06
Feb
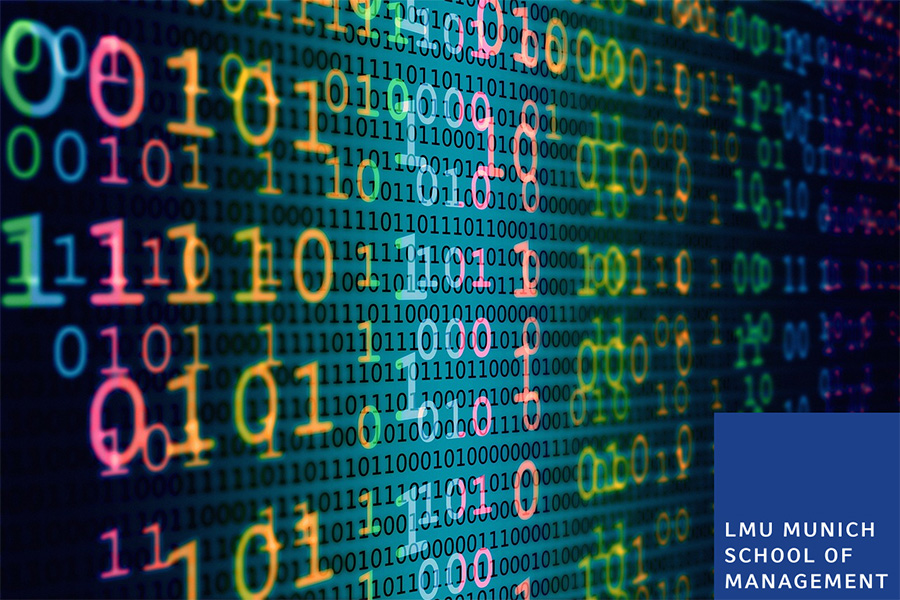
©geralt - pixabay.com
Lecture
Causal Discovery: What Can We Learn From Heterogeneous Noise?
Alexander Marx, TU Dortmund
06.02.2025
11:00 am - 12:00 pm
LMU Munich, Ludwigstr. 28 VG/II; Room 211b
Causal discovery aims to learn causal networks, i.e., directed acyclic graphs (DAGs), from observational data. Although the problem is not feasible in the most general form, as it is not possible to infer causal relations from pure correlations, we can define structural assumptions about the functional relations underlying our observed system to ease this task. One of the earliest works in this direction assumes that all causal relationships are linear and all noise sources can be characterised as non-Gaussian. Under these assumptions we can provably learn causal graphs from observational data—however, they are quite restrictive. In this talk, we relax these assumptions and show that the broader class of location-scale or heteroscedastic noise models (LSNMs) allows for learning causal graphs up to pathological cases. Further, we emphasise that estimation of such functions also plays a key role in causal discovery and discuss several estimators for LSNMs ranging from consistent estimators to Bayesian neural networks.
Organized by:
LMU Munich School of Management
Related
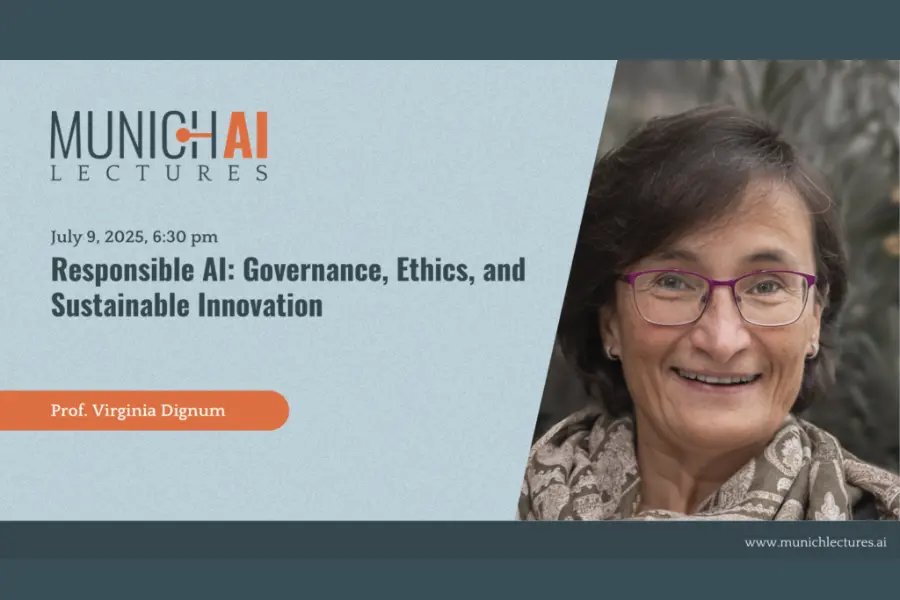
Munich AI Lectures • 09.07.2025 • Plenarsaal of the Bavarian Academy of Sciences and Humanities (BAdW), Alfons-Goppel-Straße 11, 80539 Munich
Foundational Methods for Foundation Models for Scientific Machine Learning
The Munich AI Lectures on March 26, 2025, will feature Michael Mahoney and cover foundational methods for SciML foundation models.
