14.06.2024
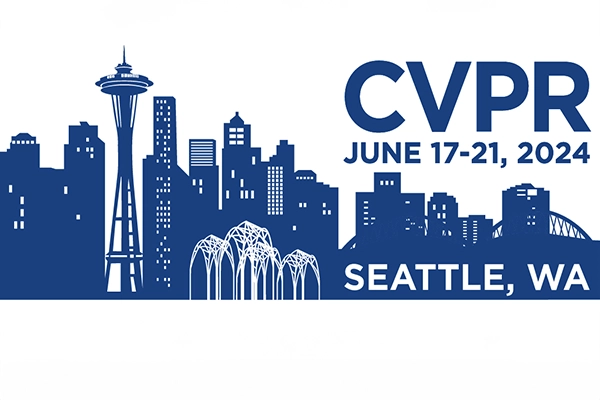
MCML Researchers With 16 Papers at CVPR 2024
IEEE/CVF Conference on Computer Vision and Pattern Recognition (CVPR 2024). Seattle, WA, USA, 17.06.2024–21.06.2024
We are happy to announce that MCML researchers are represented with 16 papers at CVPR 2024. Congrats to our researchers!
Sparse Views, Near Light: A Practical Paradigm for Uncalibrated Point-light Photometric Stereo.
CVPR 2024 - IEEE/CVF Conference on Computer Vision and Pattern Recognition. Seattle, WA, USA, Jun 17-21, 2024. DOI
Abstract
Neural approaches have shown a significant progress on camera-based reconstruction. But they require either a fairly dense sampling of the viewing sphere, or pre-training on an existing dataset, thereby limiting their generalizability. In contrast, photometric stereo (PS) approaches have shown great potential for achieving high-quality reconstruction under sparse viewpoints. Yet, they are impractical because they typically require tedious laboratory conditions, are restricted to dark rooms, and often multi-staged, making them subject to accumulated errors. To address these shortcomings, we propose an end-to-end uncalibrated multi-view PS frameworkfor reconstructing high-resolution shapes acquiredfrom sparse viewpoints in a real-world environment. We relax the dark room assumption, and allow a combination of static ambient lighting and dynamic near LED lighting, thereby enabling easy data capture outside the lab. Experimental validation confirms that it outperforms existing baseline approaches in the regime of sparse viewpoints by a large margin. This allows to bring high-accuracy 3D reconstruction from the dark room to the real world, while maintaining a reasonable data capture complexity.
MCML Authors
SecondPose: SE(3)-Consistent Dual-Stream Feature Fusion for Category-Level Pose Estimation.
CVPR 2024 - IEEE/CVF Conference on Computer Vision and Pattern Recognition. Seattle, WA, USA, Jun 17-21, 2024. DOI
Abstract
Category-level object pose estimation, aiming to predict the 6D pose and 3D size of objects from known categories, typically struggles with large intra-class shape variation. Existing works utilizing mean shapes often fall short of cap-turing this variation. To address this issue, we present Sec-ondPose, a novel approach integrating object-specific ge-ometric features with semantic category priors from DI-NOv2. Leveraging the advantage of DINOv2 in providing SE(3)-consistent semantic features, we hierarchically extract two types of SE(3)-invariant geometric features to further encapsulate local-to-global object-specific information. These geometric features are then point-aligned with DINOv2 features to establish a consistent object represen-tation under SE(3) transformations, facilitating the map-ping from camera space to the pre-defined canonical space, thus further enhancing pose estimation. Extensive exper-iments on NOCS-REAL275 demonstrate that SecondPose achieves a 12.4% leap forward over the state-of-the-art. Moreover, on a more complex dataset HouseCat6D which provides photometrically challenging objects, SecondPose still surpasses other competitors by a large margin.
MCML Authors
Computer Aided Medical Procedures & Augmented Reality
Computer Aided Medical Procedures & Augmented Reality
Partial-to-Partial Shape Matching with Geometric Consistency.
