12.11.2024
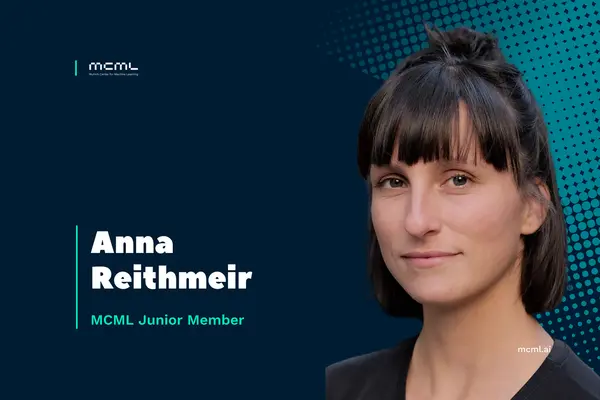
Exploring the Impact of AI in Medicine
Researcher in Focus: Anna Reithmeir
MCML Junior Member Anna Reithmeir is writing her doctoral thesis at the Chair of “Computational Imaging and AI in Medicine” at TUM. Her work addresses the complexity of aligning images from different times or modalities, enabling the comparison of anatomical changes such as tumor growth.
Hello Anna! What is the focus of your research?
«For my doctoral research, I am focusing on Computer Vision and Medical Imaging. Specifically, I am working on the registration of medical images, such as MRI or CT scans.»
Anna Reithmeir
MCML Junior Member
For my doctoral research, I am focusing on Computer Vision and Medical Imaging. Specifically, I am working on the registration of medical images, such as MRI or CT scans. Registration is when you align images - that have been taken at different times - to make them comparable. This allows us to observe how the body has changed over time, for example, how a tumor has grown. While this might sound straightforward, it is a quite complex problem. I focus on regularization of the registration process to incorporate additional knowledge, such as physical information about the body, how it moves, how organs move, and which anatomical structures are present. An emerging approach to effectively regularize this optimization problem is to use AI.
Can you specify a little bit: Why is it so complicated to compare two tumor images? And where does your research come into play?
«My research explores how suitable regularization can be learned in an unsupervised manner from a set of training images.»
Anna Reithmeir
MCML Junior Member
When aligning two images of different modalities such as a CT image to an MR image, some anatomical structures are better visible in the one image than in the other. Also, image registration is an inherently under-defined problem, meaning that often there is not one but multiple optimal solutions. This is why one needs regularization, which incorporates desired deformation properties in the optimization. However, human body motion is very complicated and generally there is no ground truth information available. Here, my research comes into play: It explores how suitable regularization can be learned in an unsupervised manner from a set of training images.
What is the benefit of additional knowledge about physical information regarding the body? How can this be studied?
«The close collaboration with the radiology department of the Klinikum Rechts der Isar gives me the opportunity to develop physiologically accurate regularization methods and also to evaluate novel methods on real medical data.»
Anna Reithmeir
MCML Junior Member
Precise registration is essential for clinical downstream tasks. Physical knowledge about anatomical structures, such as their material and deformation properties help the registration in finding an anatomically plausible solution. A simple example is that bones generally move rigidly while soft tissue can deform non-linearly. Naturally, such properties should be reflected in the obtained deformation. This gets even more difficult for the registration of pre- and post-operative images, where regions of the body have been removed, or contrast-enhanced images, where a contrast agent spreads throughout the body. Other challenges are that physical properties are highly patient-specific and not straightforward to model, and the lack of open-source data.
In my research I study this problem in close collaboration with the radiology department of the Klinikum Rechts der Isar. This gives me the opportunity to develop physiologically accurate regularization methods and also to evaluate novel methods on real medical data.
To what extent is AI already being used in practice in medicine?
In my experience, the research on AI in medical image registration and medical imaging in general is growing and advancing quickly. But I have the feeling that the transfer to clinical practice is still in its infancy. For example in terms of registration, radiologists still manually compare two 3d image volumes by scrolling through the images.
What do you like most about your field of research?
In the medical field, it’s especially rewarding to have a direct positive impact. You know that the problems you’re working on are genuinely beneficial to society. Also, I see a strong need for digitalization and technological innovation in the clinics and I am very happy to contribute to that.
What are your academic and personal goals?
There are two goals. On the research side, I would like to develop novel methods that advance image registration, possibly with the help of AI, though not necessarily. Ideally, I would apply and evaluate these methods in the clinical context. My personal goal is to learn how to make complex research understandable to others, especially to young people or those not (yet) involved in computer science research.
