23
Apr
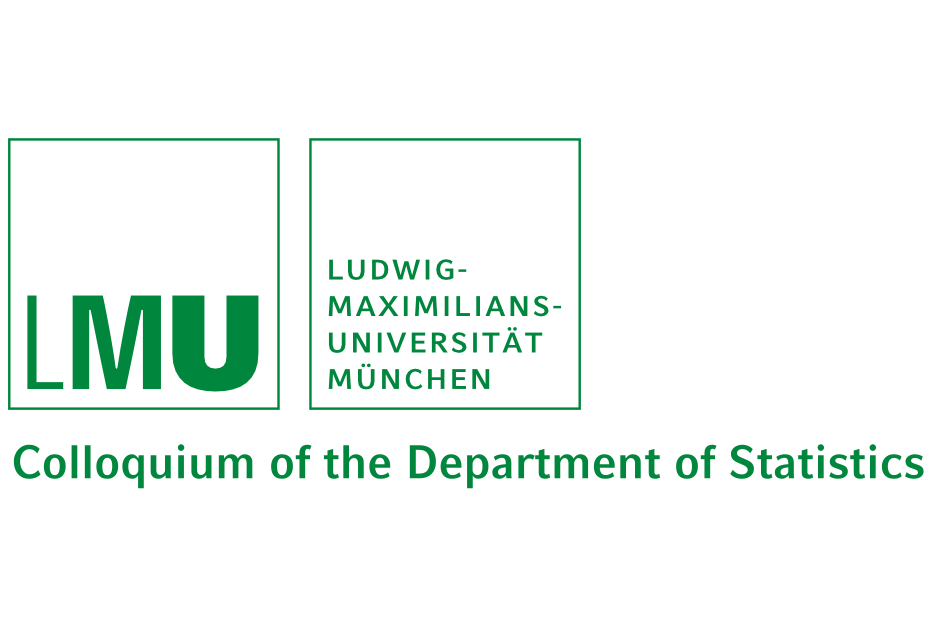
Colloquium
Story-Led Causal Inference
Jessica Young, Harvard University
23.04.2025
4:15 pm - 5:45 pm
LMU Department of Statistics and via zoom
The modern causal inference literature has expressed a deep divide over the nature of the so-called consistency condition. Consistency is popularly characterized as the condition that counterfactual/potential outcomes indexed by a hypothetical intervention of interest may be equated to measured outcomes among those whose data is consistent with that intervention. One “camp” argues consistency is a definition/axiom under the investigator’s causal model while the other insists consistency is an assumption that fails when there are “multiple versions of treatment” rendering the causal target/estimand ill-defined. In this talk, I consider a third perspective on the consistency question that falls out of prioritizing the details of an investigator’s underlying story about what is actually of interest over data that happens to be available for analysis. I will illustrate the benefits of this “story-led approach” using an example of an underlying story behind studies of the “protective effect of cancer on dementia.”