15
May
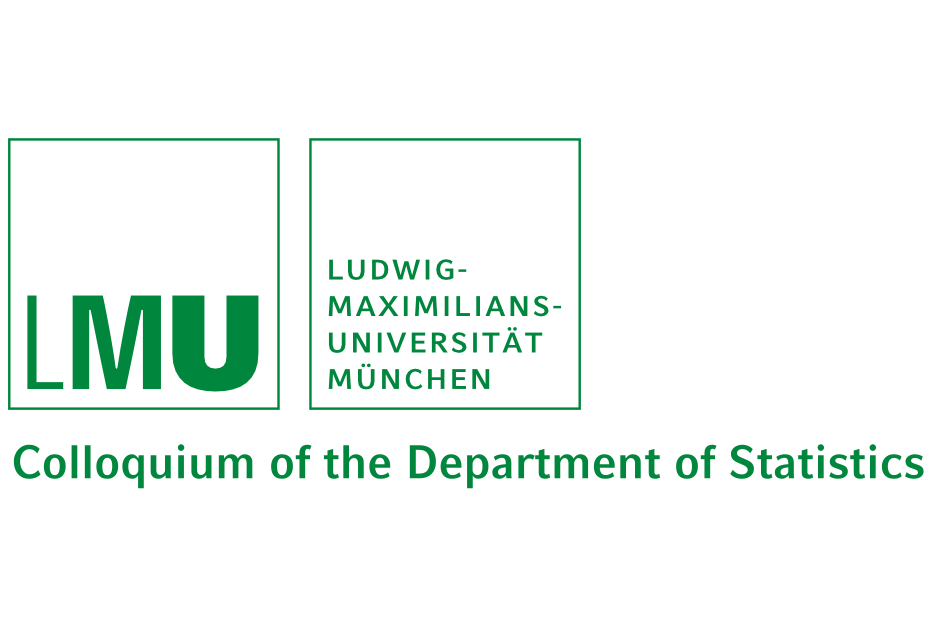
Optimal convex M-estimation via score matching
Richard Samworth, Cambridge University
15.05.2024
5:00 pm - 6:30 pm
LMU Department of Statistics and via zoom
In the context of linear regression, we construct a data-driven convex loss function with respect to which empirical risk minimisation yields opti- mal asymptotic variance in the downstream estimation of the regression coefficients. Our semiparametric approach targets the best decreasing approximation of the derivative of the log-density of the noise distribution. At the population level, this fitting process is a nonparametric extension of score matching, corresponding to a log-concave projection of the noise distribution with respect to the Fisher divergence. The procedure is com- putationally efficient, and we prove that our procedure attains the minimal asymptotic covariance among all convex M-estimators. As an example of a non-log-concave setting, for Cauchy errors, the optimal convex loss function is Huber-like, and our procedure yields an asymptotic efficiency greater than 0.87 relative to the oracle maximum likelihood estimator of the regression coefficients that uses knowledge of this error distribution; in this sense, we obtain robustness without sacrificing much efficiency.
Related
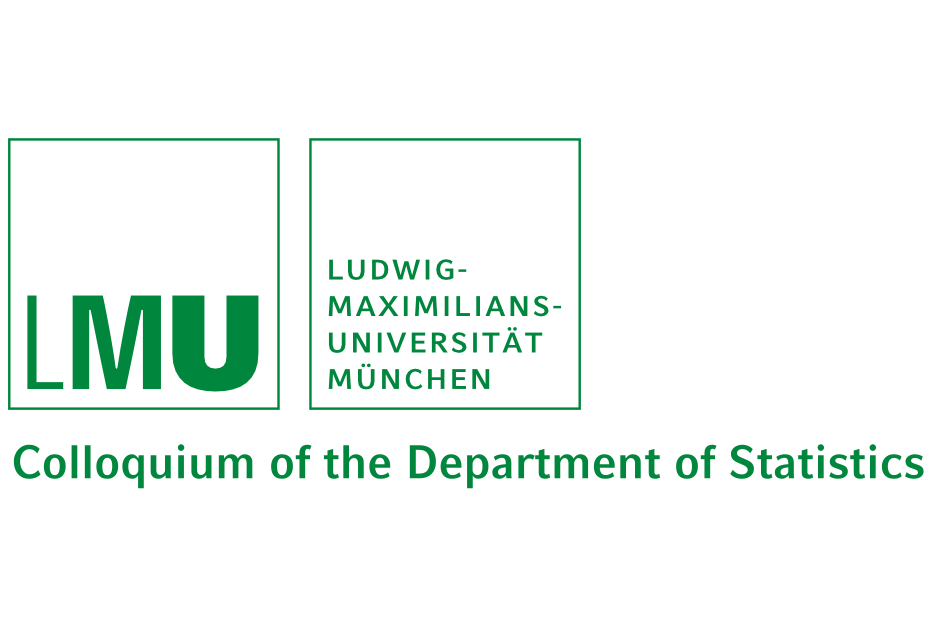
Colloquium • 16.07.2024 • LMU Department of Statistics and via zoom
Privacy, Data Privacy, and Differential Privacy
Colloquium at the LMU Department of Statistics with James Bailie from Harvard University.