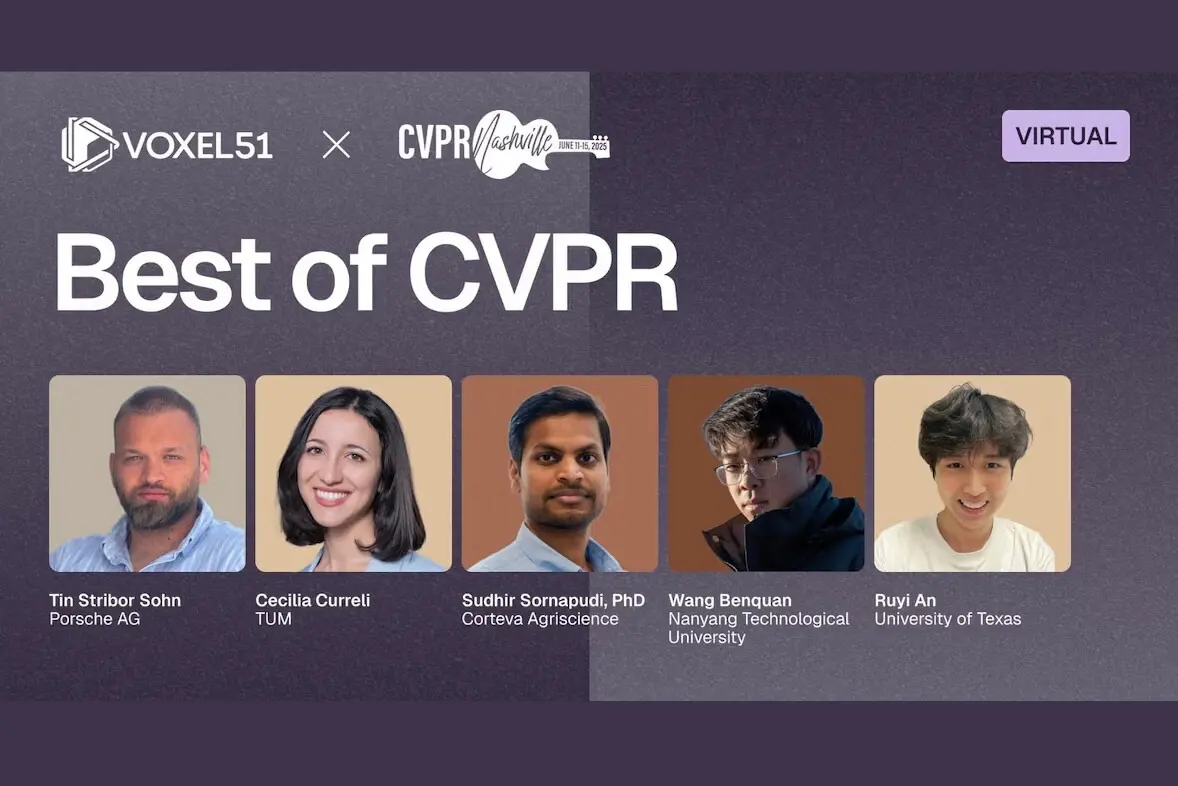
©voxel51
Meetup • 09.07.2025 • Online
The Best of CVPR 2025 Series
Voxel51’s “Best of CVPR” event features top 2025 computer vision research, including Cecilia Curreli presenting on human motion prediction.
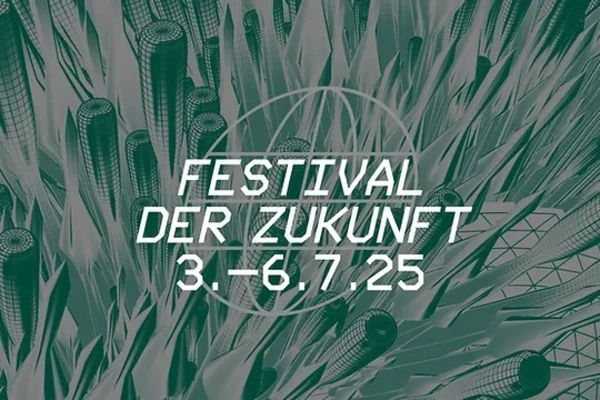
©1E9
Festival • 03.07.2025 - 06.07.2025 • Deutsches Museum, Munich
Festival of the Future 2025
Festival of the Future 2025 takes place from July 3 to 6, 2025, covering AI, sustainability, and space, with Björn Ommer as a speaker.
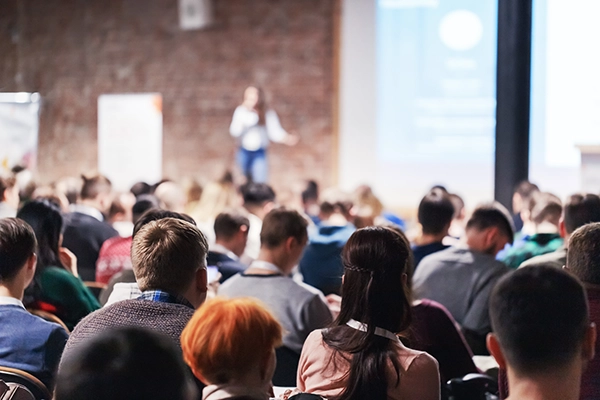
©Feel-good-studio-stock-adobe.com
Lecture • 26.06.2025 • CAS Seestraße 13 80802 Munich
Bayesian Insights on Polarization and Disagreement
As part of the CAS Research Focus, Leah Henderson explores societal polarization using Bayesian models, offering insights on handling disagreements.