04
May
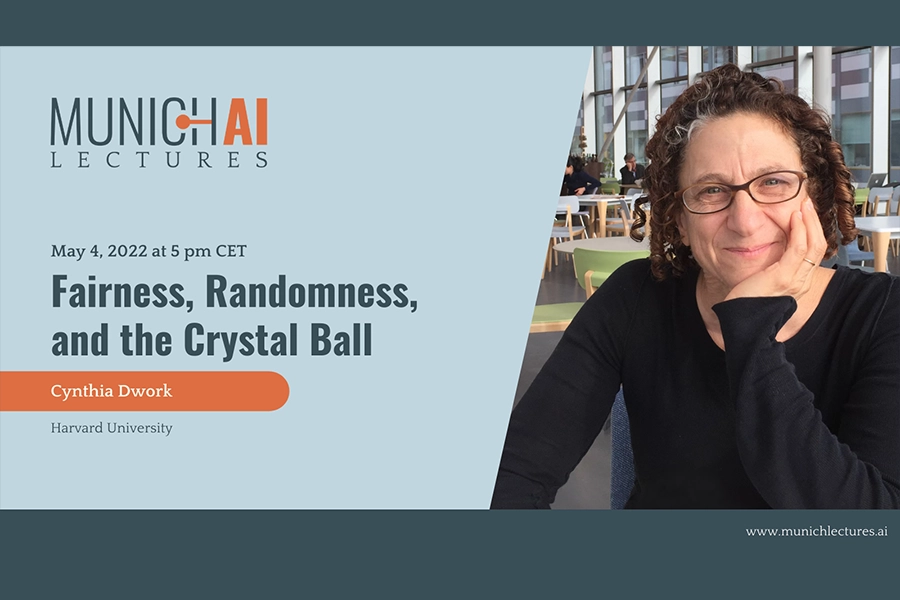
Munich AI Lectures
Fairness, Randomness, And the Crystal Ball
Cynthia Dwork, Harvard University
04.05.2022
5:00 pm - 6:30 pm
Livestream on YouTube
Prediction algorithms score individuals, or individual instances, assigning to each one a number in the range from 0 to 1. That score is often interpreted as a probability: What are the chances that this loan will be repaid? How likely is this tumor to metastasize? A key question lingers: What is the “probability” of a non-repeatable event? This is the defining problem of AI. Without a satisfactory answer, how can we even specify what we want from an ideal algorithm?
This talk will introduce ‘outcome indistinguishability’ — a desideratum with roots in computational complexity theory. We will situate the concept within the 10-year history of the theory of algorithmic fairness, and spell out directions for future research.