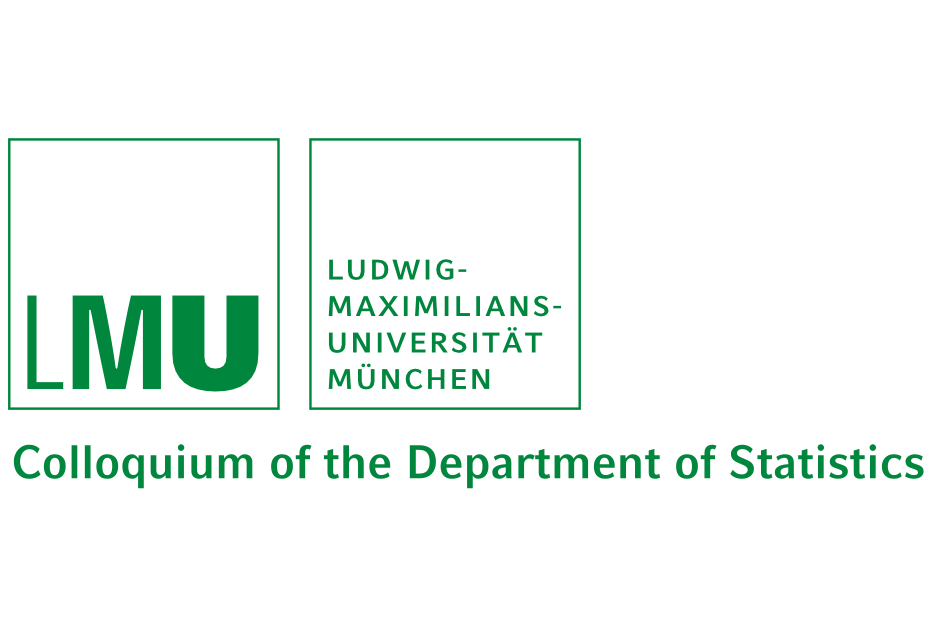
Colloquium • 10.07.2024 • LMU Department of Statistics and via zoom
Variational Learning for Large Deep Networks
Colloquium at the LMU Department of Statistics with Thomas Möllenhoff from RIKEN, Tokyo.
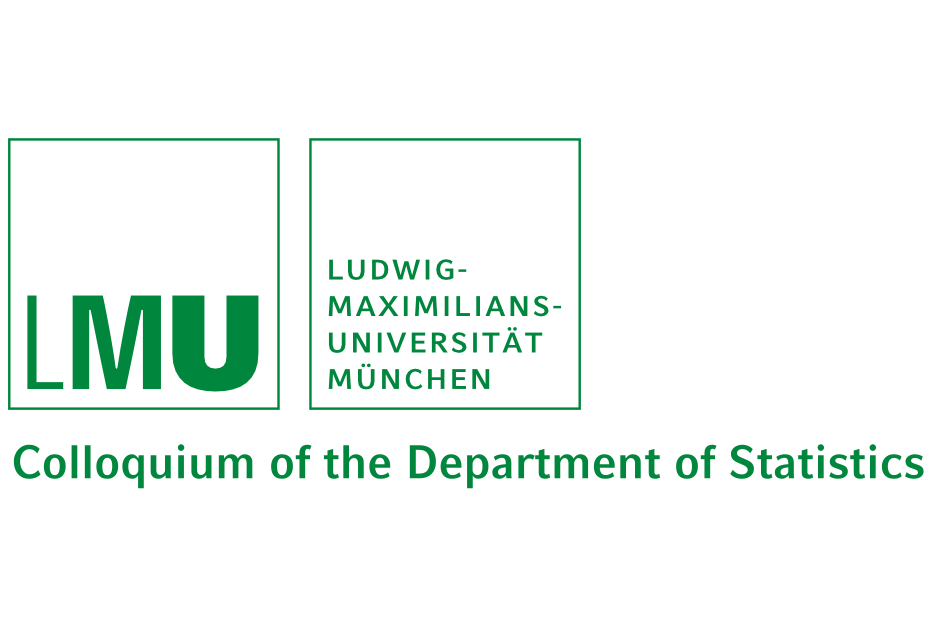
Colloquium • 03.07.2024 • LMU Department of Statistics and via zoom
Can today’s intention to treat have a causal effect on tomorrow’s hazard function?
