarities can be used to develop new modeling approaches for weather prediction based on similar methods designed originally with other applications in mind. One of my projects, for example, addressed the resolution enhancement of weather simulations, i.e., deriving high-resolution local weather forecasts based on coarser simulation outputs. In light of the parallel to digital imaging, this task appears similar to image superresolution. Image superresolution is concerned with increasing the pixel density of digital images, such as photographs, and deep learning methods work very well for this. To use this knowledge, I reviewed deep learning approaches for image superresolution and adapted the models for the meteorological application.
Explainability is becoming increasingly important in AI. How do you address the challenge of making machine learning predictions in meteorological data understandable and transparent?
«The explainability challenge in meteorology often starts before the machine learning model even sees the data.»
Kevin Höhlein
MCML Junior Member
The explainability challenge in meteorology often starts before the machine learning model even sees the data. Weather prediction datasets are usually large and have a complex structure. Exploring and visualizing such data interactively in graphical software tools helps to understand what information is in there and which aspects might be helpful in subsequent machine-learning tasks. Developing methods for this is a main research direction at the Chair of Computer Graphics and Visualization, where I am working. For subsequent modeling tasks, exploration and visualization are equally important. Meteorologists are interested in understanding why the models arrive at specific predictions and how data and physical principles back these. In my projects, for example, I usually worked with comprehensive datasets with many different parameters as sources of predictive information. Across several modeling tasks, an important question has been which parameters are important for the prediction and which might even harm the prediction quality. This information would help validate the model predictions against physics-based plausibility arguments and highlight unexpected dependencies. My colleagues and I used score-based statistical methods to obtain an overview of the average importance relations in many different prediction scenarios and more detailed attribution methods to drill down in specific cases.
What role does probabilistic modeling play in weather prediction, and how do you integrate it with deep learning techniques in your work?
«Probabilities are a native framework for formulating weather forecasts because of the inherent uncertainties in weather prediction.»
Kevin Höhlein
MCML Junior Member
Probabilities are a native framework for formulating weather forecasts because of the inherent uncertainties in weather prediction. For example, Earth observations are usually imperfect, and the numerical weather models are subject to model errors. Therefore, future predictions must be aware of uncertainties, as well. Probabilistic modeling became relevant in my work when I was trying to improve the quality of such uncertainty-aware weather predictions, so-called ensemble forecasts. An ensemble forecast consists of multiple independent weather simulations, which provide an overview of the type and probability of plausible weather scenarios. I studied deep learning models, which map ensemble forecasts, i.e., a set of possible weather scenarios, to a refined representation of the predicted probabilities. The representation of the forecast distribution and the model training based on actual weather observations are where probabilistic modeling comes into play.
What advancements in machine learning do you foresee having the greatest impact on the future of weather prediction?
There has been a lot of progress in deep learning methods for meteorology recently, and a lot is still happening. So, it is not easy to foresee the next significant advancements. Open research questions concern the consistency of deep learning models with the laws of physics, the explainability of the models’ predictions, and the reliability of data-driven models in changing climate conditions. All of this is subject to ongoing research, and it will be exciting to see the upcoming developments.
About Kevin Höhlein:
Kevin Höhlein is pursuing his doctoral research at TUM. His work emphasizes probabilistic modeling to enhance ensemble weather forecasts, utilizing deep learning techniques to better understand uncertainties in weather predictions. In his free time, Kevin enjoys various outdoor activities, including hiking and biking in and around Munich, as well as in the Alps.