Have you already done something in this regard?
Last semester, I gave a seminar that I completely redesigned from scratch, with a colleague of mine. I really liked this whole process –from structuring the seminar to guiding the students to a successful final poster presentation. Through the MCML, I also organized a workshop for Girl’s Day last year. I also participated in a school workshop to give students some insights into research work and AI in general.
When you are not conducting research, how do you spend your free time in Munich?
I’m originally from Bonn and came to Munich for my Bachelor’s degree. Apart from a semester abroad I’ve stayed here until now. Munich is a really beautiful city and I am really enjoying it here. When I’m not researching, I’m either spending time at the Isar, climbing, or cycling in the mountains on my road bike. You just have to take advantage of having the Alps right on your doorstep!
About Anna Reithmeir:
Anna Reithmeir is working on her doctoral thesis at TUM. She is supervised by MCML PI Julia Schnabel. Her research focuses on advancing medical image registration, with the aim of developing image registration methods that could potentially be applied in clinical practice.
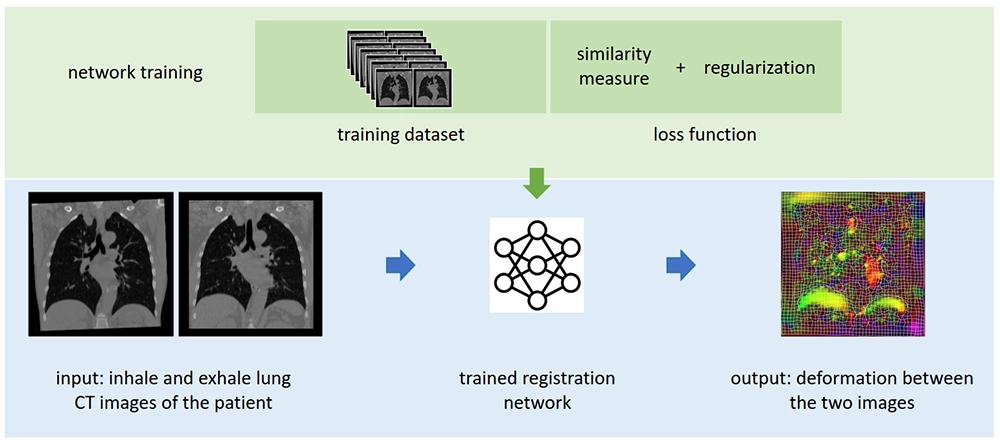
©Anna Reithmeir
Registration Network
A registration network is trained with a large dataset of paired images and a training loss function. Once trained, the network can in a few seconds predict the deformation between two given images of a patient, such as CT lung images at different breathing states. To ensure meaningful deformations, regularization is used in the training function, for example, to reflect the up-and-down breathing motion of the lungs in the predicted deformation. In her research, Anna works on including physics-inspired regularization and regularization that is itself learned with a second neural network.
12.11.2024
Related
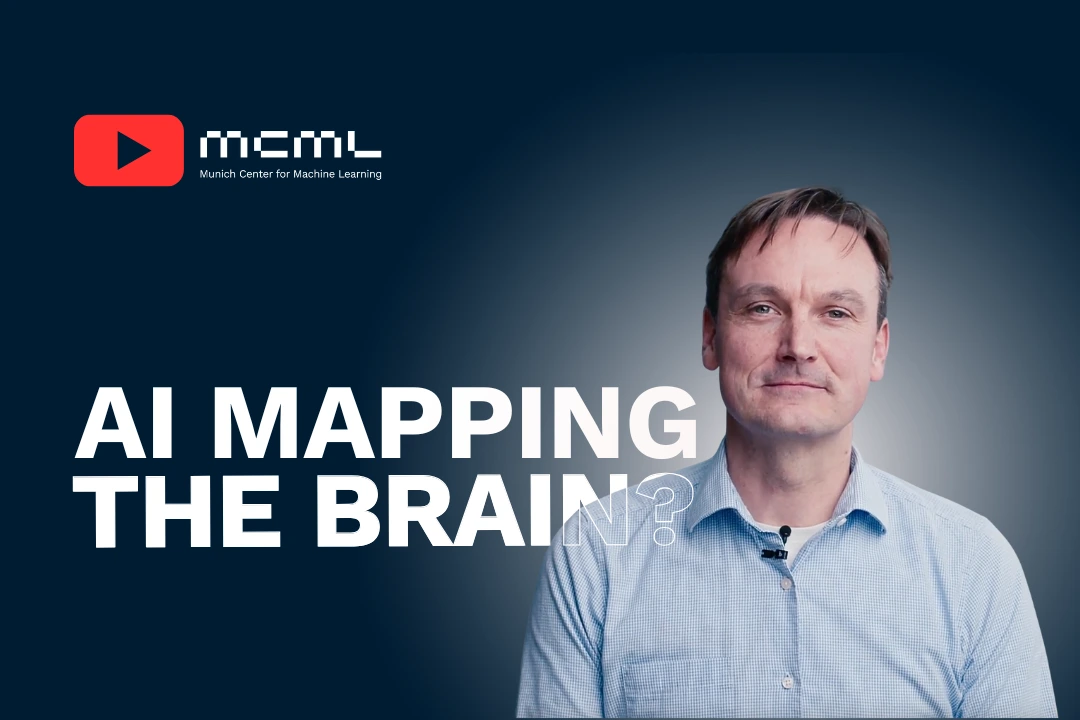
16.07.2025
AI-Powered Cortical Mapping for Neurodegenerative Disease Diagnoses - With Christian Wachinger
Research film with Christian Wachinger shows how AI maps the brain’s cortex to support diagnoses of neurodegenerative diseases.