CVPR 2024 - IEEE/CVF Conference on Computer Vision and Pattern Recognition. Seattle, WA, USA, Jun 17-21, 2024. DOI GitHub
Abstract
Finding correspondences between 3D shapes is an important and long-standing problem in computer vision, graphics and beyond. A prominent challenge are partial-to-partial shape matching settings, which occur when the shapes to match are only observed incompletely (e.g. from 3D scanning). Although partial-to-partial matching is a highly relevant setting in practice, it is rarely explored. Our work bridges the gap between existing (rather artificial) 3D full shape matching and partial-to-partial real-world set-tings by exploiting geometric consistency as a strong constraint. We demonstrate that it is indeed possible to solve this challenging problem in a variety of settings. For the first time, we achieve geometric consistency for partial-to-partial matching, which is realized by a novel integer non-linear program formalism building on triangle prod-uct spaces, along with a new pruning algorithm based on linear integer programming. Further, we generate a new inter-class dataset for partial-to-partial shape-matching. We show that our method outperforms current SOTA meth-ods on both an established intra-class dataset and our novel inter-class dataset.
MCML Authors
H-ViT: A Hierarchical Vision Transformer for Deformable Image Registration.
CVPR 2024 - IEEE/CVF Conference on Computer Vision and Pattern Recognition. Seattle, WA, USA, Jun 17-21, 2024. DOI GitHub
Abstract
This paper introduces a novel top-down representation approach for deformable image registration, which estimates the deformation field by capturing various short-and long-range flow features at different scale levels. As a Hierarchical Vision Transformer (H-ViT), we propose a dual self-attention and cross-attention mechanism that uses high-level features in the deformation field to represent low-level ones, enabling information streams in the deformation field across all voxel patch embeddings irrespective of their spatial proximity. Since high-level features contain abstract flow patterns, such patterns are expected to effectively contribute to the representation of the deformation field in lower scales. When the self-attention module utilizes within-scale short-range patterns for representation, the cross-attention modules dynamically look for the key tokens across different scales to further interact with the local query voxel patches. Our method shows superior accuracy and visual quality over the state-of-the-art registration methods in five publicly available datasets, highlighting a substantial enhancement in the performance of medical imaging registration.
MCML Authors
Artificial Intelligence in Medical Imaging
Boosting Self-Supervision for Single-View Scene Completion via Knowledge Distillation.
CVPR 2024 - IEEE/CVF Conference on Computer Vision and Pattern Recognition. Seattle, WA, USA, Jun 17-21, 2024. DOI
Abstract
Inferring scene geometry from images via Structure from Motion is a long-standing and fundamental problem in computer vision. While classical approaches and, more re-cently, depth map predictions only focus on the visible parts of a scene, the task of scene completion aims to reason about geometry even in occluded regions. With the popularity of neural radiance fields (NeRFs), implicit represen-tations also became popular for scene completion by pre-dicting so-called density fields. Unlike explicit approaches e.g. voxel-based methods, density fields also allow for ac-curate depth prediction and novel-view synthesis via image-based rendering. In this work, we propose to fuse the scene reconstruction from multiple images and distill this knowl-edge into a more accurate single-view scene reconstruction. To this end, we propose Multi-View Behind the Scenes (MVBTS) to fuse density fields from multiple posed images, trained fully self-supervised only from image data. Using knowledge distillation, we use MVBTS to train a single-view scene completion network via direct supervision called KDBTS. It achieves state-of-the-art performance on occu-pancy prediction, especially in occluded regions.
MCML Authors
Computer Vision & Artificial Intelligence
Recent Trends Challenges and Limitations of Explainable AI in Remote Sensing.
