21
Feb
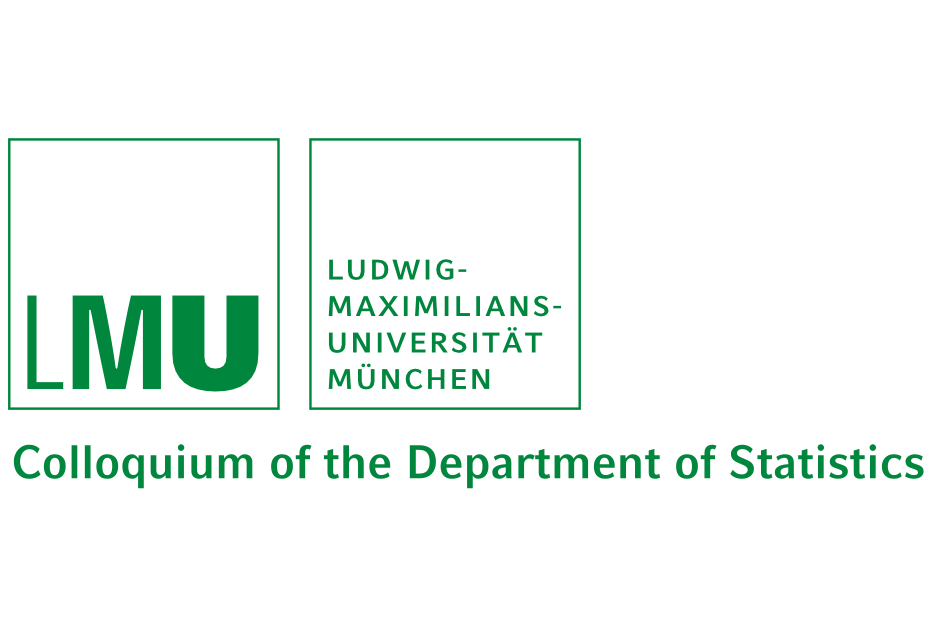
Large-scale pretraining: the nitty-gritty details
Robert Baldock, Aleph Alpha
21.02.2024
2:15 pm - 3:45 pm
LMU Department of Statistics and via zoom
This talk will give a rare close-up of the nitty-gritty details that go into training large-scale LLMs. In the autumn of 2023, Aleph Alpha Research Lab prepared to train their next generation of large language models, which are training now.
In this talk, Robert Baldock will chronicle their learnings from this process. In particular, he will describe their experiments to optimise the architecture and pretraining, their optimal scaling study, insights about efficient and numerically stable parallel training, tokenizer construction, and the preparation of the large-scale web-crawl dataset.
Related
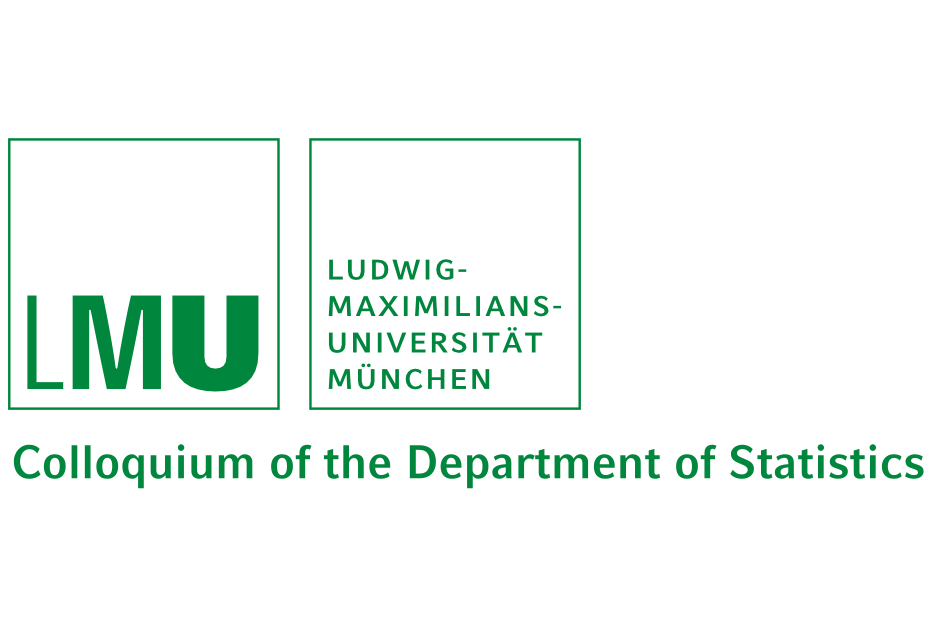
Colloquium • 16.07.2024 • LMU Department of Statistics and via zoom
Privacy, Data Privacy, and Differential Privacy
Colloquium at the LMU Department of Statistics with James Bailie from Harvard University.
