12.03.2024
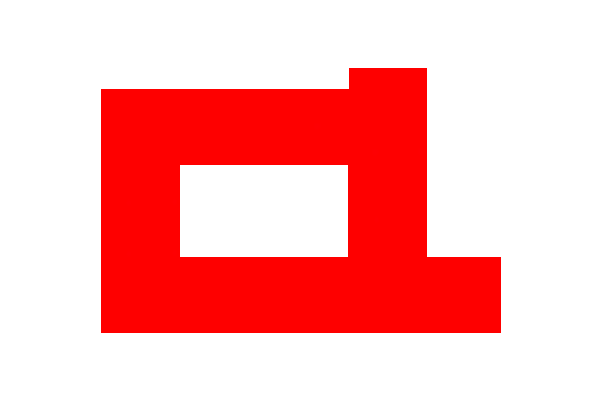
MCML researchers with ten papers at EACL 2024
18th Conference of the European Chapter of the Association for Computer Linguistics (EACL 2024). St. Julian's, Malta, 17.03.2024–22.03.2024
We are happy to announce that MCML researchers are represented with ten papers at EACL 2024:
Exploring the Robustness of Task-oriented Dialogue Systems for Colloquial German Varieties.
18th Conference of the European Chapter of the Association for Computational Linguistics (EACL 2024). St. Julians, Malta, Mar 17-22, 2024. URL.
Abstract
Mainstream cross-lingual task-oriented dialogue (ToD) systems leverage the transfer learning paradigm by training a joint model for intent recognition and slot-filling in English and applying it, zero-shot, to other languages.We address a gap in prior research, which often overlooked the transfer to lower-resource colloquial varieties due to limited test data.Inspired by prior work on English varieties, we craft and manually evaluate perturbation rules that transform German sentences into colloquial forms and use them to synthesize test sets in four ToD datasets.Our perturbation rules cover 18 distinct language phenomena, enabling us to explore the impact of each perturbation on slot and intent performance.Using these new datasets, we conduct an experimental evaluation across six different transformers.Here, we demonstrate that when applied to colloquial varieties, ToD systems maintain their intent recognition performance, losing 6% (4.62 percentage points) in accuracy on average. However, they exhibit a significant drop in slot detection, with a decrease of 31% (21 percentage points) in slot F1 score.Our findings are further supported by a transfer experiment from Standard American English to synthetic Urban African American Vernacular English.
MCML Authors
Interpreting Predictive Probabilities: Model Confidence or Human Label Variation?.
18th Conference of the European Chapter of the Association for Computational Linguistics (EACL 2024). St. Julians, Malta, Mar 17-22, 2024. URL.
Abstract
With the rise of increasingly powerful and user-facing NLP systems, there is growing interest in assessing whether they have a good representation of uncertainty by evaluating the quality of their predictive distribution over outcomes. We identify two main perspectives that drive starkly different evaluation protocols. The first treats predictive probability as an indication of model confidence; the second as an indication of human label variation. We discuss their merits and limitations, and take the position that both are crucial for trustworthy and fair NLP systems, but that exploiting a single predictive distribution is limiting. We recommend tools and highlight exciting directions towards models with disentangled representations of uncertainty about predictions and uncertainty about human labels.
MCML Authors
Order Effects in Annotation Tasks: Further Evidence of Annotation Sensitivity.
1st Workshop on Uncertainty-Aware NLP (UncertaiNLP 2024) at the 18th Conference of the European Chapter of the Association for Computational Linguistics (EACL 2024). St. Julians, Malta, Mar 17-22, 2024. URL.
Abstract
The data-centric revolution in AI has revealed the importance of high-quality training data for developing successful AI models. However, annotations are sensitive to annotator characteristics, training materials, and to the design and wording of the data collection instrument. This paper explores the impact of observation order on annotations. We find that annotators’ judgments change based on the order in which they see observations. We use ideas from social psychology to motivate hypotheses about why this order effect occurs. We believe that insights from social science can help AI researchers improve data and model quality.
MCML Authors
mPLM-Sim: Better Cross-Lingual Similarity and Transfer in Multilingual Pretrained Language Models.
Findings of the 18th Conference of the European Chapter of the Association for Computational Linguistics (EACL 2024). St. Julians, Malta, Mar 17-22, 2024. URL.