CVPR 2024 - Workshop at the IEEE/CVF Conference on Computer Vision and Pattern Recognition. Seattle, WA, USA, Jun 17-21, 2024. URL
Abstract
Training deep learning models on remote sensing imagery is an increasingly popular approach for addressing pressing challenges related to urbanization extreme weather events food security deforestation or poverty reduction. Although explainable AI is getting more frequently utilized to uncover the workings of these models a comprehensive summary of how the fundamental challenges in remote sensing are tackled by explainable AI is still missing. By conducting a scoping review we identify the current works and key trends in the field. Next we relate them to recent developments and challenges in remote sensing and explainable AI. By doing so we also point to novel strategies and promising research directions such as the work on self-interpretable deep learning models and explanation evaluation.
MCML Authors
MatchU: Matching Unseen Objects for 6D Pose Estimation from RGB-D Images.
CVPR 2024 - IEEE/CVF Conference on Computer Vision and Pattern Recognition. Seattle, WA, USA, Jun 17-21, 2024. DOI
Abstract
Recent learning methods for object pose estimation require resource-intensive training for each individual object instance or category, hampering their scalability in real applications when confronted with previously unseen objects. In this paper, we propose MatchU, a Fuse-Describe-Match strategy for 6D pose estimation from RGB-D images. MatchU is a generic approach that fuses 2D texture and 3D geometric cues for 6D pose prediction of unseen objects. We rely on learning geometric 3D descriptors that are rotation-invariant by design. By encoding pose-agnostic geometry, the learned descriptors naturally generalize to unseen objects and capture symmetries. To tackle ambiguous associations using 3D geometry only, we fuse additional RGB information into our descriptor. This is achieved through a novel attention-based mechanism that fuses cross-modal information, together with a matching loss that leverages the latent space learned from RGB data to guide the descriptor learning process. Extensive experiments reveal the generalizability of both the RGB-D fusion strategy as well as the descriptor efficacy. Benefiting from the novel designs, MatchU surpasses all existing methods by a significant margin in terms of both accuracy and speed, even without the requirement of expensive re-training or rendering.
MCML Authors
Computer Aided Medical Procedures & Augmented Reality
Computer Aided Medical Procedures & Augmented Reality
HouseCat6D -- A Large-Scale Multi-Modal Category Level 6D Object Perception Dataset with Household Objects in Realistic Scenarios.
CVPR 2024 - IEEE/CVF Conference on Computer Vision and Pattern Recognition. Seattle, WA, USA, Jun 17-21, 2024. DOI
Abstract
Estimating 6D object poses is a major challenge in 3D computer vision. Building on successful instance-level approaches, research is shifting towards category-level pose estimation for practical applications. Current category-level datasets, however, fall short in annotation quality and pose variety. Addressing this, we introduce HouseCat6D, a new category-level 6D pose dataset. It features 1) multi-modality with Polarimetric RGB and Depth (RGBD+P), 2) encompasses 194 diverse objects across 10 household cat-egories, including two photometrically challenging ones, and 3) provides high-quality pose annotations with an error range of only 1.35 mm to 1.74 mm. The dataset also includes 4) 41 large-scale scenes with comprehensive view-point and occlusion coverage,5) a checkerboard-free en-vironment, and 6) dense 6D parallel-jaw robotic grasp annotations. Additionally, we present benchmark results for leading category-level pose estimation networks.
MCML Authors
Computer Aided Medical Procedures & Augmented Reality
Computer Aided Medical Procedures & Augmented Reality
Self-Discovering Interpretable Diffusion Latent Directions for Responsible Text-to-Image Generation.
CVPR 2024 - IEEE/CVF Conference on Computer Vision and Pattern Recognition. Seattle, WA, USA, Jun 17-21, 2024. DOI GitHub
Abstract
Diffusion-based models have gained significant popularity for text-to-image generation due to their exceptional image-generation capabilities. A risk with these models is the potential generation of inappropriate content, such as biased or harmful images. However, the underlying reasons for generating such undesired content from the perspective of the diffusion model’s internal representation remain unclear. Previous work interprets vectors in an interpretable latent space of diffusion models as semantic concepts. However, existing approaches cannot discover directions for arbitrary concepts, such as those related to inappropriate concepts. In this work, we propose a novel self-supervised approach to find interpretable latent directions for a given concept. With the discovered vectors, we further propose a simple approach to mitigate inappropriate generation. Extensive experiments have been conducted to verify the effectiveness of our mitigation approach, namely, for fair generation, safe generation, and responsible text-enhancing generation.
