09
Jan
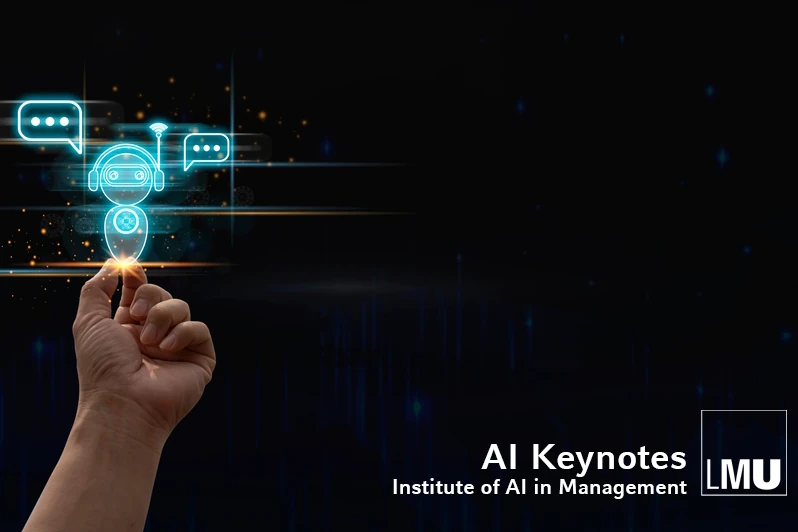
©jittawit.21 - stock.adobe.com
AI Keynote Series
Experimental Designs for a/B Testing in Marketplaces
Chengchun Shi, Department of Statistics, London School of Economics and Political Science
09.01.2025
12:00 pm - 1:30 pm
Online via Zoom
Time series experiments, in which experimental units receive a sequence of treatments over time, are frequently employed in many technological companies to evaluate the performance of a newly developed policy, product, or treatment relative to a baseline control. Many existing A/B testing solutions assume a fully observable experimental environment that satisfies the Markov condition, which often does not hold in practice.
This paper studies the optimal design for A/B testing in partially observable environments. We introduce a controlled (vector) autoregressive moving average model to capture partial observability. We introduce a small signal asymptotic framework to simplify the analysis of asymptotic mean squared errors of average treatment effect estimators under various designs. We develop two algorithms to estimate the optimal design: one utilizing constrained optimization and the other employing reinforcement learning. We demonstrate the superior performance of our designs using a dispatch simulator and two real datasets from a ride-sharing company.
Organized by:
Institute of AI in Management LMU Munich