04.12.2024
Related
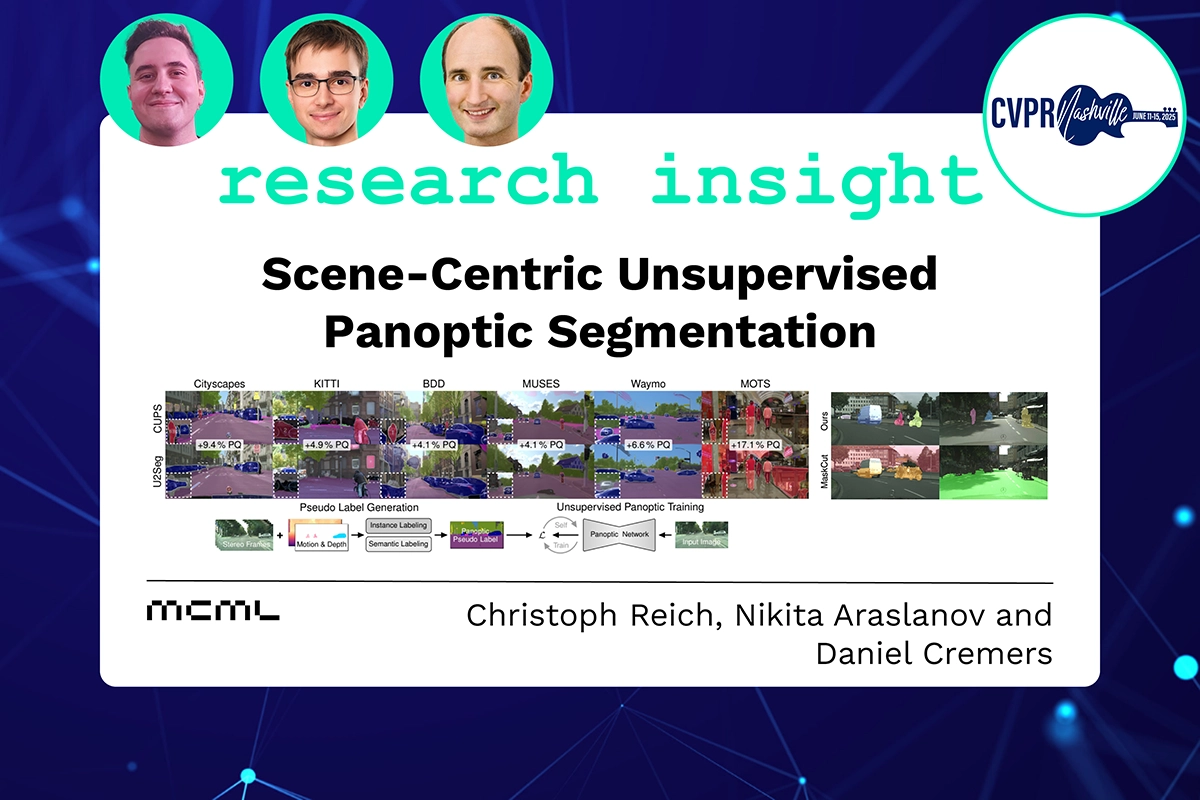
03.04.2025
CUPS: Teaching AI to Understand Scenes Without Human Labels
The team of MCML Director Daniel Cremers introduces CUPS, proving that AI can learn to understand entire scenes - without any human labels.
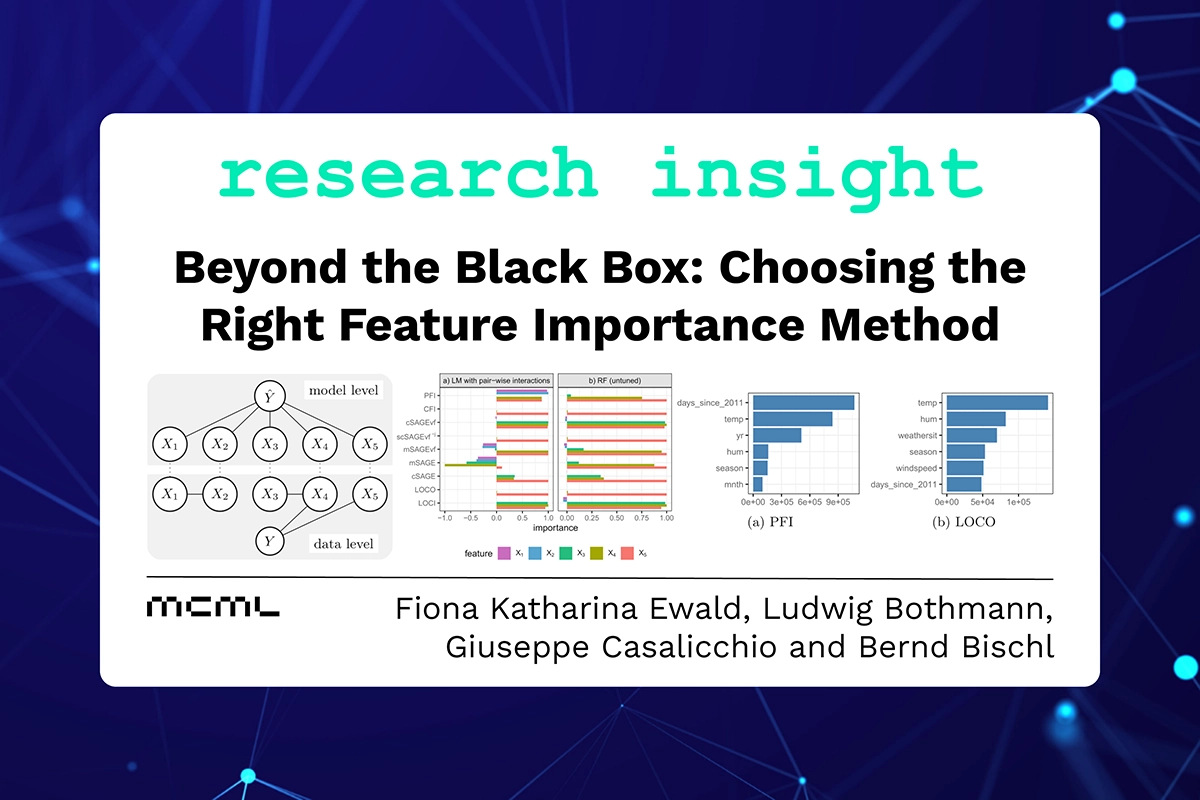
27.03.2025
Beyond the Black Box: Choosing the Right Feature Importance Method
The team of Bernd Bischl created a clear guide to feature importance methods, helping researchers and practitioners interpret AI models effectively.