Colloquium at the LMU Department of Statistics with Jan Beyersmann, University of Ulm.
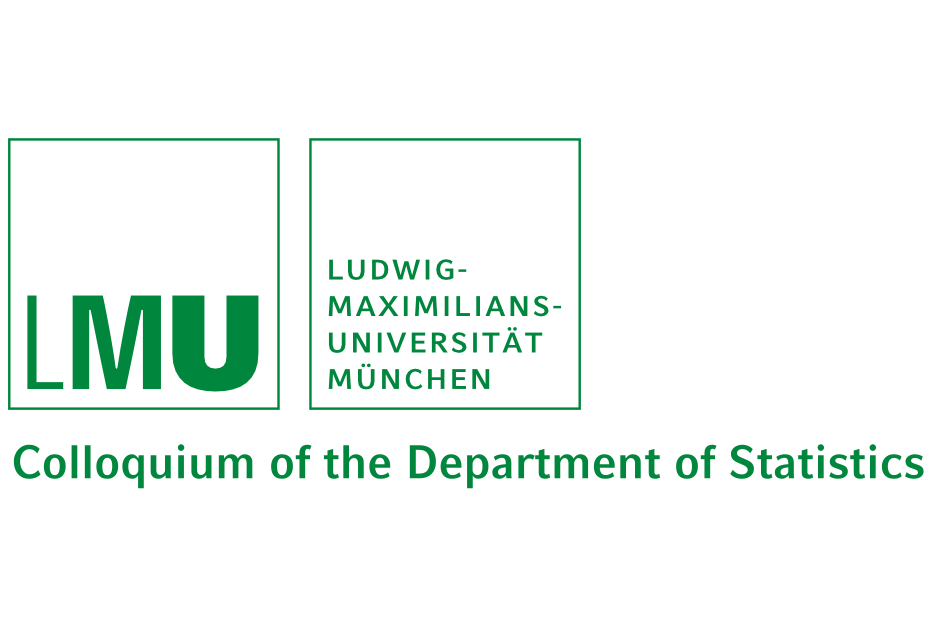
Colloquium • 26.06.2024 • LMU Department of Statistics and via zoom
The Complexities of Differential Privacy for Survey Data
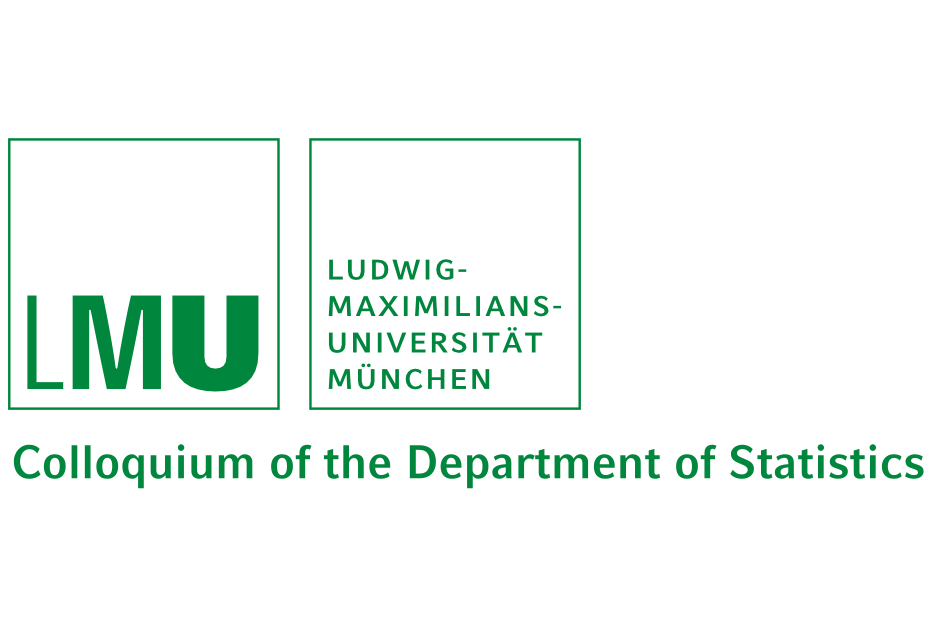
Colloquium • 19.06.2024 • LMU Department of Statistics and via zoom
Resampling-based inference for the average treatment effect in observational studies with competing risks
This talk explores three resampling methods to construct valid confidence intervals and bands for treatment effect estimation in competing risks studies.