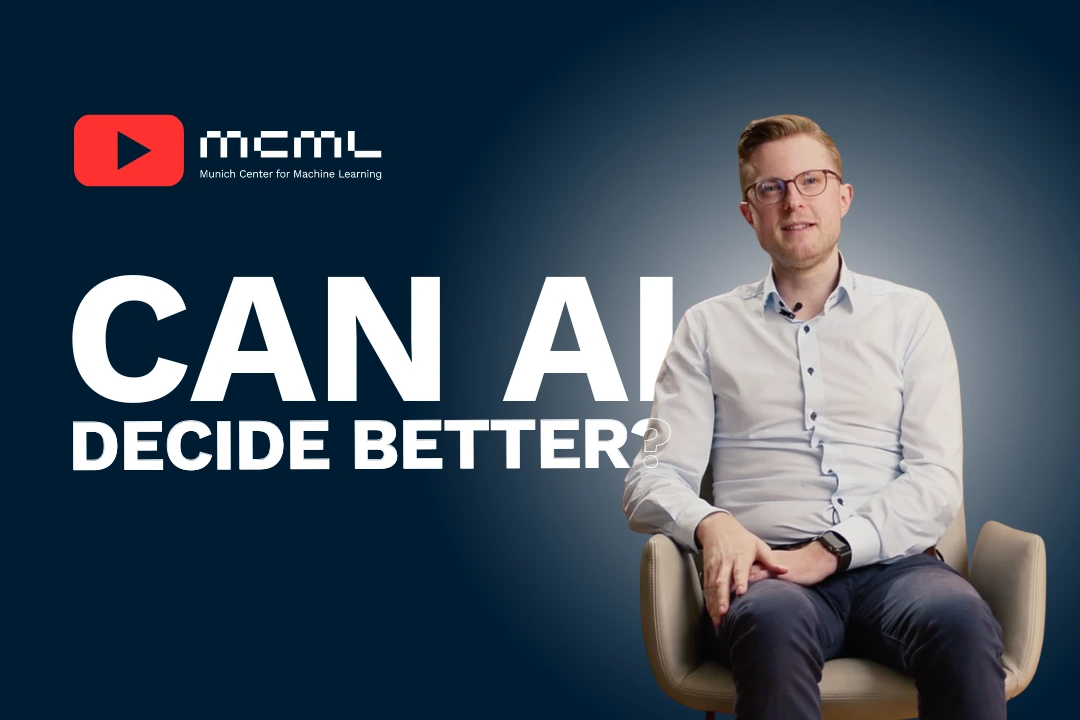
10.07.2025
Beyond Prediction: How Causal AI Enables Better Decision-Making - With Stefan Feuerriegel
Stefan Feuerriegel in our new film shows how Causal AI helps pick better actions by predicting outcomes for each possible decision.
