20
Jul
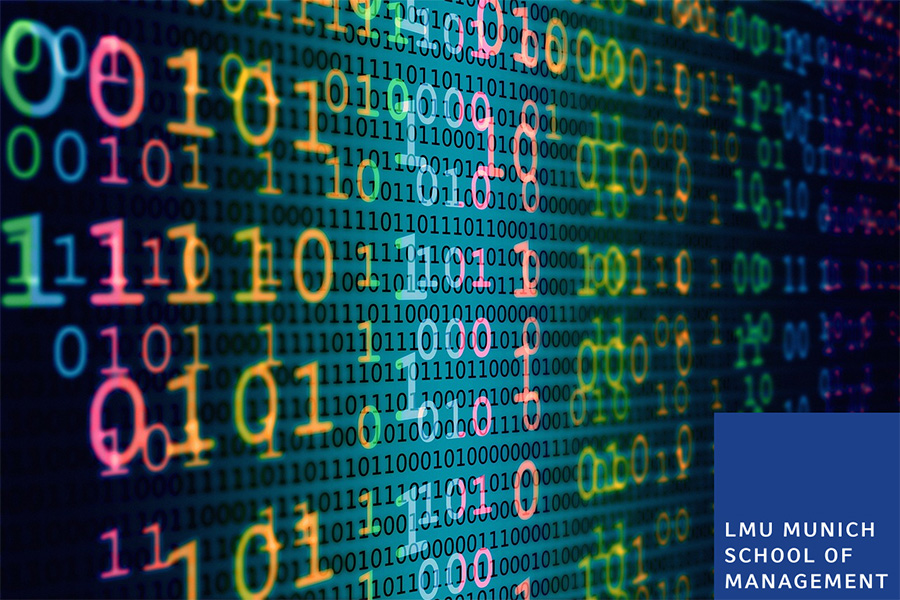
©geralt - pixabay.com
AI Keynote Series
Applied Causal Inference With Surrogate Representation
Lu Cheng, University of Illinois, Chicago
20.07.2023
6:00 pm - 7:30 pm
LMU Institute of AI in Management via zoom
The presence of unobserved confounders poses a fundamental challenge in causal inference using observational data. To tackle this challenge, the ignorable treatment assignment assumption is often employed, which assumes that all confounding bias can be captured by the background data. However, this assumption is overly strict and limits the practical applicability of causal inference. An alternative and less restrictive approach involves the use of proxy variables, or surrogates, that contain relevant information about the confounder. For instance, the socio-economic status can be approximated using a person’s income and zip code. This talk will focus on the practical aspects of causal inference and use three examples to explore how surrogate representation can be applied in domains like online reviews, fake news, and recommender systems.