14
May
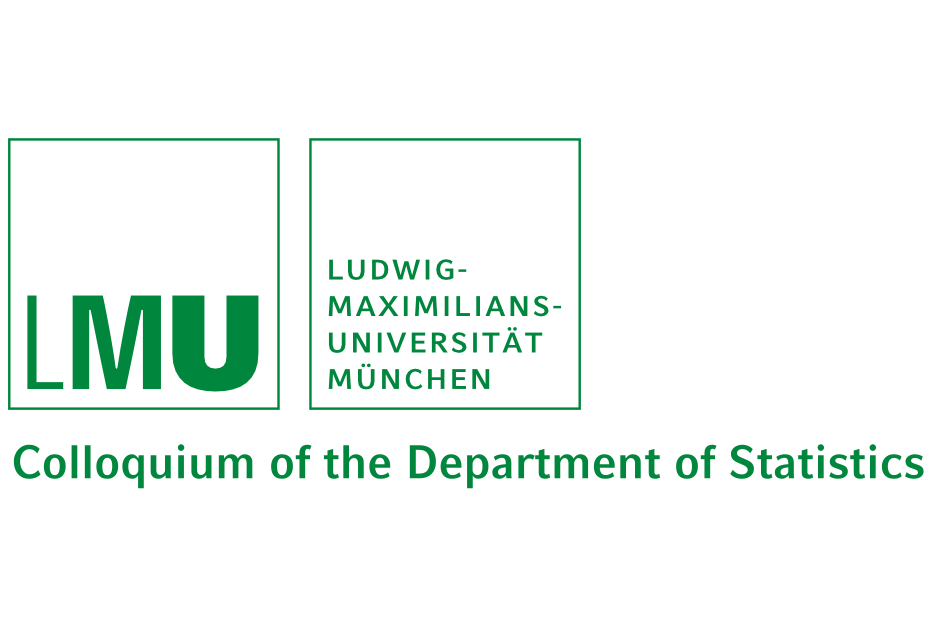
Colloquium
Robustness in Semiparametric Statistics
Rajen Shah, University of Cambridge
14.05.2025
4:15 pm - 5:45 pm
LMU Department of Statistics and via zoom
Given that all models are wrong, it is important to understand the performance of methods when the settings for which they have been designed are not met, and to modify them where possible so they are robust to these sorts of departures from the ideal. We present two examples with this broad goal in mind.
We first look at a classical case of model misspecification in (linear) mixed effect models for grouped data. Existing approaches estimate linear model parameters through weighted least squares, with optimal weights (given by the inverse covariance of the response, conditional on the covariates) typically estimated by maximising a (restricted) likelihood from random effects modelling or by using generalised estimating equations. We introduce a new ‘sandwich loss’ whose population minimiser coincides with the weights of these approaches when the parametric forms for the conditional covariance are well-specified, but can yield arbitrarily large improvements when they are not.
The starting point of our second vignette is the recognition that semiparametric efficient estimation can be hard to achieve in practice: estimators that are in theory efficient may require unattainable levels of accuracy for the estimation of complex nuisance functions. As a consequence, estimators deployed on real datasets are often chosen in a somewhat ad hoc fashion, and may suffer high variance. We study this gap between theory and practice in the context of a broad collection of semiparametric regression models that includes the generalised partially linear model. We advocate using estimators that are robust in the sense that they enjoy root n consistent uniformly over a sufficiently rich class of distributions characterised by certain conditional expectations being estimable by user-chosen machine learning methods. We show that even asking for locally uniform estimation within such a class narrows down possible estimators to those parametrised by certain weight functions and develop a new random forest-based estimation scheme to estimate the optimal weights. We demonstrate the effectiveness of the resulting estimator in a variety of semiparametric settings on simulated and real-world data.