Abstract
Recent multilingual pretrained language models (mPLMs) have been shown to encode strong language-specific signals, which are not explicitly provided during pretraining. It remains an open question whether it is feasible to employ mPLMs to measure language similarity, and subsequently use the similarity results to select source languages for boosting cross-lingual transfer. To investigate this, we propose mPLM-Sim, a language similarity measure that induces the similarities across languages from mPLMs using multi-parallel corpora. Our study shows that mPLM-Sim exhibits moderately high correlations with linguistic similarity measures, such as lexicostatistics, genealogical language family, and geographical sprachbund. We also conduct a case study on languages with low correlation and observe that mPLM-Sim yields more accurate similarity results. Additionally, we find that similarity results vary across different mPLMs and different layers within an mPLM. We further investigate whether mPLM-Sim is effective for zero-shot cross-lingual transfer by conducting experiments on both low-level syntactic tasks and high-level semantic tasks. The experimental results demonstrate that mPLM-Sim is capable of selecting better source languages than linguistic measures, resulting in a 1%-2% improvement in zero-shot cross-lingual transfer performance.
MCML Authors
ToPro: Token-Level Prompt Decomposition for Cross-Lingual Sequence Labeling Tasks.
18th Conference of the European Chapter of the Association for Computational Linguistics (EACL 2024). St. Julians, Malta, Mar 17-22, 2024. URL.
Abstract
Prompt-based methods have been successfully applied to multilingual pretrained language models for zero-shot cross-lingual understanding. However, most previous studies primarily focused on sentence-level classification tasks, and only a few considered token-level labeling tasks such as Named Entity Recognition (NER) and Part-of-Speech (POS) tagging. In this paper, we propose Token-Level Prompt Decomposition (ToPro), which facilitates the prompt-based method for token-level sequence labeling tasks. The ToPro method decomposes an input sentence into single tokens and applies one prompt template to each token. Our experiments on multilingual NER and POS tagging datasets demonstrate that ToPro-based fine-tuning outperforms Vanilla fine-tuning and Prompt-Tuning in zero-shot cross-lingual transfer, especially for languages that are typologically different from the source language English. Our method also attains state-of-the-art performance when employed with the mT5 model. Besides, our exploratory study in multilingual large language models shows that ToPro performs much better than the current in-context learning method. Overall, the performance improvements show that ToPro could potentially serve as a novel and simple benchmarking method for sequence labeling tasks.
MCML Authors
Kardeş-NLU: Transfer to Low-Resource Languages with Big Brother’s Help – A Benchmark and Evaluation for Turkic Languages.
18th Conference of the European Chapter of the Association for Computational Linguistics (EACL 2024). St. Julians, Malta, Mar 17-22, 2024. URL.
Abstract
Cross-lingual transfer (XLT) driven by massively multilingual language models (mmLMs) has been shown largely ineffective for low-resource (LR) target languages with little (or no) representation in mmLM’s pretraining, especially if they are linguistically distant from the high-resource (HR) source language. Much of the recent focus in XLT research has been dedicated to LR language families, i.e., families without any HR languages (e.g., families of African languages or indigenous languages of the Americas). In this work, in contrast, we investigate a configuration that is arguably of practical relevance for more of the world’s languages: XLT to LR languages that do have a close HR relative. To explore the extent to which a HR language can facilitate transfer to its LR relatives, we (1) introduce Kardeş-NLU, an evaluation benchmark with language understanding datasets in five LR Turkic languages: Azerbaijani, Kazakh, Kyrgyz, Uzbek, and Uyghur; and (2) investigate (a) intermediate training and (b) fine-tuning strategies that leverage Turkish in XLT to these target languages. Our experimental results show that both - integrating Turkish in intermediate training and in downstream fine-tuning - yield substantial improvements in XLT to LR Turkic languages. Finally, we benchmark cutting-edge instruction-tuned large language models on Kardeş-NLU, showing that their performance is highly task- and language-dependent.
MCML Authors
EEVEE: An Easy Annotation Tool for Natural Language Processing.
18th Linguistic Annotation Workshop (LAW 2024) at the 18th Conference of the European Chapter of the Association for Computational Linguistics (EACL 2024). St. Julians, Malta, Mar 17-22, 2024. URL.
Abstract
Annotation tools are the starting point for creating Natural Language Processing (NLP) datasets. There is a wide variety of tools available; setting up these tools is however a hindrance. We propose EEVEE, an annotation tool focused on simplicity, efficiency, and ease of use. It can run directly in the browser (no setup required) and uses tab-separated files (as opposed to character offsets or task-specific formats) for annotation. It allows for annotation of multiple tasks on a single dataset and supports four task-types: sequence labeling, span labeling, text classification and seq2seq.
MCML Authors
Donkii: Can Annotation Error Detection Methods Find Errors in Instruction-Tuning Datasets?.
18th Linguistic Annotation Workshop (LAW 2024) at the 18th Conference of the European Chapter of the Association for Computational Linguistics (EACL 2024). St. Julians, Malta, Mar 17-22, 2024. URL.
