03
Jun
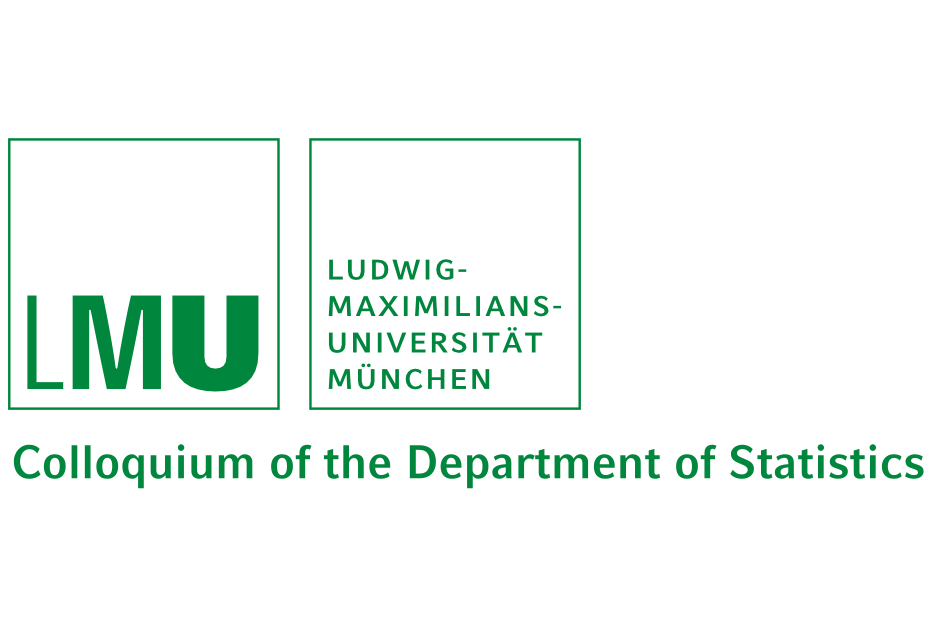
Colloquium
Explainable Methods for Reinforcement Learning
Jasmina Gajcin, Trinity College Dublin
03.06.2024
4:15 pm - 5:45 pm
LMU Department of Statistics and via zoom
Deep reinforcement learning (DRL) algorithms have been successfully devel- oped for many high-risk real-life tasks in many fields such as autonomous driving, healthcare and finance. However, these algorithms rely on neural networks, making their decisions difficult to understand and interpret.
In this talk, I will cover some of the main challenges for developing explainable DRL methods, especially focusing on the difference between supervised and reinforcement learning from the perspective of explainability. Additionally, a part of this talk will be focused on counterfactual explanations in RL. Counterfactual explanations are a powerful explanation method and can explain outcomes by contrasting them with similar events which led to a different outcome. The talk will delve into how counterfactual explanations can be utilized in an RL setting.