11
Dec
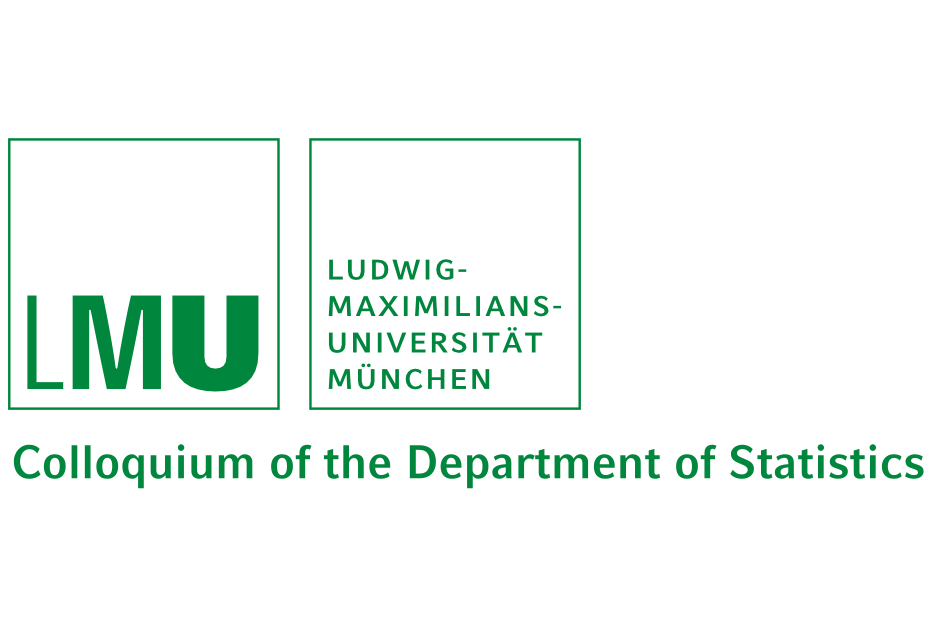
Colloquium
Assumption-Lean (Causal) Modeling
Stijn Vansteelandt, Ghent University
11.12.2024
4:00 pm - 6:00 pm
LMU Department of Statistics and via zoom
Traditional inference in (semi-)parametric models, such as generalized linear models, typically assumes that the model is correctly specified and pre-determined. However, this approach is increasingly unsatisfactory because models are often selected adaptively based on the data, introducing unacknowledged uncertainty. Moreover, these models rarely represent a known ground truth, making standard inferences prone to model misspecification bias and limiting their ability to fully leverage the information in the data. While significant progress has been made in recent decades through advancements in debiased machine learning and targeted learning, these methods are inherently model-free, which can limit their applicability and interpretability in complex settings.
Assumption-lean modeling, introduced by Vansteelandt and Dukes (2022) in a discussion paper in the Journal of the Royal Statistical Society – Series B, rethinks this trade-off between model correctness and parsimony. This framework starts with data-adaptive outcome predictions and then projects these onto specific model parameters. The projection is designed to ensure that the resulting parameters are interpretable and meaningful, even when the underlying model is misspecified. By moreover leveraging debiased machine learning techniques, assumption-lean modeling achieves minimal bias, maximal interpretability, and valid confidence intervals that account for both model misspecification and uncertainty.
In this talk, I will introduce the core ideas behind assumption-lean modeling, focusing on generalized linear models to ensure accessibility. Additionally, I will explore its extensions to causal inference and time-to-event data, highlighting recent developments aimed at balancing efficiency with interpretability.
Organized by:
Department of Statistics LMU Munich