Abstract
Instruction tuning has become an integral part of training pipelines for Large Language Models (LLMs) and has been shown to yield strong performance gains. In an orthogonal line of research, Annotation Error Detection (AED) has emerged as a tool for detecting quality problems in gold standard labels. So far, however, the application of AED methods has been limited to classification tasks. It is an open question how well AED methods generalize to language generation settings, which are becoming more widespread via LLMs. In this paper, we present a first and novel benchmark for AED on instruction tuning data: DONKII. It comprises three instruction-tuning datasets enriched with error annotations by experts and semi-automatic methods. We also provide a novel taxonomy of error types for instruction-tuning data. We find that all three datasets contain clear errors, which sometimes propagate directly into instruction-tuned LLMs. We propose four AED baselines for the generative setting and evaluate them extensively on the newly introduced dataset. Our results show that the choice of the right AED method and model size is indeed crucial and derive practical recommendations for how to use AED methods to clean instruction-tuning data.
MCML Authors
NNOSE: Nearest Neighbor Occupational Skill Extraction.
18th Conference of the European Chapter of the Association for Computational Linguistics (EACL 2024). St. Julians, Malta, Mar 17-22, 2024. URL.
Abstract
The labor market is changing rapidly, prompting increased interest in the automatic extraction of occupational skills from text. With the advent of English benchmark job description datasets, there is a need for systems that handle their diversity well. We tackle the complexity in occupational skill datasets tasks—combining and leveraging multiple datasets for skill extraction, to identify rarely observed skills within a dataset, and overcoming the scarcity of skills across datasets. In particular, we investigate the retrieval-augmentation of language models, employing an external datastore for retrieving similar skills in a dataset-unifying manner. Our proposed method, Nearest Neighbor Occupational Skill Extraction (NNOSE) effectively leverages multiple datasets by retrieving neighboring skills from other datasets in the datastore. This improves skill extraction without additional fine-tuning. Crucially, we observe a performance gain in predicting infrequent patterns, with substantial gains of up to 30% span-F1 in cross-dataset settings.