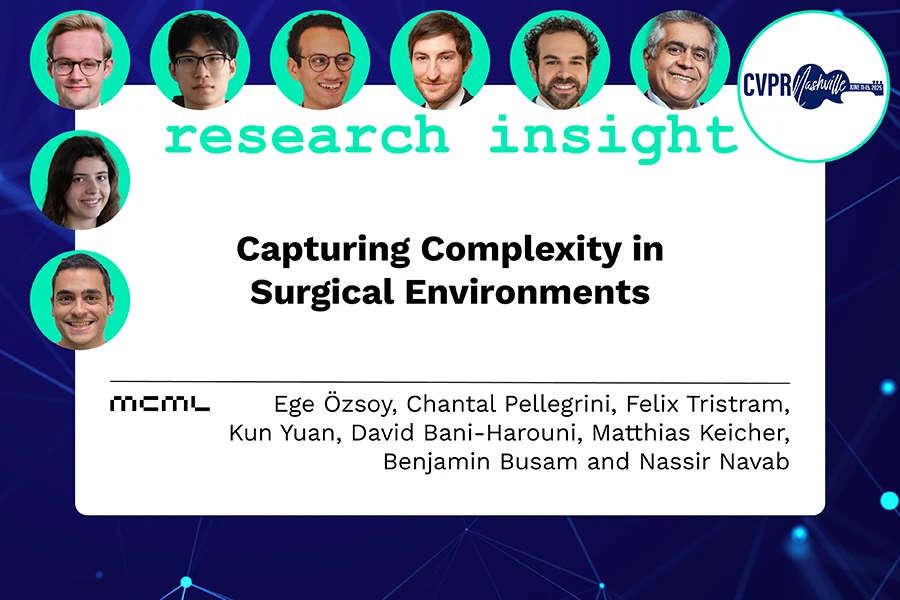
09.07.2025
Capturing Complexity in Surgical Environments
Published at CVPR 2025, MM-OR is a multimodal dataset of robotic knee surgeries, capturing OR dynamics via video, audio, tracking, and robot logs.
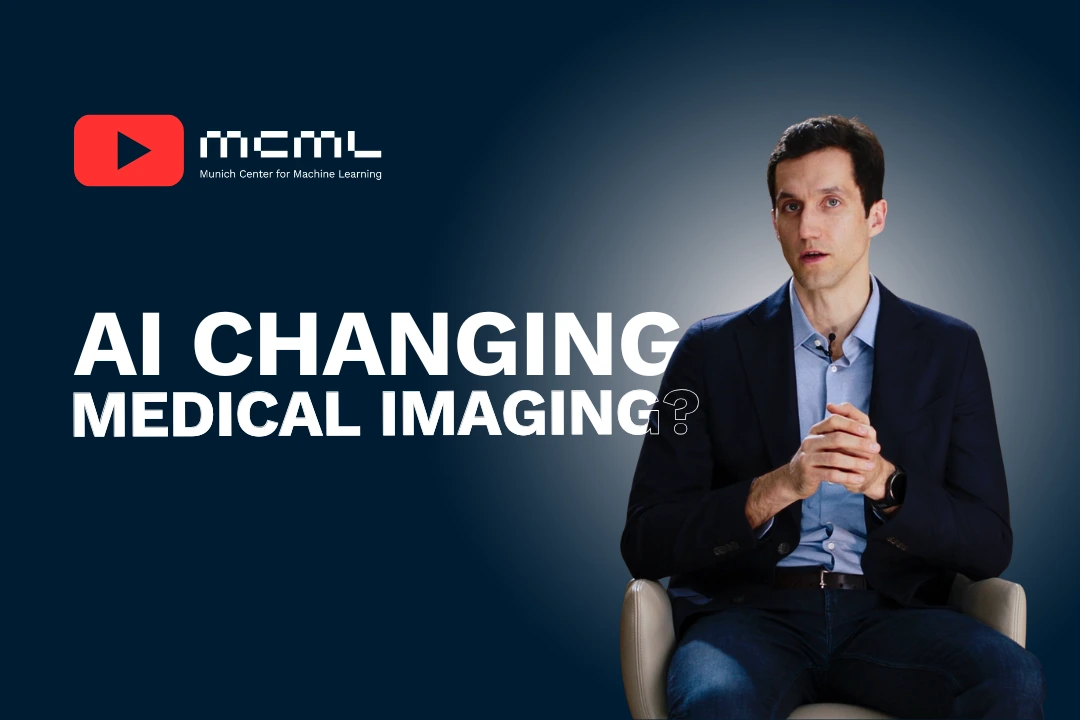
06.07.2025
How Neural Networks Are Changing Medical Imaging – With Reinhard Heckel
In the new research film, Reinhard Heckel shows how AI enables sharper heart imaging from limited or noisy data.