MCML Authors
Contrastive Pretraining for Visual Concept Explanations of Socioeconomic Outcomes.
CVPR 2024 - Workshop at the IEEE/CVF Conference on Computer Vision and Pattern Recognition. Seattle, WA, USA, Jun 17-21, 2024. DOI
Abstract
Predicting socioeconomic indicators from satellite imagery with deep learning has become an increasingly popular research direction. Post-hoc concept-based explanations can be an important step towards broader adoption of these models in policy-making as they enable the interpretation of socioeconomic outcomes based on visual concepts that are intuitive to humans. In this paper, we study the interplay between representation learning using an additional task-specific contrastive loss and post-hoc concept explainability for socioeconomic studies. Our results on two different geographical locations and tasks indicate that the task-specific pretraining imposes a continuous ordering of the latent space embeddings according to the socioeconomic outcomes. This improves the model’s interpretability as it enables the latent space of the model to associate urban concepts with continuous intervals of socioeconomic outcomes. Further, we illustrate how analyzing the model’s conceptual sensitivity for the intervals of socioeconomic outcomes can shed light on new insights for urban studies.
MCML Authors
Deep Video Codec Control for Vision Models.
CVPR 2024 - Workshop at the IEEE/CVF Conference on Computer Vision and Pattern Recognition. Seattle, WA, USA, Jun 17-21, 2024. DOI
Abstract
Standardized lossy video coding is at the core of almost all real-world video processing pipelines. Rate control is used to enable standard codecs to adapt to different network bandwidth conditions or storage constraints. However, standard video codecs (e.g., H.264) and their rate control modules aim to minimize video distortion w.r.t. human quality assessment. We demonstrate empirically that standard-coded videos vastly deteriorate the performance of deep vision models. To overcome the deterioration of vision performance, this paper presents the first end-to-end learnable deep video codec control that considers both bandwidth constraints and downstream deep vision performance, while adhering to existing standardization. We demonstrate that our approach better preserves downstream deep vision performance than traditional standard video coding.
MCML Authors
Computer Vision & Artificial Intelligence
A Perspective on Deep Vision Performance with Standard Image and Video Codecs.
CVPR 2024 - Workshop at the IEEE/CVF Conference on Computer Vision and Pattern Recognition. Seattle, WA, USA, Jun 17-21, 2024. DOI
Abstract
Resource-constrained hardware, such as edge devices or cell phones, often rely on cloud servers to provide the required computational resources for inference in deep vision models. However, transferring image and video data from an edge or mobile device to a cloud server requires coding to deal with network constraints. The use of standardized codecs, such as JPEG or H.264, is prevalent and required to ensure interoperability. This paper aims to examine the implications of employing standardized codecs within deep vision pipelines. We find that using JPEG and H.264 coding significantly deteriorates the accuracy across a broad range of vision tasks and models. For instance, strong compression rates reduce semantic segmentation accuracy by more than 80% in mIoU. In contrast to previous findings, our analysis extends beyond image and action classification to localization and dense prediction tasks, thus providing a more comprehensive perspective.
MCML Authors
Computer Vision & Artificial Intelligence
Finsler-Laplace-Beltrami Operators with Application to Shape Analysis.
