18.10.2024
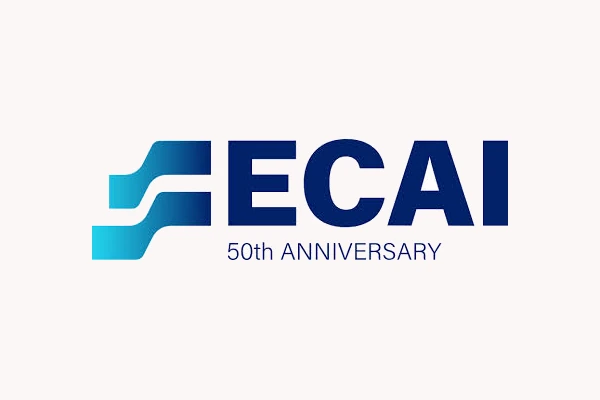
MCML researchers with three papers at ECAI 2024
27th European Conference on Artificial Intelligence (ECAI 2024). Santiago de Compostela, Spain, 19.10.2024 - 24.10.2024
We are happy to announce that MCML researchers are represented with three papers at ECAI 2024:
M. Bernhard, T. Hannan, N. Strauß and M. Schubert.
Context Matters: Leveraging Spatiotemporal Metadata for Semi-Supervised Learning on Remote Sensing Images.
DOI. GitHub.
Abstract
Remote sensing projects typically generate large amounts of imagery that can be used to train powerful deep neural networks. However, the amount of labeled images is often small, as remote sensing applications generally require expert labelers. Thus, semi-supervised learning (SSL), i.e., learning with a small pool of labeled and a larger pool of unlabeled data, is particularly useful in this domain. Current SSL approaches generate pseudo-labels from model predictions for unlabeled samples. As the quality of these pseudo-labels is crucial for performance, utilizing additional information to improve pseudo-label quality yields a promising direction. For remote sensing images, geolocation and recording time are generally available and provide a valuable source of information as semantic concepts, such as land cover, are highly dependent on spatiotemporal context, e.g., due to seasonal effects and vegetation zones. In this paper, we propose to exploit spatiotemporal metainformation in SSL to improve the quality of pseudo-labels and, therefore, the final model performance. We show that directly adding the available metadata to the input of the predictor at test time degenerates the prediction quality for metadata outside the spatiotemporal distribution of the training set. Thus, we propose a teacher-student SSL framework where only the teacher network uses metainformation to improve the quality of pseudo-labels on the training set. Correspondingly, our student network benefits from the improved pseudo-labels but does not receive metadata as input, making it invariant to spatiotemporal shifts at test time. Furthermore, we propose methods for encoding and injecting spatiotemporal information into the model and introduce a novel distillation mechanism to enhance the knowledge transfer between teacher and student. Our framework dubbed Spatiotemporal SSL can be easily combined with several state-of-the-art SSL methods, resulting in significant and consistent improvements on the BigEarthNet and EuroSAT benchmarks.Y. Liu, F. Shi, D. Wang, Y. Zhang and H. Schütze.
ChatZero: Zero-Shot Cross-Lingual Dialogue Generation via Pseudo-Target Language.
DOI.
Abstract
Although large language models(LLMs) show amazing capabilities, among various exciting applications discovered for LLMs fall short in other low-resource languages. Besides, most existing methods depend on large-scale dialogue corpora and thus building systems for dialogue generation in a zero-shot scenario remains a considerable challenge. To address this challenge, we propose a novel end-to-end zero-shot dialogue generation model ChatZero based on cross-lingual code-switching method. First, we construct code-switching language and pseudo-target language with placeholders. Then for cross-lingual semantic transfer, we employ unsupervised contrastive learning to minimize the semantics gap of the source language, code-switching language, and pseudo-target language that are mutually positive examples in the high dimensional semantic space. Experiments on the multilingual DailyDialog and DSTC7-AVSD datasets demonstrate that ChatZero can achieve more than 90% of the original performance under the zero-shot case compared to supervised learning, and achieve state-of-the-art performance compared with other baselines.J. Nama, I. Chalkidisb and M. Rezaei.
Hyperbolic Contrastive Learning for Document Representations – A Multi-View Approach with Paragraph-Level Similarities.
DOI.