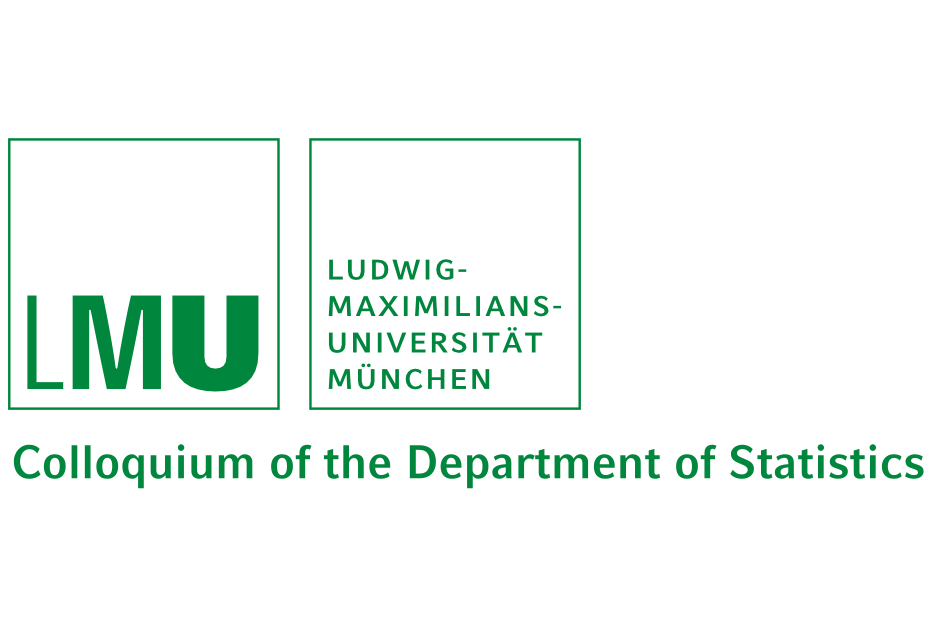
Colloquium • 10.07.2024 • LMU Department of Statistics and via zoom
Variational Learning for Large Deep Networks
Colloquium at the LMU Department of Statistics with Thomas Möllenhoff from RIKEN, Tokyo.
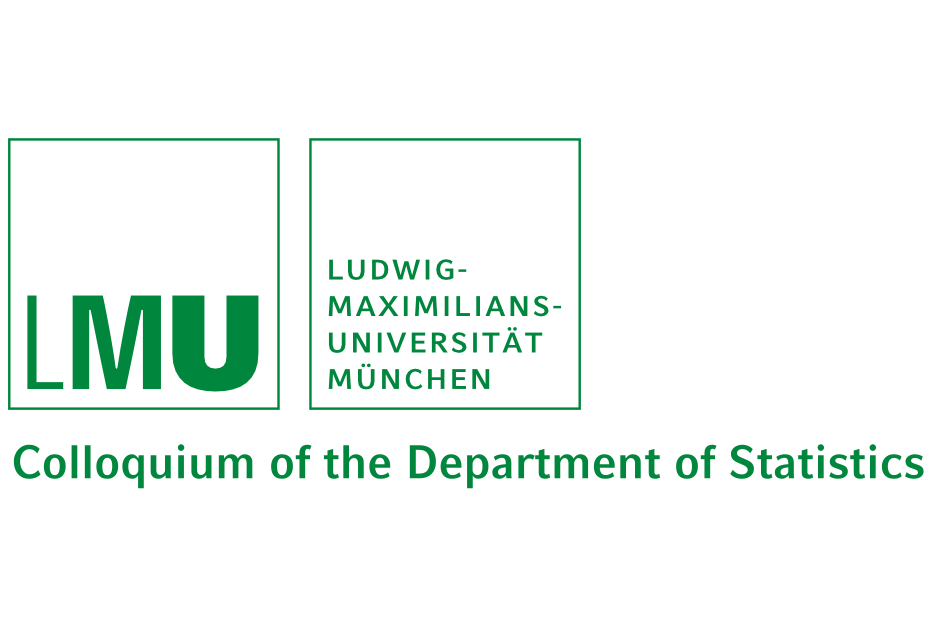
Colloquium • 03.07.2024 • LMU Department of Statistics and via zoom
Can today’s intention to treat have a causal effect on tomorrow’s hazard function?
