05.10.2023
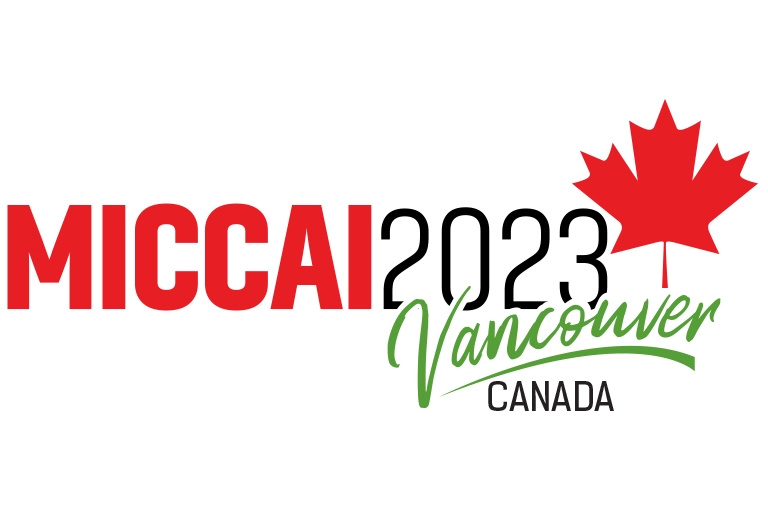
MCML researchers with two papers at MICCAI 2023
26th International Conference on Medical Image Computing and Computer Assisted Intervention (MICCAI 2023). Vancouver, Canada, 08.10.2023–12.10.2023
We are happy to announce that MCML researchers are represented with two papers at MICCAI 2023:
NISF: Neural implicit segmentation functions.
26th International Conference on Medical Image Computing and Computer Assisted Intervention (MICCAI 2023). Vancouver, Canada, Oct 08-12, 2023. DOI.
Abstract
Segmentation of anatomical shapes from medical images has taken an important role in the automation of clinical measurements. While typical deep-learning segmentation approaches are performed on discrete voxels, the underlying objects being analysed exist in a real-valued continuous space. Approaches that rely on convolutional neural networks (CNNs) are limited to grid-like inputs and not easily applicable to sparse or partial measurements. We propose a novel family of image segmentation models that tackle many of CNNs’ shortcomings: Neural Implicit Segmentation Functions (NISF). Our framework takes inspiration from the field of neural implicit functions where a network learns a mapping from a real-valued coordinate-space to a shape representation. NISFs have the ability to segment anatomical shapes in high-dimensional continuous spaces. Training is not limited to voxelized grids, and covers applications with sparse and partial data. Interpolation between observations is learnt naturally in the training procedure and requires no post-processing. Furthermore, NISFs allow the leveraging of learnt shape priors to make predictions for regions outside of the original image plane. We go on to show the framework achieves dice scores of on a (3D+t) short-axis cardiac segmentation task using the UK Biobank dataset. We also provide a qualitative analysis on our frameworks ability to perform segmentation and image interpolation on unseen regions of an image volume at arbitrary resolutions.
MCML Authors
Anatomy-Aware Masking for Inpainting in Medical Imaging.
3rd Workshop on Shape in Medical Imaging (ShapeMI 2023) at the 26th International Conference on Medical Image Computing and Computer Assisted Intervention (MICCAI 2023). Vancouver, Canada, Oct 08-12, 2023. DOI. GitHub.
Abstract
Inpainting has recently been employed as a successful deep-learning technique for unsupervised model discovery in medical image analysis by taking advantage of the strong priors learned by models to reconstruct the structure and texture of missing parts in images. Even though the learned features depend on the masks as well as the images, the masks used for inpainting are typically random and independent of the dataset, due to the unpredictability of the content of images, i.e., different objects and shapes can appear in different locations in images. However, this is rarely the case for medical imaging data since they are obtained from similar anatomies. Still, random square masks are the most popular technique for inpainting in medical imaging. In this work, we propose a pipeline to generate, position and sample the masks to efficiently learn the shape and structures of the anatomy and generate a myriad of diverse anatomy-aware masks, aiding the model in learning the statistical shape prior to the topology of the organs of interest. We demonstrate the impact of our approach compared to other masking mechanisms in the reconstruction of anatomy. We compare the effectiveness of our proposed masking approach over square-shaped masks, which are traditionally used in medical imaging, and irregular shape masks, which are used in SOTA inpainting literature.