MCML Authors
Entity Linking in the Job Market Domain.
Findings of the 18th Conference of the European Chapter of the Association for Computational Linguistics (EACL 2024). St. Julians, Malta, Mar 17-22, 2024. URL.
Abstract
In Natural Language Processing, entity linking (EL) has centered around Wikipedia, but yet remains underexplored for the job market domain. Disambiguating skill mentions can help us get insight into the current labor market demands. In this work, we are the first to explore EL in this domain, specifically targeting the linkage of occupational skills to the ESCO taxonomy (le Vrang et al., 2014). Previous efforts linked coarse-grained (full) sentences to a corresponding ESCO skill. In this work, we link more fine-grained span-level mentions of skills. We tune two high-performing neural EL models, a bi-encoder (Wu et al., 2020) and an autoregressive model (Cao et al., 2021), on a synthetically generated mention–skill pair dataset and evaluate them on a human-annotated skill-linking benchmark. Our findings reveal that both models are capable of linking implicit mentions of skills to their correct taxonomy counterparts. Empirically, BLINK outperforms GENRE in strict evaluation, but GENRE performs better in loose evaluation (accuracy@k).
MCML Authors
12.03.2024
Related
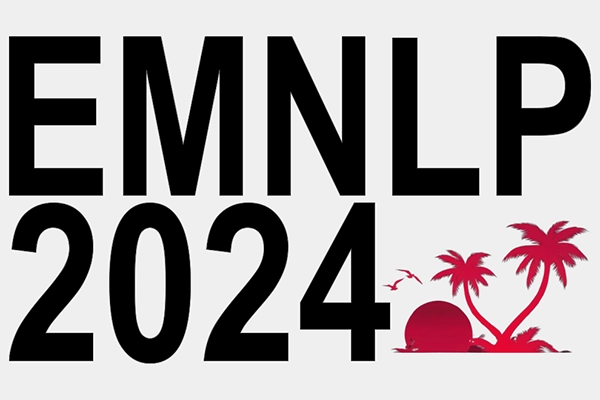
06.11.2024
MCML researchers with 20 papers at EMNLP 2024
Conference on Empirical Methods in Natural Language Processing (EMNLP 2024). Miami, FL, USA, 12.11.2024 - 16.11.2024
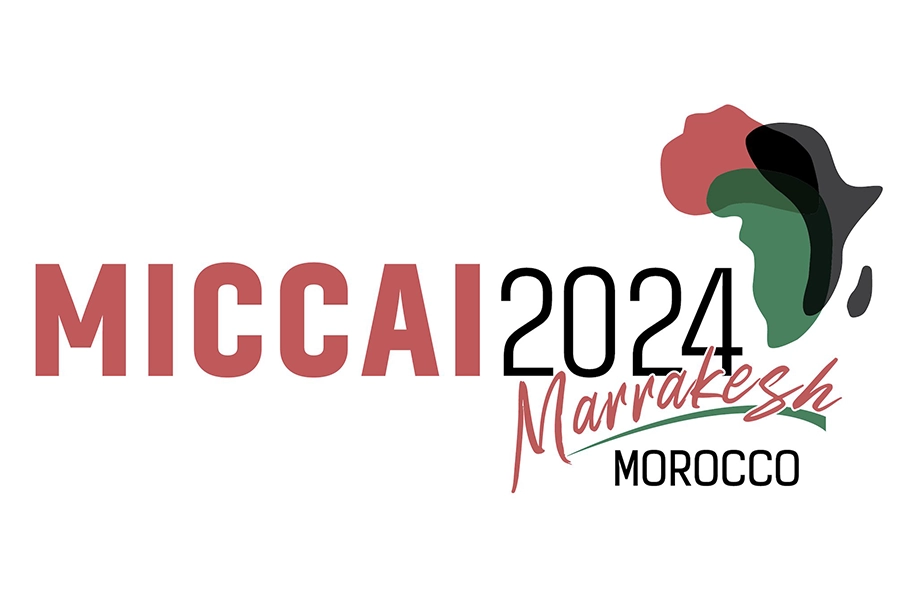
01.10.2024
MCML researchers with 16 papers at MICCAI 2024
27th International Conference on Medical Image Computing and Computer Assisted Intervention (MICCAI 2024). Marrakesh, Morocco, 06.10.2024 - 10.10.2024
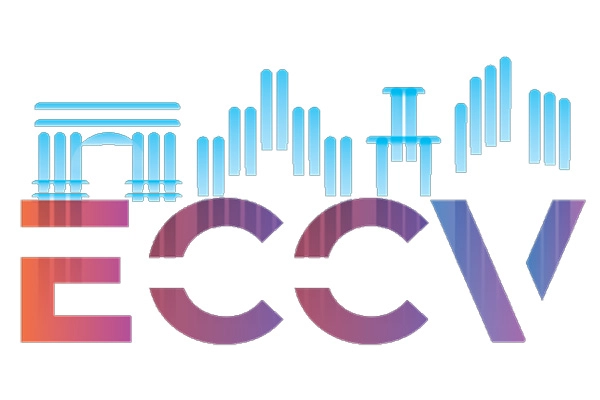
26.09.2024
MCML researchers with 18 papers at ECCV 2024
18th European Conference on Computer Vision (ECCV 2024). Milano, Italy, 29.09.2024 - 04.10.2024
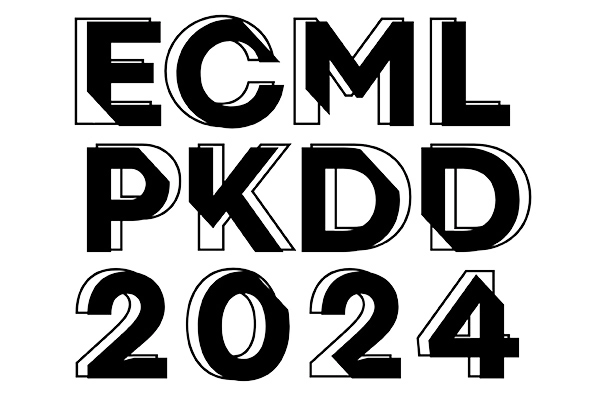
10.09.2024
MCML at ECML-PKDD 2024
We are happy to announce that MCML researchers are represented at ECML-PKDD 2024.
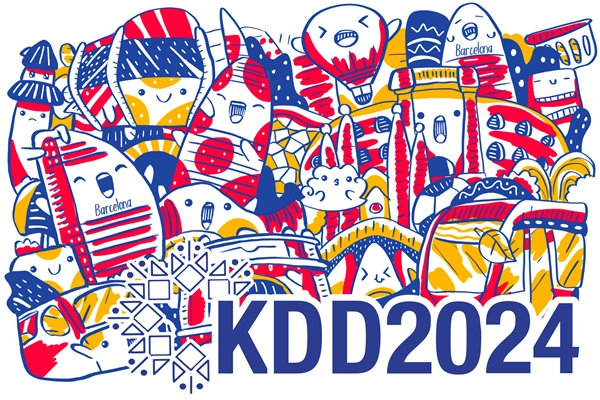
20.08.2024
MCML researchers with two papers at KDD 2024
30th ACM SIGKDD International Conference on Knowledge Discovery and Data (KDD 2024). Barcelona, Spain, 25.08.2024 - 29.08.2024