Abstract
Self-supervised learning (SSL) has gained prominence due to the increasing availability of unlabeled data and advances in computational efficiency, leading to revolutionized natural language processing with pre-trained language models like BERT and GPT. Representation learning, a core concept in SSL, aims to reduce data dimensionality while preserving meaningful aspects. Conventional SSL methods typically embed data in Euclidean space. However, recent research has revealed that alternative geometries can hold even richer representations, unlocking more meaningful insights from the data. Motivated by this, we propose two novel methods for integrating Hilbert geometry into self-supervised learning for efficient document embedding. First, we present a method directly incorporating Hilbert geometry into the standard Euclidean contrastive learning framework. Additionally, we propose a multi-view hyperbolic contrastive learning framework contrasting both documents and paragraphs. Our findings demonstrate that contrasting only paragraphs, rather than entire documents, can lead to superior efficiency and effectiveness.18.10.2024
Related
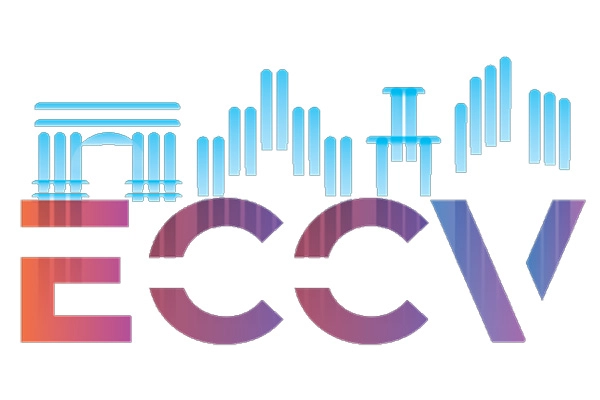
26.09.2024
MCML at ECCV 2024
We are happy to announce that MCML researchers are represented at the 18th European Conference on Computer Vision (ECCV 2024).
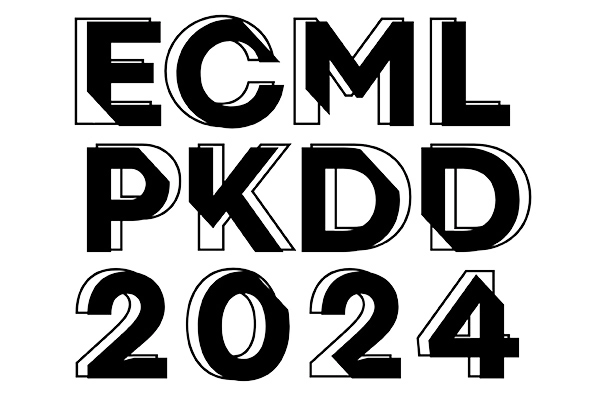
10.09.2024
MCML at ECML-PKDD 2024
We are happy to announce that MCML researchers are represented at ECML-PKDD 2024.
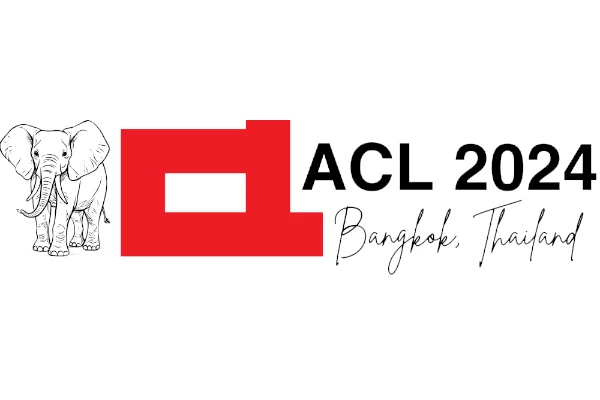
14.08.2024
MCML at ACL 2024
We are happy to announce that MCML researchers are represented at the 62nd Annual Meeting of the Association for Computational Linguistics (ACL 2024).
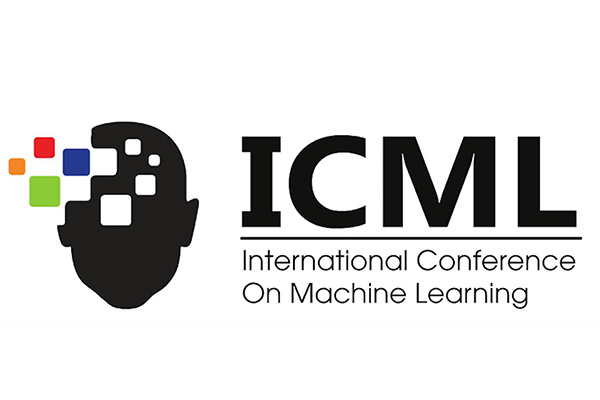
20.07.2024
MCML at ICML 2024
We are happy to announce that MCML researchers are represented at the 41st International Conference on Machine Learning (ICML 2024).
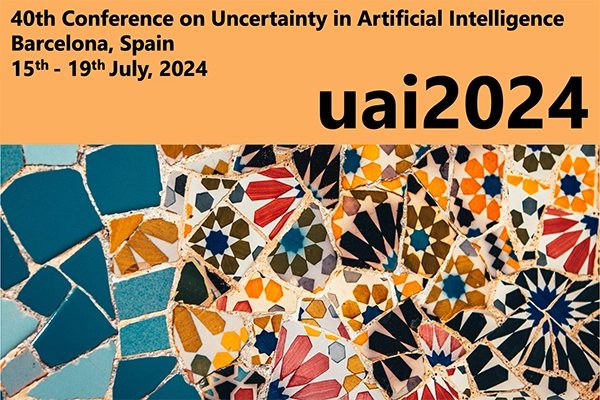
15.07.2024
MCML at UAI 2024
We are happy to announce that MCML researchers are represented at the 40th Conference on Uncertainty in Artificial Intelligence (UAI 2024).