Colloquium at the LMU Department of Statistics with Jan Beyersmann, University of Ulm.
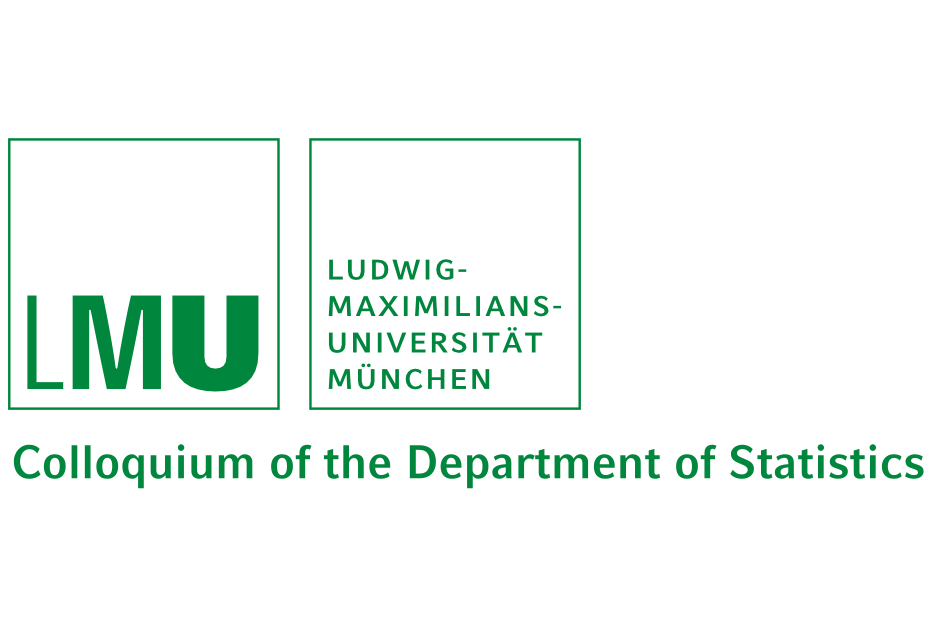
Colloquium • 26.06.2024 • LMU Department of Statistics and via zoom
The Complexities of Differential Privacy for Survey Data
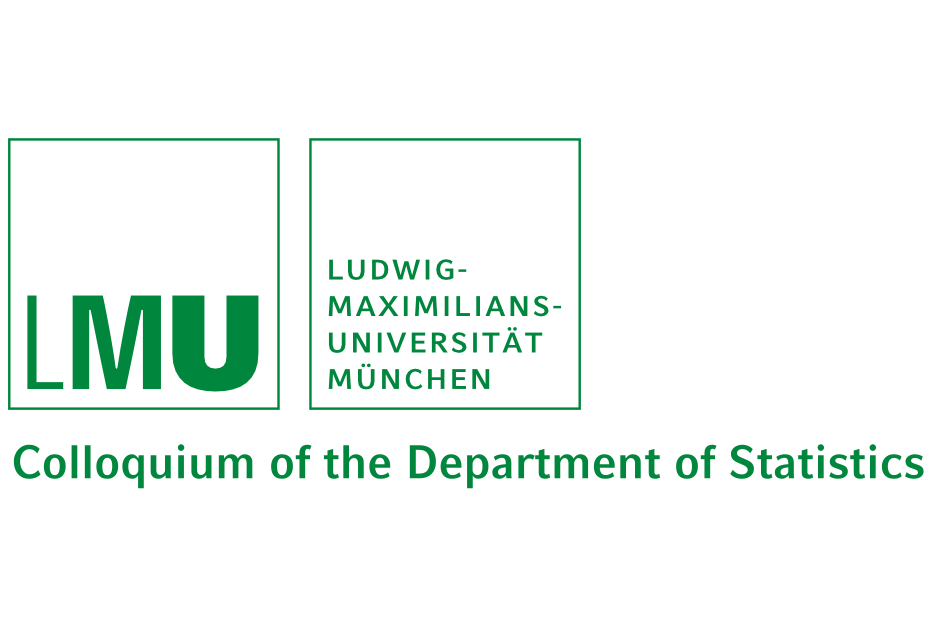
Colloquium • 19.06.2024 • LMU Department of Statistics and via zoom
Resampling-based inference for the average treatment effect in observational studies with competing risks
This talk explores three resampling methods to construct valid confidence intervals and bands for treatment effect estimation in competing risks studies.