18
Jul
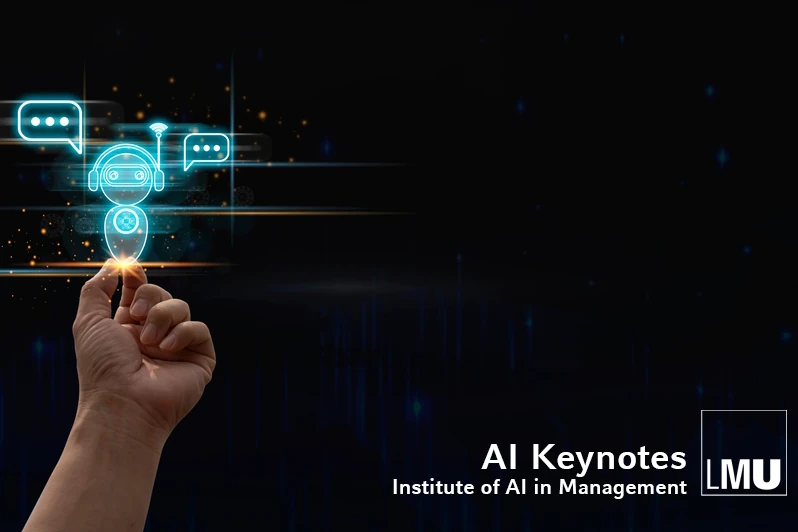
©jittawit.21 - stock.adobe.com
AI Keynote Series
Representation Learning: A Causal Perspective
Yixin Wang, University of Michigan
18.07.2024
5:00 pm - 6:30 pm
Online via Zoom
Representation learning aims to create low-dimensional representations that capture essential features of high-dimensional data, such as images and texts. Ideally, these representations should efficiently capture meaningful, non-spurious features and be disentangled for interpretability. However, defining and enforcing these qualities is challenging.
In this talk, a causal perspective on representation learning is presented. The desiderata for effective representation learning are formalized using counterfactual concepts, which lead to metrics and algorithms designed to achieve efficient, non-spurious, and disentangled representations. The talk covers the theoretical foundations of the proposed algorithm and demonstrates its performance in both supervised and unsupervised settings.
Organized by:
Institute of AI in Management LMU Munich