MCML Authors
05.10.2023
Related
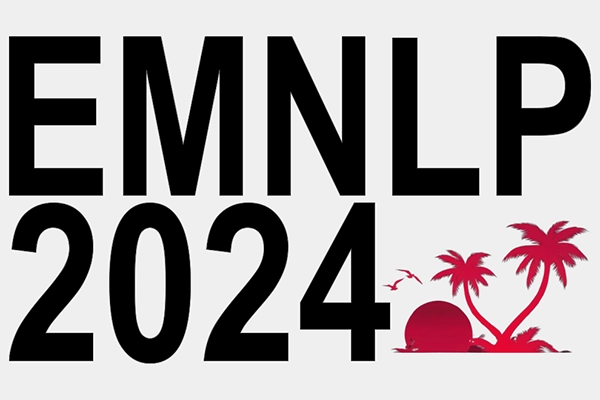
06.11.2024
MCML researchers with 20 papers at EMNLP 2024
Conference on Empirical Methods in Natural Language Processing (EMNLP 2024). Miami, FL, USA, 12.11.2024 - 16.11.2024
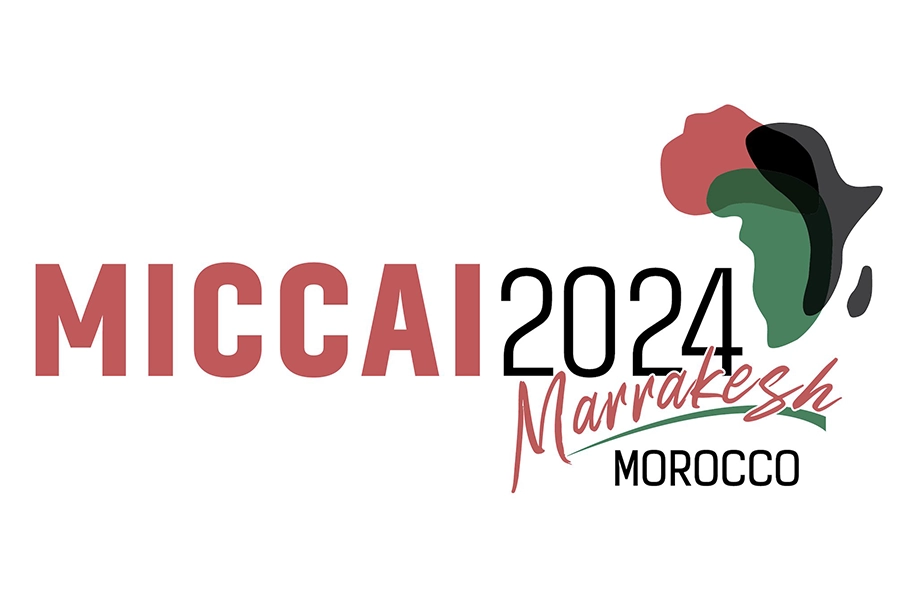
01.10.2024
MCML researchers with 16 papers at MICCAI 2024
27th International Conference on Medical Image Computing and Computer Assisted Intervention (MICCAI 2024). Marrakesh, Morocco, 06.10.2024 - 10.10.2024
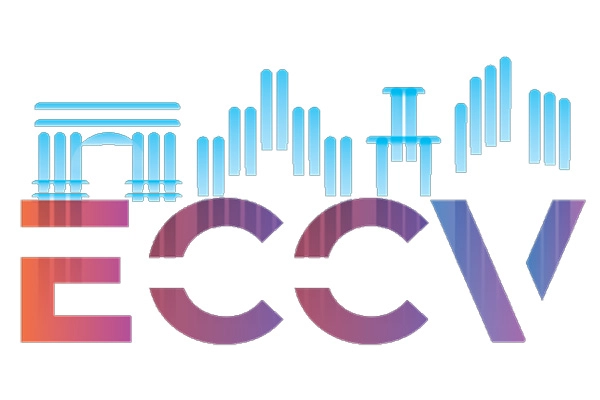
26.09.2024
MCML researchers with 18 papers at ECCV 2024
18th European Conference on Computer Vision (ECCV 2024). Milano, Italy, 29.09.2024 - 04.10.2024
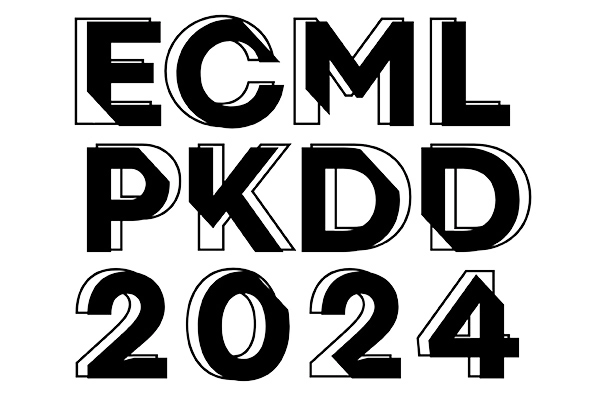
10.09.2024
MCML at ECML-PKDD 2024
We are happy to announce that MCML researchers are represented at ECML-PKDD 2024.
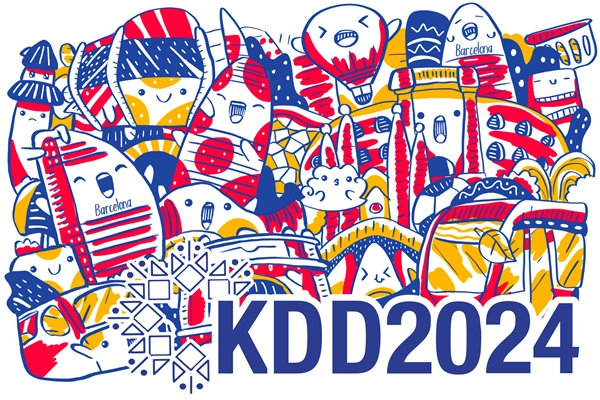
20.08.2024
MCML researchers with two papers at KDD 2024
30th ACM SIGKDD International Conference on Knowledge Discovery and Data (KDD 2024). Barcelona, Spain, 25.08.2024 - 29.08.2024