08
Feb
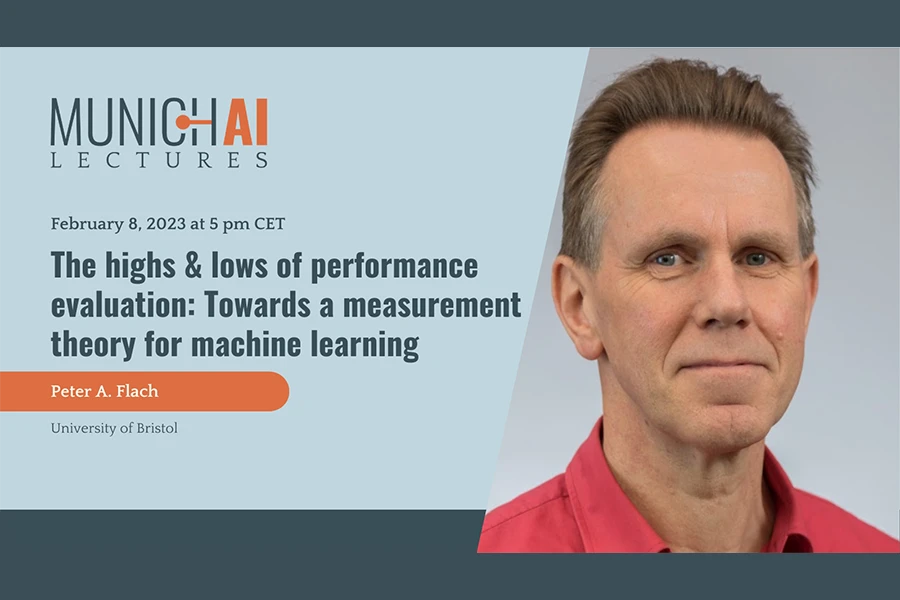
Munich AI Lectures
The Highs and Lows of Performance Evaluation: Towards a Measurement Theory for Machine Learning
Peter Flach, University of Bristol
08.02.2023
5:00 pm - 7:00 pm
Livestream on YouTube
The understanding of performance evaluation measures for machine-learned classifiers has progressed, but gaps persist, leading to questionable evaluation practices. This raises concerns about the trustworthiness of systems utilizing these algorithms. To address this, a proper measurement theory of machine learning is proposed, akin to measurement theory in other domains. This theory would explore relevant concatenation operations in data science and AI and their implications for measurement scales. The need for latent-variable models is highlighted to capture key properties like classification ability and dataset difficulty, enabling causal inferences in machine learning experiments.