CVPR 2024 - IEEE/CVF Conference on Computer Vision and Pattern Recognition. Seattle, WA, USA, Jun 17-21, 2024. DOI
Abstract
The Laplace-Beltrami operator (LBO) emerges from studying manifolds equipped with a Riemannian metric. It is often called the swiss army knife of geometry processing as it allows to capture intrinsic shape information and gives rise to heat diffusion, geodesic distances, and a mul-titude of shape descriptors. It also plays a central role in geometric deep learning. In this work, we explore Finsler manifolds as a generalization of Riemannian manifolds. We revisit the Finsler heat equation and derive a Finsler heat kernel and a Finsler-Laplace-Beltrami Operator (FLBO): a novel theoretically justified anisotropic Laplace-Beltrami operator (ALBO). In experimental evaluations we demon-strate that the proposed FLBO is a valuable alternative to the traditional Riemannian-based LBO and ALBOs for spa-tialfiltering and shape correspondence estimation. We hope that the proposed Finsler heat kernel and the FLBO will inspire further exploration of Finsler geometry in the Computer vision community.
MCML Authors
Flattening the Parent Bias: Hierarchical Semantic Segmentation in the Poincaré Ball.
CVPR 2024 - IEEE/CVF Conference on Computer Vision and Pattern Recognition. Seattle, WA, USA, Jun 17-21, 2024. DOI
Abstract
Hierarchy is a natural representation of semantic taxonomies, including the ones routinely used in image segmentation. Indeed, recent work on semantic segmentation reports improved accuracy from supervised training leveraging hierarchical label structures. Encouraged by these results, we revisit the fundamental assumptions behind that work. We postulate and then empirically verify that the reasons for the observed improvement in segmentation accuracy may be entirely unrelated to the use of the semantic hierarchy. To demonstrate this, we design a range of crossdomain experiments with a representative hierarchical approach. We find that on the new testing domains, a flat (non-hierarchical) segmentation network, in which the parents are inferred from the children, has superior segmentation accuracy to the hierarchical approach across the board. Complementing these findings and inspired by the intrinsic properties of hyperbolic spaces, we study a more principled approach to hierarchical segmentation using the Poincare ball model. The hyperbolic representation largely outperforms the previous (Euclidean) hierarchical approach as well and is on par with our flat Euclidean baseline in terms of segmentation accuracy. However, it additionally exhibits surprisingly strong calibration quality of the parent nodes in the semantic hierarchy, especially on the more challenging domains. Our combined analysis suggests that the established practice of hierarchical segmentation may be limited to in-domain settings, whereas flat classifiers generalize substantially better, especially if they are modeled in the hyperbolic space.
MCML Authors
Computer Vision & Artificial Intelligence
Cache Me if You Can: Accelerating Diffusion Models through Block Caching.
CVPR 2024 - IEEE/CVF Conference on Computer Vision and Pattern Recognition. Seattle, WA, USA, Jun 17-21, 2024. DOI GitHub
Abstract
Diffusion models have recently revolutionized the field of image synthesis due to their ability to generate photorealistic images. However, one of the major drawbacks of diffusion models is that the image generation process is costly. A large image-to-image network has to be applied many times to iteratively refine an image from random noise. While many recent works propose techniques to reduce the number of required steps, they generally treat the underlying denoising network as a black box. In this work, we investigate the behavior of the layers within the network and find that 1) the layers’ output changes smoothly over time, 2) the layers show distinct patterns of change, and 3) the change from step to step is often very small. We hypothesize that many layer computations in the denoising network are redundant. Leveraging this, we introduce block caching, in which we reuse outputs from layer blocks of previous steps to speed up inference. Furthermore, we propose a technique to automatically determine caching schedules based on each block’s changes over timesteps. In our experiments, we show through FID, human evaluation and qualitative analysis that Block Caching allows to generate images with higher visual quality at the same computational cost. We demonstrate this for different state-of-the-art models (LDM and EMU) and solvers (DDIM and DPM).
MCML Authors
Computer Vision & Artificial Intelligence
Text2Loc: 3D Point Cloud Localization from Natural Language.