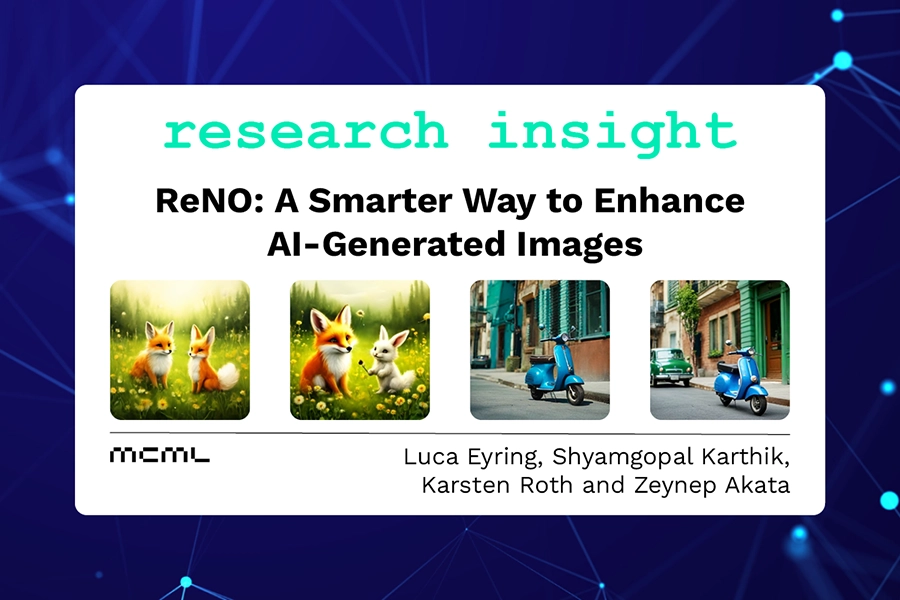
13.03.2025
ReNO: A Smarter Way to Enhance AI-Generated Images
The team of MCML PI Zeynep Akata developed ReNO that enhances image generation without requiring expensive model retraining.
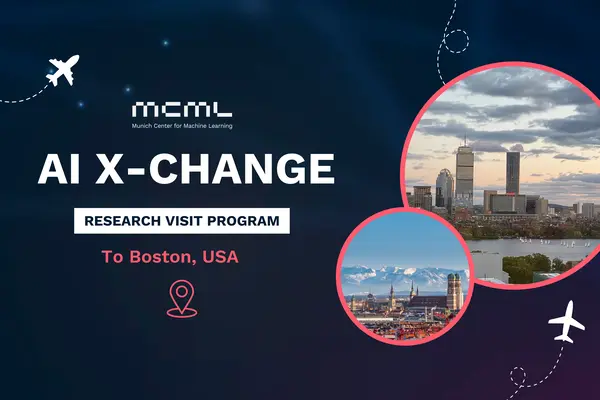
©MCML
04.03.2025
Research at EWCS at the Broad Institute of MIT and Harvard
Research stay at Broad Institute: Exploring causality in biology, microbial interactions, and ML applications through MCML AI X-change program.