CVPR 2024 - IEEE/CVF Conference on Computer Vision and Pattern Recognition. Seattle, WA, USA, Jun 17-21, 2024. DOI GitHub
Abstract
We tackle the problem of 3D point cloud localization based on a few natural linguistic descriptions and introduce a novel neural network, Text2Loc, that fully interprets the semantic relationship between points and text. Text2Loc follows a coarse-to-fine localization pipeline: text-submap global place recognition, followed by fine localization. In global place recognition, relational dynamics among each textual hint are captured in a hierarchical transformer with max-pooling (HTM), whereas a balance between positive and negative pairs is maintained using text-submap contrastive learning. Moreover, we propose a novel matching-free fine localization method to further refine the location predictions, which completely removes the need for complicated text-instance matching and is lighter, faster, and more accurate than previous methods. Extensive experiments show that Text2Loc improves the localization accuracy by up to 2 × over the state-of-the-art on the KITTI360Pose dataset.
MCML Authors
14.06.2024
Related
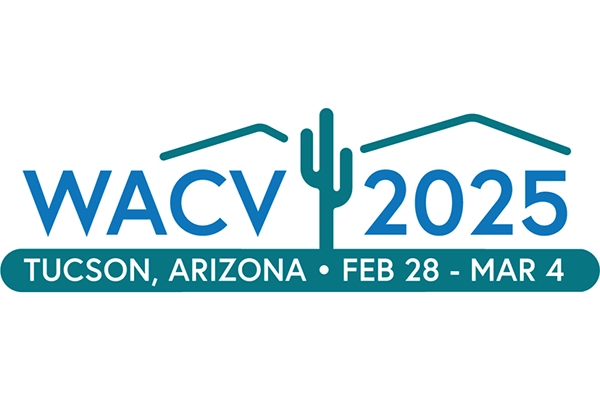
27.02.2025
MCML Researchers With Six Papers at WACV 2025
IEEE/CVF Winter Conference on Applications of Computer Vision (WACV 2025). Tucson, AZ, USA, 28.02.2025 - 04.03.2024
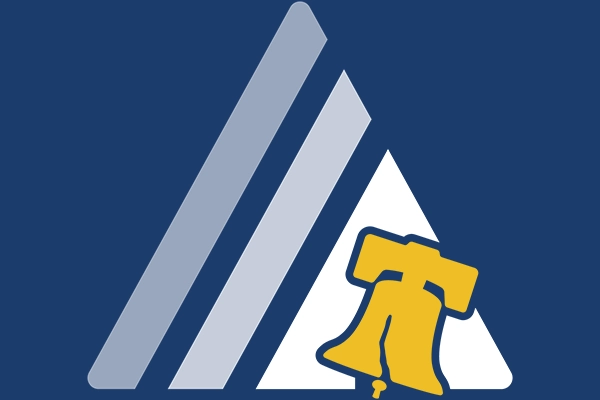
24.02.2025
MCML Researchers With Seven Papers at AAAI 2025
39th Conference on Artificial Intelligence (AAAI 2025). Philadelphia, PA, USA, 25.02.2025 - 04.03.2024
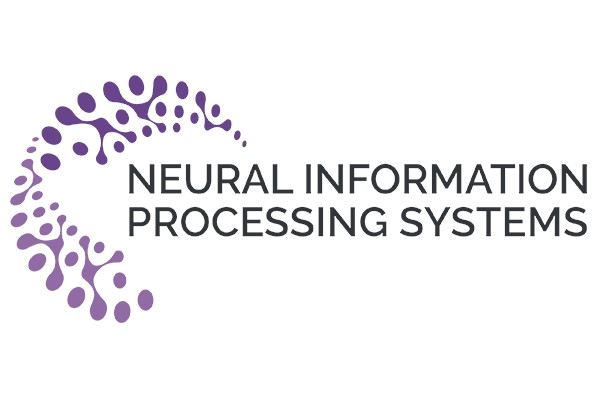
05.12.2024
MCML Researchers With 28 Papers at NeurIPS 2024
38th Conference on Neural Information Processing Systems (NeurIPS 2024). Vancouver, Canada, 10.12.2024 - 15.12.2024